Development and validation of novel radiomics-based nomograms for the prediction of EGFR mutations and Ki-67 proliferation index in non-small cell lung cancer
Introduction
Non-small cell lung cancer (NSCLC) is one of the leading causes of cancer-related death worldwide, with the most common histological types of adenocarcinoma and squamous cell carcinoma with a high reoccurrence rate and a poor prognosis for patients (1,2). Epidermal growth factor receptor (EGFR) tyrosine kinase inhibitors (TKIs) can improve the survival of patients who have lung adenocarcinoma with sensitive mutations in the EGFR gene and improve their quality of life (3,4). However, patients cannot benefit from negative or non-sensitive mutations in the EGFR gene (5). Therefore, it is extremely important to detect the status of the EGFR gene before giving targeted drug therapy to patients with lung adenocarcinoma.
Currently Ki-67 is the most widely used marker for cell proliferation assessment (6), and its expression is related to the development, metastasis and prognosis of lung cancer (7). Studies show that the Ki-67 nuclear antigen is present only in proliferating cells, thus making it a reliable avenue for rapidly evaluating the growth fraction of both normal and abnormal cells (8). Hence, Ki-67 proliferation index (PI) has been used in a variety of tumors to reflect the proliferation of tumor cells, evaluate prognosis and design molecular targeted drugs (9). It has also been shown to be an important prognostic factor for lung cancer (10-12). According to Wei et al. (7), Ki-67 has different expression levels in different tissue types of lung cancer. Among them, small cell lung cancer has the highest expression, and lung squamous cell carcinoma has higher expression than adenocarcinoma. Therefore, predicting Ki-67 PI with high accuracy may highlight tumor invasive growth patterns, which might then allow for a precise evaluation of tumor biological behavior and aid in clinical treatment decision making for the individualized management of patients.
However, in clinical practice, EGFR and Ki-67 indicators can only be obtained through immunohistochemical staining. The acquisition of tissue samples is invasive and there are certain subjectivity and sampling errors. At the same time, there are some patients without an inclination for surgery or needle biopsy for whom level of expression cannot be evaluated.
Radiomics analysis, which was proposed by Lambin et al. in 2012 (13), involves the extraction of a large number of quantitative features from digital images to determine relationships between such features and the underlying pathophysiology (14). Radiomics analysis of large imaging datasets has been successfully employed in the field of oncology for noninvasively profiling tumor heterogeneity (15,16), and there is a growing interest in devising maps that display the associations between tumor heterogeneity and imaging features (17). This involves the extraction of quantitative features from digital medical images, which enables mineable high-dimensional data to be applied within clinical decision support to offer improved diagnostic, prognostic, and predictive accuracy (18-22). Radiomics is gaining importance in personalized cancer therapy. Since computed tomography (CT) is routinely used in lung cancer diagnosis, this study intended to establish the CT imaging radiomics label of lung cancer patients, and to explore the feasibility of using it to predict the sensitive mutation of EGFR gene and Ki-67 expression level in lung cancer.
This study aimed to develop and evaluate novel radiomic scores (Rad-Scores) and nomograms to predict EGFR mutations and Ki-67 PI expression based on pre-treatment CT images of NSCLC patients. These useful radiomics-based nomograms could provide a non-invasive strategy to assess EGFR mutation status and cell proliferation, which may also help to guide proper clinical treatment.
We present the following article in accordance with the TRIPOD reporting checklist (available at https://qims.amegroups.com/article/view/10.21037/qims-21-980/rc).
Methods
Ethics and study design
The study was conducted in accordance with the Declaration of Helsinki (as revised in 2013). This retrospective study was approved by the Institutional Review Board of Shandong Cancer Hospital and individual consent for this retrospective analysis was waived. Figure 1 shows the flowchart of our study. The current study contained two tasks: task [1] was designed to differentiate EGFR mutant and wild type, and task [2] was designed to differentiate high Ki-67 PI expression from low expression.
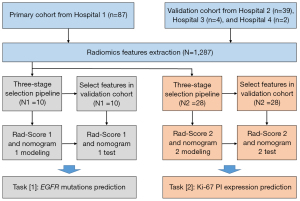
A total of 132 patients were analyzed retrospectively, and patients were divided into the primary cohort and an independent validation cohort according to the data source. The primary cohort included 87 patients from the first hospital between January 2018 and March 2019, and the validation cohort included 39 patients from the second hospital, 4 patients from the third hospital, and 2 patients from the fourth hospital between November 2018 and May 2020. Based on the dataset, the volume of interest (VOI) was manually delineated on each pre-processed CT image using ITK-SNAP. As a result, a total of 1,287 quantitative features were extracted. A three-stage feature selection method was then implemented for data mining in the primary cohort for the two tasks. Finally, the radiomic scores and nomograms of the two tasks were established in the primary cohort, and tested in the validation cohort.
Patients
Eligible patients in this study were those who had complete clinical information, histologically verified NSCLC, and confirmed sufficient tissue for EGFR and Ki-67 immunohistochemical staining. Clinical and histological characteristics of the patients (including gender, age, smoking status, tumor location, CT pattern, histological type, stage, EGFR gene status, and Ki-67 expression) in primary and validation cohorts were retrospectively obtained from medical records. Pathologic tumor stage was defined using the American Joint Committee on Cancer staging manual, the eighth edition (23). The stage was assigned retrospectively for patients whose tumors were staged before publication of the eighth edition.
