Virtual monoenergetic images from dual-energy CT: systematic assessment of task-based image quality performance
Introduction
In the last decade, beneficial applications of dual-energy CT (DECT) have been shown for different body regions (1-8). DECT offers the generation of so called virtual monoenergetic images (VMI) which can be used to increase CT attenuation of iodinated structures, to improve CT attenuation stability or to reduce metal artifacts (9-11). They can be reconstructed at different energies in kiloelectron Volt (keV) ranging from 40 to 200 keV (12). VMI at low-keVs improve the CT attenuation and therefore the contrast of iodinated structures potentially increasing the contrast-to-noise ratio (CNR). They are therefore often used in vascular and contrast-enhanced parenchymal CT imaging (5). VMI at high-keVs on the other hand, show a reduction of beam-hardening artefacts and are used to reduce metal artefacts (13).
The use of VMI images from DECT has demonstrated improved lesion conspicuity and potential for contrast medium reduction (14) at equal or even lower radiation doses compared to traditional single-energy CT (SECT) (15). This will potentially lead to increased acceptance and implementation of DECT protocols in routine clinical practice in the future. Following these lines, certain institutions now routinely reconstruct VMI at certain keVs that are optimized for the investigated body region and clinical task in addition to linear-blended images (LBI) that aim to simulate the image perception of traditional SECT images at 120 kVp. In a white paper of the Society of Computed Tomography and Magnetic Resonance, the use of VMI was suggested in abdominal imaging, e.g., to improve conspicuity of liver or pancreatic neoplasms in late arterial phase (16). Another multi-institutional consensus statement has reported preferred VMI-energies for abdominal exams, i.e., 50 keV to improve contrast and 70 keV to decrease noise (17). The increased application of VMI may establish them as the primary diagnostic image series used in CT imaging. However, the impact of VMI on objective image quality and diagnostic accuracy has to be systematically characterized to facilitate their routine use in clinical practice.
Recently, a report of the American Association of Physicists in Medicine (AAPM) Task Group (TG-233) has suggested standardized methods to objectively assess task-based image quality parameters (18). These methods include the noise power spectrum (NPS), the task transfer function (TTF), and the detectability index (d’). These task-based metrics offer the possibility to compare different CT images based on their performance related to a diagnostic task and not solely based on the subjective perception of image quality by the reader (19). Especially d’ has shown promising results as a quality metric validated in clinical studies (20-22).
Because VMI at certain keVs have been described as being subjectively blotchy or patchy (23), potential differences in noise texture may influence detectability and hamper their widespread implementation into routine clinical practice. Recently, task-based image quality parameters have been investigated for VMI at 40–140 keV among four different DECT approaches (24) as well as for different reconstruction algorithms on a dual-source dual-energy CT (25). In both studies, the authors found differences in image noise texture, spatial resolution as well as d’ depending on the imaging setup and VMI-energy. However, both studies were limited to a single phantom size, an iodinated contrast task, a single lesion size and did not include LBI nor the full range of available VMI-energies.
Therefore, the purpose of our experimental study was to systematically investigate task-based image quality parameters of VMI compared to traditional LBI as a function of detection task, VMI-energy, patient size, lesion size, and radiation dose for dual-source dual-energy CT.
We present the following article in accordance with the MDAR reporting checklist (available at https://dx.doi.org/10.21037/qims-21-477).
Methods
This study conformed to the provisions of the Declaration of Helsinki (as revised in 2013). Ethical approval was not necessary because of the design as phantom study.
Phantom and scan setup
A commercially available task-based image quality phantom was used (Mercury Phantom 4.0, Sun Nuclear Corporation, Middleton, WI, USA). The phantom consists of polyethylene (nominal contrast of −95 HU at 120 kVp), has a length of 52 cm and five different size sections with diameters of 16, 21, 26, 31, and 36 cm, respectively (Figure 1). Of these size sections, the 16, 26, and 36 cm sections were analyzed to emulate a pediatric, an intermediate-sized adult, and a large-sized adult patients. Each size section has a subsection with uniform material to measure noise magnitude and noise texture using the NPS. Furthermore, each section has a subsection with five cylindrical inserts (each diameter of 2.54 cm) of different materials and contrast properties to assess the spatial resolution as TTF. Of these inserts, the bone, polystyrene, and iodine inserts (iodine concentration of 10 mg/mL) with a nominal contrast to the background of +1,000, +50, and +335 HU, respectively, were used.
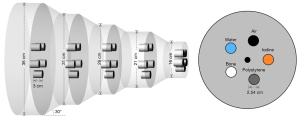
The phantom was imaged on a third-generation dual-source CT scanner (SOMATOM Force, Siemens Healthineers, Forchheim, Germany) in dual-energy mode using the vendor-specific protocol for DECT of the abdomen with the following parameters: collimation of 64×0.6 mm, gantry rotation time of 0.5 s, pitch of 0.6, tube voltage of 100 kVp for tube A and 150 kVp for tube B with additional tin filtration (Sn150 kVp). CT scans were performed at three different dose levels with a target volume CT dose index (CTDIvol) of 5, 10, and 15 mGy by adjusting the tube current time product (mAs). Scans were repeated three times for each dose level.
Datasets of the low- and high-energy spectra and LBI with a blending ratio of 0.6 were reconstructed at a slice thickness of 1.5 mm with an increment of 1 mm using a quantitative reconstruction kernel (Qr40) and an advanced modeled iterative reconstruction algorithm at a strength level of three (ADMIRE, Siemens Healthineers). These reconstruction parameters are recommended to perform dual-energy post-processing with the vendor-specific software. Here, a Qr-Kernel is optimized for quantitative analysis of dual-energy information. The field of view of the reconstruction was 420 mm.
