Deep learning radiomics for focal liver lesions diagnosis on long-range contrast-enhanced ultrasound and clinical factors
Introduction
Liver cancer is one of the most aggressive and frequent malignant tumors globally, with approximately 841,000 new cases and 782,000 deaths per year, representing a significant challenge to human health, especially in China (1,2). In clinical procedures, the combination of alpha fetoprotein (AFP) and imaging examination play a crucial role in early screening and diagnosis (3-6). Contrast-enhanced ultrasound (CEUS) plays an important role in the differential diagnosis of liver cancer from focal liver lesions (FLLs). Therefore, it has been recommended as one of the four imaging methods for the diagnosis of liver cancer (5,6). Studies show that compared with computed tomography (CT) and magnetic resonance imaging (MRI), it has the advantages of superior safety, fewer allergic reactions (7), lower cost, and real-time imaging (8,9). The diagnostic accuracy of CEUS can be higher or comparable to that of spiral CT, especially in characterizing <3 cm FLLs (10). However, the performance of CEUS is more complicated due to diverse types of FLLs, and significantly affects the application and popularization of CEUS in the differential diagnosis of FLLs (11-13). Particularly, routine clinical factors, such as hepatitis, AFP, and tumor markers, often affect the physiological and pathological changes of the liver, and should be taken into consideration during the analysis of CEUS in diagnosing liver cancer (8,14,15). However, they are often ignored, leading to unnecessary misdiagnosis and missed diagnosis. Simultaneously, comprehensive image analysis is challenging and requires tedious manual annotation by radiologists.
Deep learning (DL) with convolutional neural networks (CNNs) can automatically extract the hierarchy features of input data (16). It has been widely used for the analysis of FLLs in US (17,18), CT (19-22), and MRI (23-25). Former researchers have investigated computer technology in CEUS analysis, such as time intensity curves (TICs) (26-28) or intensity-based features (29,30). However, these features are relatively simple. In recent years, previous attempts have been made to algorithmically identify FLLs on CEUS with DL for extracting hierarchy features to improve the accuracy of diagnosis and postoperative prediction (31-35). However, these attempts mainly used CEUS images (30) or CEUS cines with two frames per second (33,34), i.e., not frame-to-frame, and did not take advantage of the spatiotemporal characteristics of CEUS. Moreover, routine clinical factors are rarely used in computer-assisted diagnosis (31-33,35).
Therefore, in this retrospective and multicenter study, we conducted DL radiomics to diagnose FLLs by simultaneously combining features from CEUS cines and clinical factors. Furthermore, we compared the classification accuracy and efficiency of the model with those of the radiologists in the internal and external validation (EV) cohorts. We present the following article in accordance with the TRIPOD reporting checklist (available at https://qims.amegroups.com/article/view/10.21037/qims-21-1004/rc).
Methods
This retrospective study was approved by the institutional review board (No. KY2019129), and was conducted in accordance with the Declaration of Helsinki (as revised in 2013). Requirement for patient consent was waived because of the retrospective nature of this study.
Patients
A total of 1017 pathologically confirmed after-surgery patients were researched from institution 1, between February 2018 and August 2019, and institutions 2 and 3, between February 2018 and August 2018. After applying the inclusion and exclusion criteria, 303 patients were enrolled (Figure 1), of which 253, 26, and 24 were from institution 1, 2, and 3, respectively. The inclusion criteria were (I) patient aged 18 years or older; (II) no ultrasound contrast allergy history; (III) ultrasound-found FLLs; and (IV) pathologically confirmed after-surgery patients. The exclusion criteria were (I) lack of complete CEUS imaging recording or clinical information; (II) poor quality of CEUS imaging; and (III) excessive motion during CEUS examination.
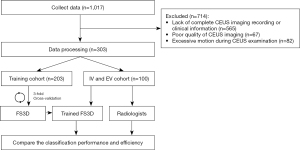
CEUS acquisition
CEUS examinations were performed by seven radiologists with more than five years of experience in liver CEUS using four ultrasound instruments (Table S1). First, the patient took the left lateral position, and the location of the lesion before CEUS was determined through B-mode US. Thereafter, the patient was observed for 5 min after injecting 2.4 mL of the second-generation contrast agents (SonoVue, Bracco Imaging, Italy) via the elbow vein followed by a 5-mL saline flush. For multiple tumors, patients received additional administrations of SonoVue to ensure each tumor was observed and the largest tumor was chosen in our study.
For each patient, approximately 20 s arterial phase cines, two portal venous phase images, and two delayed phase images of the maximum width of the lesion were acquired. All the cines were stored in .wav or .avi formats, and the images were stored in .jpg format.
Clinical information acquisition
All the patients’ demographic and clinical data were recorded from the picture archiving and communication systems, including age, sex, pathological results, hepatitis, AFP, tumor location, and tumor size in B-mode US. Hepatitis includes hepatitis B virus infection, hepatitis C virus infection, fatty liver, and hepatic cirrhosis. If patients presented with hepatitis, it would be encoded to one, else zero. AFP was measured within one week before surgery, and its value was scaled to (0, 1) by log-normalization. Tumor location included the right lobe, left lobe, and caudal lobe, according to the anatomy. Tumor size was measured according to the largest boundary of the ROI of the lesion in clinical settings.
