Bi-exponential fitting excluding b=0 data improves the scan-rescan stability of liver IVIM parameter measures and particularly so for the perfusion fraction
Introduction
In intravoxel incoherent motion (IVIM) theory, Dslow (or D) is the diffusion coefficient representing the slow ‘pure’ molecular diffusion; perfusion fraction (PF, or f) represents the fraction of the compartment related to (micro)circulation; Dfast (or D*) is the perfusion-related diffusion coefficient representing the incoherent microcirculation within the voxel, which holds information for blood perfusion’s speed (1). There have been greater interests to explore IVIM imaging to evaluate diffused liver diseases (2-4). A prerequisite to translating IVIM imaging into clinical applications is accurate measurement of IVIM parameters and acceptable reproducibility. Nevertheless, accurate liver IVIM quantification is challenging, partially due to the limited sampling and low signal-to-noise ratio (SNR) for fast diffusion data acquisition (1,3,5,6).
Recently, we published three studies demonstrating the clinical usefulness of liver IVIM in separating non-fibrotic livers and fibrotic livers. The report of Wáng et al. had 16 healthy volunteers and 33 hepatitis B liver fibrosis patients, among them 15 patients had stage-1 liver fibrosis (7); the report of Huang et al. had 26 healthy volunteers and 12 hepatitis B liver fibrosis patients, among them 4 patients had stage-1 liver fibrosis (8); the report of Li et al. had 20 healthy volunteers and 28 hepatitis B liver fibrosis patients, among them 11 patients had stage-1 liver fibrosis (9). All fibrotic livers and healthy livers in these three studies can be separated by IVIM analysis except one stage-2 fibrosis case in the study of Li et al. Interestingly, the study of Huang et al. and the study of Li et al. both had 4 patients respectively with biopsy showing no fibrosis, and these 8 subjects’ diffusing magnetic resonance imaging (MRI) measurements resembled healthy volunteers (8,9). To achieve these good results, we have taken four measures to improve IVIM data analysis. The first step was to perform an initial image data quality assessment, with images with severe respiratory motion and artifacts discarded (10). In our reports on human data, this led to approximately 15% of the liver diffusion MRI scans being considered not useful for IVIM analysis (8-11). The second step was that b=0 image data was excluded from bi-exponential liver diffusion image curve fitting (7-9,11-14). The third step was, if a segmented fitting is applied, the threshold b-value of 60 s/mm2 was chosen (8,9,11,15). Finally, the signal was measured on the right liver with ROI (region-of-interest) based approach, as the left liver is more susceptible to artifact due to content in the stomach and cardiac motion. The ROI-based analysis offers better estimation than the pixelwise-fitting method when the SNR is low (16).
There is strong evidence that the relationship between liver diffusion weighted imaging (DWI) signal and b-value does not follow bi-exponential decay; instead, it can be better fitted by an addition of a very fast component with a tri-exponential decay model (14,17,18). However, with clinical scan settings, the fitting of a tri-exponential decay model can be quite unstable at individual study subject’s levels (17,18). We have empirically demonstrated that liver IVIM analysis can be approximated by the bi-exponential liver diffusion image curve fitting starting from a low b-value [such as b-value =10 s/mm2 (7), b-value =15 s/mm2 (8), b-value =2 s/mm2 (9,11)]. With fitting starting from a non-zero low b-value, the relationship between DWI signal and b-value better follow a bi-exponential decay (12,14). IVIM parameter estimated excluding b=0 s/mm2 image improves the separating of non-fibrotic livers and fibrotic livers, which, in our empirical experience, was the most decisive factor to achieve good diagnostic power for separating non-fibrotic livers and fibrotic livers (Figure 1). Due to the fact that a bi-exponential model cannot fit a decay which more resembles a tri-exponential pattern and thus would increase the fitting instability (18), we postulate that IVIM data fitting by excluding b=0 image data improves the reproducibility of liver IVIM measurement. Utilizing the healthy volunteers’ liver IVIM data, this study aims to confirm this hypothesis.
