A phantom study comparing low-dose CT physical image quality from five different CT scanners
Introduction
Due to the aging population in China, the prevalence of osteoporosis and the risk of fragility fractures have increased significantly (1). The diagnosis and prevention of osteoporosis is mainly dependent on opportunistic screening by quantitative computed tomography (QCT) and the accuracy of the bone mineral density (BMD) measurements obtained (2,3). However, patients with a high risk of osteoporosis are often exposed to a significant amount of radiation dose due to repeated lumbar CT scans. According to the principle of “as low as reasonably achievable (ALARA)” (4), it is essential to optimize the radiation dose patients are exposed to while still maintaining the quality of the clinical diagnostic image. Low-dose computed tomography (LDCT) has been applied as a promising approach for lung cancer screening, especially for early-stage lung cancer (3,5,6), as it can reduce the radiation dose patients receive (7), thereby reducing mortality (8) in high-risk populations. Hence, the combination of LDCT and lumbar QCT was proposed by the China Health Big Data (China Biobank) project to screen for osteoporosis and lung cancer simultaneously with high precision. This method allows for improved quality of clinical diagnostic images without the need for extra equipment, repeated examination, extra patient time, excessive radiation exposure, or substantial additional costs (3,9,10). BMD is a primary tool used to evaluate the fracture risk of osteoporosis in patients. It is also used for health screening, assisting clinicians and patients in making therapeutic decisions, and monitoring therapies (11). When measuring the BMD values of the lumbar spine and in standard-of-care diagnostic settings, the tube voltage of the LDCT scan can be set to 120 kV, according to the current recommendation for QCT, without affecting the number of Hounsfield units (HU) or BMD values compared with other tube voltages (12). However, reducing the radiation dose can increase the image noise and significantly degrade the image quality. This is particularly important for spinal LDCT, and it is thus essential to identify the image quality and differences between different CT scanners using an anthropomorphic phantom for calibration-based quality assurance.
While many studies have examined the use of LDCT screening for lung cancer and osteoporosis, few have compared the physical image quality obtained from the Catphan 500 using CT scanners from different manufacturers (3,9,13). Therefore, this study performed phantom experiment to systematically investigate the physical image quality of CT scanners from 5 different manufacturers using an LDCT scan protocol.
Methods
CT scanners
This study was performed by clinicians with postgraduate or higher qualifications, the majority of whom had expertise in osteoporosis preservation and medical physics. Technologists performed weekly air calibration on the CT scanners and data acquisitions. The weekly quality assurance (QA) testing and the image synthesis and evaluation of the CT number linearity, modulation transfer function (MTF), as well as the noise power spectrum (NPS) curves were acquired by engineers and performed by radiologists, respectively. The image acquisitions were performed on CT scanners from 5 different manufacturers, including Revolution CT (GE Healthcare, WI, USA), Brilliance iCT (Philips Healthcare, OH, USA), Somatom Force (Siemens Healthineers, Erlangen, Germany), Minfound 128 ScintCare CT (MinFound Medical Systems, Zhejiang, China), and Neusoft NeuViz Glory (Neusoft Medical Systems, Shenyang, China). Each scanner had <5 years of scanning, and a patient workload (an average/a maximum) of 80 scans/day. Monthly QA testing was performed on all CT scanners, and the CT number calibration was conducted before each data acquisition throughout the experimental period to ensure optimal image quality.
Catphan 500 phantom
QA assessment of the CT images was performed with a phantom study to measure the standard physical metrics of the CT scanners using the Catphan 500 phantom (Phantom Laboratory, Salem, NY, USA) which consists of 4 modules. The CTP401 module consists of 4 wire ramps raised at 23° angles from the base to the top of the module, with a diameter of 15 cm and a slice width of 2.5 cm. It is used to evaluate the CT number linearity and accuracy of 4 insert materials made from Teflon, acrylic, low-density polyethylene (LDPE), and air. The CTP528 module consists of 21 line-pairs-per-centimeter (lp/cm) high-resolution test gauges and 2 impulse beads cast into a uniform material. It has a diameter of 15 cm and a slice width of 4 cm, and is used to measure the high-contrast spatial resolution. The CTP515 module is configured with supraslice and subslice contrast targets. It has a diameter of 15 cm and a slice width of 4 cm and is used to measure the low-contrast detectability. The CTP486 module is designed to be within a 2% of water density, has a diameter of 15 cm and a slice width of 5cm, and is used to evaluate image noise and image uniformity. The phantom was positioned separately in the isocenter of each CT scanner according to the manufacturer’s recommendations (14).
Data acquisition and image reconstruction
CT images were obtained by LDCT with a low-tube current and manufacturer-specific iterative reconstruction (IR) algorithms, and by helical acquisition with a fixed tube voltage of 120 kV. The radiation dose was restricted to an ultralow-dose level, and the tube current was set to yield a volume CT dose index (CTDIvol) at 2 equivalent dose levels of 0.25 and 0.75 mGy, which corresponds to the dose settings for LDCT lung cancer screening in our previous study (9). The images reconstructed with filtered back projection (FBP) were acquired from all CT scanners. The images from the 5 CT scanners were reconstructed with manufacturer-specific algorithms using adaptive statistical iterative reconstruction (ASiR-V 50%) and new generation deep learning image reconstruction (DLIR) at 3 different levels (low, medium and high), fourth-generation hybrid iterative reconstruction (iDose4; level 3), advanced modeled iterative reconstruction (ADMIRE; level 3), nano dose iteration (NDI; level 3), and clear view (CV; 50%), sequentially. The scan parameters for all scanners are presented in Table 1. All CT scanners were performed with the same scan parameters, and all data acquisitions were performed 3 consecutive times.
