Evaluation of semi-quantitative measures of 18F-flutemetamol PET for the clinical diagnosis of Alzheimer’s disease
Introduction
Positron emission tomography (PET) with amyloid-beta (Aβ) tracers, such as 18F-flutemetamol, is useful to evaluate cortical Aβ burden in patients with cognitive impairment (1,2). Aβ-PET is acknowledged as a biomarker for Alzheimer’s disease (AD), used together with other biomarkers to support a clinical diagnosis (3,4) and to classify patients in the AD continuum (5). 18F-flutemetamol PET is validated for binary visual classification (6) which is used in the clinical setting for diagnostic purposes. Semi-quantification of tracer uptake is frequently used in research (5,7) and may be used to support a visual classification in clinical practice, which is particularly helpful in equivocal cases. The clinical usefulness of thresholds is still under evaluation. Other strengths of semi-quantification are that it offers a more objective image evaluation compared to visual classification, and it functions as a continuous measure of the Aβ burden. This might facilitate the detection of changes in Aβ burden over time or in response to an intervention. However, different Aβ tracers, imaging protocols, PET scanners, and software for semi-quantification are used between centers. These factors represent a challenge when results are compared across centers and when thresholds are applied. Different commercially available software are used to semi-quantify Aβ-PET images and many centers have additionally developed their own semi-quantification methods. SyngoVia (Siemens Healthineers) has been validated for the semi-quantification of 18F-florbetapir (8). CortexID suite (GE Healthcare), hereafter referred to as CortexID, has been validated for clinical use to support the visual classification of 18F-flutemetamol PET images (9-12). SyngoVia and CortexID do not enable magnetic resonance imaging (MRI) to be used and utilize only PET or PET together with a low dose computed tomography (CT). PMOD (PMOD Technologies LLC) is a research software comprising a tool for post-processing brain PET images (PNEURO). PNEURO facilitates the use of MRI during post-processing of PET to enable the segmentation of MRI images into grey and white matter. This is beneficial for a subsequent correct assessment of cortical 18F-flutemetamol and partial volume correction (PVC), to correct errors due to limited spatial resolution of PET images. “The Centiloid Project” was recently published with the purpose to standardize the semi-quantification of Aβ-PET (7). To our knowledge, no studies have compared standardized uptake value ratios (SUVR) from commercially available software for semi-quantification of 18F-flutemetamol PET, although methods of semi-quantification have been explored quite extensively (9,13-16). Hence, it is of interest to explore differences in SUVR obtained from different software and compare SUVR and Centiloid (CL) with validated visual classification to facilitate appropriate use of semi-quantification and thresholds in both research and clinical practice.
We compare 18F-flutemetamol SUVRs obtained with two commonly used PET-only software (SyngoVia and CortexID) and PMOD using two different atlases (Automatic anatomical labeling (AAL)-merged atlas and CL atlas). The aims are: (I) to evaluate how SUVRpons and SUVRcer from CortexID, SyngoVia, and PMOD correspond to each other and visual classification, as well as how CL corresponds to visual classification; (II) to estimate semi-quantitative thresholds that may be applied to support visual classification in a memory clinic population; (III) to assess differences in SUVR between patients with AD and non-AD diagnoses.
Methods
Study population
This observational cross-sectional study includes 195 patients with cognitive complaints, who had 18F-flutemetamol PET performed between February 2015 and October 2018. All patients were referred from the Memory Clinic at Oslo University Hospital (OUH), Ullevål. The study was conducted in accordance with the Declaration of Helsinki (as revised in 2013). Informed consent was obtained from all participants in the study by inclusion in the Norwegian registry of persons assessed for cognitive symptoms (NorCog). At the time of inclusion in NorCog, all patients were deemed to have sufficient cognitive capacity to consent. The Regional Ethics Committee for medical research in the South-East of Norway (REC 2017/1929) and the Data Protection Officer at our institution approved the study.
