Intraindividual difference between supraclavicular and subcutaneous proton density fat fraction is associated with cold-induced thermogenesis
Introduction
Brown adipose tissue (BAT) differs from white adipose tissue (WAT) in its distribution, morphology, and function. While white adipocytes are primarily responsible for energy storage, brown adipocytes are highly specified to produce heat for human thermoregulation by non-shivering thermogenesis (1). The supraclavicular fossa represents a typical adipose tissue depot containing BAT, WAT, as well as white adipocytes undergoing browning—a crossover with ability of both thermoregulation and energy storage depending on external regulation and often referred to as beige adipocytes (2,3). In general, it is a matter of debate as to whether BAT may play a role in human energy metabolism. However, in an activated state, BAT can increase energy expenditure by up to 400 kilocalories (kcal) per day (4,5) and may reduce insulin resistance by meal induced glucose consumption and triglyceride clearance (6,7). Since obesity plays a leading role in public health (8), those findings indicate BAT as a promising treatment target (9). BAT activation can also be induced by cold exposure, resulting in an increase of resting energy expenditure (REE) (10), which is especially triggered by activation of the sympathetic nervous system (11). This increase in calorie combustion is measured by indirect calorimetry and referred to as cold-induced thermogenesis (CIT) (12).
Fluorine-18 fluorodeoxyglucose (18F-FDG) positron emission tomography (PET) is considered as the gold standard method for non-invasive BAT detection (13), even though its use is limited due to exposure to ionizing radiation, the type of tracer used (mainly glucose), and the method being bound to a metabolically active status of BAT. Magnetic resonance imaging (MRI) is an upcoming method for BAT imaging, mainly using water-fat imaging techniques to calculate tissue fat fraction both statically (14,15) and dynamically (16-23). Working with cooling protocols for BAT activation, while simultaneously observing changes in fat fraction, multiple studies have reported significant drops in fat fraction when observing BAT depots during cold exposure (16-23). In addition, fat fraction mapping at thermoneutral conditions rests on morphometric differences in adipose tissue types, with BAT exhibiting a lower fat fraction than WAT (15,24), rendering MRI a potential modality for BAT detection regardless of its activation state (25). By now, the lower fat fraction of BAT has been validated in humans by histological and immunohistochemical confirmation (26,27) and by PET (25,28,29). Advances in fat fraction mapping rely on the extraction of the proton density fat fraction (PDFF), a standardized quantitative MRI-parameter based on a chemical-shift encoding-based water-fat separation, additionally accounting for known confounding factors (multiple fat peaks, T2* decay, T1 bias-effects). PDFF is already well established in MR-based metabolic phenotyping (30), for example in liver (31) and bone marrow imaging (32). A consensus implemented PDFF as MR-based biomarker for standardized fat quantification (33) and some studies have employed PDFF to study human supraclavicular fat (34-38). Consequently, exploring the quantitative relation of CIT and supraclavicular PDFF has been of rising interest, but so far no stable quantitative correlation of PDFF and CIT has been reported (39).
The lack in consistent outcomes in supraclavicular PDFF mapping could be based upon some confounding factors: First, supraclavicular fat is a mixture of brown, beige, and white adipocytes in varying proportions (40,41) and therefore BAT is hard to detect via MRI with limited resolution and partial volume effects embedded in complex anatomy. Thus, results are highly dependent on segmentation methods, for example small manually selected regions of interest (ROI) compared to segmenting the entire supraclavicular fossa. Second, adipose tissue PDFF in itself, both in potentially BAT-containing areas and in pure WAT, can differ noticeably between individuals and shows correlations to body mass index (BMI) and other anthropometric markers (34). Persons with overweight display a greater proportion of WAT, impairing the sensitivity of supraclavicular PDFF measurements.
In the present study, a breathing-triggered acquisition, and a reproducible segmentation technique, covering the whole supraclavicular fossa, are combined with referencing supraclavicular PDFF to gluteal subcutaneous PDFF, to compensate for interindividual and body compositional PDFF variability. The main purpose of the present work was to investigate the association between supraclavicular PDFF and CIT, while considering adiposity and gluteal subcutaneous PDFF as confounding factors.
We present the following article in accordance with the STROBE reporting checklist (available at https://qims.amegroups.com/article/view/10.21037/qims-21-986/rc).
Methods
Study design
For this bicentric prospective cross-sectional study conducted at the Technical University Munich, adult volunteers were recruited from a cross-sectional study implemented by the Institute for Nutritional Medicine, School of Medicine, Technical University of Munich (42), which investigated the impact of cold exposure on REE. All procedures performed in this study were conducted in accordance with the Declaration of Helsinki (as revised in 2013). Study protocols and procedures were approved by the Ethics Committee of the Faculty of Medicine of the Technical University of Munich, Germany. And informed consent was taken from all individual participants. The study was registered at the German Clinical Register DRKS (accession number: DRKS00016629).
Participants
Inclusion criteria were defined as healthy, non-smoking, less than 10 h of vigorous exercise per week, Caucasian descent, and stable body weight within the last three months (<3 kg change in body weight). Pregnant and breast-feeding women were excluded. REE measurements were conducted from October 2016 to February 2020. From January 2019 to January 2020, 174 participants of the first study part received an invitation for additional MRI examination. Forty-one participants accepted invitation, were screened for standard contraindications for MRI and an appointment for MRI examination was set for the next possible date. One participant was excluded due to MRI contraindications. Thus, MRI data was gathered for 40 participants. One of whom showed outlying CIT values in the Grubb’s test was excluded from the current analysis, leaving 39 participants (24 female, 15 male) for statistical analysis. Characteristics of the final cohort are presented in Table 1. Due to the study design, time periods in between both appointments show a wide variation with a median of 411 days (−7 to 928 days) and a flow chart illustrating the recruitment process can be found in the supplementary material (Figure S1).
Table 1
Characteristics | Value |
---|---|
N | 39 |
Female, n (%) | 24 (61.5) |
Normally distributed, mean ± SD [range] | |
Weight, kg | 77.3±17.1 [50.1–121.4] |
Height, cm | 174.3±10.5 [157.2–195.2] |
BF%, % | 25.2±11.6 [4.6–45.3] |
TF%, % | 30.0±11.7 [11.4–67.2] |
CIT, % | 4.7±9.0 [−10.6 to 24.3] |
Not normally distributed | |
Age, years | 27 [20–53] |
BMI, kg/m2 | 23.2 [19.0–38.5] |
SAT volume (normalized to trunk length), mL/cm | 114.6 [28.6–447.8] |
VAT volume (normalized to trunk length), mL/cm | 23.3 [4.0–184.2] |
Supraclavicular PDFF, % | 82.5 [69.7–88.1] |
Gluteal PDFF, % | 91.1 [82.3–93.5] |
Delta PDFF, % | 8.8 [3.9–21.9] |
BF%, body fat percentage; BMI, body mass index; CIT, cold induced thermogenesis; n, number of participants; PDFF, proton density fat fraction; SAT, subcutaneous adipose tissue; TF%, trunk fat percentage; VAT, visceral adipose tissue; SD, standard deviation.