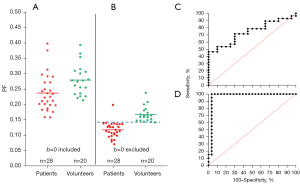
Methods
For evaluating scan-rescan repeatability, a subject was scanned twice during the same session without any change of anatomical positioning (Figure 2A). MRI data of healthy participants were acquired in Shenzhen, China, from Apr 21 to Nov 17, 2019. To control the increase of blood flow to the liver due to food intake, participants were asked to fast for 6 hours before imaging. Study subjects were scanned using a 1.5-T magnet (Achieva, Philips Healthcare, Best, The Netherlands). Diffusion images with 16 b-values of 0, 2, 4, 7, 10, 15, 20, 30, 46, 60, 72, 100, 150, 200, 400, 600 s/mm2 were acquired. The TR was 1,600 ms and the TE was 63 ms. Other parameters included slice thickness =7 mm and inter-slice gap =1 mm, matrix =124×97, FOV =375 mm × 302 mm, NEX =2, number of slices =7. The included slices were focused on the central part of the liver.
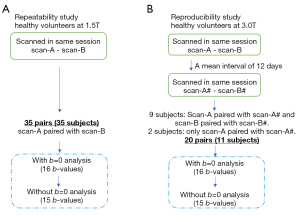
For evaluating scan-rescan reproducibility, each subject was scanned twice in the first scan session with the second scan performed after an interval of 5–18 days (mean: 12 days) and also scanned twice (Figure 2B). MRI data of healthy participants were acquired in Nanjing, China, from Apr 14 to May 7, 2017. A 3T magnet and a 32 channels dStream Torso coil (Ingenia, Philips Healthcare, Best, The Netherlands) was used. Diffusion images with 16 b-values of 0, 3, 10, 25, 30, 40, 45, 50, 80, 200, 300, 400, 500, 600, 700 and 800 s/mm2 were acquired. NEX was 2 for b=700 s/mm2 and b=800 s/mm2, and NSA =1 for other b-values. Other parameters included TR =2,149 ms, TE =55 ms, slice thickness =6 mm, matrix =100×116, FOV =360×300 mm, EPI factor =29, sensitivity-encoding (SENSE) factor =4, number-of-slices =26.
In both studies, the diffusion scan was based on a single-shot spin-echo type echo-planar sequence. Spectral Pre-saturation with Inversion-Recovery technique was used for fat suppression. The data of repeatability study was acquired later than the reproducibility study, and the b-value distribution for repeatability study is considered to be more ‘optimized’ where the highest b-value was 600 s/mm2 and b-value around 60 s/mm2 was more densely sampled, and 6 hours’ pre-scan fasting was adopted (11). Respiratory-gating was applied in all scans. For both 1.5T study and 3.0T study, the IVIM data acquisition time was around 5–6 min for one scan depending on the respiration cycle of the subjects. The study was conducted in accordance with the Declaration of Helsinki (as revised in 2013). The MRI data acquisition was approved by the local institutional ethics committees in Shenzhen and Nanjing, and informed consent was obtained from all subjects.
All data analysis was implemented in MATLAB (MathWorks, Natick, MA, USA). The IVIM analysis for Dslow, PF, and Dfast followed our previous descriptions (8,9,11). In brief, after MRI data acquisition, we performed a data quality assessment prior to IVIM analysis. Images with notable motion and artifacts were discarded. To avoid potential bias, once a dataset was included for including b=0 data analysis, this dataset would not be excluded for without b=0 data analysis which is the last step. ROIs were placed on b=0 s/mm2 image (for including b=0 data fitting) or on b=2 (or 3) s/mm2 image (for excluding b=0 data fitting) to cover a large portion of right liver parenchyma while avoiding large vessels and then copied to the images of other b-values of this slice. For ROI analysis, the IVIM parameters were calculated based on the mean signal intensity of the whole ROI. The mean of all included slice measurements was then regarded as the value of the examination, with the last step weighted by the ROI area of each slice. For repeatability analysis, the signal attenuation was modeled according to Eq. [1] or Eq. [2].
where SI(b) and SI(2) denote the signal-intensity acquired with the b-factor value of b and b=2 s/mm2, respectively. Both full fitting and segmented fitting were performed (18). For segmented fitting, the threshold b-value to separate the fast component and slow component was 60 s/mm2 (11,15). After imaging data considered of insufficient quality were discarded, data of 35 subjects and 35 paired scans (11 males, mean age: 41.82 years, range: 32–60 years; 24 females, mean age: 42.67 years, range: 20–71 years) were available for repeatability analysis (Figure 2A).