CEUS pre-processing
CEUS cines were collected with ultrasonic instruments, which usually include two parts, B mode and CEUS mode, which are in the RGB mode and are usually arranged in a left-right layout. First, the original CEUS cines were split into two separate parts: B mode and CEUS mode. Second, the optical flow for each cine was calculated using the Gunnar Farneback algorithm (36), which could help us better capture the hidden dynamic motion information of videos. Third, four cines for each patient, two RGB parts and two optical flow parts with the same width, height and frames, were cut into several short segments, known as four-stream segments with sixteen 224×224 frames, because of the limited graphics memory of GPUs. Finally, the pixels in each segment were normalized to (0, 1) (Figure 2).
The cines were processed using FFmpeg 4.2.2 (https://ffmpeg.org/) and Python Imaging Library Pillow 3.3.1 (https://pypi.python.org/pypi/Pillow/3.3.1).
DL radiomics model
A 3D CNN was trained on the four-stream cines above and named four-stream 3D (FS3D) CNN (Figure 3). These segments were fed sequentially into two independent CNNs, inflated 3D CNN (I3D) and channel-separated CNN (CSN) for feature extraction (37,38). The extracted features were then fused by channel concatenation to obtain a feature vector with a fixed length of 8192, and incorporated with clinical information. Finally, 544 of the most important features were selected by setting the importance threshold to 0.02, and combined with clinical factors to classify FLLs using a classification CNN (39).
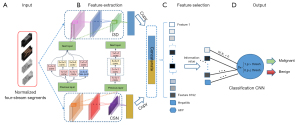
The I3D network is a classical video-classification CNN with a 3×3×3 3D convolutional layer, 1×1×1 3D convolutional layer, and 3×3×3 3D Max-pooling layer. It gathers information from four different paths with different convolutional kernels and max pooling layers to aggregate spatial and temporal features at different scales. The CSN network is mainly composed of a 1×1×1 3D CNN and 3×3×3 depthwise CNN, which are used to extract channel interactions and local interactions, respectively. This structure leads to improved video-classification accuracy and lower computation cost. The features extracted by I3D and CSN are complementary; they can be combined to obtain a complete feature representation of the dynamic CEUS cines.
Four models, FS3DU (trained with CEUS cines only), FS3DU+A (trained with CEUS cines and AFP), FS3DU+H (trained with CEUS cines and hepatitis history), and FS3DU+A+H (trained with CEUS cines, AFP, and hepatitis history) were investigated to analyze their diagnostic capabilities.
Experimental details
In the training stage, 3-fold cross-validation was used to adjust the network architecture (hyper-parameters, number of iterations, regularization method, and class weights). For each fold, one model was trained with a subset of 2/3 of the training dataset, and the remaining 1/3 was used for validation. After three cycles, the model with the highest AUC was chosen, and the holdout internal validation (IV) and EV cohorts were used for the final evaluation (Tables S2-S4).
Transfer learning was used in this study. The parameters of the I3D and CSN were initialized with those from the Kinetics dataset (40) and fine-tuned with our dataset (41). Using pretrained weights helped the model converge faster on our smaller dataset. We downloaded parameters that were generated from the training DL model with the Kinetics dataset and initialized our model for training. We trained 5,000 iterations with a learning rate of 0.001, batch size of one, and the learning optimizer was Adam.
The models were built using Python 3.5 (https://www.python.org/downloads/release/python-350/) and Pytorch 1.2 (https://pytorch.org/). All the experiments were run on an NVIDIA GeForce GTX 1080 GPU.
Comparative evaluation of diagnostic performance
Patients were stratified into three subgroups according to lesion size measured in the B-mode US (<20.0, 20.0–50.0, and >50.0 mm). Two radiologists with 12 years and 5 years of experience were invited to evaluate the IV and EV cohorts according to (3) based on the imaging characteristic and clinical factors. All the information, including approximately 20 s arterial phase cines, two portal venous phase images, two delayed phase images, and clinical data, except for pathological results, were presented to the radiologists in .ppt format. The start time was when the radiologists started to read the first page of the PPT.
Statistical analysis
Pearson’s chi-square tests were conducted for categorical clinical factors, which were described as percentages. For continuous clinical factors, Student’s t-tests were conducted.
The area under the receiver operating characteristic curve (AUC), accuracy (ACC), sensitivity (SEN), specificity (SPE), positive predictive value, negative predictive value, and receiver operating characteristic curve (ROC) for diagnosing each category were calculated for the IV and EV cohorts. Two-sided Delong tests were used to calculate statistical differences between AUC values. The statistical analyses were performed using Python 3.5 (https://www.python.org/downloads/release/python-350/), and P<0.05 was considered significant.
Results
Baseline characteristics
A total of 303 patients from three hospitals were enrolled according to the enrollment criteria. Up to 565 patients were excluded because of a lack of complete CEUS imaging or clinical data, and 67 and 82 patients were excluded because of poor imaging quality and excessive motion, respectively. All the enrolled patients were divided into a training cohort (n=203, 123 men and 80 women, mean age: 48.5±13.3, 85 benign and 118 malignant lesions, 15 FLL types), an IV cohort (n=50, 30 men and 20 women, mean age: 52.6±10.8, 12 benign and 38 malignant lesions, 7 FLL types) with a ratio of 4:1 from institution 1, and an EV cohort (n=50, 22 men and 28 women, mean age: 49.2±12.2, 21 benign and 29 malignant lesions, 7 FLL types) from the other two institutions. The baseline characteristics of all the enrolled patients are summarized in Table 1. There were no significant differences in characteristics and demographics between the training, IV, and EV cohorts, except for the ultrasound equipment (P<0.05, Table 1). In total, 18 types of FLLs were enrolled in this study (Table S5).