Anthropometric measurements
Body height was measured with a stadiometer (Seca, Hamburg, Germany). Body weight and body composition were measured by bioelectrical impedance analysis (BIA) on a TANITA Body Composition Analyzer Type BC-418 MA (Tanita Europe GmbH, Sindelfingen, Germany). Measurements were performed barefoot and in underwear, on the same day as the CIT examination. BMI was calculated based on weight and height. Body fat percentage (BF%) was calculated as the ratio of body fat mass in kilograms to body weight in kilograms.
CIT measurements
REE was assessed by indirect calorimetry, under thermoneutral conditions (REETN) and under cold exposure (REECE). For indirect calorimetry, measuring consumption of oxygen (VO2) and production of carbon dioxide (VCO2), a ventilated canopy hood system by Cosmed (COSMED Quark RMR, Fridolfing, Germany) was used. Under both thermal conditions, measurements were performed over a time frame of 30 min, during which individuals should reach steady state. Steady state was defined as stable period of at least 4 min with less than 10% variance in VO2 and VCO2. The respiratory quotient (RQ) was calculated as the ratio of VCO2 and VO2. Energy expenditure was calculated according to the shortened Weir equation (43).
Controlled thermal environment was achieved utilizing a combination of a water perfused blanket and mattress, allowing a precise regulation of water temperature. First REETN was assessed with water temperature set to 32 ℃. Afterwards cold exposure was conducted by an individualized cooling protocol, guaranteeing participants were kept above their individual shivering threshold. Water temperature was reduced gradually until when shivering occurred water temperature was raised by 2 ℃. Thus, determined individual exposure temperature was maintained for 120 min, before REECE was assessed. The approach was described in detail elsewhere (42). For the calculation of CIT, REETN was used as baseline and was set in relation to the difference of REETN and REECE, generating CIT values expressed in %.
MRI measurements
Participants underwent an MRI of the neck and abdomen/pelvis on a 3T-system (Elition, Philips Healthcare, Best, Netherlands) in an air-conditioning-controlled scanner room with a steady ambient temperature of 21 ℃, using a head-neck-coil and a combination of anterior and posterior coil arrays, respectively. Time for adequate acclimatization was given before the scan while the procedure was explained, and final consent was collected.
Supraclavicular and gluteal PDFF mapping
An axial six-echo multi-echo 3D-spoiled gradient echo sequence with bipolar readouts was acquired, of both the neck and pelvic region. A six-echo acquisition was employed to correct for T2* decay effects and extract the PDFF (instead of a signal-weighted fat fraction) in the supraclavicular and gluteal regions (14,44,45). Based on localizer sequences, scans were carefully planned. The neck sequence covered the supraclavicular fat depot including the coracoid process and vocal cords. The pelvic sequence was positioned in the upper pelvic region, for detailed imaging of subcutaneous adipose tissue (SAT) posterior of the iliac bone, corresponding to the gluteal region. Pelvic scans were performed during free breathing, whereas the neck region required respiratory triggering to reduce motion artifacts. The respiratory motion was monitored constantly by breathing belt measurements. Acquisitions only took place in expiration. Imaging parameters are listed in Table 2. After phase error correction, the multi-echo data was processed using a complex-based water-fat separation algorithm accounting for known confounding factors (multi-peak fat spectrum and single T2* decay correction) using the online vendor’s algorithms, resulting in PDFF maps of neck and upper pelvis (46). The multi-peak fat spectrum from (47) was employed.
Table 2
Parameters | Two-echo Dixon, SAT/VAT volume | Six-echo Dixon, supraclavicular PDFF | Six-echo Dixon, gluteal PDFF |
---|---|---|---|
TR, ms | 4.0 | 7.8 | 8.3 |
TE1/TE2/ΔTE, ms | TE1 =1.32 | TE1 =1.24 | TE1 =1.34 |
TE2 =2.60 | ΔTE =1.00 | ΔTE =1.10 | |
Flip angle | 10° | 5° | 5° |
Bandwidth, Hz/pixel | 1,004 | 1,413 | 1,437 |
Acquisition matrix size | 332×219×44 | 268×201×93 | 200×267×40 |
Reconstruction matrix size | 512×512 | 288×288 | 320×320 |
Field of view (FOV), mm3 | 500×445×220 | 400×300×140 | 400×400×60 |
Acquisition voxel | 1.5×2.03×5.0 | 1.5×1.5×1.5 | 1.5×1.5×1.5 |
Reconstructed voxel, mm3 | 0.98×0.98×5.00 | 1.389×1.389×1.500 | 1.25×1.25×1.50 |
Slices | 44 | 93 | 40 |
SENSE reduction factor | 2.5x1.0 (AP 2.5) | 2.5×1.0 (AP 2.5) | 2.5×1.0 (LR 2.5) |
Partial Fourier | 075×0.80 | No | No |
Respiratory triggering | No | Yes | No |
Scan time | 10 s | 2 min 30 s | 46 s |
TE1, first echo time; TE2, second echo time; ΔTE, difference between echo times. AP, anterior posterior; FOV, field of view; LR, left right; MR, magnetic resonance; PDFF, proton density fat fraction; SAT, subcutaneous adipose tissue; TR, repetition time; TE, echo time; SENSE, sensitivity encoding; VAT, visceral adipose tissue.
Two stacks of axial two-echo Dixon 3D spoiled gradient echo images were acquired to cater information about abdominal SAT and visceral adipose tissue (VAT) volumes. The sequence covered the trunk at least from liver dome to femoral head. Data was acquired while participants performed breath-holds (10.6 s for each stack).
Imaging data analysis
Supraclavicular and gluteal PDFF maps segmentation
Manual segmentation work was conducted using ITK-SNAP 3.8.0 (www.itksnap.org) (48), by an experienced researcher (fifth year of medical training). Supraclavicular PDFF was assessed segmenting the supraclavicular fat depot bilaterally. First, the ROI was manually outlined on every second slice in axial plane, starting from the coracoid process, which was defined as the caudal border and ending at the vocal cords, representing the cranial border. With interpolation a 3D-mask was created, generating the volume of interest (VOI). Non-adipose tissue was excluded applying a PDFF- and T2*-threshold (Python language version 2.7; Python Software Foundation, https://www.python.org/). PDFF values below 50% were excluded, ruling out muscle, vessels and lymph nodes typically exhibiting lower PDFF values. Bone was excluded by excluding T2* values lower than 5 ms. The here assessed T2*-values are derived from a water-fat signal model assuming a common T2* decay for both the water and fat components, which is sufficient for T2* decay correction and segmentation. Finalizing the segmentation mask, an erosion step (using a spherical kernel and one iteration) was executed in MATLAB (MathWorks, Natick, MA, USA) to reduce partial volume effects. An example is shown in Figure 1.