For reproducibility analysis, the signal attenuation was modeled according to Eq. [1] or Eq. [3].
where SI(b) and SI(3) denote the signal-intensity acquired with the b-factor value of b and b=3 s/mm2, respectively. Only segmented fitting was conducted, with the threshold b-value of 50 s/mm2 chosen. With full fitting, preliminary testing showed general fitting instability for the reproducibility data, which concur with previous reports and thus full fitting was not included for reproducibility data (18,19). Data of 11 subjects with 20 paired scans (4 males, mean age: 26.25 years, range: 25–27 years; 7 females, mean age: 25.57 years, range: 24–27 years) were available for reproducibility analysis (Figure 2B). Repeatability and reproducibility (i.e., stability) of PF, Dslow and Dfast were assessed by the within-subject standard deviation (wSD), Bland-Altman mean difference and 95% limits of agreements (BA-LA), and intraclass correlation coefficient (ICC). wSD is defined by Eq. [4]:
With n being the number of subjects (=35 or 20 in this study) and x1 and x2 are the duplicate parameter measurements for each subject. Statistical analysis was performed using GraphPad Prism Software (GraphPad Software Inc., San Diego, CA, USA).
Results
Bi-exponential decay fitting starting from a non-zero low b-value generally offered better fittings (Figure 3). The scan-rescan measures for repeatability are graphically shown in Figure 4 and Figure 5, and measures for reproducibility are graphically shown in Figure 6 and Figure 7. Graphical data suggest the most apparent improvement for without b=0 data analysis is seen with the stability of PF measure.
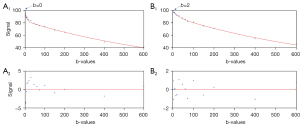
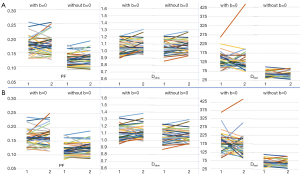
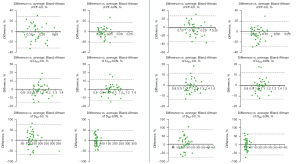
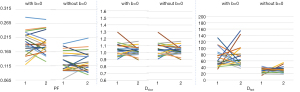
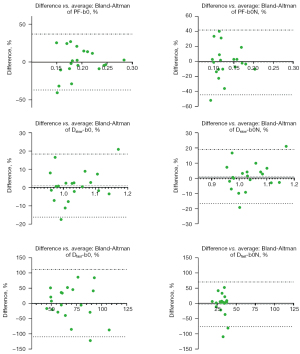
Repeatability and reproducibility quantitative results are shown in Table 1. Table 1 shows fitting without b=0 data generally improved the repeatability and reproducibility for all PF, Dslow, and Dfast. For the segmented fitting repeatability, by removing b=0 from analysis, ICC of PF improved from 0.647 to 0.837, wSD of Dslow improved from 0.054 to 0.047, and ICC of Dfast improved from 0.466 to 0.512. For reproducibility, by removing b=0 from analysis, ICC of PF improved from 0.671 to 0.738 and BA 95% limit for Dfast improved from −109.7% to 112.1% to −76.16% to 69.56%. Quantitative stability measures were better for repeatability than reproducibility (such as an ICC of 0.837 vs. 0.738). For repeatability, quantitative stability measures were marginally better for segmented fitting than for full fitting (such as an ICC of 0.837 vs. 0.789). It appears that the most notable improvement of stability by excluding b=0 data was seen with segmented fitting for repeatability. Though repeatability and reproducibility for Dfast generally improved by fitting without b=0, they remained suboptimal (Table 1).