Table 1
Characteristic | All patients (n=303) | Training cohort (n=203) | IV cohort (n=50) | EV cohort (n=50) |
---|---|---|---|---|
Age (years), mean ± SD | 52.31±13.0 | 48.5±13.3 | 52.6±10.8 | 49.2±12.2 |
Sex (%) | ||||
Male | 175 (57.8) | 123 (60.6) | 30 (60.0) | 22 (44.0) |
Female | 128 (42.2) | 80 (39.4) | 20 (40.0) | 28 (56.0) |
Chronic liver disease, n (%) | ||||
HBV | 151 (49.8) | 94 (46.3) | 33 (66.0) | 24 (48.0) |
HCV | 1 (0.3) | 0 | 0 | 1 (2.0) |
Fatty liver | 13 (4.3) | 9 (4.4) | 2 (4.0) | 1 (2.0) |
Liver cirrhosis | 65 (21.5) | 49 (21.1) | 14 (28.0) | 2 (4.0) |
Normal | 141 (46.5) | 103 (50.7) | 16 (32.0) | 22 (44.0) |
Tumor, n (%) | ||||
Benign | 118 (38.9) | 85 (41.9) | 12 (24.0) | 21 (42.0) |
Malignant | 185 (61.1) | 118 (58.1) | 38 (76.0) | 29 (58.0) |
Tumor location, n (%) | ||||
Right lobe | 206 (68.0) | 133 (65.5) | 38 (76.0) | 35 (70.0) |
Left lobe | 92 (30.4) | 67 (33.0) | 10 (20.0) | 15 (30.0) |
Caudate | 5 (1.6) | 3 (1.5) | 2 (4.0) | 0 |
Equipment, n (%)* | ||||
512 | 155 (51.1) | 122 (60.1) | 28 (56.0) | 5 (10.0) |
E9 | 65 (21.5) | 29 (14.3) | 11 (22.0) | 25 (50.0) |
S2000 | 63 (20.8) | 52 (25.6) | 11 (22.0) | 0 |
iU22 | 20 (6.6) | 0 | 0 | 20 (40.0) |
AFP, n (%) | ||||
>200 ng/mL | 57 (18.8) | 29 (14.3) | 16 (32.0) | 13 (26.0) |
<200 ng/mL | 246 (81.2) | 174 (85.7) | 34 (68.0) | 37 (74.0) |
Tumor size (mm), mean ± SD | 55.2±34.7 | 60.4±34.9 | 61.3±35.3 | 37.3.04±24.9 |
*, P<0.05 for comparison among training, IV, and EV cohorts. IV, internal validation; EV, external validation; AFP, alpha fetoprotein; HBV, hepatitis B virus; HCV, hepatitis C virus.
Predictive performance of the models
The diagnostic performance of the FS3D models is shown in Table 2 and Figure 4. FS3DU+H+A, which incorporated CEUS cines, hepatitis, and AFP, achieved superior diagnostic performance, with AUC values of 0.969 (0.95% CI: 0.901–1.000) and 0.957 (0.95% CI: 0.894–1.000) in the IV and EV cohorts, respectively. The results were statistically improved compared to FS3DU (P<0.05, Table 2) in the IV cohort as well as FS3DU (P<0.05, Table 2) and FS3DU+H (P<0.05, Table 2) in the EV cohort.
Table 2
IV cohort | EV cohort | ||||||||
---|---|---|---|---|---|---|---|---|---|
FS3DU | FS3DU+H | FS3DU+A | FS3DU+H+A | FS3DU | FS3DU+H | FS3DU+A | FS3DU+H+A | ||
AUC (95% CI) | 0.898* (0.780, 1.000) | 0.938 (0.844, 1.000) | 0.950 (0.865, 1.000) | 0.969 (0.901, 1.000) | 0.798* (0.668, 0.928) | 0.849* (0.734, 0.964) | 0.892 (0.793, 0.991) | 0.957 (0.894, 1.000) | |
ACC (95% CI) | 0.840 (0.709, 0.928) | 0.940 (0.835, 0.988) | 0.920 (0.808, 0.978) | 0.960 (0.863, 0.995) | 0.800 (0.663, 0.900) | 0.880 (0.757, 0.955) | 0.920 (0.808, 0.978) | 0.940 (0.835, 0.988) | |
SEN (95% CI) | 0.838 (0.680, 0.938) | 0.946 (0.818, 0.993) | 0.919 (0.781, 0.983) | 0.973 (0.858, 0.999) | 0.862 (0.683, 0.961) | 0.966 (0.822, 0.999) | 0.966 (0.822, 0.999) | 0.966 (0.822, 0.999) | |
SPE (95% CI) | 0.846 (0.546, 0.981) | 0.923 (0.640, 0.998) | 0.923 (0.640, 0.998) | 0.923 (0.640, 0.998) | 0.714 (0.478, 0.887) | 0.762 (0.528, 0.918) | 0.857 (0.637, 0.970) | 0.905 (0.696, 10.988) | |
PPV (95% CI) | 0.939 (0.798, 0.993) | 0.972 (0.855, 0.999) | 0.971 (0.851, 0.999) | 0.973 (0.858, 0.999) | 0.806 (0.625, 0.926) | 0.848 (0.681, 0.949) | 0.903 (0.743, 0.980) | 0.933 (0.779, 0.992) | |
NPV (95% CI) | 0.647 (0.383, 0.858) | 0.857 (0.572, 0.982) | 0.800 (0.519, 0.957) | 0.923 (0.640, 0.998) | 0.789 (0.544, 0.940) | 0.941 (0.713, 0.999) | 0.947 (0.740, 0.999) | 0.950 (0.751, 0.999) | |
Speed (sec) | 10.76 | 10.76 | 10.76 | 10.76 | 10.76 | 10.76 | 10.76 | 10.76 |
Comparisons of the AUCs of model FS3DU+H+A among four subgroups were performed by Delong test. *, differences were significant when AUC of FS3DU+H+A were compared to other models (P<0.05). FS3DU = FS3DCEUS; FS3DU+A = FS3DCEUS+AFP; FS3DU+H = FS3DCEUS+Hepatitis; FS3DU+A+H = FS3DCEUS+AFP+Hepatitis. 95% CI, confidence interval of 95%; IV, internal validation; EV, external validation; AUC, the area under the receiver operating characteristic curve; ACC, accuracy; SEN, sensitivity; SPE, specificity; PPV, positive predictive value; NPV, negative predictive value; FS3D, Four-Stream three-dimensional.