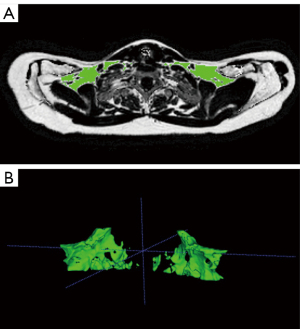
The approach was examined by the means of inter-rater reliability in a subgroup of five participants. Manual segmentation was performed independently by three different raters: two researchers (fifth year of medical training) and one board-certified radiologist (6 years of experience in whole-body imaging), achieving high agreement and reproducibility with an intraclass correlation coefficient of 0.97 (95% confidence interval: 0.73 to 1.0).
Deep gluteal subcutaneous fat was segmented semi-automatically similar to the supraclavicular fossa. Bilateral manual crude delineation of deep subcutaneous fat tissue was performed on every second slice outlined a ROI posterior of the iliac bone but deep to the great body fascia—Scarpa’s fascia, to solely include deep gluteal SAT. Deep gluteal SAT is characterized by large white adipocytes and widely spaced fascial septa (49) and considered a reliable WAT depot for adipose tissue imaging without partial voluming even in lean individuals. Upper and lower border were defined by the first and last cut through the fifth lumbar vertebra. Interpolation generated the VOI. The automated post processing was performed according to the supraclavicular segmentation work, adding a PDFF- (PDFF >50%) and T2*-threshold (T2* >5 ms) and erosion step (sphere, one iteration).
Mean supraclavicular PDFF (PDFFSUPRACLAVICULAR) and mean gluteal PDFF (PDFFGLUTEAL) were extracted, and delta PDFF (∆PDFF) was calculated as the difference of both mean values (PDFFGLUTEAL − PDFFSUPRACLAVICULAR).
SAT and VAT volumes segmentation
SAT and VAT volumes were extracted from the water- and fat-separated images of the two-echo Dixon scans, using a custom-built semi-automatic post-processing algorithm (https://github.com/BMRRgroup/vatsatseg). The segmentation was performed as described in earlier studies (34,50,51). The upper border of the segmentation was represented by the liver dome and the lower border by the center of the femoral head. In order to control for height differences, adapted SAT and VAT volumes were calculated taking body height into account (34,52). SAT and VAT volumes in ml were divided by lower trunk length (from liver dome to femoral head) in cm, resulting in adapted values in mL/cm. As a substitute for BF% assessed by whole body MRI, an approximation the lower trunk fat percentage (TF%) was calculated as the sum of VAT and SAT volumes divided by total lower trunk volume.
Subgroup definition and statistical analysis
According to WHO guidelines subgroups were defined by BMI: non-overweight (BMI <25 kg/m2), overweight (BMI ≥25 kg/m2) (53). Further subgroups were built by gender adapted BF%-cutoffs according to several publications (54-56): normal BF% (male BF% <25%, female BF% <30%), high BF% (male BF% ≥25%, female BF% ≥30%).
Statistical analysis was conducted using IBM SPSS Statistics for MacOS, version 26 (IBM Corp., Armonk, NY, USA). Testing was performed on a significance level of 0.05 (two-sided). Due to the explorative study design no correction for multiple testing was executed. Measured parameters were non-normally distributed, except for weight, height, CIT, BF% and TF%. Therefore, the majority of the data was expressed as median and range in parentheses. Normally distributed parameters were expressed as mean ± standard deviation and range in parentheses. Non-parametric testing (Wilcoxon) was used for comparison of PDFFSUPRACLAVICULAR to PDFFGLUTEAL. All correlation analyses were conducted with the help of Spearman’s rho correlation coefficient. Partial correlation analysis was performed as partial rank correlation based on Spearman’s rho.
Results
Median age was 27 years (20–53 years). Participants showed a broad range of BMI with a median of 23.2 kg/m2 (19.0–38.5 kg/m2). Mean BF% was 25.2%±11.6% (4.6–45.3%). PDFFSUPRACLAVICULAR with 82.5% (69.7–88.1%) was significantly lower than PDFFGLUTEAL with 91.1% (82.3–93.5%) (P<0.0001). The PDFF difference of the two compartments is illustrated in the PDDF maps of a single person in Figure 2. For ∆PDFF the median was 8.8% (3.9–21.9%). Mean CIT for the whole cohort was 4.7%±9.0% (−10.6% to 24.3%). Descriptive statistics of the cohort are presented in Table 1 and for the different subgroups as supplementary material (Tables S1,S2).
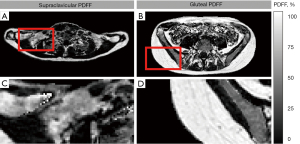
Correlation analysis
Correlation analysis revealed a statistically significant correlation between BMI and BF% (rho =0.44, P=0.005). In addition, the BIA-based BF% correlated significantly with the MRI-measured TF% (rho =0.86, P<0.001). Regarding the associations of the PDFF measurements, a significant relation of both PDFFSUPRACLAVICULAR and PDFFGLUTEAL to SAT and VAT volumes was found. Moreover, correlations of PDFF measurements with body composition, characterized by BF% and to some degree characterized by BMI, were detected. PDFF measurements in association to anthropometric traits, as well as to adipose tissue volumes are displayed in Table 3.
Table 3
Variables | BMI, kg/m2 | BF%, % | SAT, mL/cm | VAT, mL/cm |
---|---|---|---|---|
Supraclavicular PDFF, % | rho =0.39; P=0.014 | rho =0.37; P=0.019 | rho =0.55; P<0.001 | rho =0.59; P<0.001 |
Gluteal PDFF, % | rho =0.30; P=0.067 | rho =0.49; P=0.002 | rho =0.64; P<0.001 | rho =0.55; P<0.001 |
BF%, body fat percentage; BMI, body mass index; PDFF, proton density fat fraction; SAT, subcutaneous adipose tissue; VAT, visceral adipose tissue.
Correlation of PDFF and CIT in the whole cohort
In the whole cohort (n=39), correlation analysis of CIT and PDFFSUPRACLAVICULAR showed no significant association (rho =−0.17, P=0.302).