Table 1
Fittings | Agreements | PF | Dslow | Dfast | |||||
---|---|---|---|---|---|---|---|---|---|
b=0 | No b=0 | b=0 | No b=0 | b=0 | No b=0 | ||||
Seg# (n=35) | wSD | 0.019 | 0.009§ | 0.054 | 0.047§ | 38.49 | 10.64§ | ||
BA % difference | −1.588 | −1.960 | −0.974 | −0.196§ | 4.698 | −1.281§ | |||
BA 95% limit | −31.52 to 28.35 | −20.78 to 16.86§ | −15.15 to 13.20 | −12.76 to 12.37§ | −66.98 to 76.37 | −51.47 to 48.91§ | |||
ICC | 0.647 | 0.837§ | 0.635 | 0.718§ | 0.466 | 0.512§ | |||
Full# (n=35) | wSD | 0.019 | 0.011§ | 0.049 | 0.048 | 50.51 | 13.03§ | ||
BA % difference | −2.275 | −4.135 | −0.444 | −0.011§ | 7.487 | 0.848§ | |||
BA 95% limit | −32.74 to 28.19 | −28.02 to 19.75§ | −12.84 to 11.95 | −12.62 to 12.60 | −72.98 to 87.96 | −61.12 to 62.82§ | |||
ICC | 0.662 | 0.789§ | 0.726 | 0.692 | 0.354 | 0.479§ | |||
Seg¶ (n=20) | wSD | 0.022 | 0.017§ | 0.065 | 0.066 | 32.39 | 8.80§ | ||
BA % difference | 0.074 | −1.227 | 0.982 | 1.072 | 1.236 | −3.301 | |||
BA 95% limit | −36.82 to 36.97 | −44.58 to 42.12 | −16.36 to 18.32 | −16.70 to 18.85 | −109.7 to 112.1 | −76.16 to 69.56§ | |||
ICC | 0.671 | 0.738§ | <0.4## | <0.4## | <0.4 | <0.4 |
For wSD, BA % difference, and BA 95% limit, a smaller value indicates a better scan-rescan stability. For ICC, a larger value indicates a better scan-rescan stability. §, indicates better scan-rescan stability by excluding b=0 from analysis. Note, wSD intrinsically favors measurements with a smaller value. ICC favors measurements with a larger ‘dynamic range’. The quantitative performances for repeatability or reproducibility as shown in this table should be considered comprehensively (rather than individually). b=0: b=0 data is included for biexponential fitting; No b=0: b=0 data is not included for biexponential fitting; #, repeatability measures; ¶, reproducibility measures; ##, note the dynamic range of Dslow is very small. IVIM, intravoxel incoherent motion; n, number of scan pairs used for repeatability or reproducibility analysis; Seg, segmented fitting; Full, full fitting; wSD, within-subject standard deviation; BA, Bland and Altman; ICC, intraclass correlation coefficient.
Discussion
Accurate liver IVIM quantification is known to be challenging (6,20). The current study tested the hypothesis that IVIM data fitting by excluding b=0 data improves the stability of liver IVIM measurement. As expected, except Dslow, this study shows PF values computed without b=0 data were modestly smaller than the values computed with b=0 data; Dfast values computed without b=0 data were substantially smaller than the values computed with b=0 data. As can be seen from Eq. [4], wSD intrinsically favors measurements with a smaller value. On the other hand, ICC favors measurements with a larger ‘dynamic range’. Dslow has a very small ‘dynamic range’ and tends to only fluctuate around 1.0 for healthy subjects. Thus, despite good reproducibility, the Dslow reproducibility ICCs for measures with b=0 data and without b=0 data were both <0.4. Therefore, the quantitative performances for repeatability or reproducibility as shown in Table 1 should be considered comprehensively (rather than individually).
In this study, using two data sets acquired at two magnet field strengths and at two sites, and with slightly different b-value distributions, we confirmed that excluding b=0 image data improves scan-rescan stability of liver IVIM estimations, despite there was one data point fewer for without b=0 data fitting than for including b=0 data fitting (i.e., 15 datapoints for without b=0 data fitting and 16 datapoints for including b=0 data fitting). The improvement seemed more apparent with PF and when the b-value distribution is more ‘optimized’ (i.e., that of repeatability study). In liver IVIM studies, it has been repeatedly demonstrated that PF is the most important parameter (3,21-23). Dslow suffers from a small dynamic range, and Dfast suffers from poor stability (6,24). While it has been well demonstrated that liver fibrosis is associated with progressive reduction of all three IVIM parameters, most reports by other authors did not show reliable detection of early-stage liver fibrosis (6). It is expected that this improved measure reproducibility by excluding b=0 data had contributed to our good results that, in three medium-sized studies, IVIM analysis almost completely separated all non-fibrotic livers and fibrotic livers (including those of mild stage fibrosis) (7-9). In our earlier analysis, we showed that, analysis without b=0 data offered a smaller CoV (coefficient-of-variation) for IVIM parameters in a cohort of healthy volunteers, and also a bigger difference between patients’ measure and volunteers’ measure (9). It is noted that, in addition to us, other authors have also adopted IVIM fitting without b=0 data, such as the recent work of Koopman et al. on head and neck imaging (25). Gambarota et al. (26) proposed to remove vessel pixels firstly, and then perform diffusion analysis assuming the remaining liver parenchyma without vessel pixels following a bi-exponential model. However, due to the existence of sub-pixel small vessels, even after vessel pixels are removed from the analysis, the remaining liver parenchyma will still follow a tri-exponential decay pattern (14).