Table 1
CT parameters | GE Healthcare | Philips | Siemens | Minfound | Neusoft |
---|---|---|---|---|---|
Tube voltage (kV) | 120 | 120 | 120 | 120 | 120 |
CTDIvol (mGy) | 0.25/0.75 | 0.25/0.75 | 0.25/0.75 | 0.25/0.75 | 0.25/0.75 |
Tube current-time product (mAs) | 25/75 | 20/60 | 28/82 | 10/30 | 25/75 |
Thickness/increment (mm) | 1.25 | 1.0 | 1.0 | 1.25 | 1.25 |
Pitch (approximate number) | 1 | 1 | 1 | 1 | 1 |
Detector configuration (mm) | 256×0.625 | 64×0.625 | 96×0.6 | 64×0.625 | 64×0.625 |
Matrix size | 512×512 | 512×512 | 512×512 | 512×512 | 512×512 |
Voxel size (mm) | 0.61 | 0.49 | 0.49 | 0.61 | 0.61 |
DFOV (mm) | 250 | 250 | 250 | 250 | 250 |
Reconstruction kernel | Standard | Standard | Standard | Standard | Standard |
Reconstruction algorithms | FBP/ASiR-V50%/DLIR (L/M/H) | FBP/iDose4 [3]/IMR [2] | FBP/ADMIRE [3] | FBP/NDI [3] | FBP/CV50% |
Acquisition mode | Helical | Helical | Helical | Helical | Helical |
Gantry rotation time (s) | 0.5 | 0.5 | 0.5 | 0.5 | 0.5 |
CT, computed tomography; CTDIvol, volume CT dose index; mGy, milligray; DFOV, display field of view; FBP, filtered back projection; ASiR-V50%, adaptive statistical iterative reconstruction 50%; DLIR (L/M/H), deep learning image reconstruction, level low, medium, and high; iDose4 [3], fourth-generation hybrid iterative reconstruction, level 3; IMR [2], iterative model reconstruction, level 2; ADMIRE [3], advanced modeled iterative reconstruction, level 3; NDI [3], nano dose iteration, level 3; CV 50%, clear view 50%.
Dosimetry parameters
The computed tomography dose index (CTDI) consists of 3 dose indicators of CTDI100, while the weighted CTDI (CTDIw) and the CTDIvol were the QA dose quantities used to compare the doses among the different scan protocols (15,16).
CTDI100
CTDI100 is a linear measure of the dose distribution for a pencil dosimeter (16), denoted by the following formula:
where N is the number of slices acquired by a single scan, T is the nominal thickness of one slice, and D (z) is a dose profile along the longitudinal axis, centered at z =0 (17). The measurement of CTDI100 in a phantom is made at the center and at 4 peripheral positions 1 cm below the phantom surface (15).
CTDIw
CTDIw represents the CTDI100 weighted average over the cross-section of standardized PMMA phantoms (16) and is calculated using the following formula:
where CTDI100,c and CTDI100,p refer to the CTDI100 measured at the center hole and the average of 4 measurements at the peripheral positions in the phantom, respectively (15).
CTDIvol
CTDIvol is the pitch-corrected CTDIw with a unit of mGy (16), and is calculated as follow:
Data measurement and image evaluation
CT number linearity
The expected values of the 4 insert materials ranged from approximately +1,000 HU to –1,000 HU (Teflon ≈990 HU, acrylic ≈120 HU, LDPE ≈–100 HU, and air ≈–1,000 HU) (14). Four circle-shaped regions of interest (ROIs) with radii of 4–5 mm were drawn and placed in the periphery of the image at the center of the module to measure the HU value and the standard deviation (SD) of the 4 insert materials. To evaluate the CT number linearity, the expected HU values of the 4 materials were defined as the horizontal axis and the measured HU values as the vertical axis. A simple straight line was then used for linear fitting. Ideally, if the measured HU value is equal to the expected HU value, the curve will pass through the origin of coordinates with a slope of 1. The closer the curve is to the actual fitting, the better the linearity will be.
High-contrast spatial resolution
The high-contrast spatial resolution determines the ability of a CT system to resolve high-contrast objects of increasing spatial frequency (18). It is evaluated by visual evaluation with 21 lp/cm high-resolution gauges, and the bead point source and the MTF are estimated by an objective evaluation of the impulse source. The high-resolution bars of the scan images were resolved directly through adjustment of the window width (WW) and window level (WL) until the highest number of visible line pairs was resolved. The high-resolution bars were visually counted independently and blindly by 2 radiologists, each with 5 years of radiology experience, to determine the high-contrast spatial resolution (19-21). An objective evaluation was conducted to measure the high-contrast spatial resolution via the MTF curve that could distinguish the line pairs to a decimal level and analyze the curve trend within the low- and high-frequency ranges. The MTF curve represents the imaging capability of the CT system for the different frequency components.
An in-plane MTF characterized the high-contrast spatial resolution. A ROI centered on the bead-point object image was selected to calculate the MTF, while the background of the ROI was subtracted, and the point spread function was integrated to yield the line spread function (LSF). The MTF curve was derived from a Fourier transform of the LSF and normalized with zero frequency (22).