18F-flutemetamol PET and MRI acquisition
All patients were examined using the same PET/CT scanner, Siemens Biograph40 mCT (Siemens Healthineers, Erlangen, Germany). A standard protocol for 18F-flutemetamol PET/CT was used for all patients. Image acquisition started approximately 90 minutes (mean 91.2, SD 6.6) after a bolus injection of 185 MBq (mean 187.4, SD 7.9) 18F-flutemetamol. Low-dose CT was performed for attenuation correction and anatomic information, without contrast enhancement (120 kV, 70 mAs, and with a slice thickness of 3 mm). PET data were acquired for 20 minutes (four frames of 5 minutes each). 3D dynamic emission data were reconstructed using a resolution recovery algorithm with time of flight (TrueX with 2 iterations, 21 subsets, and a Gaussian filter with a full width half maximum (FWHM) of 2 mm and a matrix size of 400×400). The slice thickness of the reconstructed image series was 2 mm.
Most patients had MRI performed within 1 year of PET, where 101 patients had volumetric T1-weighted images available. MRI was performed as part of the diagnostic evaluation in the clinical routine on scanners at different referring hospitals and clinics.
Semi-quantification of 18F-flutemetamol uptake
Image selection for the different software is shown in Figure 1. All PET images were semi-quantified using the “Ratio” function (in later versions named “cortex”) in MI-neurology application from SyngoVia (version VB20) and CortexID (AW server, version 3.2). In SyngoVia the PET volume is spatially normalized to a template built on MNI space, using the AAL-atlas and a VOI set that has been adopted from Fleisher et al. (8). CortexID uses an adaptive template method as described by Lundqvist et al. (12) to spatially normalize the PET volume to a template similar to “the narrow VOI set” previously described (9,12). SyngoVia provides six regions (anterior cingulate, frontal, parietal, posterior cingulate, precuneus, temporal) covering both hemispheres while CortexID provides eight cortical regions (prefrontal, anterior cingulate, precuneus/posterior cingulate, parietal, lateral temporal, occipital, sensorimotor, temporal mesial) in each hemisphere. Both SyngoVia and CortexID allow pons and cerebellar cortex to be used as reference region. Volumes for each region are not provided, consequently, the composite SUVRs are not volume-weighted but represent the mean of SUVR across all regions. All PET images with volumetric T1-weighted MRI available within 1 year were semi-quantified with PMOD (17). PMOD uses a co-registration of the PET volume to the patients’ MRI. Two atlases were applied in two separate processing pipelines, namely the AAL-merged atlas and Centiloid atlas. All images in the AAL pipeline were processed manually, each volume of interest (VOI) was based on the segmented grey matter from the MRI, and region-based voxel-wise PVC was applied (18). Smaller VOIs were combined to create larger volume-weighted VOIs. For further details of volume merging please refer to the Appendix 1. All segmented grey matter was included in the composite VOI, except for regions covering subcortical grey matter (caudate, putamen, thalamus). The settings for the Centiloid pipeline were applied as described by the manufacturer (19). Visual inspection of VOI placement was used for quality control. SUVR normalized to the whole cerebellum was converted to CL using the equation from the recently published research article validating PMOD for obtaining CL (14).
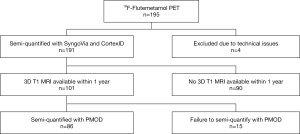
Details of the PMOD processing steps that were performed for this study are outlined in Appendix 1 and are based on previous experience as well as the user manuals (17,19). An overview of differences between software may be inspected in Appendix 1.
Visual classification of 18F-flutemetamol uptake
All 18F-flutemetamol PET images were visually classified, blinded from all clinical information, by one nuclear medicine physician (EGM) with more than seven years of experience with PET neuroimaging. This visual classification was compared to the image report in the patient’s medical record, which was a consensus read performed by at least two nuclear medicine physicians with experience interpreting 18F-flutemetamol PET. If there was discrepancy between the study-specific classification and the classification in the image report another reader (MER) classified the images, and the majority read was used for further analyses. SyngoVia was used for image interpretation and all nuclear medicine physicians interpreting 18F-flutemetamol PET had completed the electronic image reader training program (6). With this validated method, an 18F-flutemetamol PET scan is regarded as positive when at least one of the 5 bilateral regions (frontal, lateral temporal, lateral parietal, posterior cingulate/precuneus, and striatum) show increased cortical uptake, using pons as reference. If no regions show increased cortical uptake the image is regarded as negative. The regional visual classification was performed by assessing each of the five regions separately.