The low- and high-energy datasets were used to create VMI using the vendor-specific workstation and a second-generation VMI reconstruction algorithm (Syngo.via VB10, Dual-Energy Workflow, Monoenergetic Plus application, Siemens Healthineers). This algorithm reconstructs VMI from the image space domain using a frequency-split approach. For further details please refer to (27). VMI were reconstructed at 15 different energies ranging from 40 to 190 keV at increments of 10 keV. Figure 2 shows example CT images of the insert sections for different phantom diameters, doses, and VMI-energies.
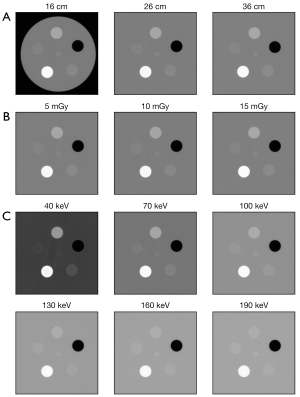
Image quality assessment
The linear-blended and VMI datasets were batch-processed using the open-source software suggested by AAPM TG-233 for TB-IQ (imQuest, Duke University, Durham, NC, USA) (18). The software contains an automated procedure specifically developed for the phantom used in this study: for each of the phantom’s size sections, the procedure locates the five inserts and returns a discrete estimation of the NPS of the noise module (one per section), the TTF (one per section and insert), and the detectability index d’ (one per section and insert) in the resolution module.
Task-based image quality assessment of the image types was divided in three elements. First, image noise magnitude and noise texture were characterized by assessing the NPS and the normalized NPS (nNPS) (28). The software automatically classified the slices to use for NPS calculation by verifying the validity of a set of conditions (absence of task inserts, absence of landmark insert, anomalous HU values); typical numbers lie in the range 25–35. On each slice the software defined 8 square ROIs of size 30 mm × 30 mm, equally spaced in 45° radial increments. The bidimensional NPS was then calculated for each ROI and averaged over the ROIs and the slices, as per Eq. [1]:
where px and py are the pixel size in the x- and y-directions, Rx and Ry are the ROI size in pixels along the x and y axis, respectively, Nsl and NROI are the numbers of slices and ROIs, FT is the Fourier transform and is the mean pixel value of each ROI measured using a second-order polynomial fitting.
The software provided 1D radially averaged NPS curves, noise magnitude (pixel SD), and NPS average and peak spatial frequency (fav and fpeak). fpeak was used to compare noise texture. For nNPS, the NPS was normalized by a function of the ROI mean (29), namely the variance of the pixels in the ROI (30).
Second, resolution was measured for each insert using the spatial frequency at which the TTF reached 50% of its maximum (TTF50) (31,32). The software automatically classified the slices to use for TTF calculation by checking for the presence of the task inserts which have a distinctive diameter; typical numbers lie in the range 25–35. On each ROI, the HU values of the pixels were plotted as a function of their distance to the insert center to calculate the edge spread function (ESF). Deriving the ESF returned the line spread function (LSF) and the TTF was calculated by taking the normalized Fourier transform of the LSF. The TTF calculations were performed on each slice separately and then averaged. If the CNR of any slice was too low for the TTF to be significant (28), the analysis was performed on a single ESF ensemble using all the slices at once.
Third, the detectability index d’ served as a metric for overall image quality because it aims to measure how well a certain material can be detected in the presence of noise (31,33). In this phantom setup, d’ represents the task of detecting a uniform circular contrast object of a certain size on a uniform background. The d’ values are calculated using a non-prewhitening matching filter (NPW) as model for the observer (18) according to the Eq. [2]:
Here, Wtask describes a 2D circular shape representing a lesion. Table 1 reports the parameters used by the software to model the lesion.
Table 1
d’ parameter | Value |
---|---|
Observer model | NPW |
Task contrast | Contrast of simulated lesion |
Task diameter | Diameter of simulated lesion |
Weight coefficient (n) | 1 |
Noise geometry | 2D |
Pixel number | 300×300 |
Pixel size | 0.05 mm |
Field of view | 15 mm |
NPW, non-prewhitening matching filter.
We decided to analyze the bone, iodine, and polystyrene inserts, as they mimic contrast differences encountered in abdominal studies in patients. Here, the bone insert served as an unenhanced high-contrast task (e.g., calcium-containing stone), the iodine insert as a contrast-enhanced high-contrast task (e.g., enhanced vascular or strongly enhancing parenchymal structure as for example a hepatocellular carcinoma), and the polystyrene insert as an unenhanced low-contrast task (e.g., non-vascular lesion or hematoma). In addition, the detectability index d’ was estimated for different simulated diameters of the three inserts varying from 3 to 10 mm. For each VMI-energy we therefore calculated 24 values (3 dose levels times 8 diameter sizes).
Results
Noise properties
Results of the NPS and nNPS as a function of image type and radiation dose are summarized in Figure 3A-3C, Tables 2-4, Tables S1-S4 and for the full frequency range in Figures S1,S2 of the Supplemental file.
