Long-term prognostic value of the serial changes of CT-derived fractional flow reserve and perivascular fat attenuation index
Introduction
Coronary computed tomography angiography (CCTA) is a useful imaging modality for noninvasive diagnosis of obstructive coronary artery disease (CAD) (1). In addition to the evaluation of stenotic extent, CCTA also plays an important role in the assessment of high-risk plaque features, which predict unfavorable clinical outcomes (2-4). Moreover, the fat attenuation index (FAI) has been recently introduced as a novel imaging biomarker for in vivo evaluation of coronary vascular inflammation, and a higher FAI is associated with increased risks of a major adverse cardiac event (MACE) (5,6). According to one previous study, statin treatment is able to reduce the lesion-specific FAI for noncalcified and mixed plaques, possibly due to its anti-inflammatory effect (7).
In contrast to plaque analysis, machine learning (ML)-based computed tomography (CT) fractional flow reserve (CT-FFR) is an accurate approach for diagnosing hemodynamically significant coronary stenosis (8-11). For CAD patients treated with optimal medical treatment (OMT), lesion-specific CT-FFR has reportedly also been improved at follow-up (12).
According to previous studies, OMT has a potential impact on the dynamic changes of both the FAI and CT-FFR. However, the relationship between clinical outcome and the serial changes of CT-FFR and FAI together as prognostic indicators remains unclear. In light of the above results, we hypothesized that the dynamic changes of both FAI and CT-FFR might reflect the plaque response to OMT and may possibly be associated with prognosis. Therefore, we aimed to investigate the serial changes of ML-based CT-FFR and perivascular FAI after OMT and their relationships with midterm clinical outcomes.
We present the following article in accordance with the TRIPOD reporting checklist (available at https://dx.doi.org/10.21037/qims-21-424).
Methods
Patient population
Between April 2009 and September 2016, consecutive patients with stable angina or atypical chest pain were referred for CCTA to rule out obstructive disease in 3 tertiary hospitals. The patients were prospectively enrolled for follow-up of the serial change of CT plaque features if they met the following inclusion criteria: (I) the pretest probability of obstructive CAD was intermediate according to updated Diamond-Forrester score (defined as pretest probability between 15% and 85%), (II) baseline CCTA revealed at least 1 lesion with a diameter stenosis (DS) of 30–70% on major epicardial arteries (diameter ≥2 mm), and (III) patients were referred for OMT. All included patients were referred for a second CCTA at 1 to 1.5-year intervals to monitor the serial imaging changes of atherosclerotic plaques and were followed up until September 2019 after the second CCTA. The exclusion criteria were as follows: (I) image quality of baseline or follow-up CCTA was severely impaired (severe artifact, nondiagnostic), (II) patients experienced MACEs prior to the follow-up CCTA, (III) patients refused to undergo a follow-up CCTA, and (IV) patients were lost to follow-up (Figure 1). This study was conducted in accordance with the Declaration of Helsinki (as revised in 2013), and the research protocol was approved by all local hospital ethics committees (approval number: 2009-023). All patients gave informed consent. In the present study, we performed a post hoc analysis of this prospective cohort regarding the serial changes of ML-based CT-FFR and perivascular FAI.
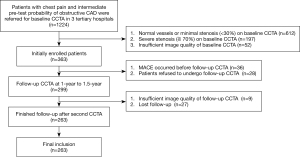
CT acquisition and reconstruction
Three models of CT scanners, including the 128-slice multidetector CT (Definition AS+, Siemens Healthineers, Erlagen, Germany), the second-generation dual source CT (SOMATOM Flash, Siemens Healthineers), and the 256-slice CT scanner (Brilliance iCT, Philips Healthcare, Amsterdam, The Netherlands), were used for CCTA imaging. A beta-blocker (25–50 mg, Betaloc ZOK; AstraZeneca, Cambridge, UK) was administered orally 1 hour prior to the examination in patients with a baseline heart rate ≥70 bpm and scanned using the 128-slice multidetector CT and 256-slice CT scanner. For patients scanned using dual source CT, a beta-blocker was not used. Nitroglycerin (Shanghai Shyndec Pharmaceutical Co., Ltd, China) was given sublingually in all patients from 3 sites. Prospective electrocardiogram (ECG)-triggered sequential acquisition was used in all patients with the triggering window covering from the end-systolic to mid-diastolic phase (from 35% to 75% of the R-R interval). Details regarding the acquisition and reconstruction parameters are shown in the online appendix. The same acquisition parameters were used for both baseline and follow-up CCTA in each individual.
CT-based plaque and FAI analysis
CCTA data with best image quality from all available phases were transferred to the core lab, and all lesions with ≥30% DS on major epicardial arteries (diameter ≥ 2mm) were included for further analysis. Conventional qualitative and quantitative plaque parameters were evaluated using a dedicated plaque analysis software (Coronary Plaque Analysis, version 4.3, Siemens Healthineers). The following indices were measured and recorded: (I) DS, (II) remodeling index and the presence of positive remodeling (13), (III) low-attenuation plaque (LAP) (13), (IV) spotty calcification (13), and (V) napkin-ring sign as defined by a previous study (14). Detailed definitions of the above parameters are provided in the online appendix. The patient-based stenosis extent was evaluated using the Coronary Artery Disease-Reporting and Data System (CAD-RADS) (15). Lesions with at least 2 high-risk plaque features (positive remodeling, LAP, spotty calcification, and napkin-ring sign) were deemed high-risk plaques, and patients with at least 1 high-risk plaque were considered to have vulnerable plaques (15).