In task [1], 75 patients had the EGFR mutations, and 57 patients had EGFR wild type. In task [2], 52 patients had high Ki-67 PI expression, and 80 patients had low Ki-67 PI expression. The basic clinical data of patients are provided in Table 1.
Table 1
Characteristics | All patients (N=132) | Primary cohort (N=87) | Validation cohort (N=45) | P* |
---|---|---|---|---|
Gender, n (%) | 0.304 | |||
Male | 68 (51.52) | 42 (48.28) | 26 (57.78) | |
Female | 64 (48.48) | 45 (51.72) | 19 (42.22) | |
Age, mean [range] (years) | 58.8 [27–80] | 58.0 [27–80] | 60.4 [38–78] | 0.204 |
Smoking status, n (%) | 0.149 | |||
Never | 90 (68.18) | 63 (72.41) | 27 (60.00) | |
Smoker | 42 (31.82) | 24 (27.59) | 18 (40.00) | |
Tumor location, n (%) | 0.643 | |||
Right upper lobe | 48 (36.36) | 31 (35.63) | 17 (37.78) | |
Right middle lobe | 7 (5.30) | 5 (5.75) | 2 (4.44) | |
Right lower lobe | 27 (20.45) | 15 (17.24) | 12 (26.67) | |
Left upper lobe | 36 (27.27) | 27 (31.03) | 9 (20.00) | |
Left lower lobe | 14 (10.61) | 9 (10.34) | 5 (11.11) | |
CT pattern, n (%) | 0.074 | |||
Pure solid nodule | 74 (56.06) | 44 (50.57) | 30 (66.67) | |
Part-solid nodule | 48 (36.36) | 35 (40.23) | 13 (28.89) | |
Ground glass nodules | 10 (7.58) | 8 (9.20) | 2 (4.44) | |
Histological type, n (%) | 0.779 | |||
LAC | 122 (92.42) | 80 (91.95) | 42 (93.33) | |
LSC | 10 (7.58) | 7 (8.05) | 3 (6.67) | |
Stage, n (%) | 0.001 | |||
Tis | 3 (2.27) | 0 (0.00) | 3 (6.67) | |
I | 113 (85.61) | 84 (96.55) | 29 (64.44) | |
II and III | 16 (12.12) | 3 (3.45) | 13 (28.89) | |
EGFR status, n (%) | 0.835 | |||
Mutant-type | 75 (56.82) | 50 (57.47) | 25 (55.56) | |
Wild-type | 57 (43.18) | 37 (42.53) | 20 (44.44) | |
Ki-67 expression, n (%) | 0.008 | |||
High | 52 (39.39) | 27 (31.03) | 25 (55.56) | |
Low | 80 (60.61) | 60 (68.97) | 20 (44.44) |
*, the differences were assessed by Mann-Whitney U test or Chi-squared test. CT, computed tomography; LAC, lung adenocarcinoma; LSC, lung squamous carcinoma; EGFR, epidermal growth factor receptor.
EGFR mutation status and Ki-67 PI assessment
In regards to molecular profiles, tumor specimens were obtained using surgical resection. EGFR mutations were identified on four tyrosine kinase domains (exons 18–21), which are frequently mutated in lung cancer. If any exon mutation was detected, the tumor was identified as an EGFR mutant; otherwise, the tumor was identified as EGFR wild type. Therefore, in this study, we focused on predicting these binary outcomes (EGFR mutant and wild-type) for the patients.
The Ki-67 PI is recorded as the percentage of malignant cells stained positive. According to the St. Gallen International Expert Consensus (24), the Ki-67 PI is judged as follows: a Ki-67 PI ≥14% is recorded as high expression, and Ki-67 PI <14% is recorded as low expression.
Image acquisition
All cases were evaluated with a spiral CT scan of the chest (Somatom Force, Somatom Flash dual-source CT, Siemens, Germany or Brilliance 256 iCT, Philips Healthcare, Cleveland, USA). Scanning parameters: tube voltage 120 kV, automatic tube current, pitch 0.984–1.200, matrix 512×512, FOV 350 mm × 350 mm. After the original data collection, all patients underwent interval reconstruction of 1.0–3.0 mm. High-resolution lung algorithm was adopted, with lung window width of 1,200 HU and window level of −500 HU. CT images were retrieved from the picture archiving and communication system workstation. Then, the image pre-processing (removing sensitive information, normalization, etc.) was implemented before radiomics analysis.
Tumor segmentation and feature extraction
All the VOIs were manually delineated slice by slice using ITK-SNAP software (version 3.8.0; www.itksnap.org). This was performed independently by two experienced thoracic radiologists (with 8 and 21 years of experience in chest CT imaging) blinded to the patients’ clinical diagnosis and gene mutation status. Feature reproductive analysis was performed afterward. The details of image preprocessing were provided in Table S1. A total of 1,287 quantitative radiomic features were then extracted from each VOI using Pyradiomics (version 3.0.1; https://github.com/Radiomics/pyradiomics) (25), which were shown in Table S2.