Another point of note is that full fitting is generally considered to be unstable and not commonly used in liver studies (18,19). However, in this study with our ‘optimised’ b-value selection and image analysis, the full fitting repeatability was only marginally inferior to segmented fitting repeatability (Table 1). On the other hand, while fitting without b=0 data appeared to have improved measure stability for Dfast, the final results remain suboptimal in this study, with relatively large wSD, large BA difference, large BA 95% agreement limit, and ICC equaled 0.512 for repeatability study, was <0.4 for reproducibility study. According to Fleiss (27), an ICC value of <0.4 represents poor agreement, a value >0.75 represents good agreement and a value between 0.4 and 0.75 represents fair to moderate agreement. However, it is also possible that, Dfast measures fitted without b=0 data reduced dynamic range, thus potentially can avoid artificial ‘large fluctuations’ (6,24).
On DWI, blood vessels show a high signal when there is no diffusion gradient (b=0 s/mm2) and a low signal even when very low b-values (e.g., 1 s/mm2) are applied. Therefore, the signal difference between images when the diffusion gradient is off and images when the diffusion gradient is on reflects the extent of tissue vessel density (referred to as diffusion-derived vessel density, DDVD) (11-13). It has been shown that DDVD is a useful biomarker for the separation of livers with and without fibrosis, with liver fibrosis associated with a lower DDVD (13,28). According to the original definition, PF which can reflect total hepatic perfusion volume, is estimated to be around 18% (6,29,30). As noted earlier, liver perfusion and diffusion are better measured by DWI with a tri-exponential decay model, where perfusion related diffusion is mathematically (though not anatomically) divided into a very fast compartment and a fast compartment (17,18). The very fast compartment represents the initial very fast signal drop from b=0 to very low b-values, and it is highly unstable during IVIM modeling (17,18). In the recent study of Riexinger et al. (31), 24 b-values were applied: 0.2, 0.4, 0.7, 0.8, 1.1, 1.7, 3, 3.8, 4.1, 4.3, 4.4, 4.5, 4.9, 10, 15, 20, 30, 50, 60, 90, 95, 150, 180 and 500 s/mm2. However, this approach of applying multiple very low b-values to measure the very fast compartment is only feasible in dedicated research scanners. In our study when b=0 data was not used in the IVIM analysis, the very fast compartment is not fully captioned; thus the PF values derived from without b=0 analysis were lower than the physiological value of 18%. Our approach constitutes a removal or a partial removal of the highly instable very fast compartment from the perfusion related diffusion IVIM calculation. We can argue that the DWI signal difference between b=0 and b=2 (or b=3), which are measured by DDVD in our studies (13,28), partially reflects the very fast compartment. On the other hand, as shown in our earlier studies, it appears that measuring the fast component only (while without measuring the vary fast component) may be sufficient to differentiate fibrotic livers from non-fibrotic livers in most of the cases (7-9). It is very difficult to precisely measure the very last compartment by IVIM modeling using routine clinical MRI scanners (3). A highly instable very fast compartment’s contribution to the IVIM parameters derived from with b=0 analysis may compromise the utility of liver IVIM imaging in clinical practice.
There are a number of limitations for this study. The stability for Dfast remains suboptimal in this study. This is a well-known limitation of IVIM analysis, and thus even denser sampling of very low b-value may be required for stable Dfast fitting (18). Secondly, this study adopted conventional IVIM segmented fitting and full fitting, it is possible in some cases better results can be obtained by Bayesian analysis (32-35). Thirdly, for the reproducibility study, we didn’t ask volunteers to fast before the MRI examination (36). Fourthly, before computing the IVIM results, we had a process to remove subjects or slices of insufficient data quality. Till now this remained subjective decision for which image data to include and which image data to exclude, and we are currently working to decrease the ratio for unused data, including taking measures of Bayesian analysis, de-noising, and better image registration. After these steps, we plan to develop objective criteria to determine which image data can be used and which image data should be rejected. However, the current subjectivity would not affect the main conclusion drawn in this study, as this study is on the comparisons of healthy subjects’ results with b=0 calculation vs. results without b=0 calculation. Once one image datum was included for including b=0 data analysis, this image datum would not be excluded for without b=0 data analysis. It has been noted that magnetic field difference will have some implications for IVM results. It has been noted that 1.5T scanners’ results have higher Dslow and lower PF, while 3.0T scanners’ results have lower Dslow and higher PF (6,31). This study did not aim to directly compare the scan-rescan stability of a 1.5 scanner vs. a 3T scanner.