Lesion-based perivascular FAI was measured for all of the above plaques. A dedicated FAI analysis software (Coronary FAI Analysis, version 1.0.2, Siemens Healthineers) was used for quantification. Briefly, perivascular adipose tissue was sampled radially outward from the outer vessel wall of the plaques and measured as voxels with an attenuation between −190 and −30 HU. FAI was defined as the mean CT attenuation of adipose tissue, which was within a radial distance from the outer vessel wall equal to the diameter of the target vessel (5,6). The detailed steps of FAI analysis are provided in the online appendix. The difference between the follow-up and baseline FAI values was recorded as △FAI. A recent study revealed that vessel locations might intrinsically impact the measured values of FAI (16). Therefore, rather than using the lesion with highest baseline FAI value, we measured the FAI of lesions with the most significant hemodynamic status (lowest CT-FFR value) in the patient-based analysis.
All the above parameters were independently analyzed by 2 cardiovascular radiologists (with 12 years and 4 years of experience in cardiac imaging), and the mean values of measurement were used for further analysis.
CT-FFR measurement
Lesion-specific CT-FFR values were measured in the core lab for all stenoses with DS ≥30%. The current study used a ML-based approach for CT-FFR simulation (cFFR, version 3.0, Siemens Healthineers). Briefly, this model was trained on a large database of synthesized coronary anatomies, where the reference values are computed using a computational fluid dynamics-based model (17). The diagnostic performance of this algorithm has been validated by previous studies with reference to invasive FFR (8,9). The details regarding how this ML-based model was trained and how onsite processing was performed are provided in the online appendix. The lesion-specific CT-FFR values were measured at 1–2 cm distal to the lesion. The difference between follow-up and baseline CT-FFR values was recorded as △CT-FFR. The lesions with the lowest baseline CT-FFR values were used in subsequent patient-based analyses.
Two cardiovascular radiologists (with 12 years and 4 years of experience of cardiac imaging) independently calculated the CT-FFR values of all targeting lesions, and the mean values were used for further analysis.
Clinical follow-up and study end points
All enrolled patients were referred for OMT according to their baseline clinical characteristics, including hypertension, diabetes, and hyperlipidemia. Clinical follow-up was conducted after the second CCTA until September 2019 via outpatient clinic visits. The radiologists were blinded to the clinical outcome. The primary end point of the present study was the association between serial changes of CT-FFR, FAI, and MACEs. An MACE was defined as all-cause mortality, myocardial infarction, revascularization, and rehospitalization due to heart failure or aggravated angina symptoms.
Statistical analysis
Overall, <5% of observations were missing, and continuous variables were imputed using the corresponding mean value. The serial changes in study parameters were calculated as absolute differences between baseline and follow-up. The 1-sample Kolmogorov-Smirnov test was used to check the assumption of normal distribution. Quantitative variables with normal distribution are expressed as mean ± standard deviation; otherwise, medians and quartiles are used. Categorical variables are reported as counts and percentages and were compared using Fisher’s exact test or the chi-square test according to the data cell size. The paired t test was used for normally distributed data, and the Wilcoxon signed-rank test was used for nonnormally distributed data. The average measures for interobserver reliability are expressed as an intraclass correlation coefficient (ICC) for continuous variables and Cohen’s kappa coefficient for categorical variables.
Univariable and multivariable Cox proportional hazards models were used to determine the study parameters associated with MACEs. The Kaplan-Meier curve was used for the proportional hazards (PH) assumption test for binary variables and Schoenfeld residuals test for continuous variables. Stratified Cox regression was used when the variables did not meet the assumptions of the model PH assumption. The optimum model candidates were selected based on the significant variables in the univariable analysis (P<0.05). A clinical and an imaging model was created to avoid overfitting. Nested models were used to assess the incremental value of change of FAI and change of FFR in 3 modeling steps. The first step consisted of fitting a multivariable model of traditional risk factors, the second step included the change of FAI, and the third step included the change of FFR. The change in overall log-likelihood ratio was used to assess the increase in predictive power. The time-dependent receiver operating characteristics curve (ROC) and Harrell’s Concordance statistic were used to evaluate the discriminatory power of the models. The calibration curve was plotted using the model-predicted probability against the actual probability of the optimum model to evaluate the reliability with 500 bootstrap resamples.
Statistical analysis was performed using standard statistical software packages (SPSS software version 23.0, IBM Corp., Armonk, NY, USA; and R software version 3.6.3, R Foundation for Statistical Computing, Vienna, Austria), and statistical significance was defined as a 2-tailed P value <0.05.