Feature selection
Feature selection was performed in the primary cohort. The interrelationship between all the features is provided in Figure S1. Here, a three-stage feature selection pipeline was designed for mining the valuable predictive factors (26). In the first stage, a Mann-Whitney U test was implemented to remove the features that showed no significant difference in EGFR mutations and no Ki-67 PI expressions. The features with P value less than 0.05 were evaluated in the next stage. Next, a Spearman test was performed to examine the correlation between radiomic features. According to the rankings in the first stage, the redundant features were removed (correlation coefficient |r| >0.80). Finally, a random forest (RF) based Boruta algorithm (27) was performed to select the final correlation features. Figure 2 shows the details of the RF based Boruta selection. The red features were removed as unimportant factors, while the green and yellow features were selected as important factors. All the details of radiomic feature selection pipeline in the two tasks are provided in Table 2.
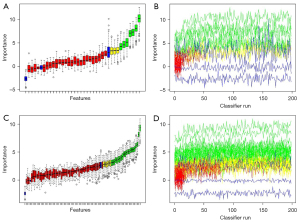
Table 2
Task | Extracted features | Mann-Whitney U test | Spearman test | RF based Boruta |
---|---|---|---|---|
EGFR | 1,287 | 168 | 35 | 10 |
Ki-67 | 1,287 | 852 | 83 | 28 |
RF, random forest; EGFR, epidermal growth factor receptor.
Radiomics models construction and validation
After feature selection, the Rad-Score was calculated with the respective weighted coeffcients by using a logistic regression algorithm, implemented in the primary cohort. Two Rad-Scores were tested in the validation cohort. Univariate and multivariate analyses were used to identify the potential valuable predictors among the clinical characteristics, and the clinical models were built by logistic regression. Then, the identified clinical characteristics and Rad-Score were selected to build the radiomics-based nomograms. To verify the robustness and clinical gain of the final nomograms, the receiver operating characteristic (ROC) curve, calibration curve, and decision curve were plotted, respectively. And the area under ROC curve (AUC), C-index, sensitivity, and specificity were calculated for assessment.
Statistical analysis
All statistical tests were performed using R software version 4.0.2 and SPSS version 25.0. SPSS software was used to perform the Mann-Whitney U test, Spearman test, and univariate/multivariate analyses for feature selection. The RF based Boruta algorithm was performed by using R “Boruta” package. The nomogram, calibration curve, and decision curve were calculated mainly by using R “rmc” and “rmda” packages. The statistically significant level was P<0.05.
Results
Patient characteristics
The details of characteristics in the whole dataset and two tasks are shown in Table 1 and Table 3. Smoking status (P=0.003) and histological type (P<0.001) showed a statistical difference between EGFR mutant and wild type. Gender (P=0.019), smoking status (P=0.001), histological type (P=0.001), and stage (P=0.005) all were significant factors to differentiate high Ki-67 PI expression from low Ki-67 PI expression. Other clinical and histological characteristics (age, tumor location, CT pattern, etc.) were not identified as potential factors for prediction in the two tasks.
Table 3
Characteristics | EGFR mutant/wild-type status | Ki-67 PI high/low expression |
---|---|---|
Gender | NS | P=0.019 |
Age | NS | NS |
Smoking status | P=0.003 | P=0.001 |
Tumor location | NS | NS |
CT pattern | NS | NS |
Histological type | P<0.001 | P=0.001 |
Stage | NS | P=0.005 |
NS, not significant; EGFR, epidermal growth factor receptor; PI, proliferation index; CT, computed tomography.
The selected radiomic features
The extracted radiomic features contained 13 shape-based features, 18 first-order features, 73 texture features, and 1,183 transform features. The feature extraction examples are provided in https://github.com/JZK00/NSCLC_prediction. As shown in Figure S1, there was much redundancy between 1,287 radiomic features, so a three-stage selection method was designed to select strong correlation and remove the redundant features. Through the Mann-Whitney U test and Spearman test, 35 and 83 features were selected in the two tasks, respectively. Then, RF based Boruta selected 3 tentative (yellow) and 7 confirmed (green) features as final features in task [1], and 6 tentative and 22 confirmed features were selected in task [2]. Table S3 showed the detailed parameters. The correlation between radiomic features and clinical characteristics is shown in Figure S2, and no significant correlation was found.
Rad-Score
The Rad-Scores of the two tasks were calculated and provided in Table S4, using 10 and 28 radiomic features, respectively. The validation performance (five-fold cross validation and independent validation) of Rad-Scores is shown in Figure S3. Rad-Score 1 for differentiation of EGFR mutations had a mean AUC of 0.83 in the primary cohort, and an AUC of 0.80 in the validation cohort. Rad-Score 2 of Ki-67 PI expression exhibited better performance (mean AUC of 0.92 in the primary cohort; AUC of 0.82 in the validation cohort). As shown in Figure S4, Rad-Score 1 in the EGFR mutation was significantly higher than wild type (P<0.001), and Rad-Score 2 in Ki-67 PI high expression was significantly higher than Ki-67 PI low expression (P<0.001).