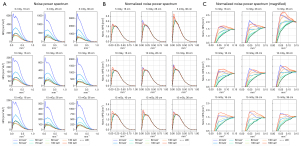
Table 2
Image type | Radiation dose, noise magnitude (HU) | ||
---|---|---|---|
5 mGy | 10 mGy | 15 mGy | |
Linear-blended images | 14.3±0.03 | 10.4±0.03 | 8.4±0.02 |
VMI 40 keV | 27.6±0.08 (+93.7%) | 20±0.05 (+92.7%) | 17.1±0.02 (+102%) |
VMI 50 keV | 20.3±0.01 (+42.3%) | 14.8±0.03 (+41.9%) | 12.4±0.03 (+46.1%) |
VMI 60 keV | 15.8±0.01 (+10.8%) | 11.5±0.02 (+11.5%) | 9.5±0.02 (+12.5%) |
VMI 70 keV | 13.2±0.04 (−7.6%) | 9.6±0.02 (−7.4%) | 7.8±0.04 (−7.7%) |
VMI 80 keV | 12.2±0.07 (−14.6%) | 8.8±0.02 (−14.4%) | 7.2±0.03 (−15.3%) |
VMI 90 keV | 12.3±0.08 (−14.0%) | 9.0±0.03 (−13.2%) | 7.3±0.02 (−13.6%) |
VMI 130 keV | 12.5±0.06 (−12.6%) | 9.2±0.02 (−12.0%) | 7.5±0.03 (−11.5%) |
VMI 190 keV | 12.6±0.05 (−11.6%) | 9.2±0.02 (−11.6%) | 7.5±0.02 (−10.8%) |
Absolute and relative noise magnitude values of each VMI-energy compared to the linear-blended images for each of the three radiation doses at the intermediate phantom size (26 cm). Linear-blended images (LBI) served as reference standard. Values are given as mean ± standard deviation. Percentage change in noise magnitude of each VMI-energy compared to LBI is given in brackets. VMI, virtual monoenergetic imaging
Table 3
Image type | Radiation dose, peak noise frequency (mm−1) | ||
---|---|---|---|
5 mGy | 10 mGy | 15 mGy | |
Linear-blended images | 0.178±0.007 (0%) | 0.189±0.001 (0%) | 0.157±0.005 (0%) |
VMI 40 keV | 0.079±0.001 (−56%) | 0.079±0.002 (−58%) | 0.079±0.013 (−50%) |
VMI 50 keV | 0.163±0.007 (−9%) | 0.184±0.020 (−3%) | 0.189±0.020 (+20%) |
VMI 60 keV | 0.163±0.007 (−9%) | 0.189±0.013 (0%) | 0.189±0.007 (+20%) |
VMI 70 keV | 0.168±0.007 (−6%) | 0.189±0.013 (0%) | 0.189±0.013 (+20%) |
VMI 80 keV | 0.168±0.015 (−6%) | 0.184±0.007 (−3%) | 0.189±0.013 (+20%) |
VMI 90 keV | 0.168±0.015 (−6%) | 0.184±0.007 (−3%) | 0.142±0.007 (−10%) |
VMI 130 keV | 0.094±0.007 (−47%) | 0.100±0.007 (−47%) | 0.094±0.007 (−40%) |
VMI 190 keV | 0.079±0.013 (−56%) | 0.084±0.007 (−56%) | 0.079±0.007 (−50%) |
Absolute and relative peak noise frequency (fpeak) values of each VMI-energy compared to the linear-blended images for each of the three radiation doses at the intermediate phantom size (26 cm). Linear-blended images (LBI) served as reference standard. Values are given as mean ± standard deviation. Percentage change in noise magnitude of each VMI-energy compared to LBI is given in brackets. VMI, virtual monoenergetic imaging.
Table 4
Image type | Radiation dose, average noise frequency (mm−1) | ||
---|---|---|---|
5 mGy | 10 mGy | 15 mGy | |
Linear-blended images | 0.265±0.001 (0%) | 0.273±0.002 (0%) | 0.273±0.001 (0%) |
VMI 40 keV | 0.245±0.002 (−8%) | 0.254±0.001 (−7%) | 0.256±0.003 (−6%) |
VMI 50 keV | 0.256±0.002 (−4%) | 0.264±0.001 (−3%) | 0.265±0.002 (−3%) |
VMI 60 keV | 0.264±0.002 (−1%) | 0.271±0.002 (−1%) | 0.272±0.007 (−0%) |
VMI 70 keV | 0.267±0.001 (+0%) | 0.273±0.007 (−0%) | 0.275±0.013 (+1%) |
VMI 80 keV | 0.264±0.001 (−0%) | 0.271±0.003 (−1%) | 0.272±0.007 (−1%) |
VMI 90 keV | 0.261±0.001 (−2%) | 0.268±0.009 (−2%) | 0.267±0.007 (−2%) |
VMI 130 keV | 0.251±0.001 (−6%) | 0.259±0.013 (−5%) | 0.255±0.001 (−7%) |
VMI 190 keV | 0.246±0.007 (−8%) | 0.255±0.007 (−7%) | 0.249±0.007 (−9%) |
Absolute and relative average noise frequency (fav) values of each VMI-energy compared to the linear-blended images for each of the three radiation doses at the intermediate phantom size (26 cm). Linear-blended images (LBI) served as reference standard. Values are given as mean ± standard deviation. Percentage change in noise magnitude of each VMI-energy compared to LBI is given in brackets. VMI, virtual monoenergetic imaging.
Noise magnitude increased with decreasing radiation dose (e.g., for the intermediate size at 40 keV from 17.1 HU at 15 mGy to 27.6 HU at 5 mGy) and with decreasing VMI-energy (from 12.6 HU at 190 keV and 5 mGy to 27.6 HU at 40 keV and 5 mGy) for all three sizes. Noise magnitude was higher for VMI at 40, 50, and 60 keV compared to the LBI for all three radiation doses (Figure 3A).
Differences in noise texture (nNPS) were found for both very low and very high VMI-energies (40–60 keV, 130–190 keV) with a stronger noise contribution in the low spatial frequency region between 0.0 and 0.1 mm−1 (Figure 3B). VMI at 60-80 keV demonstrated similar noise texture compared to LBI. Furthermore, a slight frequency shift to lower frequencies was found with increasing size (Figure 3B,3C).
Resolution properties
Results of spatial resolution (TTF) as a function of insert, image type, and radiation dose are summarized in Figure 4A-4C and Table 5.