Results
Patient characteristics and clinical outcomes
Between April 2009 and September 2016, 1,224 patients with chest pain and suspected of CAD were screened for potential inclusion, and 363 patients met the inclusion criteria. After initial enrollment, 100 patients were excluded due to various reasons (details shown in Figure 1). CT-FFR simulation was successfully performed in 95.8% (1,172/1,224) of patients at baseline and 97% (290/299) patients in follow-up. Finally, 263 patients were included in the study. The mean cumulated effective dose for 2 CCTA scans was 6.58±1.63 mSv. The mean analyzing times for CT-FFR and FAI were 10.1±3.6 and 13.3±1.9 min, respectively. The CT scan parameters of the baseline and follow-up examinations were identical. Detailed demographic data are shown in Table 1. Good intra- and interobserver agreement was found for measurements of all parameters (Tables S1,S2).
Table 1
Characteristics | N=263 |
---|---|
Male, n (%) | 186 (70.7) |
Age, years | 64.8±11.0 |
Body mass index, kg/m2 | 24.76±3.58 |
Risk factors, n (%) | |
Hypertension | 155 (58.9) |
Diabetes mellitus | 82 (31.2) |
Dyslipidemia | 109 (41.4) |
Smoking | 71 (27.0) |
Angina, n (%) | |
CCS class I | 133 (50.6) |
CCS class II | 84 (31.9) |
CCS class III | 46 (17.5) |
CACS | 62.0 (5.0-272.1) |
Tube voltage, n (%) | |
100 kVp | 36 (13.7) |
120 kVp | 218 (82.9) |
140 kVp | 9 (3.4) |
CACS, coronary artery calcium score; CCS, Canadian Cardiovascular Society.
During follow-up {median, 42 months [interquartile range (IQR): 36–54 months]}, MACEs occurred in 38 patients (14.4%), including 1 case of sudden cardiac death, 2 cases of acute myocardial infarction, 20 cases of revascularization, and 15 cases of rehospitalization due to heart failure or aggravated angina symptoms.
Serial change of imaging parameters between baseline and follow-up CCTA
In the MACE group, lesion-specific CT-FFR decreased significantly at follow-up CCTA [0.80 (0.74–0.90) versus 0.85 (0.76–0.93); P=0.01], whereas FAI did not notably increase (–70.4±8.9 versus –71.3±7.1 HU; P=0.436; Figure 2). In the non-MACE group, lesion-specific CT-FFR increased markedly [0.91 (0.84–0.95) versus 0.90 (0.82–0.94); P<0.001], while FAI markedly decreased (–74.0±10.8 HU versus –72.4±11.5 HU; P=0.004; Figure 3). Moreover, the plaque volume of noncalcified components was noted as being significantly reduced in the non-MACE group, while a significant difference was observed between the 2 groups in terms of coronary artery calcium score (CACS), minimal lumen area (MLA), and the plaque volume of calcified components between baseline and follow-up CCTA (details are shown in Table 2).
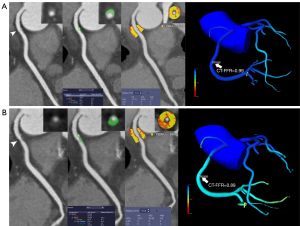
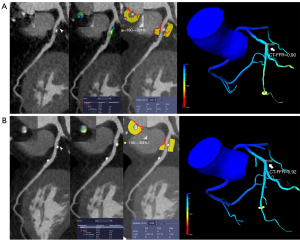
Table 2
MACE after follow-up CCTA (n=38) | P value | MACE free after follow-up CCTA (n=225) | P value | |||
---|---|---|---|---|---|---|
Baseline | Follow-up | Baseline | Follow-up | |||
CAD-RADS (%) | ||||||
CAD-RADS 1 | 0 (0) | 0 (0) | – | 0 (0) | 20 (8.9) | <0.001 |
CAD-RADS 2 | 14 (36.8) | 10 (26.3) | 0.324 | 127 (56.4) | 104 (46.2) | 0.258 |
CAD-RADS 3 | 24 (63.2) | 24 (63.2) | 1.000 | 98 (43.6) | 77 (34.2) | 0.042 |
CAD-RADS 4A | 0 (0) | 4 (10.5) | 0.058 | 0 (0) | 24 (10.7) | <0.001 |
CACS | 74.05 (9.2–258.9) | 129.3 (24.6–258.9) | <0.001 | 59.4 (4.9–285.5) | 90.6 (10.1–350.0) | <0.001 |
MLA (mm2) | 3.33±2.28 | 2.89±1.97 | 0.013 | 4.27±2.20 | 4.47±2.30 | 0.015 |
High-risk plaque features (%) | ||||||
Napkin-ring sign | 5 (13.1) | 3 (7.9) | 0.356 | 36 (16.0) | 21 (9.3) | 0.046 |
LAP | 0 (0) | 1 (2.6) | 1.000 | 4 (1.8) | 3 (1.3) | 1.000 |
Spotty calcification | 11 (28.9) | 10 (26.3) | 0.798 | 26 (11.6) | 20 (8.9) | 0.350 |
Positive remodeling | 5 (13.2) | 7 (18.4) | 0.529 | 32 (14.2) | 22 (9.8) | 0.147 |
Total plaque volume (mm3) | 67.0 (46.9–98.1) | 76.5(49.7–104.0) | 0.708 | 63.4 (39.3–112.5) | 65.3 (38.1–122.5) | 0.240 |
Noncalcified component volume (mm3) | 45.2 (0.0–63.6) | 34.0(0.0–71.2) | 0.332 | 39.8 (0.0–72.8) | 33.4 (0.0–71.9) | 0.007 |