Radiomics-based nomogram
Figure S5 shows the predicted performance of the clinical models. In task [1], the Rad-Score 1 and confirmed clinical features (smoking status and histological type) were incorporated to establish the radiomics-based nomogram (Figure 3A), and the calibration curves in the primary cohort (Figure 3B) and the validation cohort (Figure 3C) were confirmed. The nomogram had a C-index of 0.891 and mean squared error (MSE) of 0.00025 in the primary cohort, and a C-index of 0.798 and MSE of 0.00124 in the validation cohort. In task [2], Rad-Score 2 and 4 potential factors (gender, smoking status, histological type and stage) were used to develop the nomogram for Ki-67 PI prediction, and the calibration curves were calculated (Figure 4). C-index of the nomogram was 0.981 in the primary cohort with MSE of 0.00052, and the C-index was 0.828 with MSE of 0.00333 in the validation cohort. All of the calibration curves showed good agreements between the observed and predicted results.
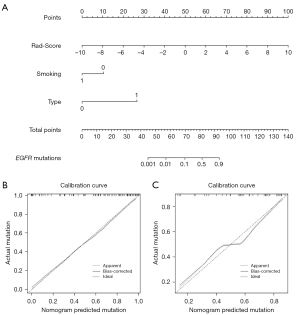
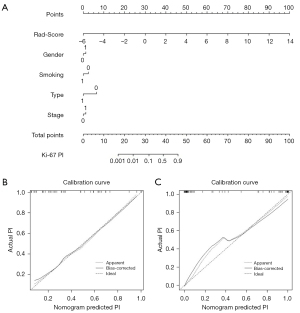
Next, we calculated the ROC curves of two nomograms in two tasks (Figure 5). The nomogram of EGFR mutations had an AUC of 0.798 (95% CI: 0.664 to 0.931), a sensitivity of 84.2%, and a specificity of 65.4% in the validation cohort. The nomogram of Ki-67 PI showed an AUC of 0.828 (95% CI: 0.703 to 0.953), a sensitivity of 80.8%, and a specificity of 73.7% in the validation cohort.
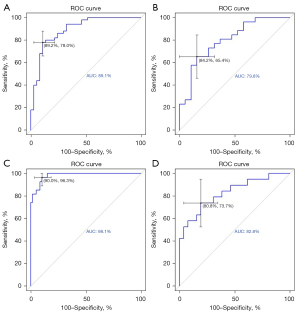
Clinical utility
For further confirming the clinical gain of radiomic models, the decision curves (clinical characteristics, Rad-Scores, and nomograms) were developed and compared in the two tasks, respectively. As shown in Figure 6, using radiomics-based nomograms and Rad-Scores for EGFR and Ki-67 PI prediction added more benefit than using the treat-all scheme or the treat-none scheme at any given threshold of probability, in both the primary and validation cohorts. For EGFR mutation prediction, clinical characteristics added little benefit (threshold probabilities <40%). However, clinical characteristics added more benefit for Ki-67 PI prediction when threshold probabilities >20%. The clinical gain of Rad-Score and nomogram showed similar results.
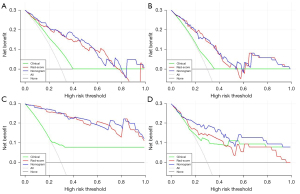
Discussion
Research value
The relationship between tumor biomarkers and imaging features has always been a contentious point. Previous researchers mostly used positron emission tomography (PET)/CT, magnetic resonance imaging (MRI), etc. to evaluate studies (28-30). However, most of these studies have complex or demanding imaging methods and often underutilized the digital information contained in medical images. In fact, CT images, which are more commonly used in tumor diagnosis, treatment and monitoring, can not only show routine descriptive signs, but also contain a huge amount of digital information that can be further extrapolated (16,31). Quantitative radiomics show that these imaging features correlate with the underlying gene expression profile of the tumor and can also predict the molecular typing of some tumors (32,33). CT has become a conventional imaging examination method for diagnosis, treatment and monitoring of lung cancer due to its non-invasive, convenient and quick advantages. Starting with quantitative imaging and using CT images routinely conducted in clinical diagnosis and treatment of tumors, this study found that tumor molecular level information can be obtained from tumor texture analysis. The study further explored the biological behavior of tumors, which is of great importance for determining patients’ prognoses and recommendations of clinical therapy. EGFR gene mutation determines the therapeutic efficacy of EGFR-TKIs, and the Ki-67 PI has been recognized as a valuable tumor biomarker, guiding the diagnosis, treatment, and prognosis of tumor patients. However, both tests are often invasive. Therefore, it is of great clinical significance to predict the EGFR mutation status and the expression of Ki-67 through the analysis of tumor CT images.
In this multicentre study, we successfully solved two important clinical tasks (EGFR mutation prediction and Ki-67 PI expression prediction) using radiomics analysis based on pre-treatment CT images, and we provided the novel radiomic scores and nomograms for clinical application.
Model performance
By analyzing ROC curves, calibration curves and decision curves, we found that our novel radiomic models not only had high accuracy and robustness, but they also had high clinical gain. As shown in Table S5, several former studies (32,34-48) also tried to build radiomics-based classifiers to predict EGFR mutation or Ki-67 PI expression in lung cancer.