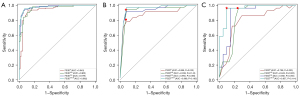
Compared to their performance in the EV cohort, the performance of all models in the IV cohort deteriorated. However, the Delong tests showed no significant differences among the four models in the IV and EV cohorts (P>0.05 for all, Table 2; Table S6).
Stratification analysis of the models and radiologists
In the stratification analysis, the FS3DU+H+A model exhibited statistically improved AUCs compared with R2 in the IV and EV cohorts (P<0.05 for all, Table 3). It also showed slightly improved AUCs compared with R1, while the improvement was not statistically significant.
Table 3
IV cohort (n=50) | EV cohort (n=50) | ||||||
---|---|---|---|---|---|---|---|
FS3DU+H+A | R1 | R2 | FS3DU+H+A | R1 | R2 | ||
Total | 0.969 (0.901, 1.000) | 0.935 (0.839, 1.000) | 0.867* (0.735, 0.999) | 0.957 (0.894, 1.000) | 0.935 (0.857, 1.000) | 0.864* (0.754, 0.974) | |
<20 mm (n=26) | 0.900 (0.783, 1.000) | 1.000 (1.000, 1.000) | 0.950 (0.768, 1.000) | 0.881 (0.778, 0.984) | 0.929 (0.778, 1.000) | 0.786 (0.531, 1.000) | |
20–50 mm (n=34) | 1.000 (1.000, 1.000) | 0.900 (0.596, 1.000) | 0.800 (0.402, 1.000) | 0.933 (0.854, 1.000) | 0.899 (0.743, 1.000) | 0.899 (0.743, 1.000) | |
>50 mm (n=40) | 0.956 (0.852, 1.000) | 0.938 (0.816, 1.000) | 0.879 (0.713, 1.000) | 0.983 (0.943, 1.000) | 1.000 (1.000, 1.000) | 0.917 (0.751, 1.000) | |
Speed (sec) | 10.76 | 23.74 | 25.95 | 10.76 | 27.75 | 29.50 |
Comparisons of the AUCs of model FS3DU+H+A to radiologists among three subgroups were performed by Delong test. FS3DU+A+H = FS3DCEUS+AFP+Hepatitis. *, differences were significant when AUC of FS3DU+H+A were compared to radiologists (P<0.05). 95% CI, confidence interval of 95%; IV, internal validation; EV, external validation; FS3D, Four-Stream three-dimensional; AUC, the area under the receiver operating characteristic curve.
Meanwhile, a hierarchical analysis was performed according to the tumor size measured in the US images (Table 3; Tables S7-S9). In the <20 mm subgroup (n=26, 19 malignant lesions and 7 benign lesions), the AUCs of FS3DU+H+A were lowest in the IV cohort but higher than that of R2 in the EV cohort. In the 20–50 mm (n=34, 23 malignant lesions and 11 benign lesions) and >50 mm (n=40, 25 malignant lesions and 15 benign lesions) subgroups, the FS3DU+H+A model achieved the best performance compared to R1 and R2.
Predictive efficiency of the models compared to the radiologists
In terms of predictive efficiency, the four models, which achieved the same diagnosis speed (10.67 s per patient, Table 2), were almost three times faster than the radiologists in the IV cohort and approximately two times faster than the radiologists in the EV cohort. The experienced radiologist, R1, was faster than the young radiologist, R2.
Location performance of the model
To better understand the ability of the proposed models, the feature maps were converted into Gradient-weighted Class Activation Mapping (Grad-CAM) and visualized (Figure 5) (42). Each pixel in the maps was encoded using pseudo-color, and the warm color (red) represents a more substantial contribution to the predictive classification. By reading Grad-CAM heat-maps, we preliminarily concluded that the red/warm color regions occurred in patients with hyper-enhancement in the arterial phase. It indicates that our model is tracking the flow of CAs. Not only does it provide a visualization and interpretable capability for the network, but in future research we can also use Grad-CAM for ROI localization (43,44).