Low-contrast detectability
Low-contrast detectability refers to the ability to differentiate objects with slightly different densities from their backgrounds (23). The CTP515 module consists of 3 groups of cylindrical rods at 3 contrast levels of 1%, 0.5%, and 0.3% nominal contrast, with diameters of 15, 9, 8, 7, 6, 5, 4, 3, and 2 mm. The supraslice target readability enables a visual evaluation of small objects with low-contrast from their backgrounds. The low-contrast detectability of CT images was determined by the smallest supraslice target diameter at the nominal contrast level of 1.0% (14). The WW and WL were adjusted to identify the diameter of the clearest supraslice target on the CT images at different dose levels and in the IR algorithms. Before manually counting the smallest supraslice target diameter, a direct side-by-side comparison was made by the same 2 radiologists to acquire the low-contrast detectability, and readings were carried out independently and blindly (20). The equations are as follows:
where CTM is the mean CT number of contrast holes, CTB is the mean CT number of the background, and SDmax is the largest SD of the image noise. The subjective evaluation of image quality was performed by visually detecting low-contrast objects in various sizes (24).
Image noise
The CTP486 module is the image uniformity module cast from a uniform material, of which the CT number is designed to be within 2% of water density (–25 to 25 HU). It dedicated to the typical CT number in the range of 5–18 HU, and it is scanned to measure the mean and SD of the CT numbers within an ROI and image noise (14). Five circle-shaped regions of interest (ROIs) with radii of 5–6 mm were drawn and placed in central and peripheral locations (clock positions 12, 3, 6, and 9) within the scan images to determine the mean CT number, SD, and the image uniformity (14). The image uniformity was calculated by the deviation between the central and peripheral locations of the minimum and maximum CT number values with a recommended standard level of ±4 HU (25,26).
Noise characterization is a significant component of image quality measurement, and it evaluates the NPS curve that represents the frequency and magnitude of image noise. Fourier transform represents the zero-mean input and output distribution; thus, the NPS is derived from the average modulus-squared Fourier transform of the zero-mean image signal. The 2D NPS is computed using the discrete Fourier transform (DFT), and 1D normalized NPS (NNPS) is determined by averaging the NPS values in terms of the same radial frequency in order to compare the NPS results and decrease the statistical fluctuations (22). Finally, an 11th order polynomial is used to fit the curve to further reduce the statistical fluctuations without changing the shape of the NPS curve. The image noise is calculated by using the following equation:
where ROIi(x, y) is the signal in the i-th ROI, is the mean of ROIi(x, y), N is the total number of ROIs(N=1), and FFT is the fast Fourier transform. The quantities of Dx, Dy, Nx, and Ny are the pixel spacing and the number of pixels at the x-direction and y-direction (27,28).
Two sets of the same CT images were acquired and subtracted using MATLAB software (MathWorks, Natick, MA, USA) to obtain an NPS curve for each reconstruction condition.
Statistical analysis
All data analyses were performed using SPSS 20 software (IBM Corp., Armonk, NY, USA). All curves of the CT number linearity, the MTF, and the NPS were computed by MATLAB R2018b. Data are expressed as mean ± SD for continuous variables. Image high-contrast spatial resolution and image low-contrast detectability were compared directly, while CT number linearity, image uniformity, and image noise were compared with intergroup differences using one-way analysis of variance (ANOVA). Under different conditions, the least significant difference (LSD) was used to compare groups, and a homogeneity of variance test was performed to check the homogeneity assumption. A P value <0.05 was considered statistically significant.
Results
CT number linearity
For all 5 CT scanners examined, the measured HU values were consistent with the expected HU values, and the fitting curve indicated unbiased CT number linearity (Figure 1, Figure S1, Tables S1-S5). There was no statistically significant difference in CT number linearity of the 4 insert materials, namely, acrylic [95% confidence interval (CI): 120.35 to 121.27; P=0.134], LDPE (95% CI: –98.43 to –97.43; P=0.070), air (95% CI: –996.16 to –994.51; P=0.018), and Teflon (95% CI: 984.40 to 986.87; P=0.883) among the 5 CT scanners under different radiation doses and IR algorithms.
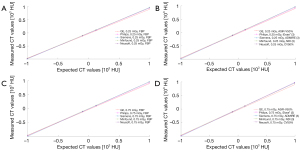
High-contrast spatial resolution
Subjective evaluation
The images acquired from the CTP528 module were visually inspected (Figure 2, Figure S2). In general, the high-contrast spatial resolution was comparable for each of the CT scanners from the 5 different manufacturers, and most of the high-resolution bars were clearly separable at 7 lp/cm but became blurred at 8 lp/cm. The resolving power was high-qualified, and the images showed similar spatial resolution on the 5 CT scanners. The bars of all acquired images at 0.25 mGy were less visible than those at 0.75 mGy.
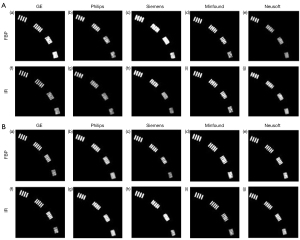
Objective evaluation
Generally, the MTF curve showed similar trends among the 5 different CT scanners, and the resolution levels for each scanner were approximately identical at 50% MTF, 10% MTF, and 5% MTF (Figure 3, Figure S3). The resolving power was similar between the objective evaluation and the subjective evaluation at 10% MTF (6.98±0.40 lp/cm). The observations demonstrated that the resolution at 10% MTF could be used to evaluate the high-contrast spatial resolution of the CT scanner. The MTF values of all the reconstruction algorithms at 10% MTF, and 5% MTF were not significantly different. The conventional IR algorithms had 50% MTF values of about 4 lp/cm or less, while DLIR at all 3 levels had 50% MTF values about 4.5 lp/cm. The MTF curves of FBP were better than those of the manufacturer-specific IR algorithms at 0.25 and 0.75 mGy, and the MTF curves of DLIR at 3 strength levels were better than that of IMR [2] algorithms (Figure S3). There were no significant differences among the manufacturer-specific IR algorithms on the 5 CT scanners (P>0.05).