In conclusion, we demonstrated the proof-of-principle that the scan-rescan repeatability and reproducibility of IVIM parameters can be improved by bi-exponential fitting without b=0 data, which is particularly so for the most important IVIM parameter of PF. This approach constitutes a removal of the highly instable very fast compartment from the perfusion related diffusion IVIM calculation. We suggest that this improved measure stability may contribute to a better diagnostic performance of IVIM parameters for liver pathologies.
Acknowledgments
Funding: This study was partially supported by Hong Kong GRF Projects (Nos. 14109218 and 14112521).
Footnote
Conflicts of Interest: All authors have completed the ICMJE uniform disclosure form (available at https://qims.amegroups.com/article/view/10.21037/qims-2022-02/coif). Author YXJW serves as the Editor-in-Chief of Quantitative Imaging in Medicine and Surgery. Author YXJW is the founder of Yingran Medicals Co., Ltd., which develops medical image-based diagnostics software. The other authors have no conflicts of interest to declare.
Ethical Statement: The authors are accountable for all aspects of the work in ensuring that questions related to the accuracy or integrity of any part of the work are appropriately investigated and resolved. The study was conducted in accordance with the Declaration of Helsinki (as revised in 2013). The MRI data acquisition was approved by the local institutional ethics committees in Shenzhen and Nanjing, and informed consent was obtained from all subjects.
Open Access Statement: This is an Open Access article distributed in accordance with the Creative Commons Attribution-NonCommercial-NoDerivs 4.0 International License (CC BY-NC-ND 4.0), which permits the non-commercial replication and distribution of the article with the strict proviso that no changes or edits are made and the original work is properly cited (including links to both the formal publication through the relevant DOI and the license). See: https://creativecommons.org/licenses/by-nc-nd/4.0/.
References
- Le Bihan D, Turner R, Moonen CT, Pekar J. Imaging of diffusion and microcirculation with gradient sensitization: design, strategy, and significance. J Magn Reson Imaging 1991;1:7-28. [Crossref] [PubMed]
- Iima M, Le Bihan D. Clinical Intravoxel Incoherent Motion and Diffusion MR Imaging: Past, Present, and Future. Radiology 2016;278:13-32. [Crossref] [PubMed]
- Wang YXJ, Huang H, Zheng CJ, Xiao BH, Chevallier O, Wang W. Diffusion-weighted MRI of the liver: challenges and some solutions for the quantification of apparent diffusion coefficient and intravoxel incoherent motion. Am J Nucl Med Mol Imaging 2021;11:107-42. [PubMed]
- Federau C. Intravoxel incoherent motion MRI as a means to measure in vivo perfusion: A review of the evidence. NMR Biomed 2017; [Crossref] [PubMed]
- Padhani AR, Liu G, Koh DM, Chenevert TL, Thoeny HC, Takahara T, Dzik-Jurasz A, Ross BD, Van Cauteren M, Collins D, Hammoud DA, Rustin GJ, Taouli B, Choyke PL. Diffusion-weighted magnetic resonance imaging as a cancer biomarker: consensus and recommendations. Neoplasia 2009;11:102-25. [Crossref] [PubMed]
- Li YT, Cercueil JP, Yuan J, Chen W, Loffroy R, Wáng YX. Liver intravoxel incoherent motion (IVIM) magnetic resonance imaging: a comprehensive review of published data on normal values and applications for fibrosis and tumor evaluation. Quant Imaging Med Surg 2017;7:59-78. [Crossref] [PubMed]
- Wáng YXJ, Deng M, Li YT, Huang H, Leung JCS, Chen W, Lu PX. A Combined Use of Intravoxel Incoherent Motion MRI Parameters Can Differentiate Early-Stage Hepatitis-b Fibrotic Livers from Healthy Livers. SLAS Technol 2018;23:259-68. [Crossref] [PubMed]