Clinical evaluation
As described previously (20), all patients were assessed according to a standardized and comprehensive protocol including detailed information from the patients and the caregivers about symptoms, previous disorders, use of medication, and demographic information (21). The cognitive function was assessed by several cognitive tests, including the Mini-Mental State Examination (MMSE) (22), the Consortium to Establish a Registry of Alzheimer’s Disease (CERAD) 10-item word list and constructional praxis exercise (23), the Clock Drawing Test (CDT) (24), the Trail Making Tests A and B (TMT A and B) (25), the animal-naming test, the Controlled Oral Word Association Test (COWAT-FAS test) (26-28) and the 15-word short form of the Boston Naming Test (BNT) (29). For this study, the severity of the cognitive impairment was scored by an experienced rater (THE) using the Clinical Dementia Rating scale (CDR) (30). Diagnosis and staging of cognitive impairment were made by two experienced memory clinic physicians (THE and ABK). Diagnoses were made retrospectively based on all available information in medical records, in time-proximity to the PET examination. A third experienced physician was consulted in equivocal cases. All patients were assessed for clinical etiology, hereafter referred to as clinical diagnosis, and stage (subjective cognitive decline (SCD), mild cognitive impairment (MCI), or dementia). SCD was diagnosed using the criteria from the Subjective Cognitive Decline Initiative (31). MCI or dementia, as well as clinical AD diagnoses, were based on the National Institute of Aging and the Alzheimer’s Association (NIA-AA)-criteria from 2011 (3,4). According to the NIA-AA 2011 criteria (3), AD mixed is used as a clinical diagnosis when a patient meets all core clinical criteria of AD but has evidence of concomitant disease (cerebrovascular disease, dementia with Lewy bodies, neurological disease, etc.). AD mixed was diagnosed, when appropriate, to all stages of cognitive impairment and not limited to diagnose dementia patients. All other diagnoses were made using the diagnostic criteria as follows: vascular dementia (32), frontotemporal dementia (33), primary progressive aphasia (34), Parkinson’s disease dementia (35), dementia with Lewy bodies (36) and other Parkinson plus disorders (37-40). Patients that did not fit the criteria for any of the above-mentioned disorders or had clear evidence of other diagnoses, were classified as having a non-neurodegenerative disease.
Statistical analyses
Statistical analyses were performed with IBM SPSS®, version 26 (IBM Corp., Armonk, NY, USA), STATA (version 16.1, StataCorp LLC, Texas, USA), and selected plots were created using Python version 3.6.8 (Python Software Foundation, Beaverton, OR, USA) using the following packages: Jupyter Notebook (v6.0.3), Pandas (v1.0.3), Matplotlib (v3.2.1) and Seaborn (v0.10.1). Excel 2016, version 16.34 (Microsoft Corp., Redmond, WA, USA) was used to calculate Youden’s indices.
Descriptive statistics were performed on all variables to assess mean, standard deviation (SD), and confidence interval. The distribution of parameters was assessed with histograms, QQ-plots, and Shapiro-Wilk tests. Cohen’s κ was calculated for assessing interrater agreement of visual 18F-flutemetamol classifications. Receiver operating characteristics (ROC) curves were used to compare composite SUVRs and CL to visual classification and to compare regional SUVRs to regional visual classifications. Wilcoxon signed-rank test was performed to assess differences between composite SUVRpons and SUVRcer from SyngoVia, CortexID, and PMOD. Related samples sign test was performed to assess differences between regional SUVRpons and SUVRcer from SyngoVia, CortexID, and PMOD. Youden’s indices were calculated and used to inspect optimal thresholds. Correlations between CL, SUVRpons, and SUVRcer across software were assessed with Pearson correlation coefficients.
False negatives (FN) were used to describe patients with visually positive PET and SUVR below the derived thresholds and false positives (FP) were used to describe patients with visually negative PET and SUVR above the derived thresholds. Patients with clinical diagnoses with expected amyloid pathology, such as in AD, AD mixed, and logopenic variant of primary progressive aphasia were included in the AD group (41,42). The remaining patients were included in the non-AD-group. Mann-Whitney U test was performed to assess for differences in median SUVRcer, SUVRpons, and CL between the AD-group and the non-AD-group. Binomial logistic regression was performed to assess the likelihood of AD diagnosis from the different semi-quantitative measures, with adjustment for age and sex.