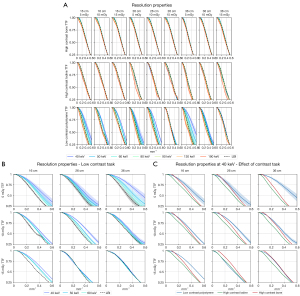
Table 5
Image type | Radiation dose | ||||||||||
---|---|---|---|---|---|---|---|---|---|---|---|
5 mGy | 10 mGy | 15 mGy | |||||||||
Bone | Iodine | Poly | Bone | Iodine | Poly | Bone | Iodine | Poly | |||
Linear-blended | 0.37±0.003 | 0.35±0.01 | 0.34±0.04 | 0.39±0.001 | 0.37±0.004 | 0.34±0.013 | 0.39±0.04 | 0.38±0.013 | 0.32±0.04 | ||
VMI 40 keV | +1.8% | −4.5% | +27.7% | +1.4% | −6.1% | +40.0% | +1.5% | −5.9% | +6.3% | ||
VMI 50 keV | +1.7% | −2.8% | +14.6% | +1.3% | −4.1% | +19.1% | +1.5% | −4.1% | +3.6% | ||
VMI 60 keV | +1.6% | −0.5% | +7.3% | +1.3% | −1.5% | +8.2% | +1.4% | −1.5% | +0.8% | ||
VMI 70 keV | +1.4% | +2.1% | +1.2% | +1.2% | +1.8% | +2.5% | +1.3% | +1.4% | −0.6% | ||
VMI 80 keV | +0.2% | +0.4% | −5.4% | +0.2% | +0.7% | −3.8% | +0.2% | +0.6% | −3.3% | ||
VMI 90 keV | −1.0% | −1.6% | −9.4% | −0.8% | −0.8% | −6.2% | −0.8% | −0.4% | −0.6% | ||
VMI 130 keV | −3.7% | −10.6% | −15.6% | −3.2% | −5.7% | −11.0% | −3.3% | −4.8% | −6.9% | ||
VMI 190 keV | −5.4% | −28.0% | −16.8% | −4.7% | −10.2% | −12.3% | −4.9% | −9.6% | −5.7% |
Task transfer function-frequency shift (TTF50) for each VMI-energy relative to the linear-blended images as a function of insert type and radiation dose at the intermediate phantom size (26 cm). Data is reported to illustrate general trends. For non-iodinated tasks, a monotonic behavior of VMI energies was found with decreasing resolution as the VMI-energy increases. For the iodinated task worse resolution compared to LBI was found at low and high energies and slightly improved resolution at 70–80 keV. Data indicates TTF50 in cycles per mm with standard deviation for linear-blended images as the reference standard. Data for VMI-energies is giving in percentage change compared to the reference. A positive change indicates a higher spatial frequency and therefore a higher in-plane spatial resolution. VMI, virtual monoenergetic imaging.
Overall, the shape of the TTFs was unaffected by all three parameters for the bone and iodine inserts, indicating similar in-plane spatial resolution for high-contrast tasks (Figure 4A). The only exception was VMI at 190 keV for the iodine insert which showed decreased spatial resolution. For the polystyrene insert representing the low-contrast task, higher TTF50-frequencies were observed at low VMI-energies of 40–60 keV, indicating higher in-plane spatial resolution. This effect was on average pronounced at the lowest radiation dose (Figure 4B,4C). This increased spatial resolution is also highlighted in the corresponding edge spread functions (ESF) as an increase in the slope of the ESF curves at low VMI-energies for the polystyrene insert (Figure S3 in the Supplemental file).
Detectability properties
Results of the detectability index for each insert as a function of image type, radiation dose, phantom size, and lesion diameter are visualized in Figure 5A-5C.
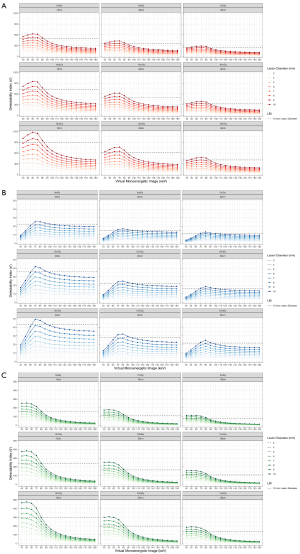
For all image types and inserts, the detectability index increased with increasing radiation dose, decreasing phantom size, and increasing lesion diameter. The increase in d’ with increasing radiation dose was non-linear showing a stronger increase between 5 and 10 mGy than between 10 and 15 mGy.
For the bone and iodine insert (high-contrast tasks), d’ was higher for VMI at 40–80 keV compared to LBI at all three radiation doses. For both inserts, d’ dropped substantially at higher VMI-energies (Figure 6). D’ was highest for 60 keV for the high-contrast bone task and for 50 keV for the high-contrast iodine task.
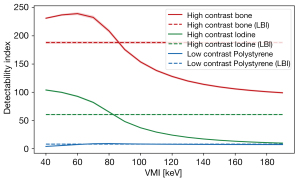
For the polystyrene insert (low-contrast task), a higher d’ was found for VMI at 70–90 keV while d’ was lower for VMI at 40–60 keV (Figure 6).
Discussion
In this study we systematically characterized task-based image quality metrics of VMI compared to traditional LBI from dual-energy CT. As VMI have become increasingly accepted as an alternative to conventional SECT images, particularly in abdominal CT, objective task-based characterization of their image quality has become important to benchmark and ensure diagnostic accuracy throughout the process of clinical implementation. Our results suggest that the detectability improved with increasing radiation dose, decreasing phantom size, and increasing lesion diameter, and differed depending on the VMI-energy and contrast task. Averaged across all settings, the detectability index d’ was highest for VMI at 60 keV for the high-contrast bone task, for VMI at 50 keV for the high-contrast iodine task, and for VMI at 70–80 keV for the unenhanced low-contrast task (polystyrene). Taking into account the prevalence and clinical role of contrast-enhanced imaging, e.g., in parenchymal lesion detection and vascular imaging, we consider the iodine contrast task as the task with the highest clinical impact out of the three investigated tasks. Therefore, our findings suggest VMI at 50–60 keV as an overall comparable to superior alternative to LBI.