The radiomics research for EGFR prediction has made a lot of headway, but Ki-67 PI radiomics research has been relatively lacking. EGFR testing standards have been very mature and uniform, which is helpful for machine learning to implement supervised learning relying on the uniform standard. Although Ki-67 has important clinical application value, the definition of the threshold of its positive expression rate is still controversial, which brings some difficulties to clinical application and research. The three studies in Table S5 for task [2] had three different assessment criteria for high/low Ki-67 PI expression, which could influence comparison and how the research is communicated.
Some studies such as Liu et al. (34) and Gu et al. (48) only modeled on a single central dataset, which limited the generalization of the produced models. An independent validation dataset helps determine the robustness and generalization of the model, which improves the quality of radiomics research. The radiomic signature established by Wang et al. (43) showed that the EGFR mutations could be evaluated in patients with brain metastasis from lung adenocarcinoma using MRI, which highlights a new potential of radiomics.
Zhao et al. (36) and Zhang et al. (45) focused on deep learning-based radiomics and showed high application potential. However, the “black box” problem has always hindered the application of deep learning prediction (49). Hand-crafted radiomics has remained the dominant method in radiomics research (50). Meanwhile, radiomic score and nomogram built by using logistic regression were the most popular forecasting tools.
In our study, the Rad-Scores and nomograms all showed appreciable performance in the independent validation cohort. The AUC of 0.798 for EGFR mutations prediction (Figure 5B) and AUC of 0.828 for Ki-67 PI prediction (Figure 5D) in the validation cohort all showed high diagnostic accuracy. DCA in Figure 6 highlighted the clinical application value of Rad-Scores and nomograms. Compared to similar studies (Table S5), our study was complete and comprehensive, with relatively accurate and reliable results.
Main findings
In our study, smoking status and histological type all showed strong correlation with EGFR and Ki-67 expression (Table 3), which was consistent with previous studies (44,48). In addition, more clinical characteristics showed significant correlation with Ki-67 PI expression, like gender and stage, which may provide reference for clinical work.
The CT radiomic features selected for modeling are provided in Table S5. Similar to previous studies, most of them were texture features, showing the value of inter-tumor heterogeneity in predicting gene expression.
Radiomics quality score (RQS) evaluation
RQS was the most mentionable tool for radiomics quality evaluation (18), which was worth generalizing to every radiomics study. The total RQS score was 36, and our score was 24 (Table S6), which is higher than most radiomics studies (51). This high RQS showed the scientific integrity and reproducibility of this research.
Study limitation
There are still some limitations to our current work. First, the retrospective analysis had its own potential bias, and some clinical information was missing, so we could not provide more analyses on other mutations. Second, although the reliability of multicentre validation was high, our dataset was not big enough to derive more generalizable evidence. In future studies, we need to reappraise the performance of radiomics analysis by designing a prospective multicentre study.
Conclusions
In conclusion, Rad-Scores and radiomics-based nomograms can be implemented as useful, non-invasive tools for identifying the EGFR mutations and Ki-67 PI expression in patients with NSCLC. Our methodology may provide a novel strategy to assess the EGFR mutation status and cell proliferation.
Acknowledgments
The authors thank all volunteers who participated in the study.
Funding: None.
Footnote
Reporting Checklist: The authors have completed the TRIPOD reporting checklist. Available at https://qims.amegroups.com/article/view/10.21037/qims-21-980/rc
Conflicts of Interest: All authors have completed the ICMJE uniform disclosure form (available at https://qims.amegroups.com/article/view/10.21037/qims-21-980/coif). The authors have no conflicts of interest to declare.
Ethical Statement: The authors are accountable for all aspects of the work in ensuring that questions related to the accuracy or integrity of any part of the work are appropriately investigated and resolved. The study was conducted in accordance with the Declaration of Helsinki (as revised in 2013). The study was approved by the Institutional Review Board of Shandong Cancer Hospital and individual consent for this retrospective analysis was waived.
Open Access Statement: This is an Open Access article distributed in accordance with the Creative Commons Attribution-NonCommercial-NoDerivs 4.0 International License (CC BY-NC-ND 4.0), which permits the non-commercial replication and distribution of the article with the strict proviso that no changes or edits are made and the original work is properly cited (including links to both the formal publication through the relevant DOI and the license). See: https://creativecommons.org/licenses/by-nc-nd/4.0/.