All analyses performed with CL, SUVRpons, and SUVRcer from PMOD were performed on the subgroup of 86 patients. All analyses performed with SUVRpons and SUVRcer from CortexID and SyngoVia were performed on the whole group of 191 patients. Results were considered significant if P<0.05.
Results
Patient characteristics are summarized in Table 1. There was a mean of 67.1 days (SD 151.9) between PET and MRI. Interrater agreement between the visual classification performed for this study and the visual classification from the clinical report (n=191) gave a Cohen’s κ of 0.94 (SE 0.26) with disagreement in image classification in six patients. The SUVRpons ranged from 0.31 to 1.09 in SyngoVia (n=191), from 0.32 to 1.09 in CortexID (n=191) and from 0.42 to 1.08 in PMOD (n=86). The SUVRcer ranged from 1.11 to 3.69 in SyngoVia (n=191), from 1.04 to 2.9 in CortexID (n=191) and from 1.11 to 3.04 in PMOD (n=86). CL ranged from −16.30 to 153.26 (n=86). Distribution of SUVRs and CL for patients eligible for semi-quantification with all three software (n=86) are displayed in Figure 2.
Table 1
Patient characteristics | Values | Stage of disease | ||
---|---|---|---|---|
SCI, n | MCI, n | Dementia, n | ||
Females, n | 84 | – | – | – |
Males, n | 107 | – | – | – |
Age (years), mean (min–max, SD) | 67.2 (42–82, 7.9) | – | – | – |
Age (years) females, mean (SD) | 67.0 (7.9) | – | – | – |
Age (years) males, mean (SD) | 67.3 (8.0) | – | – | – |
Visual classification 18F-Flutemetamol PET | ||||
Positive, n | 108 | – | – | – |
Negative, n | 83 | – | – | – |
Cognitive tests | ||||
MMSE, mean (SD)† | 25 (4.5) | – | – | – |
CDR-SOB, mean (SD)‡ | 3.5 (2.7) | – | – | – |
Clinical diagnoses, n (%) | ||||
Alzheimer’s disease | 105 (55.0) | 1 | 25 | 79 |
Vascular dementia | 13 (6.8) | 0 | 3 | 10 |
Frontotemporal lobar degeneration | 4 (2.1) | 0 | 0 | 4 |
Primary progressive aphasia | 9 (4.7) | 0 | 3 | 6 |
Lewy body dementia | 7 (3.7) | 0 | 0 | 7 |
Parkinson disease dementia | 1 (0.5) | 0 | 0 | 1 |
Other Parkinson plus neurodegeneration | 3 (1.6) | 0 | 1 | 2 |
Non-neurodegenerative disease | 49 (25.6) | 17 | 26 | 6 |
†, missing in 24 patients within 1 year from PET; ‡, missing in 7 patients within 1 year from PET; §, including Alzheimer’s disease and mixed Alzheimer’s disease. n, number of patients; y, years; SD, standard deviation; MMSE, mini mental status examination; CDR-SOB, clinical dementia rating - sum of boxes; SCI, subjective cognitive impairment; MCI, mild cognitive impairment.
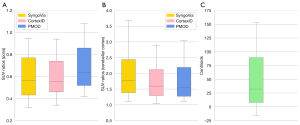
SUVR against visual classification
ROC curves with composite SUVRpons, composite SUVRcer, and CL against visual classification showed high AUC (>0.98) for all software (Table 2). ROC curves with regional SUVRpons and SUVRcer (frontal, temporal, parietal, and posterior cingulate/precuneus) from SyngoVia, Cortex ID, and PMOD against regional visual classification showed high AUC (>0.96) for all software. Please refer to the Appendix 1 for further details of AUCs from regional classifications.