LAP volume (mm3) | 0.54 (0.00–1.69) | 0.12 (0.00–1.12) | 0.578 | 0.54 (0.00–1.68) | 0.10 (0.00–0.92) | 0.198 |
Calcified component volume (mm3) | 11.5 (0.0–43.9) | 20.0 (0.0–50.9) | 0.011 | 10.1 (0.0–44.4) | 14.4 (0.0–58.4) | <0.001 |
CT-FFR | 0.85 (0.76-0.93) | 0.80 (0.74–0.90) | 0.010 | 0.90 (0.82–0.94) | 0.91 (0.84–0.95) | <0.001 |
FAI (HU) | −71.3±7.1 | −70.4±8.9 | 0.436 | −72.4±11.5 | −74.0±10.8 | 0.004 |
CACS, coronary artery calcium score; CAD-RADS, Coronary Artery Disease-Reporting and Data System; CCTA, coronary computed tomography angiography; CT-FFR, computed tomography fractional flow reserve; FAI, fat attenuation index; LAP, low attenuation plaque; MACE, major adverse cardiac event; MLA, minimal lumen area.
Prognostic value of baseline and serial changes of various parameters
According to the univariable Cox regression analysis, both the baseline [hazard ratio (HR): 1.039, 95% confidence interval (CI): 1.012–1.067; P=0.004] and serial changes of CT-FFR (HR: 1.070, 95% CI: 1.033–1.107; P<0.001) were associated with MACEs. The Kaplan-Meier curve showed that the predictive value of decreased CT-FFR for MACEs was significantly different before (HR: 1.000, 95% CI: 0.322–3.100; P=0.999) and after (HR: 5.520, 95% CI: 1.167–26.14; P=0.031) the 15th month postsecond CCTA scan (Figure 4, Table 3). This phenomenon was also most prominent in the noncalcified lesion subgroup (Figure 4). Similarly, increased FAI, presence of high-risk plaques, decreased MLA, and several baseline clinical characteristics were also associated with the occurrence of MACEs (Table 3). In contrast to the above findings, it is worth noting that the baseline FAI and CACS were not predictors of MACEs (Table 3). According to the ROC analysis, the serial changes of CT-FFR and FAI outperformed the baseline CT-FFR [area under curve (AUC) for serial changes of CT-FFR =0.678 vs. AUC for baseline CT-FFR =0.635; P=0.004] and FAI (AUC for serial changes of FAI =0.651 versus AUC for baseline FAI =0.522; P=0.047) for identifying patients with MACEs.
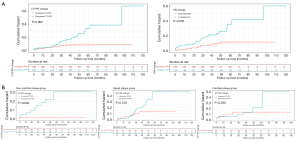
Table 3
HR | 95% CI | P value | |
---|---|---|---|
Baseline characteristics | |||
Gender | 0.973 | 0.481–1.966 | 0.939 |
Age | 0.996 | 0.967–1.026 | 0.775 |
Risk factors | |||
Hypertension | 2.169 | 1.040–4.522 | 0.039 |
Diabetes mellitus | 1.033 | 0.527–2.026 | 0.925 |
Dyslipidemia | 2.051 | 1.075–3.912 | 0.029 |
Smoking | 0.967 | 0.467–1.999 | 0.927 |
CACS | 0.895 | 0.733–1.094 | 0.278 |
CCS angina type | 2.294 | 1.635–3.218 | <0.001 |
Diamond-Forrester score | 2.388 | 1.251–4.560 | 0.008 |
CAD-RADS | 1.787 | 0.935–3.415 | 0.079 |
CT-FFR | 1.039 | 1.012–1.067 | 0.004 |
FAI | 0.860 | 0.613–1.206 | 0.382 |
High-risk plaque* | 2.175 | 1.026–4.610 | 0.037 |
Serial change | |||
MLA, per 1 mm2 increase | 0.633 | 0.492–0.815 | <0.001 |
Total plaque volume, per 1 mm3 increase | 1.000 | 0.991–1.009 | 0.999 |
Noncalcified component volume, per 1 mm3 increase | 1.003 | 0.993–1.014 | 0.514 |
Calcified component volume, per 1 mm3 increase | 0.989 | 0.969–1.009 | 0.278 |
LAP volume, per 1 mm3 increase | 1.007 | 0.912–1.112 | 0.891 |
CT-FFR | |||
Per 0.01 decrease | 1.070 | 1.033–1.107 | <0.001 |
Decreased CT-FFR | 2.912 | 1.413–5.999 | 0.003 |
Before 15th month after second CCTA | 1.000 | 0.322–3.100 | 0.999 |
15th month to end | 5.520 | 1.167–26.14 | 0.031 |
FAI | |||
FAI, per 10 HU increase | 1.581 | 0.987–2.531 | 0.057 |
Increased FAI | 3.185 | 1.616–6.279 | 0.001 |
*, patients with presence of at least 2 high-risk plaque features. CACS, coronary artery calcium score; CAD-RADS, Coronary Artery Disease-Reporting and Data System; CCS, Canadian Cardiovascular Society; CCTA, coronary computed tomography angiography; CI, confidence interval; CT-FFR, computed tomography fractional flow reserve; FAI, fat attenuation index; HR, hazard ratio; LAP, low attenuation plaque; MACE, major adverse cardiac event; MLA, minimal lumen area.