Noise magnitude and texture
Assessment of the NPS revealed, as expected, decreasing noise magnitude with increasing radiation dose level and decreasing phantom size. Moreover, noise magnitude increased substantially for low-keV VMI-energies. Noise texture was comparable for the majority of VMI-energies with the exception of very low- and very-high keVs. These showed frequency shifts to lower frequencies compared to LBI indicating blotchier noise texture. This finding is in line with the results by Greffier et al. who found a peak in the average spatial frequency at 70 keV with a shift towards lower frequencies from 70–40 and 70–100 keV on the same dual-source DECT system (25). We found comparable noise magnitude and noise texture to LBI for VMI at 70–90 keV which confirms prior reports of similar appearance of VMI at 75 keV compared to traditional images at 120 kVp (32). In addition, we found a slight shift to lower frequency noise content at lower radiation dose. To compensate for these low signal conditions, the scanner and reconstruction algorithm applied low-signal corrections, which led to a stronger smoothing during the reconstruction process (32). The increased noise magnitude of low-keV VMI-energies could be reduced in the future by applying advanced denoising techniques, e.g., prior-knowledge-aware iterative denoising (mono-PKAID) (23). PKAID demonstrated decreased image noise compared to the second-generation VMI algorithm used in this study which consecutively improved iodine CNR (23).
Spatial resolution
Assessment of spatial resolution properties revealed no dependence of the TTF on the radiation dose level, phantom size, or the VMI-energy for both high-contrast tasks (bone and iodine). However, a right shift to higher frequencies of the TTF50 was found for the low-contrast polystyrene insert for low-energy VMI (40–60 keV), indicating higher in-plane spatial resolution. An increase in spatial resolution at low keV-energies has been also described by Greffier et al. in three studies in which an acrylic insert was used. Here, the highest TTF50 values were found for VMI at 40 keV and a shift towards lower frequencies was observed with increasing VMI-energy (24,25,34). The authors hypothesized that this finding was related to changes of the enhancement of the border of the acrylic insert with decreasing VMI-energy which introduced on overshoot on the edge spread function (ESF) curves which improved the TTF (24,34). We observed the same effect for the polystyrene in our study as demonstrated by the increased slopes of the ESF curves at low VMI-energies. We therefore think that this increased spatial resolution is mainly caused by the insert material and not a true property of VMI images of this contrast task. However, despite the measured improvement in spatial resolution, lower d’-values were found for the low-contrast task at 40–60 keV VMI which might be caused by the increased low-frequency noise found in the NPS of these images.
Detectability
VMI at low-energies (e.g., 40–60 keV) achieved substantially higher d’-values for the high-contrast tasks despite inferior noise properties compared to LBI. We attribute this to the increased CT-attenuation and contrast of bone and iodine at lower energies. This finding is in line with results of former clinical studies that demonstrated benefits using VMI at low-keVs for imaging of contrast-enhanced structures, e.g., vascular imaging (35,36). Interestingly, d’ increased slightly from 40 to 50 keV for the iodine contrast task before decreasing with increasing VMI-energy. This behavior is comparable to the insert with lower iodine concentration (2 mgI/mL) in the study by Greffier et al. (25) while it differs from the insert with higher iodine concentration (4 mgI/mL) for which a decrease of d’ was observed for VMI-energies higher than 40 keV (24,25).
As a general observation, our results indicate that the optimization of VMI-energy offers potential for dose reduction. In many cases, d’-values of LBI can be achieved using certain VMI-energies of a lower radiation dose, e.g., for the iodine insert the d’ of the LBI at 15 mGy were comparable to the d’ of 70 keV-VMI at 5 mGy. This indicates potential for dose reduction if VMI are properly chosen dependent on the clinical task (high vs. low-contrast task).
We observed similar trends among the VMI-energies for the three inserts for all simulated lesion diameters with, as expected, increasing d’ at increasing lesion diameter. Furthermore, we observed the same trends for all three simulated patient sizes.
The results of our study indicate that the optimal VMI-energy depends on the contrast task. The low-contrast task in our study aimed to emulate a non-enhancing low-contrast lesion as for example a cystic lesion, a hematoma or a necrotic lesion after therapy. Our findings highlight that the optimal detectability for lesion with these contrast properties differs from highly vascular/strongly iodine enhancing lesions. Interpreting our findings in regards to clinical relevance, we consider the iodine contrast task as the task with the highest clinical impact due to the frequency of contrast-enhanced image studies in diagnostic imaging and the role of iodine-enhancement in lesion detection and characterization. While the iodine concentration in our study was rather high (10 mgI/mL), it was in the reported range of highly vascular tumors as for example hepatocellular carcinoma (37). Based on our results, we suggest VMIs at 50–60 keV as most useful in a clinical context in addition or as a surrogate for LBI yielding comparable or higher d’-values for all three contrast tasks at all three sizes. This is comparable to the results of former clinical studies in which low-energy VMIs of 40–60 keV demonstrated improved lesion delineation [e.g., 40 keV in hypoattenuating liver metastasis (38) and improved lesion conspicuity (39), e.g., 50 keV in hypervascular liver lesions (40)]. Furthermore, a comparable VMI-energy of 50 keV was proposed by Zhou et al. who found a greater or similar iodine CNR and iodine detectability compared to traditional images from single-energy CT in an abdominal phantom simulating liver lesions (41) and compared to iodine maps from photon-counting detector CT (42). A difference to our study was the use of a photon-counting detector CT, the comparison of VMI to single-energy images instead of LBI and the use of a channelized Hotelling observer (CHO) for lesion detectability. The results by Zhou et al. and our study promote the standard use of VMIs at 50 or 60 keV for contrast-enhanced imaging which could reduce the complexity of VMI imaging from a workflow perspective in which the user/radiologist typically has to choose from a large number of possible VMI-energies.