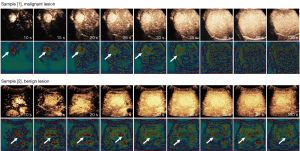
Here, we visualized and analyzed two samples: Sample [1], obtained from a 28-year-old man with liver cirrhosis, exhibited a malignant lesion of dimensions 38 mm × 35 mm (hepatocellular carcinoma, HCC) in the right liver, with an AFP concentration of 4.22 ng/mL. The imaging features showed rapid hyper-enhancement from the periphery to the center of the lesion in the artery phase and iso-enhancement in the portal venous phase. It is a case of HCC with atypical imaging, and the R1 misdiagnosed it; however, it was correctly diagnosed by the FS3DU+H+A model; Sample [2], obtained from a 32-year-old woman with no history of hepatitis, exhibited a benign lesion of dimensions 41 mm ×27 mm (focal nodular hyperplasia, FNH) in the right liver, with an AFP concentration of 2.5 ng/mL. The imaging features showed slow hyper-enhancement from the center to the periphery in the late artery phase and wash-out in the late portal venous phase. It is a case of benign FLL with atypical imaging, and R2 misdiagnosed it, whereas the FS3DU+H+A model correctly diagnosed it.
In addition, the misclassified cases of our model were analyzed, and we found that they mainly presented atypical imaging characteristics in CEUS (Figure 6). Case A of hemangioma was misdiagnosed as a malignant tumor. It presented peripheral annular enhancement with obvious internal thrombosis, which was different from the typical nodular enhancement, and was hard to differentiate from hepatocellular carcinoma with partial internal necrosis. Case B of cholangiocarcinoma was misdiagnosed as a benign tumor. It showed inhomogeneous and slight hyper-enhancement, and an unclear boundary. It was not the typical annular enhancement, and was difficult to differentiate from inflammatory lesions. Case C of primary liver cancer was misdiagnosed as a benign tumor. The enhancement pattern in the arterial phase was annular and nodular hyper-enhancement because of the necrotic areas, and was difficult to differentiate from that of hemangioma.
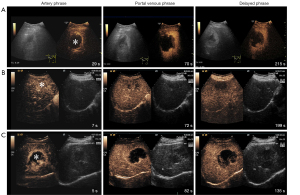
Discussion
Rapid wash-in and wash-out is the typical imaging characteristic of liver cancer. The hepatocarcinogenesis is accompanied by decline in normal vascularity and the development of neoangiogenesis and sinusoidal capillarization. Microbubble contrast agents in CEUS can enhance the echo signals of the blood supply, and real-time perfusion information about the lesion can be analyzed frame-to-frame. Hence CEUS plays an important role in the early diagnosis of liver cancer in clinical practice. However, recent studies have shown that the imaging features of liver cancer can be presented through various features corresponding to clinicopathological characteristics. For example, small HCC or well-differentiated HCC could exhibit iso-enhancement for atypical imaging in the late phase, which is consistent with the imaging characteristic of dysplastic nodules, and is easily misdiagnosed. Therefore, combining the patient’s medical history and related laboratory examinations is of significant importance for the accurate identification of liver cancer.
DL has been shown to perform well in extracting features of medical imaging. 3D CNNs, in particular, can extract spatiotemporal information effectively. Therefore, the established the FS3DU+H+A model, incorporated long-range CEUS cines with approximately 20 frames per second and clinical factors, and achieved the best performance in identifying FLLs among other models, and better than earlier studies (28,29,32), which only analyzed CEUS and reported an average accuracy of 94.3%, 93.1%, and 90.3%, respectively. The results indicate that clinical factors are important for computer-assisted diagnosis; this indication is consistent with clinical diagnosis investigation.
In stratified analysis, the FS3DU+H+A model was significantly advantageous over the younger R2 and provided a better AUC than the more experienced R1 for lesions in the ≥20 mm groups, while slightly worse in the <20 mm group. It may be because the rapid motion in CEUS cine may easily occlude lesions, resulting in the blood perfusion of small lesions in some key frames not being captured. However, the total AUC of the model was higher than that of the radiologists on CEUS in former reports, who achieved an average accuracy of 85% (9,45) and even in CT and MRI (9,45,46). Hence, our model learns discriminative spatiotemporal representations from long-range CEUS cines and clinical factors, and offers remarkable capabilities in the differential diagnosis of FLLs. The EV, 3-fold cross-validation, and variety of CEUS equipment proved the robustness of our models.
It is worth mentioning that diagnosing liver cancer in liver cirrhosis is challenging in clinical practice. Our study’s IV and EV cohorts included 16 patients with liver cirrhosis, including 2 benign and 14 malignant lesions. The diagnostic accuracy of both R1 and FS3DU+H+A models were 100%, while that of the younger R2 was 93.75%. The results indicate that the model also performed well in diagnosis of liver cancer in liver cirrhosis. However, the comprehensive analysis of misclassified cases show that our model still lacks the differential diagnosis of patients with atypical imaging features, mainly due to the small number of cases included in this study.
In terms of diagnosis speed, our models took 10.76 s to diagnose each patient, which is faster than the manual assessment (9,45,46). Hence, our method could be widely applicable as a cost-effective and safe method in clinical practice, and may replace CT or MRI. In addition, feature maps generated by the algorithm can clearly indicate the location of the lesion and help radiologists focus on blood perfusion information, overcoming observable limitation factors such as breath motion. They can interpret the CNN results, which has important implications for clinical diagnosis and navigation in the future.
Our study has some limitations. First, although fully trained CNNs require a large dataset, the sample size and the number of medical centers in our study were smaller, and there is still an imbalance in the types of FLLs. We also need additional data to verify the performance of the model in the heterogeneity diagnosis of FLLs. Therefore, in subsequent research, more multicenter data with standard formats should be collected and applied. Second, a binary classification of FLLs was achieved, which is only the first step toward clinical applications. Therefore, the classification of different types of FLLs will be one of our future focus areas, especially for the accurate diagnosis of HCC. Third, CT and MRI also provide important information on the extent of the local tumor, which should be included for multimodal analysis in subsequent studies.