Although multivariable models were limited by the sparse number of events, the significant associations between decreased FFR, increased FAI, and MACEs were still observed even after adjustment for baseline hypertension, dyslipidemia, angina types, presence of high-risk plaque features, serial change of MLA, and noncalcified plaque volume (Table 4). Furthermore, decreased CT-FFR (adjusted overall HR =2.455; P=0.023) and increased FAI (adjusted HR =2.956, P=0.002) were 2 strong independent predictors of MACEs after adjustment for conventional clinical and imaging parameters (Table S3).
Table 4
Characteristics | HR | 95% CI | P value |
---|---|---|---|
Clinical model | |||
Hypertension | 2.991 | 1.138–6.477 | 0.005 |
Dyslipidemia | 1.460 | 0.758–2.816 | 0.258 |
CCS angina type | 1.903 | 1.350–2.683 | 0.001 |
Decreased CT-FFR | 3.172 | 1.524–6.604 | 0.002 |
Before 15th month after second CCTA | 1.125 | 0.361–3.508 | 0.839 |
15th month to end | 5.576 | 1.178–26.407 | 0.030 |
Increased FAI | 3.189 | 1.594–6.381 | 0.001 |
Imaging model | |||
Decreased MLA | 0.710 | 0.539–0.935 | 0.015 |
Noncalcified plaque | 0.993 | 0.983–1.003 | 0.148 |
High-risk plaque* | 2.900 | 1.347–6.242 | 0.007 |
Decreased CT-FFR | 2.647 | 1.195–5.863 | 0.016 |
Before 15th month after second CCTA | 0.924 | 0.281–3.039 | 0.897 |
15th month to end | 5.610 | 1.184–26.583 | 0.030 |
Increased FAI | 2.819 | 1.384–5.740 | 0.004 |
*, patients with presence of at least 2 high-risk plaque features. CACS, coronary artery calcium score; CAD-RADS, Coronary Artery Disease-Reporting and Data System; CCS, Canadian Cardiovascular Society; CCTA, coronary computed tomography angiography; CI, confidence interval; CT-FFR, computed tomography fractional flow reserve; FAI, fat attenuation index; HR, hazard ratio; LAP, low attenuation plaque; MACE, major adverse cardiac event; MLA, minimal lumen area.
Discriminatory capacity of serial changes of CT-FFR and FAI to predict events
The MACE-discrimination capacity of the traditional risk factors (model 1), combination with increased FAI (model 2), and combination with increased FAI and decreased CT-FFR (model 3), as measured by the C-statistic, were 0.673 (95% CI: 0.664–0.682), 0.716 (95% CI: 0.707–0.725), and 0.762 (95% CI: 0.753–0.771), respectively. The clinical variable-based model was significantly improved by the addition of increased FAI, and further enhanced by adding decreased CT-FFR (P=0.003 and P=0.004, respectively; Table 5 and Table S4). The calibration plot is presented in Figure 5.
Table 5
C-statistic | 95% CI | P value | |
---|---|---|---|
Model 1 | 0.673 | 0.585–0.761 | – |
Model 2 | 0.716 | 0.624–0.812 | 0.019* |
Model 3 | 0.762 | 0.676–0.848 | 0.004# |
Model 1 was adjusted for age, gender, hypertension (HTN), series change of MLA, and high-risk plaque. Model 2 was adjusted for HTN, series change of MLA, high-risk plaque, and FAI change. Model 3 was adjusted for the same variables as model 2 as well as FFR change. *, P stands for the comparison of models 1 and 2. #, P stands for the comparison of models 2 and 3. CI, confidence interval; CT-FFR, computed tomography fractional flow reserve; FAI, fat attenuation index; MACE, major adverse cardiac event; MLA, minimal lumen area.
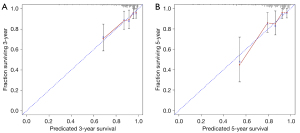
Discussion
The major finding of the present study showed that decreased CT-FFR and increased FAI at follow-up CCTA were the 2 strongest predictors of MACEs (among all parameters) in long-term follow-up. In addition, serial changes of CT-FFR and FAI provided incremental prognostic value over conventional clinical and imaging parameters for risk stratification.