Table 2
Semi-quantitative measure | Software | n | Threshold | Sensitivity | Specificity | FN/FP | AUC |
---|---|---|---|---|---|---|---|
SUVRpons | SyngoVia | 191 | 0.537 | 98% | 100% | 2/0 | 0.992 |
0.418 | 100% | 43% | |||||
CortexID | 191 | 0.550 | 96% | 100% | 4/0 | 0.990 | |
0.425 | 100% | 37% | |||||
PMOD | 86 | 0.637 | 96% | 98% | 2/1 | 0.995 | |
0.583 | 100% | 91% | |||||
SUVRcer | SyngoVia | 191 | 1.869 | 94% | 100% | 6/0 | 0.989 |
1.513 | 100% | 69% | |||||
CortexID | 191 | 1.640 | 93% | 100% | 8/0 | 0.992 | |
1.395 | 100% | 78% | |||||
PMOD | 86 | 1.556 | 93% | 98% | 3/1 | 0.986 | |
1.366 | 100% | 71% | |||||
Centiloids | PMOD | 86 | 39.578 | 93% | 100% | 3/0 | 0.995 |
23.332 | 100% | 88% |
n, number of patients; FN, false negatives; FP, false positives; AUC, area under the curve; SUVRpons, standardized uptake value ratio normalized to pons; SUVRcer, standardized uptake value ratio normalized to cerebellar cortex.
Thresholds from different software
Thresholds corresponding to the highest Youden’s index showed different values for each software and each normalization region, although SyngoVia and CortexID showed similar numerical SUVRpons thresholds (Table 2). Only PMOD with SUVRpons and CL was able to maintain specificities above 80% (PMOD 91% and CL 88%) with a fixed 100% sensitivity.
Comparison of SUVR between software
Wilcoxon signed-rank test showed no significant median differences between composite SUVRcer from CortexID and PMOD. For all other composite SUVR, there were significant median differences between the software (Table 3). SUVRs and CL were not normally distributed. The correlation was assessed with scatterplots and there were linear relationships between SUVRpons and SUVRcer between the three software as well as between CL and SUVRs from SyngoVia and CortexID (Figures 3,4). Pearson correlation coefficients (r) showed a very strong correlation (P<0.001) between SUVRpons and SUVRcer from all three software, and between CL and the SUVRs from SyngoVia and CortexID.
Table 3
Composite ratios | Software compared | n | Negative diff. | Positive diff. | Ties | P value |
---|---|---|---|---|---|---|
SUVRpons | SyngoVia - PMOD | 86 | 84 | 2 | 0 | <0.001 |
CortexID - PMOD | 86 | 81 | 5 | 0 | <0.001 | |
SyngoVia - CortexID | 86 | 52 | 34 | 0 | 0.024 | |
191 | 111 | 80 | 0 | 0.006 | ||
SUVRcer | SyngoVia - PMOD | 86 | 8 | 78 | 0 | <0.001 |
CortexID - PMOD | 86 | 48 | 38 | 0 | 0.933 | |
SyngoVia - CortexID | 86 | 6 | 80 | 0 | <0.001 | |
191 | 16 | 175 | 0 | <0.001 |
P values were obtained through Wilcoxon signed rank tests. n, number of patients; diff, difference; SUVRpons, SUVR normalized to pons; SUVRcer, SUVR normalized to cerebellar cortex.

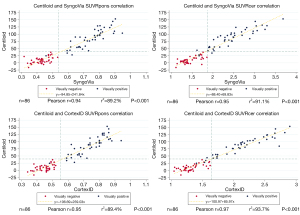
Sign tests for exploring differences in regional SUVR showed no significant median differences in SUVRcer for the anterior cingulate region (P=0.332) and frontal region (P=0.332) between SyngoVia and PMOD and no significant differences in SUVRcer for the parietal region (P=0.332) and the temporal region (P=0.914) between CortexID and PMOD. SUVRcer for the posterior cingulate/precorneal region as well as SUVRpons for all regions showed significant differences (P<0.001) between the three software. For complete results of the related samples sign tests please refer to the Appendix 1. Figure 5 gives an overview of the distribution of regional SUVRs from the different software.