In conclusion, the proposed DL radiomics captured the dynamic perfusion information of liver cancer, and combined the patients’ AFP and hepatitis history, which is the key link of diagnosis in clinical practice. Finally, the strategy is more in line with the clinical diagnosis of liver cancer, and achieves an outstanding performance and higher speed in the diagnosis of FLLs, and is superior to skilled radiologists. Hence, it is promising for the wide application of CEUS as a time- and cost-effective imaging method in clinical settings, and could drive further innovation in medicine.
Acknowledgments
The authors are grateful for the support and participation from the Sonographers Branch of the Chinese Medical Doctors Association.
Funding: This work was supported by International Science & Technology Cooperation Program of China (No. 2015DFA30920), Science and Technology (International Science & Technology Cooperation) Research Base Construction Program of Chongqing (No. cstc2014gjhz110004), and the National Natural Science Foundation of China (No. 31671251).
Footnote
Reporting Checklist: The authors have completed the TRIPOD reporting checklist (available at https://qims.amegroups.com/article/view/10.21037/qims-21-1004/rc).
Conflicts of Interest: All authors have completed the ICMJE uniform disclosure form (available at https://qims.amegroups.com/article/view/10.21037/qims-21-1004/coif). MZ used to be an employee of CHISON Medical Technologies Co., LTD., and LL is a current employee of CHISON Medical Technologies Co., LTD. They provided technology support in this study. The other authors have no conflicts of interest to declare.
Ethical Statement: The authors are accountable for all aspects of the work in ensuring that questions related to the accuracy or integrity of any part of the work are appropriately investigated and resolved. This study received institutional review board approval (No. KY2019129), and was conducted in accordance with the Declaration of Helsinki (as revised in 2013). Requirement for patient consent was waived because of the retrospective nature of this study.
Open Access Statement: This is an Open Access article distributed in accordance with the Creative Commons Attribution-NonCommercial-NoDerivs 4.0 International License (CC BY-NC-ND 4.0), which permits the non-commercial replication and distribution of the article with the strict proviso that no changes or edits are made and the original work is properly cited (including links to both the formal publication through the relevant DOI and the license). See: https://creativecommons.org/licenses/by-nc-nd/4.0/.
References
- Bray F, Ferlay J, Soerjomataram I, Siegel RL, Torre LA, Jemal A. Global cancer statistics 2018: GLOBOCAN estimates of incidence and mortality worldwide for 36 cancers in 185 countries. CA Cancer J Clin 2018;68:394-424. [Crossref] [PubMed]
- Zheng R, Qu C, Zhang S, Zeng H, Sun K, Gu X, Xia C, Yang Z, Li H, Wei W, Chen W, He J. Liver cancer incidence and mortality in China: Temporal trends and projections to 2030. Chin J Cancer Res 2018;30:571-9. [Crossref] [PubMed]
- Heimbach JK, Kulik LM, Finn RS, Sirlin CB, Abecassis MM, Roberts LR, Zhu AX, Murad MH, Marrero JA. AASLD guidelines for the treatment of hepatocellular carcinoma. Hepatology 2018;67:358-80. [Crossref] [PubMed]
- European Association for the Study of the Liver. EASL Clinical Practice Guidelines: Management of hepatocellular carcinoma. J Hepatol 2018;69:182-236. [Crossref] [PubMed]
- National Health and Family Planning Commission of the People’s Republic of China. Diagnosis, management, and treatment of hepatocellular carcinoma (V2019). Chin J Pract Surg 2020;1:5-23.
- Kokudo N, Hasegawa K, Akahane M, Igaki H, Izumi N, Ichida T, et al. Evidence-based Clinical Practice Guidelines for Hepatocellular Carcinoma: The Japan Society of Hepatology 2013 update (3rd JSH-HCC Guidelines). Hepatol Res 2015; [Crossref] [PubMed]
- Sidhu PS, Cantisani V, Dietrich CF, Gilja OH, Saftoiu A, Bartels E, et al. The EFSUMB Guidelines and Recommendations for the Clinical Practice of Contrast-Enhanced Ultrasound (CEUS) in Non-Hepatic Applications: Update 2017 (Long Version). Ultraschall Med 2018;39:e2-e44. [Crossref] [PubMed]
- Dietrich CF, Nolsøe CP, Barr RG, Berzigotti A, Burns PN, Cantisani V, et al. Guidelines and Good Clinical Practice Recommendations for Contrast-Enhanced Ultrasound (CEUS) in the Liver-Update 2020 WFUMB in Cooperation with EFSUMB, AFSUMB, AIUM, and FLAUS. Ultrasound Med Biol 2020;46:2579-604. [Crossref] [PubMed]