CT-FFR has been validated as an accurate approach for noninvasive evaluation of the hemodynamic status of coronary stenosis (8,9), and the CT-FFR value is also thought to have predictive value for MACEs (18,19). However, these previous studies only focused on the baseline result of CT-FFR; the value of the dynamic changes of CT-FFR after OMT has not been explored. Given the fact the lesion-specific CT-FFR might change during follow-up (12), it is of clinical interest to investigate whether or not this serial change of CT-FFR is related to patient prognosis. According to the present findings, CT-FFR decrease at follow-up CCTA was a strong independent predictor of MACE occurrence. △CT-FFR represents the serial change of hemodynamically significant coronary stenosis after medical treatment. In cases with CT-FFR decrease at follow-up, lesion progression (in terms of its hemodynamic significance) is considered to be present. Under these circumstances, aggravated angina symptoms and late revascularization are more likely to occur. Therefore, it is expected that CT-FFR decrease has a significant value in predicting for MACEs.
In addition, it is also important to note that the predictive value of △CT-FFR was not evident at early follow-up, and was predominantly observed after 15 months. This could potentially be attributed to gradual lesion progression (in terms of its hemodynamic significance). In contrast to acute myocardial infarction caused by the rupture of vulnerable plaques, late revascularization is usually associated with the progression from non-flow-limiting lesions to flow-limiting lesions. The latter is a relatively long-standing progress and its impact is conceivably more evident over a longer follow-up period, as reflected by CT-FFR decrease. Furthermore, a small number of MACEs might have influenced the statistical results and led to nonsignificant findings in terms of the predictive value of △CT-FFR at early follow-up. Moreover, the predictive value of △CT-FFR was found to be mostly prominent in the noncalcified lesion subgroup. One recent study has demonstrated that the serial change of CT-FFR was more likely to be present in noncalcified plaques after statin treatment (12). The results of the present study complement this previous finding, as we further confirmed the prognostic value of the serial change of CT-FFR, especially in the noncalcified lesion subgroup.
Similar to △CT-FFR, lesion-specific FAI was also observed to change over time. In the current cohort, FAI increase at follow-up was a strong independent predictor of MACEs. This finding can be explained by the potential progression of perivascular inflammation caused by vulnerable plaques, which are prone to rupture and lead to acute coronary syndrome. Under these circumstances, the paracrine inflammatory signals from the vessel walls of vulnerable plaques may prevent lipid accumulation, which gives rise to increased perivascular FAI (20). The previous CRISP-CT (Cardiovascular RISk Prediction using Computed Tomography study) study confirmed the prognostic value of baseline FAI as increased FAI is associated with unfavorable clinical outcomes (6). However, a recent study showed that FAI value could be affected by different tube voltage settings (16), which might limit the value of an isolated interpretation of baseline FAI. In the present study, the baseline and follow-up FAI values were calculated using the CCTA data acquired with identical parameters. Thus, the △FAI reflected the real alteration of pericoronary adipose tissue density with elimination of the impact of extrinsic technical factors. Moreover, the △FAI was a strong independent predictor of MACEs, while the absolute baseline FAI was not. This could also be attributed to the varying range of FAI caused by different tube voltage settings.
The potential clinical implications of the present study are outlined below. First, as shown in the current study, △CT-FFR and △FAI outperformed baseline CT-FFR and FAI in the prediction of MACEs. Serial changes of CT-FFR and FAI offer novel ways to evaluate the risk of MACEs in patients with intermediate pretest probability of obstructive CAD. Although previous studies have addressed the prognostic value of CT-FFR and FAI (6,19), those values only represent the baseline status of hemodynamic significance and perivascular inflammation. OMT is usually given to patients and may lead to dynamic changes of CT-FFR and FAI (7,12). Thus, it is thought to be of greater clinical importance to associate clinical outcomes with the medical treatment response, which can be reflected by the serial changes of CT-FFR and FAI. Second, the serial changes of CT-FFR and FAI provide incremental values over conventional risk factors for prognosis evaluation. The model integrating clinical characteristics, conventional imaging features, and serial changes of CT-FFR and FAI had the highest performance in predicting the composite end point. In light of this finding, follow-up CCTA might be warranted to monitor the dynamic changes of CT-FFR and FAI and therefore guide the treatment strategy according to the follow-up imaging results.
Despite these promising results, this study has several limitations that should be noted. First, the current cohort was restricted to symptomatic patients with an intermediate pretest probability of obstructive CAD, in whom CCTA revealed 30–70% DS on at least 1 major epicardial vessel. Low- or intermediate-risk patients without ≥30% DS on major epicardial vessels were not included. In addition, compared to a previous study, the image quality of patients with chronic chest pain was superior to those with acute chest pain (21). The success rate of CT-FFR simulation is different between patients with chronic and acute chest pain. Thus, the present findings cannot be applied to the entire population in the clinical practice of cardiology. Thirdly, the small sample size and number of MACEs in this study also limit the interpretability of the prognostic value of △CT-FFR and △FAI in clinical practice. Finally, the current research was an observational study and not an interventional one. Thus, the optimal treatment strategy in patients with decreased CT-FFR and increased FAI remains unclear and requires further investigation in future studies.