Limitations
First, there are inherent limitations of using phantoms instead of human/patient data. This includes also the shape of the phantom which did not resemble the anthropomorphic shape of a human body. In addition, the phantom modules were homogeneous and did not include heterogeneous texture as typically found in certain human organs. Heterogeneous texture has shown to impact detectability for different reconstruction algorithms (43) and its effect in VMI is unknown. Second, we did not assess the impact of automatic tube current modulation. We purposely deactivated the automatic tube current modulation for this study because we wanted to analyze the impact of size and radiation dose on task-based image quality metrics in a standardized and controlled fashion. Third, the materials of the phantom and inserts did not perfectly emulate human tissues or pathologies. However, the concept of high-contrast task with and without iodine as well as low-contrast tasks is a reasonable extrapolation for common tasks in diagnostic imaging. Forth, we have only investigated one possible tube voltage combination (100/Sn150 kVp) of the dual-source DECT. However, we do not expect significant changes in the trends of task-based image quality observed in our study at lower tube voltage combinations. Fifth, we did not investigate the impact of different reconstruction algorithms, kernels or denoising on the d’ among VMIs. Reconstruction algorithms and kernels alter noise texture and spatial resolution which impacts d’ dependent on the contrast task. Nevertheless, we hypothesize that the trends among VMI and LBI observed in our study would remain similar.
Conclusions
In conclusion, results of our study suggest that task-based image quality metrics, e.g., the detectability index, differ among different energies of VMI depending on the contrast task. Detectability increased with increasing radiation dose and decreasing size. An improved detectability index or a reduced radiation dose compared to traditional LBI can be achieved by tailoring the use of VMI-energies to the diagnostic task at hand. Considering clinical relevance of iodine, VMIs at 50–60 keV could be proposed as an alternative to LBI.
Acknowledgments
The authors thank Mrs. Sarah Euler, MScN, RN for revising the manuscript.
Funding: None.
Footnote
Reporting Checklist: The authors have completed the MDAR reporting checklist. Available at https://dx.doi.org/10.21037/qims-21-477
Conflicts of Interest: All authors have completed the ICMJE uniform disclosure form (available at https://dx.doi.org/10.21037/qims-21-477). The authors have no conflicts of interest to declare.
Ethical Statement: The authors are accountable for all aspects of the work in ensuring that questions related to the accuracy or integrity of any part of the work are appropriately investigated and resolved. This study conformed to the provisions of the Declaration of Helsinki (as revised in 2013). Ethical approval was not necessary because of the design as phantom study.
Open Access Statement: This is an Open Access article distributed in accordance with the Creative Commons Attribution-NonCommercial-NoDerivs 4.0 International License (CC BY-NC-ND 4.0), which permits the non-commercial replication and distribution of the article with the strict proviso that no changes or edits are made and the original work is properly cited (including links to both the formal publication through the relevant DOI and the license). See: https://creativecommons.org/licenses/by-nc-nd/4.0/.
References
- Bodanapally UK, Shanmuganathan K, Ramaswamy M, Tsymbalyuk S, Aarabi B, Parikh GY, Schwartzbauer G, Dreizin D, Simard JM, Ptak T, Li G, Liang Y, Fleiter TR. Iodine-based Dual-Energy CT of Traumatic Hemorrhagic Contusions: Relationship to In-Hospital Mortality and Short-term Outcome. Radiology 2019;292:730-8. [Crossref] [PubMed]
- Ohta Y, Kitao S, Yunaga H, Fujii S, Mukai N, Yamamoto K, Ogawa T. Myocardial Delayed Enhancement CT for the Evaluation of Heart Failure: Comparison to MRI. Radiology 2018;288:682-91. [Crossref] [PubMed]
- Martin SS, Weidinger S, Czwikla R, Kaltenbach B, Albrecht MH, Lenga L, Vogl TJ, Wichmann JL. Iodine and Fat Quantification for Differentiation of Adrenal Gland Adenomas From Metastases Using Third-Generation Dual-Source Dual-Energy Computed Tomography. Invest Radiol 2018;53:173-8. [Crossref] [PubMed]
- McGrath TA, Frank RA, Schieda N, Blew B, Salameh JP, Bossuyt PMM, McInnes MDF. Diagnostic accuracy of dual-energy computed tomography (DECT) to differentiate uric acid from non-uric acid calculi: systematic review and meta-analysis. Eur Radiol 2020;30:2791-801. [Crossref] [PubMed]
- Lourenco PDM, Rawski R, Mohammed MF, Khosa F, Nicolaou S, McLaughlin P, Dual-Energy CT. Iodine Mapping and 40-keV Monoenergetic Applications in the Diagnosis of Acute Bowel Ischemia. AJR Am J Roentgenol 2018;211:564-70. [Crossref] [PubMed]