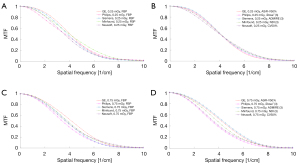
Low-contrast detectability
The low-contrast detectability for the CT scanners from the different manufacturers under different acquisition conditions are shown in Figure 4 and Figure S4. All acquired images were viewed in a fixed window setting (WW/WL, 70/100 HU) according to the above equations (24). In general, a 4 mm low-contrast object at a 0.5% contrast level could be clearly identified on all 5 CT scanners, and a diameter of <5 mm confirmed that the images were qualified in their quality (29). For all reconstruction algorithms, the image noise at 0.75 mGy was lower than that at 0.25 mGy, the image noise of the IR algorithms was lower than that of FBP, and the image noise of the DLIR and IMR [2] algorithms were significantly lower than the image noise of the 5 IR algorithms. These observations revealed that higher IR strength resulted in lower image noise.
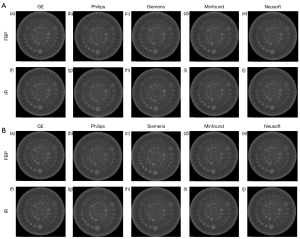
Image noise
Image uniformity
The image uniformity values for CT scanner from GE Healthcare (95% CI: 3.24 to 3.83; P=0.138), Philips (95% CI: 2.62 to 3.70; P=0.299), Siemens (95% CI: 2.10 to 3.59; P=0.054), Minfound (95% CI: 2.35 to 3.65; P=0.589), and Neusoft (95% CI: 2.63 to 3.37; P=0.900) were assessed and fell within the recommended standard level of ± 4 HU. The uniformity values ranged from 1.40–3.90 HU, and the SD ranged from 0.99–2.76 HU, while the deviation in uniformity values varied by less than 2 HU from the baseline values (25,30). There was no significant difference among the different CT scanners when imaging with different IR algorithms and radiation doses (P>0.05), which validated that the image uniformity of the CT scanners from 5 different manufacturers was satisfactory. The detailed uniformity values are presented in Table S6.
The NPS curve
The NPS curve and the NNPS curve are shown in Figures 5,6, and Figures S5,S6. Increasing the radiation dose, the strength of the IR, and the level of DLIR will induce a decrease in the NPS peak. All 3 levels of DLIR algorithms contributed to a perfectly fitting curve with no frequency shift at the 2 dose levels. The NPS curve with a high strength showed a low apex and a low noise level. The NPS peaks were higher at 0.25 mGy than at 0.75 mGy, but there was no significant shift in the NPS peak frequency as the radiation dose increased. The apex of the NPS curve with manufacturer-specific IR algorithms was significantly lower than that for the FBP algorithms. The apex of the NPS curve for DLIR algorithms was higher than that for the IMR [2] algorithm which had an irregularly normalized NPS curve.
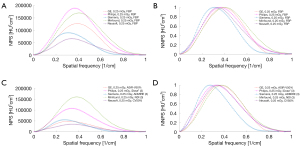
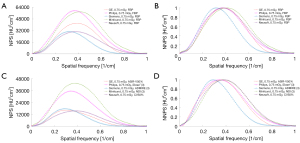
In general, all NNPS curves had similar shapes under different acquisition conditions. The NNPS curve showed a shift in the peak frequency towards a low level and had the low-frequency range as the radiation dose and IR strength increased, whereas the FBP led to a shift towards a high level.
Discussion
It should be noted that all 5 CT scanners showed similar image quality and unbiased CT number linearity in the LDCT scan, which demonstrated that this scan protocol contributes to the stability of HU values and the accuracy of BMD quantification in the different CT scanners. Therefore, it is acceptable to perform the LDCT and lumbar QCT scans together to simultaneously screen for lung cancer, osteoporosis, and osteoporosis-associated fragility fractures (11). The “China Biobank” project and some other related studies are in agreement with our study (3,9,10).
Different QCT scan protocols can change the CT values, which can then influence BMD distribution in the QCT images (31). In this study, a Catphan 500 phantom was scanned at a fixed tube voltage of 120 kV, which assured the accuracy of the BMD values. The CT attenuation numbers are only affected by bean energy (kVp), whereas the variable tube current mainly affects image noise; thus, changing the tube voltage will influence QCT measurements (32). The tube voltage of a standard lumbar spine scan is set to 120 kV. In patients, the BMD values derived from routine lumbar spine scans at 120 kV are more accurate than those at 140 kV (33). Many previous studies have shown that LDCT scans at 120 kV can accurately measure the BMD of the spine by QCT (3,9,10). At a given tube voltage of 120 kV, the image noise is mainly influenced by the tube current-exposure time product, reconstruction kernel, and slice thickness (34). Though an LDCT scan can reduce the radiation dose, its contribution to the image noise is greatly increased. To improve the image quality in LDCT, we adopted the manufacturer-specific IR algorithms for the CT scanners and compared it to FBP, including the DLIR algorithms (new-generation deep learning image reconstruction algorithms). The DLIR algorithm from GE Healthcare integrates image quality improvement knowledge into a DNN composed by layers of mathematical equations that comprise many parameters to represent the characteristics of high-quality images even when acquired CT data is degraded by lower dose or non-ideal scanning conditions. These algorithms could reduce the radiation dose significantly without altering the image noise and produced high diagnostic quality images at a low radiation dose compared to other IR algorithms and FBP (35-37).