In conclusion, decreased CT-FFR and increased FAI at follow-up CCTA were the 2 strongest predictors of MACEs. Serial changes of CT-FFR and FAI may provide incremental prognostic value over conventional clinical and imaging parameters for risk stratification.
Acknowledgments
Funding: This study was supported by Shanghai Committee of Science and Technology, China (no. 21ZR1452200), the Shanghai Municipal Education Commission-Gaofeng Clinical Medicine Grant Support (no: 20161428), and the Medical Guidance Scientific Research Support Project of Shanghai Science and Technology Commission (no. 19411965100).
Footnote
Reporting Checklist: The authors have completed the TRIPOD reporting checklist. Available at https://dx.doi.org/10.21037/qims-21-424
Conflicts of Interest: All authors have completed the ICMJE uniform disclosure form (available at https://dx.doi.org/10.21037/qims-21-424). Dr. JZ reported grant support by the Shanghai Committee of Science and Technology, China (no. 21ZR1452200), the Shanghai Municipal Education Commission-Gaofeng Clinical Medicine Grant Support (no. 20161428), and the Medical Guidance Scientific Research Support Project of Shanghai Science and Technology Commission (no. 19411965100). The other authors have no conflicts of interest to declare.
Ethical Statement: The authors are accountable for all aspects of the work in ensuring that questions related to the accuracy or integrity of any part of the work are appropriately investigated and resolved. This study was conducted in accordance with the Declaration of Helsinki (as revised in 2013). The research protocol was approved by all local hospital ethics committees (approval number: 2009-023), and all patients gave informed consent.
Open Access Statement: This is an Open Access article distributed in accordance with the Creative Commons Attribution-NonCommercial-NoDerivs 4.0 International License (CC BY-NC-ND 4.0), which permits the non-commercial replication and distribution of the article with the strict proviso that no changes or edits are made and the original work is properly cited (including links to both the formal publication through the relevant DOI and the license). See: https://creativecommons.org/licenses/by-nc-nd/4.0/.
References
- Yu M, Zhao Y, Li W, Lu Z, Wei M, Zhou W, Zhang J. Relationship of the Duke jeopardy score combined with minimal lumen diameter as assessed by computed tomography angiography to the hemodynamic relevance of coronary artery stenosis. J Cardiovasc Comput Tomogr 2018;12:247-54. [Crossref] [PubMed]
- Yu M, Li W, Lu Z, Wei M, Yan J, Zhang J. Quantitative baseline CT plaque characterization of unrevascularized non-culprit intermediate coronary stenosis predicts lesion volume progression and long-term prognosis: A serial CT follow-up study. Int J Cardiol 2018;264:181-6. [Crossref] [PubMed]
- Yu M, Lu Z, Li W, Wei M, Yan J, Zhang J. Coronary plaque characteristics on baseline CT predict the need for late revascularization in symptomatic patients after percutaneous intervention. Eur Radiol 2018;28:3441-53. [Crossref] [PubMed]
- Yuan M, Wu H, Li R, Yu M, Dai X, Zhang J. The value of quantified plaque analysis by dual-source coronary CT angiography to detect vulnerable plaques: a comparison study with intravascular ultrasound. Quant Imaging Med Surg 2020;10:668-77. [Crossref] [PubMed]
- Antonopoulos AS, Sanna F, Sabharwal N, Thomas S, Oikonomou EK, Herdman L, et al. Detecting human coronary inflammation by imaging perivascular fat. Sci Transl Med 2017;9:eaal2658 [Crossref] [PubMed]
- Oikonomou EK, Marwan M, Desai MY, Mancio J, Alashi A, Hutt Centeno E, Thomas S, Herdman L, Kotanidis CP, Thomas KE, Griffin BP, Flamm SD, Antonopoulos AS, Shirodaria C, Sabharwal N, Deanfield J, Neubauer S, Hopewell JC, Channon KM, Achenbach S, Antoniades C. Non-invasive detection of coronary inflammation using computed tomography and prediction of residual cardiovascular risk (the CRISP CT study): a post-hoc analysis of prospective outcome data. Lancet 2018;392:929-39. [Crossref] [PubMed]
- Dai X, Yu L, Lu Z, Shen C, Tao X, Zhang J. Serial change of perivascular fat attenuation index after statin treatment: Insights from a coronary CT angiography follow-up study. Int J Cardiol 2020;319:144-9. [Crossref] [PubMed]