- Huang H, Che-Nordin N, Wang LF, Xiao BH, Chevallier O, Yun YX, Guo SW, Wáng YXJ. High performance of intravoxel incoherent motion diffusion MRI in detecting viral hepatitis-b induced liver fibrosis. Ann Transl Med 2019;7:39. [Crossref] [PubMed]
- Li T, Che-Nordin N, Wáng YXJ, Rong PF, Qiu SW, Zhang SW, Zhang P, Jiang YF, Chevallier O, Zhao F, Xiao XY, Wang W. Intravoxel incoherent motion derived liver perfusion/diffusion readouts can be reliable biomarker for the detection of viral hepatitis B induced liver fibrosis. Quant Imaging Med Surg 2019;9:371-85. [Crossref] [PubMed]
- Chevallier O, Zhou N, He J, Loffroy R, Wáng YXJ. Removal of evidential motion-contaminated and poorly fitted image data improves IVIM diffusion MRI parameter scan-rescan reproducibility. Acta Radiol 2018;59:1157-67. [Crossref] [PubMed]
- Huang H, Zheng CJ, Wang LF, Che-Nordin N, Wáng YXJ. Age and gender dependence of liver diffusion parameters and the possibility that intravoxel incoherent motion modeling of the perfusion component is constrained by the diffusion component. NMR Biomed 2021;34:e4449. [Crossref] [PubMed]
- Wáng YXJ. Living tissue intravoxel incoherent motion (IVIM) diffusion MR analysis without b=0 image: an example for liver fibrosis evaluation. Quant Imaging Med Surg 2019;9:127-33. [Crossref] [PubMed]
- Xiao BH, Huang H, Wang LF, Qiu SW, Guo SW, Wáng YXJ. Diffusion MRI Derived per Area Vessel Density as a Surrogate Biomarker for Detecting Viral Hepatitis B-Induced Liver Fibrosis: A Proof-of-Concept Study. SLAS Technol 2020;25:474-83. [Crossref] [PubMed]
- Wáng YXJ, Wang X, Wu P, Wang Y, Chen W, Chen H, Li J. Topics on quantitative liver magnetic resonance imaging. Quant Imaging Med Surg 2019;9:1840-90. [Crossref] [PubMed]
- Wáng YXJ, Li YT, Chevallier O, Huang H, Leung JCS, Chen W, Lu PX. Dependence of intravoxel incoherent motion diffusion MR threshold b-value selection for separating perfusion and diffusion compartments and liver fibrosis diagnostic performance. Acta Radiol 2019;60:3-12. [Crossref] [PubMed]
- Yuan J, Wong OL, Lo GG, Chan HH, Wong TT, Cheung PS. Statistical assessment of bi-exponential diffusion weighted imaging signal characteristics induced by intravoxel incoherent motion in malignant breast tumors. Quant Imaging Med Surg 2016;6:418-29. [Crossref] [PubMed]
- Chevallier O, Wáng YXJ, Guillen K, Pellegrinelli J, Cercueil JP, Loffroy R. Evidence of Tri-Exponential Decay for Liver Intravoxel Incoherent Motion MRI: A Review of Published Results and Limitations. Diagnostics (Basel) 2021;11:379. [Crossref] [PubMed]
- Chevallier O, Zhou N, Cercueil JP, He J, Loffroy R, Wáng YXJ. Comparison of tri-exponential decay versus bi-exponential decay and full fitting versus segmented fitting for modeling liver intravoxel incoherent motion diffusion MRI. NMR Biomed 2019;32:e4155. [Crossref] [PubMed]
- Park HJ, Sung YS, Lee SS, Lee Y, Cheong H, Kim YJ, Lee MG. Intravoxel incoherent motion diffusion-weighted MRI of the abdomen: The effect of fitting algorithms on the accuracy and reliability of the parameters. J Magn Reson Imaging 2017;45:1637-47. [Crossref] [PubMed]
- Andreou A, Koh DM, Collins DJ, Blackledge M, Wallace T, Leach MO, Orton MR. Measurement reproducibility of perfusion fraction and pseudodiffusion coefficient derived by intravoxel incoherent motion diffusion-weighted MR imaging in normal liver and metastases. Eur Radiol 2013;23:428-34. [Crossref] [PubMed]
- Joo I, Lee JM, Yoon JH, Jang JJ, Han JK, Choi BI. Nonalcoholic fatty liver disease: intravoxel incoherent motion diffusion-weighted MR imaging-an experimental study in a rabbit model. Radiology 2014;270:131-40. [Crossref] [PubMed]