Correlation of PDFF and CIT in different subgroups
Similar non-significant findings have been observed for most subgroups. Results of the subgroup analysis are presented in Table 4. For the non-overweight cohort (n=25)—according to BMI—an enhanced Spearman’s rho, but a still non-significant correlation of PDFFSUPRACLAVICULAR and CIT (rho =−0.34; P=0.099) was found. Only the subgroup consisting of participants with normal BF% (n=24) revealed a statistically significant correlation of PDFFSUPRACLAVICULAR and CIT with rho =−0.59 (P=0.003). Scatterplots for the relationship between PDFFSUPRACLAVICULAR and CIT of the whole cohort (n=39) and the subgroup with normal BF% (n=24) are presented in Figure 3. An example of supraclavicular PDFF in a person with a low CIT compared to one with a high CIT is shown in Figure 4.
Table 4
Subgroups | N | Correlation of supraclavicular PDFF (%) and CIT (%) |
---|---|---|
Normal weight (BMI <25 kg/m2) | 25 | rho =−0.34; P=0.099 |
Overweight/obese (BMI ≥25 kg/m2) | 14 | rho =0.23; P=0.427 |
Normal BF% (male BF% <25%, female BF% <30%) | 24 | rho =−0.59; P=0.003 |
High BF% (male BF% ≥25%, female BF% ≥30%) | 15 | rho =0.25; P=0.369 |
BF%, body fat percentage; BMI, body mass index; CIT, cold induced thermogenesis; n, number of participants; PDFF, proton density fat fraction.
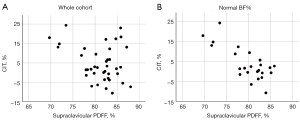
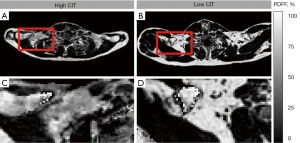
Confounders in the whole cohort
A partial rank correlation for PDFFSUPRACLAVICULAR and CIT adjusted for sex, BMI, BF% or PDFFGLUTEAL was conducted for the whole cohort. When controlled by sex and BF% the correlation improved slightly, but only adjustment for PDFFGLUTEAL returned a significant correlation (rho =−0.34; P=0.037). Results of the partial rank correlation can be found in Table 5. The calculation of ∆PDFF using PDFFGLUTEAL as an intraindividual reference resulted in a significant correlation to CIT (rho =0.36; P=0.026).
Table 5
Controlled by | Correlation of supraclavicular PDFF (%) and CIT (%) |
---|---|
Sex | rho =−0.18; P=0.275 |
BMI | rho =−0.11; P=0.533 |
BF% | rho =−0.20; P=0.240 |
Gluteal PDFF | rho =−0.34; P=0.037 |
BF%, body fat percentage; BMI, body mass index; CIT, cold induced thermogenesis; PDFF, proton density fat fraction.
Discussion
The present study provides evidence for an association between supraclavicular PDFF, as an estimate for brown fat cell presence in the supraclavicular fat depot, and a cold-induced increase in REE. This association was not seen in the whole cohort, comprising healthy participants over a wide BMI range, but only in the subgroup with normal body fat mass shown as BF% and representing normal-weight participants. Using BMI as a crude marker of body fat mass resulted only in a borderline significant correlation between supraclavicular PDFF and CIT in those categorized as normal-weight by BMI (18.5–24.9 kg/m²). The latter is not surprising, as BMI is a poor indicator of body fat mass (57). Therefore, measurement of BF%, e.g., by bioimpedance analysis may be more accurate and useful in this context.
The lack of a significant correlation between absolute supraclavicular PDFF and CIT for the whole study cohort is in line with studies reporting supraclavicular PDFF (39) or rather supraclavicular fat fraction (58) in relation to quantitatively assessed non-shivering thermogenesis. Both studies addressed the potentially confounding role of body composition only briefly, by excluding participants with BMI >30 in advance (39), or simply correcting for body weight in kg (58). Under these conditions, associations may be masked in a similar manner as in the present study and a more precise analysis of body composition may be needed.
In addition, we also observed a positive association between supraclavicular PDFF and BMI, as already reported in a previous study from our group (34) and others (18,35), suggesting that there is less browning capacity and CIT with increasing BMI. Likewise, we found a significantly positive correlation between supraclavicular PDFF and BF% as well as the volumes of VAT and SAT, other parameters of total and regional body fat mass. Deng et al. reported those associations of PDFF, and markers of adiposity, remain unchanged under different thermal conditions and across all weight groups (18).
Furthermore, our results showed a statistically significant difference in PDFF between the supraclavicular adipose tissue depot and the gluteal SAT depot, reflecting differences in morphology and cellular composition of both compartments and is in line with many other studies (22,28,34-36,59,60). Differentiation, without any stimulatory conditions and BAT in a metabolically inactive state, was demonstrated several times before (19,25,28), underlining the value of PDFF measurements for BAT detection.
Using gluteal PDFF as a WAT reference, it turned out as a novel finding that the difference between gluteal PDFF and supraclavicular PDFF was positively associated with CIT, suggesting that the latter may be explained by the presence of brown/beige fat cells in the supraclavicular fat depot and supporting the concept that BAT may contribute to energy expenditure (1,61,62), at least under cold exposure (4,5).
Therefore, in the current study, a model for BAT imaging independent of body composition was developed by merging the first main finding, adiposity influencing PDFF measurements, to the targeted correlation of supraclavicular PDFF and CIT. Partial correlation controlled for gluteal PDFF, firstly demasked the correlation of supraclavicular PDFF and CIT in the whole cohort. By means of this intra-individual referencing, using a region of pure WAT, the individual morphological difference of supraclavicular PDFF to gluteal PDFF was quantified as delta PDFF, supposedly reflecting the respective likelihood of BAT presence for every individual. The delta PDFF correlated positively with CIT, which indicates delta PDFF as a better approach to indirectly assess BAT. Franssens et al. showed accordingly, that BAT presence, as suggested by a large difference in supraclavicular fat fraction compared to fat fraction of WAT inversely correlates with the number of cardiovascular risk factors (35).
The association of supraclavicular PDFF and CIT in cohorts with a wide BMI range is hard to detect. While in subgroups with low BF% the relation with CIT was clear and significant, adiposity makes BAT detection increasingly difficult as brown/beige fat cells may be replaced by white adipocytes.
Women naturally display higher BF% than men and when grouping by BF% is performed a critical investigation of sex difference is needed. Although grouping by BF% was performed by sex adapted cut offs, the great majority in the high BF% group were female (86.7%), compared to 45.8% females in the normal BF% group. Consequentially, the stronger correlation of supraclavicular PDFF to CIT in the normal BF% group could be interpreted as BAT predominance in men—unlike many other studies suggest. Even though data regarding sex differences in BAT activity is ambiguous, with studies reporting an existing difference (63,64) as well as no existing difference (65,66), the large retrospective PET-CT study of Brendle et al. (64) revealed that BAT positive individuals are predominantly young, lean, and female. In contrast our related investigation detected no significant difference in CIT between men and woman but found more pronounced hormonal changes in women—also indicating a stronger response to cold exposure. Granted, that women display more active BAT compared to men, their higher BF% could obscure results in heterogenous and predominantly female cohorts, like the present.