To investigate the influence of radiation dose and reconstruction algorithm on CT image quality and the dose reduction potential in a routine LDCT scan, we analyzed the CT images of 5 different CT scanners designed for QA assessment at 2 ultralow-dose levels. There was no statistically significant difference in CT number linearity of the 4 insert materials (P>0.05) among the 5 CT scanners under different radiation doses and IR algorithms. The resolution level at 10% MTF was 6.98 lp/cm on average, which was similar to the subjective evaluation results (mostly up to 7 lp/cm). Increasing the radiation dose and IR strength will decrease the image noise, NPS curve peak frequency, and a leftward shift of the NNPS curve, while improving the low-contrast detectability.
The principle of QCT measurement is to measure the volumetric bone mineral density (vBMD) of the lumbar vertebral body for the diagnosis of osteoporosis by converting a QCT number into a calibrated HU (38-40). CT number linearity is a significant consideration for the quantitative analysis of QCT, and our results showed that CT number linearity was unbiased, which contributed to the stability of HU values of the 5 CT scanners. The accurate BMD quantification calculated by the amount of calcium hydroxyapatite (HA) per volume unit of bone is the key to evaluating osteoporosis (19). The European Spine Phantom (No.145) contains 3 different inserts with trabecular bone compartments consisting of nominal design values of 50.2, 100.6, and 199.2 mg/cm3, which mimic the physiological range of BMD (13). A previous study showed that for reconstructed HA images of 0, 100, 200 mg/cm3, 120kVp, and 3 energy levels of 40, 70, and 140 keV, the mean HU value was within the range of 0–900 HU (41). The expected HU values of acrylic, LDPE, air, and Teflon were 120, –100, –1,000, and 990 HU, respectively (14). Thus, the HU values of HA had an excellent correlation with the 4 insert materials. Therefore, the LDCT scan protocol is significantly suitable for QCT measurements of BMD values.
In terms of high-contrast spatial resolution, there were no significant differences among the 5 CT scanners, which showed that the resolution level at 50% MTF exhibited excellent performance for low-contrast spatial frequency, which is effective for the detection of low-contrast targets, such as the breast and abdomen (42-44). The resolving power was similar between 10% MTF and the subjective evaluation. The high-contrast spatial resolution at 5% MTF approached 8.00 lp/cm, reaching its limit at 2% MTF (9.29 lp/cm), as suggested in the user manual (14). These findings demonstrated that at 10% and 5% MTF, a superior high-contrast spatial resolution was achieved for high-contrast targets. Therefore, the high-contrast spatial resolution was evaluated using the value of 10% MTF (44,45). In our study, at 50% MTF, 10% MTF, and 5% MTF, the high-contrast spatial resolution of FBP was higher than that of the IR algorithms, which confirmed that high-contrast spatial resolution decreased with the increase in the strength of the IR algorithms. DLIR at all 3 levels had the highest 50% MTF values among all reconstruction algorithms. FBP is sufficient for the screening of high-contrast lesions, while IR algorithms are effective for low-contrast lesions. There are some controversies regarding the effects of IR algorithms on high-contrast spatial resolution. It has been reported in some studies that high-contrast spatial resolution is decreased by IR algorithms (46,47), which is consistent with our results. However, some studies suggest that IR algorithms do not affect the high-contrast spatial resolution compared to FBP (48-50), but the subjective image quality assessment indicated that the high-contrast spatial resolution varied greatly among different clinicians. Thus, MTF analysis may more accurately evaluate spatial resolution.
Image uniformity describes the consistency of the CT numbers of a homogenous material in the image, and the measurement of image uniformity has great significance in avoiding cupping and beam-hardening artifacts (22). The image uniformity values ranged from 1.40–3.90 HU and the SD ranged from 0.99–2.76 HU, which varied by less than ±4 HU (corresponding to the recommended standard level) and 2 HU from baseline values on the CT scanners from 5 different manufacturers, respectively. This was consistent with previous studies (25,26,30,51). However, the study by Miura et al. (52) differed slightly, as these authors reported that the image uniformity of simultaneous cone-beam CT (SCBCT) and dual cone-beam CT (DCBCT) was 6.8/10.9 and 31.1 HU, respectively, which was higher than 4 HU.
Image noise is an essential factor for image quality, while the radiation dose and IR algorithm are the main factors for image noise. After increasing the radiation dose and IR strength simultaneously, the apex of the NPS curve of the radiation dose declined faster than that of the IR algorithm. The NNPS curve showed a slight shift in the peak frequency with increased radiation dose. It had a shift in the peak frequency towards a lower level with IR algorithms, but shifted to a higher level with FBP. The 3 levels of DLIR algorithms can reduce image noise while preserving noise texture and the IMR [2] algorithm contributed to the lowest image noise without compromising the image quality. Therefore, all of reconstructions used in this study improved the detectability of low-contrast objects, whereas FBP improved the detectability of high-contrast objects. In a previous study, FBP was shown to be sufficient for detecting high-contrast lesions, such as osteoporotic fractures with an optimal cutoff of 117.9 HU, and pseudoarthrosis with a value of 139.8 HU (53).
In comparison, the IR algorithm can improve the detection of low-contrast lesions such as synthetic ground-glass opacities (GGOs) with a CT value of –800 HU and emphysema with a threshold of –950 HU (53-55). Lesion detectability was improved by optimizing the IR algorithms corresponding to the clinical diagnosis. Notably, all 3 levels of DLIR algorithms can reduce image noise while preserving noise texture and anatomical and pathological details compared to other algorithms (56).