- Tesche C, De Cecco CN, Baumann S, Renker M, McLaurin TW, Duguay TM, Bayer RR 2nd, Steinberg DH, Grant KL, Canstein C, Schwemmer C, Schoebinger M, Itu LM, Rapaka S, Sharma P, Schoepf UJ, Coronary CT. Angiography-derived Fractional Flow Reserve: Machine Learning Algorithm versus Computational Fluid Dynamics Modeling. Radiology 2018;288:64-72. [Crossref] [PubMed]
- Coenen A, Kim YH, Kruk M, Tesche C, De Geer J, Kurata A, Lubbers ML, Daemen J, Itu L, Rapaka S, Sharma P, Schwemmer C, Persson A, Schoepf UJ, Kepka C, Hyun Yang D, Nieman K. Diagnostic Accuracy of a Machine-Learning Approach to Coronary Computed Tomographic Angiography-Based Fractional Flow Reserve: Result From the MACHINE Consortium. Circ Cardiovasc Imaging 2018;11:e007217 [Crossref] [PubMed]
- Yu M, Lu Z, Li W, Wei M, Yan J, Zhang J. CT morphological index provides incremental value to machine learning based CT-FFR for predicting hemodynamically significant coronary stenosis. Int J Cardiol 2018;265:256-61. [Crossref] [PubMed]
- Yu M, Lu Z, Shen C, Yan J, Wang Y, Lu B, Zhang J. The best predictor of ischemic coronary stenosis: subtended myocardial volume, machine learning-based FFR(CT), or high-risk plaque features? Eur Radiol 2019;29:3647-57. [Crossref] [PubMed]
- Yu M, Dai X, Yu L, Lu Z, Shen C, Tao X, Zhang J. Hemodynamic Change of Coronary Atherosclerotic Plaque After Statin Treatment: A Serial Follow-Up Study by Computed Tomography-Derived Fractional Flow Reserve. J Am Heart Assoc 2020;9:e015772 [Crossref] [PubMed]
- Motoyama S, Sarai M, Harigaya H, Anno H, Inoue K, Hara T, Naruse H, Ishii J, Hishida H, Wong ND, Virmani R, Kondo T, Ozaki Y, Narula J. Computed tomographic angiography characteristics of atherosclerotic plaques subsequently resulting in acute coronary syndrome. J Am Coll Cardiol 2009;54:49-57. [Crossref] [PubMed]
- Min JK, Shaw LJ, Devereux RB, Okin PM, Weinsaft JW, Russo DJ, Lippolis NJ, Berman DS, Callister TQ. Prognostic value of multidetector coronary computed tomographic angiography for prediction of all-cause mortality. J Am Coll Cardiol 2007;50:1161-70. [Crossref] [PubMed]
- Cury RC, Abbara S, Achenbach S, Agatston A, Berman DS, Budoff MJ, Dill KE, Jacobs JE, Maroules CD, Rubin GD, Rybicki FJ, Schoepf UJ, Shaw LJ, Stillman AE, White CS, Woodard PK, Leipsic JA. CAD-RADS(TM) Coronary Artery Disease - Reporting and Data System. An expert consensus document of the Society of Cardiovascular Computed Tomography (SCCT), the American College of Radiology (ACR) and the North American Society for Cardiovascular Imaging (NASCI). Endorsed by the American College of Cardiology. J Cardiovasc Comput Tomogr 2016;10:269-81. [Crossref] [PubMed]
- Ma R, Ties D, van Assen M, Pelgrim GJ, Sidorenkov G, van Ooijen PMA, van der Harst P, van Dijk R, Vliegenthart R. Towards reference values of pericoronary adipose tissue attenuation: impact of coronary artery and tube voltage in coronary computed tomography angiography. Eur Radiol 2020;30:6838-46. [Crossref] [PubMed]
- Itu L, Rapaka S, Passerini T, Georgescu B, Schwemmer C, Schoebinger M, Flohr T, Sharma P, Comaniciu D. A machine-learning approach for computation of fractional flow reserve from coronary computed tomography. J Appl Physiol (1985) 2016;121:42-52. [PubMed]
- Lee JM, Choi G, Koo BK, Hwang D, Park J, Zhang J, et al. Identification of High-Risk Plaques Destined to Cause Acute Coronary Syndrome Using Coronary Computed Tomographic Angiography and Computational Fluid Dynamics. JACC Cardiovasc Imaging 2019;12:1032-43. [Crossref] [PubMed]
- von Knebel Doeberitz PL, De Cecco CN, Schoepf UJ, Albrecht MH, van Assen M, De Santis D, Gaskins J, Martin S, Bauer MJ, Ebersberger U, Giovagnoli DA, Varga-Szemes A, Bayer RR 2nd, Schönberg SO, Tesche C. Impact of Coronary Computerized Tomography Angiography-Derived Plaque Quantification and Machine-Learning Computerized Tomography Fractional Flow Reserve on Adverse Cardiac Outcome. Am J Cardiol 2019;124:1340-8. [Crossref] [PubMed]
- Grant RW, Stephens JM. Fat in flames: influence of cytokines and pattern recognition receptors on adipocyte lipolysis. Am J Physiol Endocrinol Metab 2015;309:E205-13. [Crossref] [PubMed]
- Eberhard M, Nadarevic T, Cousin A, von Spiczak J, Hinzpeter R, Euler A, Morsbach F, Manka R, Keller DI, Alkadhi H. Machine learning-based CT fractional flow reserve assessment in acute chest pain: first experience. Cardiovasc Diagn Ther 2020;10:820-30. [Crossref] [PubMed]