- Yu Z, Mao T, Xu Y, Li T, Wang Y, Gao F, Sun W. Diagnostic accuracy of dual-energy CT in gout: a systematic review and meta-analysis. Skeletal Radiol 2018;47:1587-93. [Crossref] [PubMed]
- Shi L, Lu M, Bennett NR, Shapiro E, Zhang J, Colbeth R, Star-Lack J, Wang AS. Characterization and potential applications of a dual-layer flat-panel detector. Med Phys 2020;47:3332-43. [Crossref] [PubMed]
- Jacobsen MC, Thrower SL, Ger RB, Leng S, Court LE, Brock KK, Tamm EP, Cressman ENK, Cody DD, Layman RR. Multi-energy computed tomography and material quantification: Current barriers and opportunities for advancement. Med Phys 2020;47:3752-71. [Crossref] [PubMed]
- Michalak G, Grimes J, Fletcher J, Halaweish A, Yu L, Leng S, McCollough C. Technical Note: Improved CT number stability across patient size using dual-energy CT virtual monoenergetic imaging. Med Phys 2016;43:513. [Crossref] [PubMed]
- Noid G, Tai A, Schott D, Mistry N, Liu Y, Gilat-Schmidt T, Robbins JR, Li XA. Technical Note: Enhancing soft tissue contrast and radiation-induced image changes with dual-energy CT for radiation therapy. Med Phys 2018; Epub ahead of print. [Crossref] [PubMed]
- McCollough CH, Boedeker K, Cody D, Duan X, Flohr T, Halliburton SS, Hsieh J, Layman RR, Pelc NJ. Principles and applications of multienergy CT: Report of AAPM Task Group 291. Med Phys 2020;47:e881-912. [Crossref] [PubMed]
- Duan X, Arbique G, Guild J, Xi Y, Anderson J. Technical Note: Quantitative accuracy evaluation for spectral images from a detector-based spectral CT scanner using an iodine phantom. Med Phys 2018;45:2048-53. [Crossref] [PubMed]
- Rajiah P, Sundaram M, Subhas N. Dual-Energy CT in Musculoskeletal Imaging: What Is the Role Beyond Gout? AJR Am J Roentgenol 2019;213:493-505. [Crossref] [PubMed]
- Carrascosa P, Leipsic JA, Capunay C, Deviggiano A, Vallejos J, Goldsmit A, Rodriguez-Granillo GA. Monochromatic image reconstruction by dual energy imaging allows half iodine load computed tomography coronary angiography. Eur J Radiol 2015;84:1915-20. [Crossref] [PubMed]
- Pinho DF, Kulkarni NM, Krishnaraj A, Kalva SP, Sahani DV. Initial experience with single-source dual-energy CT abdominal angiography and comparison with single-energy CT angiography: image quality, enhancement, diagnosis and radiation dose. Eur Radiol 2013;23:351-9. [Crossref] [PubMed]
- De Cecco CN, Boll DT, Bolus DN, Foley WD, Kaza RK, Morgan DE, Rofsky NM, Sahani DV, Schoepf UJ, Shuman WP, Siegel MJ, Vrtiska TJ, Yeh BM, Berland LL. White Paper of the Society of Computed Body Tomography and Magnetic Resonance on Dual-Energy CT, Part 4: Abdominal and Pelvic Applications. J Comput Assist Tomogr 2017;41:8-14. [Crossref] [PubMed]
- Patel BN, Alexander L, Allen B, Berland L, Borhani A, Mileto A, Moreno C, Morgan D, Sahani D, Shuman W, Tamm E, Tublin M, Yeh B, Marin D. Dual-energy CT workflow: multi-institutional consensus on standardization of abdominopelvic MDCT protocols. Abdom Radiol (NY) 2017;42:676-87. [Crossref] [PubMed]
- Samei E, Bakalyar D, Boedeker KL, Brady S, Fan J, Leng S, Myers KJ, Popescu LM, Ramirez Giraldo JC, Ranallo F, Solomon J, Vaishnav J, Wang J. Performance evaluation of computed tomography systems: Summary of AAPM Task Group 233. Med Phys 2019;46:e735-56. [Crossref] [PubMed]
- Greffier J, Frandon J, Larbi A, Beregi JP, Pereira F. CT iterative reconstruction algorithms: a task-based image quality assessment. Eur Radiol 2020;30:487-500. [Crossref] [PubMed]
- Solomon J, Mileto A, Ramirez-Giraldo JC, Samei E. Diagnostic Performance of an Advanced Modeled Iterative Reconstruction Algorithm for Low-Contrast Detectability with a Third-Generation Dual-Source Multidetector CT Scanner: Potential for Radiation Dose Reduction in a Multireader Study. Radiology 2015;275:735-45. [Crossref] [PubMed]
- Smith TB, Solomon J, Samei E. Estimating detectability index in vivo: development and validation of an automated methodology. J Med Imaging (Bellingham) 2018;5:031403 [PubMed]
- Lacy T, Ding A, Minkemeyer V, Frush D, Samei E. Patient-based Performance Assessment for Pediatric Abdominal CT: An Automated Monitoring System Based on Lesion Detectability and Radiation Dose. Acad Radiol 2021;28:217-24. [Crossref] [PubMed]
- Tao S, Rajendran K, Zhou W, Fletcher JG, McCollough CH, Leng S. Improving iodine contrast to noise ratio using virtual monoenergetic imaging and prior-knowledge-aware iterative denoising (mono-PKAID). Phys Med Biol 2019;64:105014 [Crossref] [PubMed]
- Greffier J, Si-Mohamed S, Dabli D, de Forges H, Hamard A, Douek P, Beregi JP, Frandon J. Performance of four dual-energy CT platforms for abdominal imaging: a task-based image quality assessment based on phantom data. Eur Radiol 2021;31:5324-34. [Crossref] [PubMed]
- Greffier J, Dabli D, Hamard A, Akessoul P, Belaouni A, Beregi JP, Frandon J. Impact of dose reduction and the use of an advanced model-based iterative reconstruction algorithm on spectral performance of a dual-source CT system: A task-based image quality assessment. Diagn Interv Imaging 2021;102:405-12. [Crossref] [PubMed]