A strength of the current study is the analysis of subgroups not only by BMI but also by BF%, hence the poor diagnostic assessment of obesity via BMI is a possible origin for inaccuracy. At least widely used BMI cutoffs show high specificity, but very low sensitivity missing nearly half the patients who should be considered obese due to high BF% (57,67). Concerning data quality, one of the major strengths was the applied breathing trigger for supraclavicular PDFF mapping, distinguishing this study from others in the field (39) and rendering the data more precise with less partial volume effects and less image blurring. Furthermore, verifying the BIA-scale measurements with the aid of quantitative MRI ensured robust grouping into different cohorts. Another strength of our study is the combination of imaging data with metabolic quantification under cold exposure, by CIT measurements—a rather complex and time-consuming technique.
The present study has several limitations. A first limitation of this study is the lacking comparison of histological biopsies in this analysis. It is still the gold standard for adipose tissue characterization and without histological tissue analysis the presence of BAT cannot be indubitably proven. However, histology even with immunohistochemical staining using uncoupling protein 1 (UCP1) antibodies, would only provide information about BAT presence, not give a quantitative result regarding the metabolic activity of the tissue. Especially since UCP1-independent thermogenesis is known to play a significant role in human thermogenesis (68,69). No cold activation was applied for the PDFF measurements and given that the PDFF mapping method is not dependent on BAT function, histology for validation could have been beneficial. Furthermore, with the isotropic voxel size being 1.5 mm in each dimension, partial volume effects from very small vessels and lymph nodes must be considered. Due to the complicated anatomy of the supraclavicular fossa, partial volume effects carry probably even more weight when measuring supraclavicular PDFF compared to gluteal PDFF. However, the applied methods minimized those effects with the help of breathing triggered scans and erosion algorithms in the segmentations. Furthermore, average PDFF values over the VOI will always include a mixture of BAT and WAT, thus only delivering indications of crude BAT presence in this region.
Due to the discussed confounding factors (adiposity, individual adipose tissue morphology) and high variability of both PDFF and CIT measurements, the predictive value of supraclavicular PDFF for CIT is low. Even though the calculation of delta PDFF seems to address the confounding effect of adiposity at least partially, delta PDFF as predictive parameter must be interpreted with caution and larger prospective studies for validation are needed. Consequentially the rather small sample size limits the interpretation of predictive power and is smaller compared to related studies in the field.
In the study design simultaneous CIT and MRI measurements were not intended, as no cold exposure is needed to assess supraclavicular morphology with MRI. However, in some individuals both measurements were conducted with a long-time separation, which could lead to potentially not captured individual adaptation in supraclavicular composition. This is definitely a limitation based in the applied study design and subsequent studies should aim for a consistent and short time period between both measurements.
Limitations finally apply to the employed water-fat model. The employed multi-peak fat spectrum model from (47) is constant and could not account for differences between subjects and adipose tissue depots. Recent magnetic resonance spectroscopy (MRS) results have showed that the amount of unsaturated fatty acids is lower in BAT compared to WAT (70) and such variations in the fat spectrum model might need to be considered in future PDFF studies in BAT. Another limitation is the inability to report T2* values over the supraclavicular fossa. A water-fat model accounting for a single common T2* decay for the water and fat components was presently adopted. Since only 6-echo data were evaluated, which was found to be insufficient for reliant T2* measurements in an earlier publication (71), T2* values were only used for segmentation purposes to assure exclusion of bone. Moreover, reporting T2* would depict a different aspect of adipose tissue characterization (increased field inhomogeneity in BAT due to iron containing mitochondria, perfusion, and oxygenation), then the here highlighted compositional differentiation of BAT and WAT.
Conclusions
MRI-based PDFF measurements of the supraclavicular fossa could correlate with CIT, if body adiposity is considered, as adipose tissue PDFF is highly dependent on adiposity. This dependence was reflected in the strong association of gluteal and supraclavicular PDFF to anthropometric markers and adipose tissue volumes. Still, this study demonstrated an association between delta PDFF and CIT in a heterogenous cohort. It is noteworthy that a high interindividual variation seems to exist, both for delta PDFF and CIT. To further validate the functional relevance of delta PDFF for energy metabolism and obesity risk large prospective observational studies are needed.
Acknowledgments
The authors are grateful to all volunteers participating in the study as well as to the students assisting in the study execution.
Funding: The present work was supported by the European Research Council (grant agreement No. 677661, ProFatMRI), the German Research Foundation (No. DFG-SFB824/A9), Philips Healthcare, the Else Kroener-Fresenius-Foundation, Bad Homburg, Germany, and the Helmholtz cross-program topic “Metabolic Dysfunction”. This work reflects only the authors´ view and the funders are not responsible for any use that may be made of the information it contains.
Footnote
Reporting Checklist: The authors have completed the STROBE reporting checklist. Available at https://qims.amegroups.com/article/view/10.21037/qims-21-986/rc
Conflicts of Interest: All authors have completed the ICMJE uniform disclosure form (available at https://qims.amegroups.com/article/view/10.21037/qims-21-986/coif). DCK receives grant support from Philips Healthcare. The other authors have no conflicts of interest to declare.
Ethical Statement:
Open Access Statement: This is an Open Access article distributed in accordance with the Creative Commons Attribution-NonCommercial-NoDerivs 4.0 International License (CC BY-NC-ND 4.0), which permits the non-commercial replication and distribution of the article with the strict proviso that no changes or edits are made and the original work is properly cited (including links to both the formal publication through the relevant DOI and the license). See: https://creativecommons.org/licenses/by-nc-nd/4.0/.