References
- Siegel RL, Miller KD, Jemal A. Cancer statistics, 2018. CA Cancer J Clin 2018;68:7-30. [Crossref] [PubMed]
- Devarakonda S, Morgensztern D, Govindan R. Genomic alterations in lung adenocarcinoma. Lancet Oncol 2015;16:e342-51. [Crossref] [PubMed]
- Byrne BJ, Garst J. Epidermal growth factor receptor inhibitors and their role in non-small-cell lung cancer. Curr Oncol Rep 2005;7:241-7. [Crossref] [PubMed]
- Rosell R, Carcereny E, Gervais R, Vergnenegre A, Massuti B, Felip E, et al. Erlotinib versus standard chemotherapy as first-line treatment for European patients with advanced EGFR mutation-positive non-small-cell lung cancer (EURTAC): a multicentre, open-label, randomised phase 3 trial. Lancet Oncol 2012;13:239-46. [Crossref] [PubMed]
- Takano T, Fukui T, Ohe Y, Tsuta K, Yamamoto S, Nokihara H, Yamamoto N, Sekine I, Kunitoh H, Furuta K, Tamura T. EGFR mutations predict survival benefit from gefitinib in patients with advanced lung adenocarcinoma: a historical comparison of patients treated before and after gefitinib approval in Japan. J Clin Oncol 2008;26:5589-95. [Crossref] [PubMed]
- Warth A, Cortis J, Soltermann A, Meister M, Budczies J, Stenzinger A, Goeppert B, Thomas M, Herth FJ, Schirmacher P, Schnabel PA, Hoffmann H, Dienemann H, Muley T, Weichert W. Tumour cell proliferation (Ki-67) in non-small cell lung cancer: a critical reappraisal of its prognostic role. Br J Cancer 2014;111:1222-9. [Crossref] [PubMed]
- Wei DM, Chen WJ, Meng RM, Zhao N, Zhang XY, Liao DY, Chen G. Augmented expression of Ki-67 is correlated with clinicopathological characteristics and prognosis for lung cancer patients: an up-dated systematic review and meta-analysis with 108 studies and 14,732 patients. Respir Res 2018;19:150. [Crossref] [PubMed]
- Gerdes J, Lemke H, Baisch H, Wacker HH, Schwab U, Stein H. Cell cycle analysis of a cell proliferation-associated human nuclear antigen defined by the monoclonal antibody Ki-67. J Immunol 1984;133:1710-5. [PubMed]
- Yang C, Zhang J, Ding M, Xu K, Li L, Mao L, Zheng J. Ki67 targeted strategies for cancer therapy. Clin Transl Oncol 2018;20:570-5. [Crossref] [PubMed]
- Martin B, Paesmans M, Mascaux C, Berghmans T, Lothaire P, Meert AP, Lafitte JJ, Sculier JP. Ki-67 expression and patients survival in lung cancer: systematic review of the literature with meta-analysis. Br J Cancer 2004;91:2018-25. [Crossref] [PubMed]
- Ahn HK, Jung M, Ha SY, Lee JI, Park I, Kim YS, Hong J, Sym SJ, Park J, Shin DB, Lee JH, Cho EK. Clinical significance of Ki-67 and p53 expression in curatively resected non-small cell lung cancer. Tumour Biol 2014;35:5735-40. [Crossref] [PubMed]
- Tabata K, Tanaka T, Hayashi T, Hori T, Nunomura S, Yonezawa S, Fukuoka J. Ki-67 is a strong prognostic marker of non-small cell lung cancer when tissue heterogeneity is considered. BMC Clin Pathol 2014;14:23. [Crossref] [PubMed]
- Lambin P, Rios-Velazquez E, Leijenaar R, Carvalho S, van Stiphout RG, Granton P, Zegers CM, Gillies R, Boellard R, Dekker A, Aerts HJ. Radiomics: extracting more information from medical images using advanced feature analysis. Eur J Cancer 2012;48:441-6. [Crossref] [PubMed]
- Mayerhoefer ME, Schima W, Trattnig S, Pinker K, Berger-Kulemann V, Ba-Ssalamah A. Texture-based classification of focal liver lesions on MRI at 3.0 Tesla: a feasibility study in cysts and hemangiomas. J Magn Reson Imaging 2010;32:352-9. [Crossref] [PubMed]
- Aerts HJ, Velazquez ER, Leijenaar RT, Parmar C, Grossmann P, Carvalho S, Bussink J, Monshouwer R, Haibe-Kains B, Rietveld D, Hoebers F, Rietbergen MM, Leemans CR, Dekker A, Quackenbush J, Gillies RJ, Lambin P. Decoding tumour phenotype by noninvasive imaging using a quantitative radiomics approach. Nat Commun 2014;5:4006. [Crossref] [PubMed]
- Le NQK, Hung TNK, Do DT, Lam LHT, Dang LH, Huynh TT. Radiomics-based machine learning model for efficiently classifying transcriptome subtypes in glioblastoma patients from MRI. Comput Biol Med 2021;132:104320. [Crossref] [PubMed]
- Gillies RJ, Kinahan PE, Hricak H. Radiomics: Images Are More than Pictures, They Are Data. Radiology 2016;278:563-77. [Crossref] [PubMed]
- Lambin P, Leijenaar RTH, Deist TM, Peerlings J, de Jong EEC, van Timmeren J, Sanduleanu S, Larue RTHM, Even AJG, Jochems A, van Wijk Y, Woodruff H, van Soest J, Lustberg T, Roelofs E, van Elmpt W, Dekker A, Mottaghy FM, Wildberger JE, Walsh S. Radiomics: the bridge between medical imaging and personalized medicine. Nat Rev Clin Oncol 2017;14:749-62. [Crossref] [PubMed]
- Limkin EJ, Sun R, Dercle L, Zacharaki EI, Robert C, Reuzé S, Schernberg A, Paragios N, Deutsch E, Ferté C. Promises and challenges for the implementation of computational medical imaging (radiomics) in oncology. Ann Oncol 2017;28:1191-206. [Crossref] [PubMed]
- Jiang Z, Wang B, Han X, Zhao P, Gao M, Zhang Y, Wei P, Lan C, Liu Y, Li D. Multimodality MRI-based radiomics approach to predict the posttreatment response of lung cancer brain metastases to gamma knife radiosurgery. Eur Radiol 2022; [Epub ahead of print]. [Crossref] [PubMed]
- Yu Q, Liu Y, Xie X, Liu J, Huang S, Zhang X, Ju S. Radiomics-based method for diagnosis of calciphylaxis in patients with chronic kidney disease using computed tomography. Quant Imaging Med Surg 2021;11:4617-26. [Crossref] [PubMed]
- Ou J, Wu L, Li R, Wu CQ, Liu J, Chen TW, Zhang XM, Tang S, Wu YP, Yang LQ, Tan BG, Lu FL. CT radiomics features to predict lymph node metastasis in advanced esophageal squamous cell carcinoma and to discriminate between regional and non-regional lymph node metastasis: a case control study. Quant Imaging Med Surg 2021;11:628-40. [Crossref] [PubMed]
- Rami-Porta R, Asamura H, Travis WD, Rusch VW. Lung cancer - major changes in the American Joint Committee on Cancer eighth edition cancer staging manual. CA Cancer J Clin 2017;67:138-55.