This study has several limitations. First, the accuracy of BMD values in this study was evaluated by converting the CT number into the calibrated HU. Further investigation into the accuracy of the BMD values of the lumbar spine vertebrae using the European Spine Phantom together with the LDCT and the lumbar QCT scan is recommended. Second, clinical patient experiments were not conducted to confirm this phantom study. Finally, the acquisition parameters in this study were limited to a few variations of slice thickness, radiation dose, strength of the IR algorithms, and reconstruction kernels. Subsequent studies investigating various ranges of radiation dose, strength of IR algorithms, and different reconstruction kernels should be performed to complement the findings in the present study.
Conclusions
This phantom study demonstrated that the image quality of the CT scanners from 5 different manufacturers for LDCT scan was comparable, and the CT number linearity was unbiased, which contributed to the stability of the HU values and the accuracy of the BMD quantifications.
Acknowledgments
Funding: This study was supported by grants from the National Natural Science Foundation of China (no. U1504821).
Footnote
Conflicts of Interest: All authors have completed the ICMJE uniform disclosure form (available at https://dx.doi.org/10.21037/qims-21-245). The authors have no conflicts of interest to declare.
Ethical Statement: The authors are accountable for all aspects of the work in ensuring that questions related to the accuracy or integrity of any part of the work are appropriately investigated and resolved. Our study was a phantom study without including patient experiment or animal study, so we didn’t need ethics.
Open Access Statement: This is an Open Access article distributed in accordance with the Creative Commons Attribution-NonCommercial-NoDerivs 4.0 International License (CC BY-NC-ND 4.0), which permits the non-commercial replication and distribution of the article with the strict proviso that no changes or edits are made and the original work is properly cited (including links to both the formal publication through the relevant DOI and the license). See: https://creativecommons.org/licenses/by-nc-nd/4.0/.
References
- Chen P, Li Z, Hu Y. Prevalence of osteoporosis in China: a meta-analysis and systematic review. BMC Public Health 2016;16:1039. [Crossref] [PubMed]
- Xu XM, Li N, Li K, Li XY, Zhang P, Xuan YJ, Cheng XG. Discordance in diagnosis of osteoporosis by quantitative computed tomography and dual-energy X-ray absorptiometry in Chinese elderly men. J Orthop Translat 2019;18:59-64. [Crossref] [PubMed]
- Cheng X, Zhao K, Zha X, Du X, Li Y, Chen S, et al. Opportunistic Screening Using Low-Dose CT and the Prevalence of Osteoporosis in China: A Nationwide, Multicenter Study. J Bone Miner Res 2021;36:427-35. [Crossref] [PubMed]
- Uffmann M, Schaefer-Prokop C. Digital radiography: the balance between image quality and required radiation dose. Eur J Radiol 2009;72:202-8. [Crossref] [PubMed]
- Church TR, Black WC, Aberle DR, Berg CD, Clingan KL, Duan F, Fagerstrom RM, Gareen IF, Gierada DS, Jones GC, Mahon I, Marcus PM, Sicks JD, Jain A, Baum S. Results of initial low-dose computed tomographic screening for lung cancer. N Engl J Med 2013;368:1980-91. [Crossref] [PubMed]
- Yang W, Qian F, Teng J, Wang H, Manegold C, Pilz LR, Voigt W, Zhang Y, Ye J, Chen Q, Han B. Written on behalf of the AME Thoracic Surgery Collaborative Group. Community-based lung cancer screening with low-dose CT in China: Results of the baseline screening. Lung Cancer 2018;117:20-6. [Crossref] [PubMed]
- Albert JM. Radiation risk from CT: implications for cancer screening. AJR Am J Roentgenol 2013;201:W81-7. [Crossref] [PubMed]
- Aberle DR, Adams AM, Berg CD, Black WC, Clapp JD, Fagerstrom RM, Gareen IF, Gatsonis C, Marcus PM, Sicks JD. Reduced lung-cancer mortality with low-dose computed tomographic screening. N Engl J Med 2011;365:395-409. [Crossref] [PubMed]
- Wu Y, Jiang YJ, Han XL, Wang MY, Gao JB. Application of low-tube current with iterative model reconstruction on Philips Brilliance iCT Elite FHD in the accuracy of spinal QCT using a European spine phantom. Quant Imaging Med Surg 2018;8:32-38. [Crossref] [PubMed]
- Wu Y, Guo Z, Fu XX, Wu J, Gao JB, Zeng Q, Fu HH, Cheng XG. The study protocol for the China Health Big Data (China Biobank) project. Quant Imaging Med Surg 2019;9:1095-102. [Crossref] [PubMed]
- Srivastava M, Deal C. Osteoporosis in elderly: prevention and treatment. Clin Geriatr Med 2002;18:529-55. [Crossref] [PubMed]
- Smith KE, Whiting BR, Reiker GG, Commean PK, Sinacore DR, Prior FW. Assessment of technical and biological parameters of volumetric quantitative computed tomography of the foot: a phantom study. Osteoporos Int 2012;23:1977-85. [Crossref] [PubMed]
- Zhao Y, Li K, Duanmu Y, Wang L, Xu X, Zhang Y, Tang J, Zhang Y, Li Z, Hind K, Blake GM, Cheng X. Accuracy, Linearity and Precision of Spine QCT vBMD Phantom Measurements for Different Brands of CT Scanner: A Multicentre Study. J Clin Densitom 2021; Epub ahead of print. [Crossref] [PubMed]
- Catphan 500 and 600 Manual. Greenwish, NY: The Phantom Laboratory, Inc., 2006.