References
- Enerbäck S. Human brown adipose tissue. Cell Metab 2010;11:248-52. [Crossref] [PubMed]
- Jespersen NZ, Larsen TJ, Peijs L, Daugaard S, Homøe P, Loft A, de Jong J, Mathur N, Cannon B, Nedergaard J, Pedersen BK, Møller K, Scheele C. A classical brown adipose tissue mRNA signature partly overlaps with brite in the supraclavicular region of adult humans. Cell Metab 2013;17:798-805. [Crossref] [PubMed]
- Lidell ME, Betz MJ, Dahlqvist Leinhard O, Heglind M, Elander L, Slawik M, Mussack T, Nilsson D, Romu T, Nuutila P, Virtanen KA, Beuschlein F, Persson A, Borga M, Enerbäck S. Evidence for two types of brown adipose tissue in humans. Nat Med 2013;19:631-4. [Crossref] [PubMed]
- Muzik O, Mangner TJ, Granneman JG. Assessment of oxidative metabolism in brown fat using PET imaging. Front Endocrinol (Lausanne) 2012;3:15. [Crossref] [PubMed]
- Yoneshiro T, Aita S, Matsushita M, Kameya T, Nakada K, Kawai Y, Saito M. Brown adipose tissue, whole-body energy expenditure, and thermogenesis in healthy adult men. Obesity (Silver Spring) 2011;19:13-6. [Crossref] [PubMed]
- Bartelt A, Bruns OT, Reimer R, Hohenberg H, Ittrich H, Peldschus K, Kaul MG, Tromsdorf UI, Weller H, Waurisch C, Eychmüller A, Gordts PL, Rinninger F, Bruegelmann K, Freund B, Nielsen P, Merkel M, Heeren J. Brown adipose tissue activity controls triglyceride clearance. Nat Med 2011;17:200-5. [Crossref] [PubMed]
- Fedorenko A, Lishko PV, Kirichok Y. Mechanism of fatty-acid-dependent UCP1 uncoupling in brown fat mitochondria. Cell 2012;151:400-13. [Crossref] [PubMed]
- Kopelman PG. Obesity as a medical problem. Nature 2000;404:635-43. [Crossref] [PubMed]
- Yoneshiro T, Saito M. Activation and recruitment of brown adipose tissue as anti-obesity regimens in humans. Ann Med 2015;47:133-41. [Crossref] [PubMed]
- Blondin DP, Labbé SM, Tingelstad HC, Noll C, Kunach M, Phoenix S, Guérin B, Turcotte EE, Carpentier AC, Richard D, Haman F. Increased brown adipose tissue oxidative capacity in cold-acclimated humans. J Clin Endocrinol Metab 2014;99:E438-46. [Crossref] [PubMed]
- Silva JE, Bianco SD. Thyroid-adrenergic interactions: physiological and clinical implications. Thyroid 2008;18:157-65. [Crossref] [PubMed]
- Brychta RJ, Chen KY. Cold-induced thermogenesis in humans. Eur J Clin Nutr 2017;71:345-52. [Crossref] [PubMed]
- Chen KY, Cypess AM, Laughlin MR, Haft CR, Hu HH, Bredella MA, Enerbäck S, Kinahan PE. Lichtenbelt Wv, Lin FI, Sunderland JJ, Virtanen KA, Wahl RL. Brown Adipose Reporting Criteria in Imaging STudies (BARCIST 1.0): Recommendations for Standardized FDG-PET/CT Experiments in Humans. Cell Metab 2016;24:210-22. [Crossref] [PubMed]
- Karampinos DC, Weidlich D, Wu M, Hu HH, Franz D. Techniques and Applications of Magnetic Resonance Imaging for Studying Brown Adipose Tissue Morphometry and Function. Handb Exp Pharmacol 2019;251:299-324. [Crossref] [PubMed]
- Hu HH. Magnetic Resonance of Brown Adipose Tissue: A Review of Current Techniques. Crit Rev Biomed Eng 2015;43:161-81. [Crossref] [PubMed]
- Abreu-Vieira G, Sardjoe Mishre ASD, Burakiewicz J, Janssen LGM, Nahon KJ, van der Eijk JA, Riem TT, Boon MR, Dzyubachyk O, Webb AG, Rensen PCN, Kan HE. Human Brown Adipose Tissue Estimated With Magnetic Resonance Imaging Undergoes Changes in Composition After Cold Exposure: An in vivo MRI Study in Healthy Volunteers. Front Endocrinol (Lausanne) 2020;10:898. [Crossref] [PubMed]
- Coolbaugh CL, Damon BM, Bush EC, Welch EB, Towse TF. Cold exposure induces dynamic, heterogeneous alterations in human brown adipose tissue lipid content. Sci Rep 2019;9:13600. [Crossref] [PubMed]
- Deng J, Neff LM, Rubert NC, Zhang B, Shore RM, Samet JD, Nelson PC, Landsberg L. MRI characterization of brown adipose tissue under thermal challenges in normal weight, overweight, and obese young men. J Magn Reson Imaging 2018;47:936-47. [Crossref] [PubMed]
- Gifford A, Towse TF, Walker RC, Avison MJ, Welch EB. Characterizing active and inactive brown adipose tissue in adult humans using PET-CT and MR imaging. Am J Physiol Endocrinol Metab 2016;311:E95-E104. [Crossref] [PubMed]
- Koskensalo K, Raiko J, Saari T, Saunavaara V, Eskola O, Nuutila P, Saunavaara J, Parkkola R, Virtanen KA. Human Brown Adipose Tissue Temperature and Fat Fraction Are Related to Its Metabolic Activity. J Clin Endocrinol Metab 2017;102:1200-7. [Crossref] [PubMed]
- Stahl V, Maier F, Freitag MT, Floca RO, Berger MC, Umathum R, Berriel Diaz M, Herzig S, Weber MA, Dimitrakopoulou-Strauss A, Rink K, Bachert P, Ladd ME, Nagel AM. In vivo assessment of cold stimulation effects on the fat fraction of brown adipose tissue using DIXON MRI. J Magn Reson Imaging 2017;45:369-80. [Crossref] [PubMed]
- Lundström E, Strand R, Johansson L, Bergsten P, Ahlström H, Kullberg J. Magnetic resonance imaging cooling-reheating protocol indicates decreased fat fraction via lipid consumption in suspected brown adipose tissue. PLoS One 2015;10:e0126705. [Crossref] [PubMed]