- Goldhirsch A, Wood WC, Coates AS, Gelber RD, Thürlimann B, Senn HJ. Panel members. Strategies for subtypes--dealing with the diversity of breast cancer: highlights of the St. Gallen International Expert Consensus on the Primary Therapy of Early Breast Cancer 2011. Ann Oncol 2011;22:1736-47. [Crossref] [PubMed]
- van Griethuysen JJM, Fedorov A, Parmar C, Hosny A, Aucoin N, Narayan V, Beets-Tan RGH, Fillion-Robin JC, Pieper S, Aerts HJWL. Computational Radiomics System to Decode the Radiographic Phenotype. Cancer Res 2017;77:e104-7. [Crossref] [PubMed]
- Liu Z, Li Z, Qu J, Zhang R, Zhou X, Li L, Sun K, Tang Z, Jiang H, Li H, Xiong Q, Ding Y, Zhao X, Wang K, Liu Z, Tian J. Radiomics of Multiparametric MRI for Pretreatment Prediction of Pathologic Complete Response to Neoadjuvant Chemotherapy in Breast Cancer: A Multicenter Study. Clin Cancer Res 2019;25:3538-47. [Crossref] [PubMed]
- Lai WT, Deng WF, Xu SX, Zhao J, Xu D, Liu YH, Guo YY, Wang MB, He FS, Ye SW, Yang QF, Liu TB, Zhang YL, Wang S, Li MZ, Yang YJ, Xie XH, Rong H. Shotgun metagenomics reveals both taxonomic and tryptophan pathway differences of gut microbiota in major depressive disorder patients. Psychol Med 2021;51:90-101. [Crossref] [PubMed]
- Shen G, Ma H, Pang F, Ren P, Kuang A. Correlations of 18F-FDG and 18F-FLT uptake on PET with Ki-67 expression in patients with lung cancer: a meta-analysis. Acta Radiol 2018;59:188-95. [Crossref] [PubMed]
- Caiazzo C, Di Micco R, Esposito E, Sollazzo V, Cervotti M, Varelli C, Forestieri P, Limite G. The role of MRI in predicting Ki-67 in breast cancer: preliminary results from a prospective study. Tumori 2018;104:438-43. [Crossref] [PubMed]
- Karaman A, Durur-Subasi I, Alper F, Araz O, Subasi M, Demirci E, Albayrak M, Polat G, Akgun M, Karabulut N. Correlation of diffusion MRI with the Ki-67 index in non-small cell lung cancer. Radiol Oncol 2015;49:250-5. [Crossref] [PubMed]
- Ninatti G, Kirienko M, Neri E, Sollini M, Chiti A. Imaging-Based Prediction of Molecular Therapy Targets in NSCLC by Radiogenomics and AI Approaches: A Systematic Review. Diagnostics (Basel) 2020;10:359. [Crossref] [PubMed]
- Zhang L, Chen B, Liu X, Song J, Fang M, Hu C, Dong D, Li W, Tian J. Quantitative Biomarkers for Prediction of Epidermal Growth Factor Receptor Mutation in Non-Small Cell Lung Cancer. Transl Oncol 2018;11:94-101. [Crossref] [PubMed]
- Li H, Zhu Y, Burnside ES, Huang E, Drukker K, Hoadley KA, Fan C, Conzen SD, Zuley M, Net JM, Sutton E, Whitman GJ, Morris E, Perou CM, Ji Y, Giger ML. Quantitative MRI radiomics in the prediction of molecular classifications of breast cancer subtypes in the TCGA/TCIA data set. NPJ Breast Cancer 2016; [Crossref] [PubMed]
- Liu Y, Kim J, Balagurunathan Y, Li Q, Garcia AL, Stringfield O, Ye Z, Gillies RJ. Radiomic Features Are Associated With EGFR Mutation Status in Lung Adenocarcinomas. Clin Lung Cancer 2016;17:441-448.e6. [Crossref] [PubMed]
- Jia TY, Xiong JF, Li XY, Yu W, Xu ZY, Cai XW, Ma JC, Ren YC, Larsson R, Zhang J, Zhao J, Fu XL. Identifying EGFR mutations in lung adenocarcinoma by noninvasive imaging using radiomics features and random forest modeling. Eur Radiol 2019;29:4742-50. [Crossref] [PubMed]
- Zhao W, Yang J, Ni B, Bi D, Sun Y, Xu M, Zhu X, Li C, Jin L, Gao P, Wang P, Hua Y, Li M. Toward automatic prediction of EGFR mutation status in pulmonary adenocarcinoma with 3D deep learning. Cancer Med 2019;8:3532-43. [Crossref] [PubMed]