- Friedrich-Rust M, Klopffleisch T, Nierhoff J, Herrmann E, Vermehren J, Schneider MD, Zeuzem S, Bojunga J. Contrast-Enhanced Ultrasound for the differentiation of benign and malignant focal liver lesions: a meta-analysis. Liver Int 2013;33:739-55. [Crossref] [PubMed]
- Aubé C, Oberti F, Lonjon J, Pageaux G, Seror O, N'Kontchou G, Rode A, Radenne S, Cassinotto C, Vergniol J, Bricault I, Leroy V, Ronot M, Castera L, Michalak S, Esvan M, Vilgrain V. CHIC Group. EASL and AASLD recommendations for the diagnosis of HCC to the test of daily practice. Liver Int 2017;37:1515-25. [Crossref] [PubMed]
- Vilana R, Forner A, Bianchi L, García-Criado A, Rimola J, de Lope CR, Reig M, Ayuso C, Brú C, Bruix J. Intrahepatic peripheral cholangiocarcinoma in cirrhosis patients may display a vascular pattern similar to hepatocellular carcinoma on contrast-enhanced ultrasound. Hepatology 2010;51:2020-9. [Crossref] [PubMed]
- Dong Y, Wang WP, Mao F, Zhang Q, Yang D, Tannapfel A, Meloni MF, Neye H, Clevert DA, Dietrich CF. Imaging Features of Fibrolamellar Hepatocellular Carcinoma with Contrast-Enhanced Ultrasound. Ultraschall Med 2021;42:306-13. [Crossref] [PubMed]
- Guo HL, Zheng X, Cheng MQ, Zeng D, Huang H, Xie XY, Lu MD, Kuang M, Wang W, Xian MF, Chen LD. Contrast-Enhanced Ultrasound for Differentiation Between Poorly Differentiated Hepatocellular Carcinoma and Intrahepatic Cholangiocarcinoma. J Ultrasound Med 2021; [Epub ahead of print]. [PubMed]
- Bota S, Piscaglia F, Marinelli S, Pecorelli A, Terzi E, Bolondi L. Comparison of international guidelines for noninvasive diagnosis of hepatocellular carcinoma. Liver Cancer 2012;1:190-200. [Crossref] [PubMed]
- Schellhaas B, Hammon M, Strobel D, Pfeifer L, Kielisch C, Goertz RS, Cavallaro A, Janka R, Neurath MF, Uder M, Seuss H. Interobserver and intermodality agreement of standardized algorithms for non-invasive diagnosis of hepatocellular carcinoma in high-risk patients: CEUS-LI-RADS versus MRI-LI-RADS. Eur Radiol 2018;28:4254-64. [Crossref] [PubMed]
- LeCun Y, Bengio Y, Hinton G. Deep learning. Nature 2015;521:436-44. [Crossref] [PubMed]
- Schmauch B, Herent P, Jehanno P, Dehaene O, Saillard C, Aubé C, Luciani A, Lassau N, Jégou S. Diagnosis of focal liver lesions from ultrasound using deep learning. Diagn Interv Imaging 2019;100:227-33. [Crossref] [PubMed]
- Yang Q, Wei J, Hao X, Kong D, Yu X, Jiang T, et al. Improving B-mode ultrasound diagnostic performance for focal liver lesions using deep learning: A multicentre study. EBioMedicine 2020;56:102777. [Crossref] [PubMed]
- Yasaka K, Akai H, Abe O, Kiryu S. Deep Learning with Convolutional Neural Network for Differentiation of Liver Masses at Dynamic Contrast-enhanced CT: A Preliminary Study. Radiology 2018;286:887-96. [Crossref] [PubMed]
- Zhou J, Wang W, Lei B, Ge W, Huang Y, Zhang L, Yan Y, Zhou D, Ding Y, Wu J, Wang W. Automatic Detection and Classification of Focal Liver Lesions Based on Deep Convolutional Neural Networks: A Preliminary Study. Front Oncol 2021;10:581210. [Crossref] [PubMed]
- Ben-Cohen A, Klang E, Kerpel A, Konen E, Amitai MM, Greenspan H. Fully convolutional network and sparsity-based dictionary learning for liver lesion detection in CT examinations. Neurocomputing 2018;275:1585-94. [Crossref]
- Li M, Li X, Guo Y, Miao Z, Liu X, Guo S, Zhang H. Development and assessment of an individualized nomogram to predict colorectal cancer liver metastases. Quant Imaging Med Surg 2020;10:397-414. [Crossref] [PubMed]
- Hamm CA, Wang CJ, Savic LJ, Ferrante M, Schobert I, Schlachter T, Lin M, Duncan JS, Weinreb JC, Chapiro J, Letzen B. Deep learning for liver tumor diagnosis part I: development of a convolutional neural network classifier for multi-phasic MRI. Eur Radiol 2019;29:3338-47. [Crossref] [PubMed]
- Wang CJ, Hamm CA, Savic LJ, Ferrante M, Schobert I, Schlachter T, Lin M, Weinreb JC, Duncan JS, Chapiro J, Letzen B. Deep learning for liver tumor diagnosis part II: convolutional neural network interpretation using radiologic imaging features. Eur Radiol 2019;29:3348-57. [Crossref] [PubMed]
- Dai H, Lu M, Huang B, Tang M, Pang T, Liao B, Cai H, Huang M, Zhou Y, Chen X, Ding H, Feng ST. Considerable effects of imaging sequences, feature extraction, feature selection, and classifiers on radiomics-based prediction of microvascular invasion in hepatocellular carcinoma using magnetic resonance imaging. Quant Imaging Med Surg 2021;11:1836-53. [Crossref] [PubMed]