- Oreskovich SM, Ong FJ, Ahmed BA, Konyer NB, Blondin DP, Gunn E, Singh NP, Noseworthy MD, Haman F, Carpentier AC, Punthakee Z, Steinberg GR, Morrison KM. MRI Reveals Human Brown Adipose Tissue Is Rapidly Activated in Response to Cold. J Endocr Soc 2019;3:2374-84. [Crossref] [PubMed]
- Holstila M, Virtanen KA, Grönroos TJ, Laine J, Lepomäki V, Saunavaara J, Lisinen I, Komu M, Hannukainen JC, Nuutila P, Parkkola R, Borra RJ. Measurement of brown adipose tissue mass using a novel dual-echo magnetic resonance imaging approach: a validation study. Metabolism 2013;62:1189-98. [Crossref] [PubMed]
- Holstila M, Pesola M, Saari T, Koskensalo K, Raiko J, Borra RJ, Nuutila P, Parkkola R, Virtanen KA. MR signal-fat-fraction analysis and T2* weighted imaging measure BAT reliably on humans without cold exposure. Metabolism 2017;70:23-30. [Crossref] [PubMed]
- Hu HH, Tovar JP, Pavlova Z, Smith ML, Gilsanz V. Unequivocal identification of brown adipose tissue in a human infant. J Magn Reson Imaging 2012;35:938-42. [Crossref] [PubMed]
- Reddy NL, Jones TA, Wayte SC, Adesanya O, Sankar S, Yeo YC, Tripathi G, McTernan PG, Randeva HS, Kumar S, Hutchinson CE, Barber TM. Identification of brown adipose tissue using MR imaging in a human adult with histological and immunohistochemical confirmation. J Clin Endocrinol Metab 2014;99:E117-21. [Crossref] [PubMed]
- Franz D, Karampinos DC, Rummeny EJ, Souvatzoglou M, Beer AJ, Nekolla SG, Schwaiger M, Eiber M. Discrimination Between Brown and White Adipose Tissue Using a 2-Point Dixon Water-Fat Separation Method in Simultaneous PET/MRI. J Nucl Med 2015;56:1742-7. [Crossref] [PubMed]
- McCallister A, Zhang L, Burant A, Katz L, Branca RT. A pilot study on the correlation between fat fraction values and glucose uptake values in supraclavicular fat by simultaneous PET/MRI. Magn Reson Med 2017;78:1922-32. [Crossref] [PubMed]
- Hu HH, Kan HE. Quantitative proton MR techniques for measuring fat. NMR Biomed 2013;26:1609-29. [Crossref] [PubMed]
- Reeder SB, Cruite I, Hamilton G, Sirlin CB. Quantitative Assessment of Liver Fat with Magnetic Resonance Imaging and Spectroscopy. J Magn Reson Imaging 2011;34:729-49. [Crossref] [PubMed]
- Karampinos DC, Ruschke S, Dieckmeyer M, Diefenbach M, Franz D, Gersing AS, Krug R, Baum T. Quantitative MRI and spectroscopy of bone marrow. J Magn Reson Imaging 2018;47:332-53. [Crossref] [PubMed]
- Reeder SB, Hu HH, Sirlin CB. Proton density fat-fraction: a standardized MR-based biomarker of tissue fat concentration. J Magn Reson Imaging 2012;36:1011-4. [Crossref] [PubMed]
- Franz D, Weidlich D, Freitag F, Holzapfel C, Drabsch T, Baum T, Eggers H, Witte A, Rummeny EJ, Hauner H, Karampinos DC. Association of proton density fat fraction in adipose tissue with imaging-based and anthropometric obesity markers in adults. Int J Obes (Lond) 2018;42:175-82. [Crossref] [PubMed]
- Franssens BT, Hoogduin H, Leiner T, van der Graaf Y, Visseren FLJ. Relation between brown adipose tissue and measures of obesity and metabolic dysfunction in patients with cardiovascular disease. J Magn Reson Imaging 2017;46:497-504. [Crossref] [PubMed]
- Franssens BT, Eikendal AL, Leiner T, van der Graaf Y, Visseren FL, Hoogduin JM. Reliability and agreement of adipose tissue fat fraction measurements with water-fat MRI in patients with manifest cardiovascular disease. NMR Biomed 2016;29:48-56. [Crossref] [PubMed]
- Deng J, Schoeneman SE, Zhang H, Kwon S, Rigsby CK, Shore RM, Josefson JL. MRI characterization of brown adipose tissue in obese and normal-weight children. Pediatr Radiol 2015;45:1682-9. [Crossref] [PubMed]
- Hu HH, Perkins TG, Chia JM, Gilsanz V. Characterization of human brown adipose tissue by chemical-shift water-fat MRI. AJR Am J Roentgenol 2013;200:177-83. [Crossref] [PubMed]
- Gashi G, Madoerin P, Maushart CI, Michel R, Senn JR, Bieri O, Betz MJ. MRI characteristics of supraclavicular brown adipose tissue in relation to cold-induced thermogenesis in healthy human adults. J Magn Reson Imaging 2019;50:1160-8. [Crossref] [PubMed]
- Zingaretti MC, Crosta F, Vitali A, Guerrieri M, Frontini A, Cannon B, Nedergaard J, Cinti S. The presence of UCP1 demonstrates that metabolically active adipose tissue in the neck of adult humans truly represents brown adipose tissue. FASEB J 2009;23:3113-20. [Crossref] [PubMed]
- Frontini A, Cinti S. Distribution and development of brown adipocytes in the murine and human adipose organ. Cell Metab 2010;11:253-6. [Crossref] [PubMed]
- Mengel LA, Seidl H, Brandl B, Skurk T, Holzapfel C, Stecher L, Claussnitzer M, Hauner H. Gender Differences in the Response to Short-term Cold Exposure in Young Adults. J Clin Endocrinol Metab 2020;105:dgaa110.