- Granata V, Fusco R, Catalano O, Filice S, Amato DM, Nasti G, Avallone A, Izzo F, Petrillo A. Early Assessment of Colorectal Cancer Patients with Liver Metastases Treated with Antiangiogenic Drugs: The Role of Intravoxel Incoherent Motion in Diffusion-Weighted Imaging. PLoS One 2015;10:e0142876. [Crossref] [PubMed]
- Shirota N, Saito K, Sugimoto K, Takara K, Moriyasu F, Tokuuye K. Intravoxel incoherent motion MRI as a biomarker of sorafenib treatment for advanced hepatocellular carcinoma: a pilot study. Cancer Imaging 2016;16:1. [Crossref] [PubMed]
- Zhang Q, Wang YX, Ma HT, Yuan J. Cramér-Rao bound for Intravoxel Incoherent Motion Diffusion Weighted Imaging fitting. Annu Int Conf IEEE Eng Med Biol Soc 2013;2013:511-4. [PubMed]
- Koopman T, Martens R, Gurney-Champion OJ, Yaqub M, Lavini C, de Graaf P, Castelijns J, Boellaard R, Marcus JT. Repeatability of IVIM biomarkers from diffusion-weighted MRI in head and neck: Bayesian probability versus neural network. Magn Reson Med 2021;85:3394-402. [Crossref] [PubMed]
- Gambarota G, Hitti E, Leporq B, Saint-Jalmes H, Beuf O. Eliminating the blood-flow confounding effect in intravoxel incoherent motion (IVIM) using the non-negative least square analysis in liver. Magn Reson Med 2017;77:310-7. [Crossref] [PubMed]
- Fleiss JL. Reliability of measurement: the design and analysis of clinical experiments. New York, NY: John Wiley & Sons, 1986.
- Hu GW, Zheng CJ, Zhong WX, Zhuang DP, Xiao BH, Wáng YXJ. Usefulness of diffusion derived vessel density computed from a simplified IVIM imaging protocol: An experimental study with rat biliary duct blockage induced liver fibrosis. Magn Reson Imaging 2021;84:115-23. [Crossref] [PubMed]
- Greenway CV, Stark RD. Hepatic vascular bed. Physiol Rev 1971;51:23-65. [Crossref] [PubMed]
- Strandell T, Erwald R, Kulling KG, Lundbergh P, Marions O, Wiechel KL. Measurement of dual hepatic blood flow in awake patients. J Appl Physiol 1973;35:755-61. [Crossref] [PubMed]
- Riexinger AJ, Martin J, Rauh S, Wetscherek A, Pistel M, Kuder TA, Nagel AM, Uder M, Hensel B, Müller L, Laun FB. On the Field Strength Dependence of Bi- and Triexponential Intravoxel Incoherent Motion (IVIM) Parameters in the Liver. J Magn Reson Imaging 2019;50:1883-92. [Crossref] [PubMed]
- Neil JJ, Bretthorst GL. On the use of Bayesian probability theory for analysis of exponential decay data: an example taken from intravoxel incoherent motion experiments. Magn Reson Med 1993;29:642-7. [Crossref] [PubMed]
- Barbieri S, Donati OF, Froehlich JM, Thoeny HC. Impact of the calculation algorithm on biexponential fitting of diffusion-weighted MRI in upper abdominal organs. Magn Reson Med 2016;75:2175-84. [Crossref] [PubMed]
- Troelstra MA, Witjes JJ, van Dijk AM, Mak AL, Gurney-Champion O, Runge JH, Zwirs D, Stols-Gonçalves D, Zwinderman AH, Ten Wolde M, Monajemi H, Ramsoekh S, Sinkus R, van Delden OM, Beuers UH, Verheij J, Nieuwdorp M, Nederveen AJ, Holleboom AG. Assessment of Imaging Modalities Against Liver Biopsy in Nonalcoholic Fatty Liver Disease: The Amsterdam NAFLD-NASH Cohort. J Magn Reson Imaging 2021;54:1937-49. [Crossref] [PubMed]
- Wáng YXJ, Xiao BH, Zheng CJ, Li T, Che-Nordin N, Wang W. More promising results of liver intravoxel incoherent motion imaging analysis for the assessment of nonalcoholic steatohepatitis and fibrosis. Ann Transl Med 2021;9:1283. [Crossref] [PubMed]
- Regini F, Colagrande S, Mazzoni LN, Busoni S, Matteuzzi B, Santini P, Wyttenbach R. Assessment of Liver Perfusion by IntraVoxel Incoherent Motion (IVIM) Magnetic Resonance-Diffusion-Weighted Imaging: Correlation With Phase-Contrast Portal Venous Flow Measurements. J Comput Assist Tomogr 2015;39:365-72. [Crossref] [PubMed]