- Treb K, Li K. Accuracy of weighted CTDI in estimating average dose delivered to CTDI phantoms: An experimental study. Med Phys 2020;47:6484-99. [Crossref] [PubMed]
- Li X, Zhang D, Liu B. Calculations of two new dose metrics proposed by AAPM Task Group 111 using the measurements with standard CT dosimetry phantoms. Med Phys 2013;40:081914 [Crossref] [PubMed]
- Bujila R, Kull L, Danielsson M, Andersson J. Applying three different methods of measuring CTDIfree air to the extended CTDI formalism for wide-beam scanners (IEC 60601-2-44): A comparative study. J Appl Clin Med Phys 2018;19:281-9. [Crossref] [PubMed]
- McNitt-Gray MF. MO-A-ValB-01: Tradeoffs in Image Quality and Radiation Dose for CT. Med Phys 2006;33:2154-5. [Crossref]
- van Hamersvelt RW, Schilham AMR, Engelke K, den Harder AM, de Keizer B, Verhaar HJ, Leiner T, de Jong PA, Willemink MJ. Accuracy of bone mineral density quantification using dual-layer spectral detector CT: a phantom study. Eur Radiol 2017;27:4351-9. [Crossref] [PubMed]
- Ehman EC, Yu L, Manduca A, Hara AK, Shiung MM, Jondal D, Lake DS, Paden RG, Blezek DJ, Bruesewitz MR, McCollough CH, Hough DM, Fletcher JG. Methods for clinical evaluation of noise reduction techniques in abdominopelvic CT. Radiographics 2014;34:849-62. [Crossref] [PubMed]
- van Ommen F, Bennink E, Vlassenbroek A, Dankbaar JW, Schilham AMR, Viergever MA, de Jong HWAM. Image quality of conventional images of dual-layer SPECTRAL CT: A phantom study. Med Phys 2018;45:3031-42. [Crossref] [PubMed]
- Miéville FA, Gudinchet F, Brunelle F, Bochud FO, Verdun FR. Iterative reconstruction methods in two different MDCT scanners: physical metrics and 4-alternative forced-choice detectability experiments--a phantom approach. Phys Med 2013;29:99-110. [Crossref] [PubMed]
- Abdullah KA, McEntee MF, Reed WM, Kench PL. Increasing iterative reconstruction strength at low tube voltage in coronary CT angiography protocols using 3D-printed and Catphan® 500 phantoms. J Appl Clin Med Phys 2020;21:209-14. [Crossref] [PubMed]
- Shi QL, Zhao HM, Zhang L, Ma YG, Li XT, Cui Y, Sun YS, Zhang XP. Evaluation of dose reduction and image quality in abdominal CT using adaptive statistical iterative reconstruction in a phantom study. Chinese Journal of Medical Imaging 2013;4:326-9.
- International standard IEC 61223-3-5. Evaluation and routine testing in medical imaging departments - Part 3-5: Acceptance and constancy tests - Imaging performance of computed tomography X-ray equipment. Available online: https://standards.globalspec.com/std/13473617/IEC%2061223-3-5
- Gulliksrud K, Stokke C, Martinsen AC. How to measure CT image quality: variations in CT-numbers, uniformity and low contrast resolution for a CT quality assurance phantom. Phys Med 2014;30:521-6. [Crossref] [PubMed]
- Siewerdsen JH, Cunningham IA, Jaffray DA. A framework for noise-power spectrum analysis of multidimensional images. Med Phys 2002;29:2655-71. [Crossref] [PubMed]
- Brunner C, Renger B, Hoeschen C, Kyprianou I. Investigation of a method to estimate the MTF and NPS of CT towards creating an international standard. Proc. SPIE 7961, Medical Imaging 2011: Physics of Medical Imaging, 79613C (16 March 2011). Available online:
10.1117/12.877657 10.1117/12.877657 - Li G, Gao GX, Xia HL. Detection and influencing factors of CT spatial resolution and low-contrast resolution. China Medical Devices 2010;25:7-9.
- International standard IEC 61223-2-6. Evaluation and routine testing in medical imaging departments-part 2-6: acceptance tests-imaging performance of computed tomography X-ray equipment 2006. Available online: https://standards.globalspec.com/std/38077/IEC%2061223-2-6
- Suetens P. Fundamentals of medical imaging. 2td edition. New York: Cambridge University Press, 2009:49-59.