- WEIR JB. New methods for calculating metabolic rate with special reference to protein metabolism. J Physiol 1949;109:1-9. [Crossref] [PubMed]
- Wu M, Junker D, Branca RT, Karampinos DC. Magnetic Resonance Imaging Techniques for Brown Adipose Tissue Detection. Front Endocrinol (Lausanne) 2020;11:421. [Crossref] [PubMed]
- Hu HH, Branca RT, Hernando D, Karampinos DC, Machann J, McKenzie CA, Wu HH, Yokoo T, Velan SS. Magnetic resonance imaging of obesity and metabolic disorders: Summary from the 2019 ISMRM Workshop. Magn Reson Med 2020;83:1565-76. [Crossref] [PubMed]
- Eggers H, Brendel B, Duijndam A, Herigault G. Dual-echo Dixon imaging with flexible choice of echo times. Magn Reson Med 2011;65:96-107. [Crossref] [PubMed]
- Ren J, Dimitrov I, Sherry AD, Malloy CR. Composition of adipose tissue and marrow fat in humans by 1H NMR at 7 Tesla. J Lipid Res 2008;49:2055-62. [Crossref] [PubMed]
- Yushkevich PA, Piven J, Hazlett HC, Smith RG, Ho S, Gee JC, Gerig G. User-guided 3D active contour segmentation of anatomical structures: significantly improved efficiency and reliability. Neuroimage 2006;31:1116-28. [Crossref] [PubMed]
- Markman B, Barton FE Jr. Anatomy of the subcutaneous tissue of the trunk and lower extremity. Plast Reconstr Surg 1987;80:248-54. [Crossref] [PubMed]
- Cordes C, Dieckmeyer M, Ott B, Shen J, Ruschke S, Settles M, Eichhorn C, Bauer JS, Kooijman H, Rummeny EJ, Skurk T, Baum T, Hauner H, Karampinos DC. MR-detected changes in liver fat, abdominal fat, and vertebral bone marrow fat after a four-week calorie restriction in obese women. J Magn Reson Imaging 2015;42:1272-80. [Crossref] [PubMed]
- Shen J, Baum T, Cordes C, Ott B, Skurk T, Kooijman H, Rummeny EJ, Hauner H, Menze BH, Karampinos DC. Automatic segmentation of abdominal organs and adipose tissue compartments in water-fat MRI: Application to weight-loss in obesity. Eur J Radiol 2016;85:1613-21. [Crossref] [PubMed]
- Karcher HS, Holzwarth R, Mueller HP, Ludolph AC, Huber R, Kassubek J, Pinkhardt EH. Body fat distribution as a risk factor for cerebrovascular disease: an MRI-based body fat quantification study. Cerebrovasc Dis 2013;35:341-8. [Crossref] [PubMed]
- Obesity: preventing and managing the global epidemic. Report of a WHO consultation. World Health Organ Tech Rep Ser 2000;894:i-xii, 1-253. [PubMed]
- Snitker S. Use of body fatness cutoff points. Mayo Clin Proc 2010;85:1057-author reply 1057-8. [Crossref] [PubMed]
- Frankenfield DC, Rowe WA, Cooney RN, Smith JS, Becker D. Limits of body mass index to detect obesity and predict body composition. Nutrition 2001;17:26-30. [Crossref] [PubMed]
- De Lorenzo A, Soldati L, Sarlo F, Calvani M, Di Lorenzo N, Di Renzo L. New obesity classification criteria as a tool for bariatric surgery indication. World J Gastroenterol 2016;22:681-703. [Crossref] [PubMed]
- Okorodudu DO, Jumean MF, Montori VM, Romero-Corral A, Somers VK, Erwin PJ, Lopez-Jimenez F. Diagnostic performance of body mass index to identify obesity as defined by body adiposity: a systematic review and meta-analysis. Int J Obes (Lond) 2010;34:791-9. [Crossref] [PubMed]
- Sun L, Verma S, Michael N, Chan SP, Yan J, Sadananthan SA, Camps SG, Goh HJ, Govindharajulu P, Totman J, Townsend D, Goh JP, Sun L, Boehm BO, Lim SC, Sze SK, Henry CJ, Hu HH, Velan SS, Leow MK. Brown Adipose Tissue: Multimodality Evaluation by PET, MRI, Infrared Thermography, and Whole-Body Calorimetry (TACTICAL-II). Obesity (Silver Spring) 2019;27:1434-42. [Crossref] [PubMed]
- Hu HH, Yin L, Aggabao PC, Perkins TG, Chia JM, Gilsanz V. Comparison of brown and white adipose tissues in infants and children with chemical-shift-encoded water-fat MRI. J Magn Reson Imaging 2013;38:885-96. [Crossref] [PubMed]
- Rasmussen JM, Entringer S, Nguyen A, van Erp TG, Burns J, Guijarro A, Oveisi F, Swanson JM, Piomelli D, Wadhwa PD, Buss C, Potkin SG. Brown adipose tissue quantification in human neonates using water-fat separated MRI. PLoS One 2013;8:e77907. [Crossref] [PubMed]
- Cannon B, Nedergaard J. Brown adipose tissue: function and physiological significance. Physiol Rev 2004;84:277-359. [Crossref] [PubMed]
- Rothwell NJ, Stock MJ. Luxuskonsumption, diet-induced thermogenesis and brown fat: the case in favour. Clin Sci (Lond) 1983;64:19-23. [Crossref] [PubMed]
- Orava J, Nuutila P, Noponen T, Parkkola R, Viljanen T, Enerbäck S, Rissanen A, Pietiläinen KH, Virtanen KA. Blunted metabolic responses to cold and insulin stimulation in brown adipose tissue of obese humans. Obesity (Silver Spring) 2013;21:2279-87. [Crossref] [PubMed]
- Brendle C, Werner MK, Schmadl M, la Fougère C, Nikolaou K, Stefan N, Pfannenberg C. Correlation of Brown Adipose Tissue with Other Body Fat Compartments and Patient Characteristics: A Retrospective Analysis in a Large Patient Cohort Using PET/CT. Acad Radiol 2018;25:102-10. [Crossref] [PubMed]
- van der Lans AA, Hoeks J, Brans B, Vijgen GH, Visser MG, Vosselman MJ, Hansen J, Jörgensen JA, Wu J, Mottaghy FM, Schrauwen P, van Marken Lichtenbelt WD. Cold acclimation recruits human brown fat and increases nonshivering thermogenesis. J Clin Invest 2013;123:3395-403. [Crossref] [PubMed]
- Celi FS, Brychta RJ, Linderman JD, Butler PW, Alberobello AT, Smith S, Courville AB, Lai EW, Costello R, Skarulis MC, Csako G, Remaley A, Pacak K, Chen KY. Minimal changes in environmental temperature result in a significant increase in energy expenditure and changes in the hormonal homeostasis in healthy adults. Eur J Endocrinol 2010;163:863-72. [Crossref] [PubMed]
- Romero-Corral A, Somers VK, Sierra-Johnson J, Thomas RJ, Collazo-Clavell ML, Korinek J, Allison TG, Batsis JA, Sert-Kuniyoshi FH, Lopez-Jimenez F. Accuracy of body mass index in diagnosing obesity in the adult general population. Int J Obes (Lond) 2008;32:959-66. [Crossref] [PubMed]
- Roesler A, Kazak L. UCP1-independent thermogenesis. Biochem J 2020;477:709-25. [Crossref] [PubMed]
- Ikeda K, Yamada T. UCP1 Dependent and Independent Thermogenesis in Brown and Beige Adipocytes. Front Endocrinol (Lausanne) 2020;11:498. [Crossref] [PubMed]
- Ouwerkerk R, Hamimi A, Matta J, Abd-Elmoniem KZ, Eary JF, Abdul Sater Z, Chen KY, Cypess AM, Gharib AM, Proton MR. Spectroscopy Measurements of White and Brown Adipose Tissue in Healthy Humans: Relaxation Parameters and Unsaturated Fatty Acids. Radiology 2021;299:396-406. [Crossref] [PubMed]
- Franz D, Diefenbach MN, Treibel F, Weidlich D, Syväri J, Ruschke S, Wu M, Holzapfel C, Drabsch T, Baum T, Eggers H, Rummeny EJ, Hauner H, Karampinos DC. Differentiating supraclavicular from gluteal adipose tissue based on simultaneous PDFF and T2 * mapping using a 20-echo gradient-echo acquisition. J Magn Reson Imaging 2019;50:424-34. [Crossref] [PubMed]