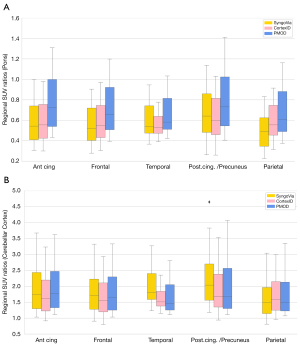
Differences in SUVR and CL between clinical diagnoses
Mann Whitney U tests were run to determine if there were differences in SUVR and CL between patients with expected amyloid pathology (AD-group) and patients without expected amyloid pathology (non-AD-group). The distribution of SUVR and CL was different, as assessed by visual inspection. The mean rank of SUVRcer and SUVRpons from all three software and CL from PMOD was significantly different between the AD-group and the non-AD-group (P<0.001) (Figure 6). Multivariate binomial logistic regression models showed that higher SUVRpons and SUVRcer from all software, and CL, were significantly associated with AD diagnosis (P>0.001), also after adjustment for age and sex. An overview of mean SUVRs and CL across each diagnosis groups is included in the Appendix 1.
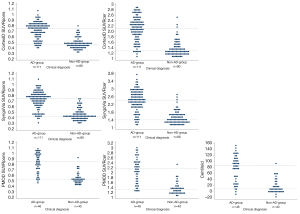
Discussion
Our study demonstrates that SUVR derived from SyngoVia, CortexID, and PMOD, as well as CL, were equally good for discriminating visually positive and negative 18F-flutemetamol PET images. There were significant median differences in composite SUVRs obtained from the different software, except for SUVRcer from CortexID and PMOD which showed no significant differences. CortexID gave slightly higher SUVRpons compared to SyngoVia, and SyngoVia gave higher SUVRcer compared to CortexID. PMOD gave higher SUVRpons compared to CortexID and SyngoVia, and slightly higher SUVRcer compared to SyngoVia.
We found a strong correlation between SUVR from all software as well as CL, demonstrated by the high Pearson correlation coefficients. Thresholds from each software are provided and may be used to aid visual classification in equivocal cases. The mean rank of SUVR and CL was significantly different between patients with expected amyloid pathology and patients without expected amyloid pathology. Logistic regression analyses showed that higher SUVRs and CL were significantly associated with AD-diagnosis, also after adjusting for age and sex.
We used default settings for obtaining SUVR from SyngoVia and CortexID, and a recently validated method for obtaining Centiloid from PMOD (14). The PMOD processing with MRI using the AAL-merged atlas is not a standardized method. Different choices during the image processing might give different results, which is undesirable in clinical routine. PMOD semi-quantification was consequently included for comparison as this software offers a more detailed delineation of cortical structures as well as facilitating PVC. The present study indicates, however, that this detailed semi-quantification is not necessary for supporting the visual classification of positive or negative 18F-flutemetamol PET. On the contrary, when monitoring the amyloid burden over time or in response to an intervention, it is desirable with a more precise and detailed approach.
Our results support previous notions that SUVR thresholds are specific for each software and tracer used (43,44). Using a threshold derived from software A to classify images semi-quantified with software B, is generally inappropriate. This is an important issue to be aware of. If thresholds are applied across software a preceding validation is important. The CL scale, however, is meant to represent a common quantitative output value across different tracers (13). Different staging systems based on amyloid PET have been proposed to obtain an estimate of the stage of histopathological changes. Staging systems based on SUVR including striatal regions (45-47) and not including striatal regions (48) as well as staging based on the number of positive regions by visual classification (49) have been evaluated. Subcortical structures are not included in SyngoVia or CortexID, however, the anterior striatum is included in the CL atlas. Visual classification, which includes striatum, is still the gold standard for evaluating amyloid PET. One may argue that inclusion of striatum in semi-quantification would be valuable, however, this is still under investigation. Striatum’s role in AD has been suggested to provide a more accurate clinicopathological diagnosis (50) as well as serving as the earliest localization for Aβ deposition in individuals with autosomal dominant AD mutations (51). Nevertheless, a standardized approach for semi-quantification, preferably including striatum, and implemented in commonly used software, would facilitate further exploration of optimal staging systems.
Previously published SUVRpons thresholds from CortexID by Thurfjell et al. varies from 0.56 to 0.62, depending on the thickness of VOIs applied in the software (9). The narrow VOI set, which corresponds to the template applied in CortexID, gave a threshold of 0.58 (9,12), very close to the CortexID threshold of 0.55 in the current study. Interestingly, our CortexID threshold is very similar to the derived SyngoVia SUVRpons threshold of 0.54. In the study by Thurfjell et al. (9), the histopathological assessment postmortem was used as the standard of truth as opposed to visual classification in the present study. Cortical 18F-flutemetamol PET signal has shown to be highly correlated to neuritic plaque burden assessed postmortem (1,6,52,53), making thresholds based on visual classification a reliable approach in a memory clinic population.