- Hui SK, Weir VJ, Brown K, Froelich J. Assessing the clinical utility of quantitative computed tomography with a routinely used diagnostic computed tomography scanner in a cancer center. J Clin Densitom 2011;14:41-6. [Crossref] [PubMed]
- Schwaiger BJ, Gersing AS, Baum T, Noël PB, Zimmer C, Bauer JS. Bone mineral density values derived from routine lumbar spine multidetector row CT predict osteoporotic vertebral fractures and screw loosening. AJNR Am J Neuroradiol 2014;35:1628-33. [Crossref] [PubMed]
- Lu Y, Engelke K, Püschel K, Morlock MM, Huber G. Influence of 3D QCT scan protocol on the QCT-based finite element models of human vertebral cancellous bone. Med Eng Phys 2014;36:1069-73. [Crossref] [PubMed]
- Willemink MJ, Noël PB. The evolution of image reconstruction for CT-from filtered back projection to artificial intelligence. Eur Radiol 2019;29:2185-95. [Crossref] [PubMed]
- Mileto A, Guimaraes LS, McCollough CH, Fletcher JG, Yu L. State of the Art in Abdominal CT: The Limits of Iterative Reconstruction Algorithms. Radiology 2019;293:491-503. [Crossref] [PubMed]
- Akagi M, Nakamura Y, Higaki T, Narita K, Honda Y, Zhou J, Yu Z, Akino N, Awai K. Correction to: Deep learning reconstruction improves image quality of abdominal ultra-high-resolution CT. Eur Radiol 2019;29:4526-7. [Crossref] [PubMed]
- Choi MK, Kim SM, Lim JK. Diagnostic efficacy of Hounsfield units in spine CT for the assessment of real bone mineral density of degenerative spine: correlation study between T-scores determined by DEXA scan and Hounsfield units from CT. Acta Neurochir (Wien) 2016;158:1421-7. [Crossref] [PubMed]
- Lee S, Chung CK, Oh SH, Park SB. Correlation between Bone Mineral Density Measured by Dual-Energy X-Ray Absorptiometry and Hounsfield Units Measu red by Diagnostic CT in Lumbar Spine. J Korean Neurosurg Soc 2013;54:384-9. [Crossref] [PubMed]
- Schreiber JJ, Anderson PA, Rosas HG, Buchholz AL, Au AG. Hounsfield units for assessing bone mineral density and strength: a tool for osteoporosis management. J Bone Joint Surg Am 2011;93:1057-63. [Crossref] [PubMed]
- Jeong DK, Lee SS, Kim JE, Huh KH, Yi WJ, Heo MS, Choi SC. Effects of energy level, reconstruction kernel, and tube rotation time on Hounsfield units of hydroxyapatite in virtual monochromatic images obtained with dual-energy CT. Imaging Sci Dent 2019;49:273-9. [Crossref] [PubMed]
- Wood TJ, Moore CS, Saunderson JR, Beavis AW. Measurement of effective detective quantum efficiency for a photon counting scanning mammography system and comparison with two flat panel full-field digital mammography systems. Phys Med Biol 2018;63:025025 [Crossref] [PubMed]
- Caballo M, Michielsen K, Fedon C, Sechopoulos I. Towards 4D dedicated breast CT perfusion imaging of cancer: development and validation of computer simulated images. Phys Med Biol 2019;64:245004 [Crossref] [PubMed]
- Higaki T, Nakamura Y, Zhou J, Yu Z, Nemoto T, Tatsugami F, Awai K. Deep Learning Reconstruction at CT: Phantom Study of the Image Characteristics. Acad Radiol 2020;27:82-7. [Crossref] [PubMed]
- Plautz TE, Bashkirov V, Giacometti V, Hurley RF, Johnson RP, Piersimoni P, Sadrozinski HF, Schulte RW, Zatserklyaniy A. An evaluation of spatial resolution of a prototype proton CT scanner. Med Phys 2016;43:6291. [Crossref] [PubMed]
- Kim HG, Chung YE, Lee YH, Choi JY, Park MS, Kim MJ, Kim KW. Quantitative analysis of the effect of iterative reconstruction using a phantom: determining the appropriate blending percentage. Yonsei Med J 2015;56:253-61. [Crossref] [PubMed]
- Brenner DJ, Hall EJ. Computed tomography--an increasing source of radiation exposure. N Engl J Med 2007;357:2277-84. [Crossref] [PubMed]
- Prakash P, Kalra MK, Kambadakone AK, Pien H, Hsieh J, Blake MA, Sahani DV. Reducing abdominal CT radiation dose with adaptive statistical iterative reconstruction technique. Invest Radiol 2010;45:202-10. [Crossref] [PubMed]
- May MS, Wüst W, Brand M, Stahl C, Allmendinger T, Schmidt B, Uder M, Lell MM. Dose reduction in abdominal computed tomography: intraindividual comparison of image quality of full-dose standard and half-dose iterative reconstructions with dual-source computed tomography. Invest Radiol 2011;46:465-70. [Crossref] [PubMed]
- Boedeker KL, McNitt-Gray MF. Application of the noise power spectrum in modern diagnostic MDCT: part II. Noise power spectra and signal to noise. Phys Med Biol 2007;52:4047-61. [Crossref] [PubMed]
- Hobson MA. Erratum: Using the ACR CT accreditation phantom for routine image quality assurance on both CT and CBCT imaging systems in a radiotherapy environment. J Appl Clin Med Phys 2014;15:5228. [Crossref] [PubMed]
- Miura H, Ozawa S, Okazue T, Kawakubo A, Yamada K, Nagata Y. Image quality and absorbed dose comparison of single- and dual-source cone-beam computed tomography. J Appl Clin Med Phys 2018;19:360-6. [Crossref] [PubMed]
- Nguyen HS, Shabani S, Patel M, Maiman D. Posterolateral lumbar fusion: Relationship between computed tomography Hounsfield units and symptomatic pseudoarthrosis. Surg Neurol Int 2015;6:S611-4. [Crossref] [PubMed]
- Nishio M, Matsumoto S, Seki S, Koyama H, Ohno Y, Fujisawa Y, Sugihara N, Yoshikawa T, Sugimura K. Emphysema quantification on low-dose CT using percentage of low-attenuation volume and size distribution of low-attenuation lung regions: effects of adaptive iterative dose reduction using 3D processing. Eur J Radiol 2014;83:2268-76. [Crossref] [PubMed]
- Ichikawa K, Kobayashi T, Sagawa M, Katagiri A, Uno Y, Nishioka R, Matsuyama J. A phantom study investigating the relationship between ground-glass opacity visibility and physical detectability index in low-dose chest computed tomography. J Appl Clin Med Phys 2015;16:202-215. [Crossref] [PubMed]
- Hsieh J, Liu E, Nett B, Tang J, Thibault JB, Sahney S. A new era of image reconstruction: TrueFidelity Technical white paper on deep learning image reconstruction. GE Healthcare, 2019. Available online: https://www.gehealthcare.com/-/jssmedia/040dd213fa89463287155151fdb01922.pdf