- Streba CT, Ionescu M, Gheonea DI, Sandulescu L, Ciurea T, Saftoiu A, Vere CC, Rogoveanu I. Contrast-enhanced ultrasonography parameters in neural network diagnosis of liver tumors. World J Gastroenterol 2012;18:4427-34. [Crossref] [PubMed]
- Wu K, Chen X, Ding M. Deep learning based classification of focal liver lesions with contrast-enhanced ultrasound. Optik (Stuttg.) 2014;125:4057-4063. [Crossref]
- Kondo S, Takagi K, Nishida M, Iwai T, Kudo Y, Ogawa K, Kamiyama T, Shibuya H, Kahata K, Shimizu C. Computer-Aided Diagnosis of Focal Liver Lesions Using Contrast-Enhanced Ultrasonography With Perflubutane Microbubbles. IEEE Trans Med Imaging 2017;36:1427-37. [Crossref] [PubMed]
- Guo Lehang, Wang Dan, Xu Huixiong, Qian Yiyi, Wang Chaofeng, Zheng Xiao, Zhang Qi, Shi Jun. CEUS-based classification of liver tumors with deep canonical correlation analysis and multi-kernel learning. Annu Int Conf IEEE Eng Med Biol Soc 2017;2017:1748-51. [Crossref] [PubMed]
- Huang Q, Pan F, Li W, Yuan F, Hu H, Huang J, Yu J, Wang W. Differential Diagnosis of Atypical Hepatocellular Carcinoma in Contrast-Enhanced Ultrasound Using Spatio-Temporal Diagnostic Semantics. IEEE J Biomed Health Inform 2020;24:2860-9. [Crossref] [PubMed]
- Hu HT, Wang W, Chen LD, Ruan SM, Chen SL, Li X, Lu MD, Xie XY, Kuang M. Artificial intelligence assists identifying malignant versus benign liver lesions using contrast-enhanced ultrasound. J Gastroenterol Hepatol 2021;36:2875-83. [Crossref] [PubMed]
- Pan F, Huang Q, Li X. Classification of liver tumors with CEUS based on 3D-CNN. 2019 IEEE 4th International Conference on Advanced Robotics and Mechatronics (ICARM) 2019:845-9.
- Liu D, Liu F, Xie X, Su L, Liu M, Xie X, Kuang M, Huang G, Wang Y, Zhou H, Wang K, Lin M, Tian J. Accurate prediction of responses to transarterial chemoembolization for patients with hepatocellular carcinoma by using artificial intelligence in contrast-enhanced ultrasound. Eur Radiol 2020;30:2365-76. [Crossref] [PubMed]
- Liu F, Liu D, Wang K, Xie X, Su L, Kuang M, Huang G, Peng B, Wang Y, Lin M, Tian J, Xie X. Deep Learning Radiomics Based on Contrast-Enhanced Ultrasound Might Optimize Curative Treatments for Very-Early or Early-Stage Hepatocellular Carcinoma Patients. Liver Cancer 2020;9:397-413. [Crossref] [PubMed]
- Ta CN, Kono Y, Eghtedari M, Oh YT, Robbin ML, Barr RG, Kummel AC, Mattrey RF. Focal Liver Lesions: Computer-aided Diagnosis by Using Contrast-enhanced US Cine Recordings. Radiology 2018;286:1062-71. [Crossref] [PubMed]
- Farneback G. Two-Frame Motion Estimation Based on Polynomial Expansion. 13th Scandinavian Conference on Image Analysis, Espoo, Finland, 2003.
- Carreira J, Zisserman A. Quo vadis, action recognition? A new model and the kinetics dataset. IEEE Conference on Computer Vision and Pattern Recognition, Honolulu, HI 2017.
- Tran D, Wang H, Torresani L, Feiszli M. Video classification with channel-separated convolutional networks. 2019 IEEE/CVF International Conference on Computer Vision, Seoul, Korea (South) 2017;5551-60.
- Řezáč M. ESIS2: information value estimator for credit scoring models. Comput Econ 2015;45:303-22. [Crossref]
- Kay W, Carreira J, Simonyan K, Zhang B, Aisserman A. The kinetics human action video dataset. ArXiv e-prints. May 19, 2017. Available online: https://arxiv.org/abs/1705.06950
- Pan SJ, Yang Q. A survey on transfer learning. IEEE Trans Knowl Data Eng 2010;10:1345-59. [Crossref]
- Selvaraju RR, Cogswell M, Das A, Vedantam R, Parikh D, Batra D. Grad-CAM: visual explanations from deep networks via gradient-based localization. ArXiv e-prints. Oct 7, 2016. Avalible online: https://arxiv.org/abs/1610.02391
- Xue H, Liu C, Wan F, Jiao J, Ye Q. DANet: Divergent Activation for Weakly Supervised Object Localization. 2019 IEEE/CVF International Conference on Computer Vision (ICCV). IEEE, 2019:6589-98.
- Yang S, Kim Y, Kim Y, Kim C. Combinational Class Activation Maps for Weakly Supervised Object Localization. 2020 IEEE Winter Conference on Applications of Computer Vision (WACV). IEEE, 2020. doi:
10.48550/arXiv.1910.05518 . 2022-03-14.10.48550/arXiv.1910.05518 - Wu M, Li L, Wang J, Zhang Y, Guo Q, Li X, Zhang X. Contrast-enhanced US for characterization of focal liver lesions: a comprehensive meta-analysis. Eur Radiol 2018;28:2077-88. [Crossref] [PubMed]
- Choi SH, Kim SY, Park SH, Kim KW, Lee JY, Lee SS, Lee MG. Diagnostic performance of CT, gadoxetate disodium-enhanced MRI, and PET/CT for the diagnosis of colorectal liver metastasis: Systematic review and meta-analysis. J Magn Reson Imaging 2018;47:1237-50. [Crossref] [PubMed]