Our threshold of 39.6 CL is higher than previously published, ranging from CL 12.2 to 24.4 (54-57), where histopathology postmortem was used as the standard of truth. In a recent study by Collij et al. a threshold of CL 17 was found to be optimal compared to visual classification in a group of 497 patients, in which 352 patients were cognitively unimpaired individuals with increased risk of AD (49). We believe the difference in CL thresholds between this study and ours is mainly due to different populations. However, the presented CL threshold is similar to a recently published threshold of 40 CL with visual classification as the standard of truth (58).
No strict consensus has been reached regarding which region to use for reference when calculating SUVRs. The choice of reference region impacts the numerical value of SUVRs due to different degrees of white and grey matter in different reference regions. 18F-flutemetamol has high non-specific binding to white matter. Using pons as a reference will give the lowest SUVR, in our material, ranging from 0.3 to 1.1. Using the whole cerebellum will give a SUVR somewhat higher, due to the mix of white and grey matter. Using cerebellar cortex will give the highest SUVR due to low 18F-flutemetamol uptake, ranging in our dataset from 1.0 to 3.7. Both pons and cerebellar cortex may exhibit amyloid plaques, however, normally not until the latest phases of amyloid deposition (47,59).
The variation in SUVR between software is likely multifactorial. The atlases and VOIs applied in the three software are different (Figure 7), as described in the methods. SyngoVia uses the AAL-atlas and applies a VOI set that has been adopted from Fleisher et al. (8). CortexID uses an adaptive template method using a VOI template previously described (9,12). SUVRs from SyngoVia and CortexID are not volume weighted. In PMOD we used the AAL atlas and each VOI is based on the segmented grey matter from the MRI. We applied both normalization to the cerebellar cortex and pons in SyngoVia, CortexID, and PMOD. To obtain SUVRs for subsequent calculation of CL, another atlas is used, with obligatory normalization against the whole cerebellum. The composite SUVRs are consequently based on different volumes for each software. In PMOD, only the segmented grey matter is included in the VOI set. For the other software, some degree of white matter will occasionally be included in the VOIs, due to individual differences in gyral pattern. Furthermore, PVC was only applied in the AAL pipeline in PMOD. Nevertheless, the explored software were equally good in discriminating visually positive from negative images in a memory clinic population. As many centers exhibit more than one software for semi-quantification, this finding may be useful. Whether this statement is true also for individuals in the earliest pre-symptomatic stages of the AD continuum should be further explored. Asymptomatic patients are rarely encountered in memory clinics and are thus not referred for amyloid imaging in clinical routine (60), which makes these study questions difficult to answer when investigating a naturalistic memory clinic cohort.
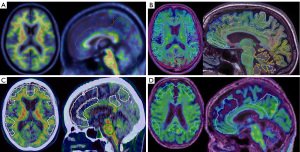
We found significant differences in the mean rank of SUVRpons, SUVRcer, and CL between the AD-group and the non-AD group. Problems of circularity may be suspected when considering that the results of the 18F-flutemetamol PET may have influenced the clinical diagnosis, however, the 2011 diagnostic criteria applied in the present study advise that biomarkers are used for support only.
Limitations of this study are the lack of a control group and the lack of histopathology as the standard of truth for PET, to ensure that the thresholds reliably reflect amyloid deposition. The deviation in image delay time is acknowledged as a limitation, possibly violating equilibrium conditions. All patients were imaged with the same scanner during clinical routine, with a relatively low variation in the remaining examination parameters. The MRIs were performed on different MRI scanners, reflecting the clinical situation with images obtained at different referring hospitals. The quality of MRI was manually assessed by visual inspection and post-processing in PMOD was canceled if the segmentation results appeared distorted. We acknowledge this as a weakness although we emphasize that MRI was used only for segmentation in PMOD.