- Zhao W, Wu Y, Xu Y, Sun Y, Gao P, Tan M, Ma W, Li C, Jin L, Hua Y, Liu J, Li M. The Potential of Radiomics Nomogram in Non-invasively Prediction of Epidermal Growth Factor Receptor Mutation Status and Subtypes in Lung Adenocarcinoma. Front Oncol 2020;9:1485. [Crossref] [PubMed]
- Tu W, Sun G, Fan L, Wang Y, Xia Y, Guan Y, Li Q, Zhang D, Liu S, Li Z. Radiomics signature: A potential and incremental predictor for EGFR mutation status in NSCLC patients, comparison with CT morphology. Lung Cancer 2019;132:28-35. [Crossref] [PubMed]
- Hong D, Xu K, Zhang L, Wan X, Guo Y. Radiomics Signature as a Predictive Factor for EGFR Mutations in Advanced Lung Adenocarcinoma. Front Oncol 2020;10:28. [Crossref] [PubMed]
- Lu X, Li M, Zhang H, Hua S, Meng F, Yang H, Li X, Cao D. A novel radiomic nomogram for predicting epidermal growth factor receptor mutation in peripheral lung adenocarcinoma. Phys Med Biol 2020;65:055012. [Crossref] [PubMed]
- Koyasu S, Nishio M, Isoda H, Nakamoto Y, Togashi K. Usefulness of gradient tree boosting for predicting histological subtype and EGFR mutation status of non-small cell lung cancer on 18F FDG-PET/CT. Ann Nucl Med 2020;34:49-57. [Crossref] [PubMed]
- Nair JKR, Saeed UA, McDougall CC, Sabri A, Kovacina B, Raidu BVS, Khokhar RA, Probst S, Hirsh V, Chankowsky J, Van Kempen LC, Taylor J. Radiogenomic Models Using Machine Learning Techniques to Predict EGFR Mutations in Non-Small Cell Lung Cancer. Can Assoc Radiol J 2021;72:109-19. [Crossref] [PubMed]
- Wang G, Wang B, Wang Z, Li W, Xiu J, Liu Z, Han M. Radiomics signature of brain metastasis: prediction of EGFR mutation status. Eur Radiol 2021;31:4538-47. [Crossref] [PubMed]
- Rossi G, Barabino E, Fedeli A, Ficarra G, Coco S, Russo A, Adamo V, Buemi F, Zullo L, Dono M, De Luca G, Longo L, Dal Bello MG, Tagliamento M, Alama A, Cittadini G, Pronzato P, Genova C. Radiomic Detection of EGFR Mutations in NSCLC. Cancer Res 2021;81:724-31. [Crossref] [PubMed]
- Zhang B, Qi S, Pan X, Li C, Yao Y, Qian W, Guan Y. Deep CNN Model Using CT Radiomics Feature Mapping Recognizes EGFR Gene Mutation Status of Lung Adenocarcinoma. Front Oncol 2021;10:598721. [Crossref] [PubMed]
- Le NQK, Kha QH, Nguyen VH, Chen YC, Cheng SJ, Chen CY. Machine Learning-Based Radiomics Signatures for EGFR and KRAS Mutations Prediction in Non-Small-Cell Lung Cancer. Int J Mol Sci 2021;22:9254. [Crossref] [PubMed]
- Zhou B, Xu J, Tian Y, Yuan S, Li X. Correlation between radiomic features based on contrast-enhanced computed tomography images and Ki-67 proliferation index in lung cancer: A preliminary study. Thorac Cancer 2018;9:1235-40. [Crossref] [PubMed]
- Gu Q, Feng Z, Liang Q, Li M, Deng J, Ma M, Wang W, Liu J, Liu P, Rong P. Machine learning-based radiomics strategy for prediction of cell proliferation in non-small cell lung cancer. Eur J Radiol 2019;118:32-7. [Crossref] [PubMed]
- Castelvecchi D. Can we open the black box of AI? Nature 2016;538:20-3. [Crossref] [PubMed]
- Mohammadi A, Afshar P, Asif A, Farahani K, Kirby J, Oikonomou A, Plataniotis KN. Lung Cancer Radiomics: Highlights from the IEEE Video and Image Processing Cup 2018 Student Competition. IEEE Signal Process Mag 2019;36:164-73. [Crossref] [PubMed]
- Park JE, Kim D, Kim HS, Park SY, Kim JY, Cho SJ, Shin JH, Kim JH. Quality of science and reporting of radiomics in oncologic studies: room for improvement according to radiomics quality score and TRIPOD statement. Eur Radiol 2020;30:523-36. [Crossref] [PubMed]