- Euler A, Solomon J, Marin D, Nelson RC, Samei E. A Third-Generation Adaptive Statistical Iterative Reconstruction Technique: Phantom Study of Image Noise, Spatial Resolution, Lesion Detectability, and Dose Reduction Potential. AJR Am J Roentgenol 2018;210:1301-8. [Crossref] [PubMed]
- Grant KL, Flohr TG, Krauss B, Sedlmair M, Thomas C, Schmidt B. Assessment of an advanced image-based technique to calculate virtual monoenergetic computed tomographic images from a dual-energy examination to improve contrast-to-noise ratio in examinations using iodinated contrast media. Invest Radiol 2014;49:586-92. [Crossref] [PubMed]
- Chen B, Christianson O, Wilson JM, Samei E. Assessment of volumetric noise and resolution performance for linear and nonlinear CT reconstruction methods. Med Phys 2014;41:071909 [Crossref] [PubMed]
- Dobbins JT 3rd, Samei E, Ranger NT, Chen Y. Intercomparison of methods for image quality characterization. II. Noise power spectrum. Med Phys 2006;33:1466-75. [Crossref] [PubMed]
- Solomon J, Lyu P, Marin D, Samei E. Noise and spatial resolution properties of a commercially available deep learning-based CT reconstruction algorithm. Med Phys 2020;47:3961-71. [Crossref] [PubMed]
- Solomon J, Wilson J, Samei E. Characteristic image quality of a third generation dual-source MDCT scanner: Noise, resolution, and detectability. Med Phys 2015;42:4941-53. [Crossref] [PubMed]
- Euler A, Solomon J, Farjat AE, Nelson RC, Samei E, Marin D. High-Pitch Wide-Coverage Fast-Kilovoltage-Switching Dual-Energy CT: Impact of Pitch on Noise, Spatial Resolution, and Iodine Quantification in a Phantom Study. AJR Am J Roentgenol 2019;212:W64-72 [Crossref] [PubMed]
- Rajagopal JR, Farhadi F, Solomon J, Sahbaee P, Saboury B, Pritchard WF, Jones EC, Samei E. Comparison of Low Dose Performance of Photon-Counting and Energy Integrating CT. Acad Radiol 2020; Epub ahead of print. [Crossref] [PubMed]
- Greffier J, Frandon J, Hamard A, Teissier JM, Pasquier H, Beregi JP, Dabli D. Impact of iterative reconstructions on image quality and detectability of focal liver lesions in low-energy monochromatic images. Phys Med 2020;77:36-42. [Crossref] [PubMed]
- Agrawal MD, Oliveira GR, Kalva SP, Pinho DF, Arellano RS, Sahani DV. Prospective Comparison of Reduced-Iodine-Dose Virtual Monochromatic Imaging Dataset From Dual-Energy CT Angiography With Standard-Iodine-Dose Single-Energy CT Angiography for Abdominal Aortic Aneurysm. AJR Am J Roentgenol 2016;207:W125-32 [Crossref] [PubMed]
- Martin SS, Wichmann JL, Weyer H, Scholtz JE, Leithner D, Spandorfer A, Bodelle B, Jacobi V, Vogl TJ, Albrecht MH. Endoleaks after endovascular aortic aneurysm repair: Improved detection with noise-optimized virtual monoenergetic dual-energy CT. Eur J Radiol 2017;94:125-32. [Crossref] [PubMed]
- Mulé S, Pigneur F, Quelever R, Tenenhaus A, Baranes L, Richard P, Tacher V, Herin E, Pasquier H, Ronot M, Rahmouni A, Vilgrain V, Luciani A. Can dual-energy CT replace perfusion CT for the functional evaluation of advanced hepatocellular carcinoma? Eur Radiol 2018;28:1977-85. [Crossref] [PubMed]
- Lenga L, Czwikla R, Wichmann JL, Leithner D, Albrecht MH, Booz C, Arendt CT, Yel I, D'Angelo T, Vogl TJ, Martin SS. Dual-energy CT in patients with colorectal cancer: Improved assessment of hypoattenuating liver metastases using noise-optimized virtual monoenergetic imaging. Eur J Radiol 2018;106:184-91. [Crossref] [PubMed]
- Hanson GJ, Michalak GJ, Childs R, McCollough B, Kurup AN, Hough DM, Frye JM, Fidler JL, Venkatesh SK, Leng S, Yu L, Halaweish AF, Harmsen WS, McCollough CH, Fletcher JG. Low kV versus dual-energy virtual monoenergetic CT imaging for proven liver lesions: what are the advantages and trade-offs in conspicuity and image quality? A pilot study. Abdom Radiol (NY) 2018;43:1404-12. [Crossref] [PubMed]
- Shuman WP, Green DE, Busey JM, Mitsumori LM, Choi E, Koprowicz KM, Kanal KM. Dual-energy liver CT: effect of monochromatic imaging on lesion detection, conspicuity, and contrast-to-noise ratio of hypervascular lesions on late arterial phase. AJR Am J Roentgenol 2014;203:601-6. [Crossref] [PubMed]
- Zhou W, Michalak GJ, Weaver JM, Gong H, Yu L, McCollough CH, Leng S. A Universal Protocol for Abdominal CT Examinations Performed on a Photon-Counting Detector CT System: A Feasibility Study. Invest Radiol 2020;55:226-32. [Crossref] [PubMed]
- Zhou W, Michalak G, Weaver J, Ferrero A, Gong H, Fetterly KA, McCollough CH, Leng S. Determination of iodine detectability in different types of multiple-energy images for a photon-counting detector computed tomography system. J Med Imaging (Bellingham) 2019;6:043501 [Crossref] [PubMed]
- Solomon J, Ba A, Bochud F, Samei E. Comparison of low-contrast detectability between two CT reconstruction algorithms using voxel-based 3D printed textured phantoms. Med Phys 2016;43:6497. [Crossref] [PubMed]