Conclusions
SUVR from different software performed equally well in discriminating visually positive from negative 18F-flutemetamol PET images. Although semi-quantitative measures from different software showed a very high correlation, there were statistically significant differences in SUVR between them, and thresholds should be considered software specific. Thresholds may be useful for supporting visual classification in clinical routine when semi-quantification is performed with the same software as the threshold is derived from. We demonstrate significant differences in the mean rank of SUVRs and CL between clinical diagnoses further supporting the usefulness of amyloid PET in a memory clinic setting.
Acknowledgments
We would like to acknowledge the Norwegian registry of persons assessed for cognitive symptoms (NorCog) for providing access to the patient data.
Funding: This work was supported by Civitan Norway Research Foundation for Alzheimer’s disease and Oslo University Hospital.
Footnote
Conflicts of Interest: All authors have completed the ICMJE uniform disclosure form (available at https://dx.doi.org/10.21037/qims-21-188). HLS has received consultancy fees from Siemens Healthineers. THE has been a rater in two clinical trials (Roche BN29553 and Boehringer-Ingelheim 1346.0023) outside the submitted work. ABK has been a national coordinator and principal investigator in three clinical trials (Roche BN29553, Boehringer-Ingelheim 1346.0023 and Novo Nordisk, EVOKE NN6535-4730) outside the submitted work. The other authors have no conflicts of interest to declare.
Ethical Statement: The authors are accountable for all aspects of the work in ensuring that questions related to the accuracy or integrity of any part of the work are appropriately investigated and resolved. The study was conducted following the Declaration of Helsinki (as revised in 2013). The Regional Ethics Committee for medical research in the South-East of Norway (REC 2017/1929) and the Data Protection Officer at our institution approved the study. Informed consent was obtained from all individual participants and all participants were deemed to have sufficient cognitive capacity to consent at the time of inclusion.
Open Access Statement: This is an Open Access article distributed in accordance with the Creative Commons Attribution-NonCommercial-NoDerivs 4.0 International License (CC BY-NC-ND 4.0), which permits the non-commercial replication and distribution of the article with the strict proviso that no changes or edits are made and the original work is properly cited (including links to both the formal publication through the relevant DOI and the license). See: https://creativecommons.org/licenses/by-nc-nd/4.0/.
References
- Curtis C, Gamez JE, Singh U, Sadowsky CH, Villena T, Sabbagh MN, et al. Phase 3 trial of flutemetamol labeled with radioactive fluorine 18 imaging and neuritic plaque density. JAMA Neurol 2015;72:287-94. [Crossref] [PubMed]
- Minoshima S, Drzezga AE, Barthel H, Bohnen N, Djekidel M, Lewis DH, Mathis CA, McConathy J, Nordberg A, Sabri O, Seibyl JP, Stokes MK, Van Laere K. SNMMI Procedure Standard/EANM Practice Guideline for Amyloid PET Imaging of the Brain 1.0. J Nucl Med 2016;57:1316-22. [Crossref] [PubMed]
- McKhann GM, Knopman DS, Chertkow H, Hyman BT, Jack CR Jr, Kawas CH, Klunk WE, Koroshetz WJ, Manly JJ, Mayeux R, Mohs RC, Morris JC, Rossor MN, Scheltens P, Carrillo MC, Thies B, Weintraub S, Phelps CH. The diagnosis of dementia due to Alzheimer's disease: recommendations from the National Institute on Aging-Alzheimer's Association workgroups on diagnostic guidelines for Alzheimer's disease. Alzheimers Dement 2011;7:263-9. [Crossref] [PubMed]
- Albert MS, DeKosky ST, Dickson D, Dubois B, Feldman HH, Fox NC, Gamst A, Holtzman DM, Jagust WJ, Petersen RC, Snyder PJ, Carrillo MC, Thies B, Phelps CH. The diagnosis of mild cognitive impairment due to Alzheimer's disease: recommendations from the National Institute on Aging-Alzheimer's Association workgroups on diagnostic guidelines for Alzheimer's disease. Alzheimers Dement 2011;7:270-9. [Crossref] [PubMed]
- Jack CR Jr, Bennett DA, Blennow K, Carrillo MC, Dunn B, Haeberlein SB, et al. NIA-AA Research Framework: Toward a biological definition of Alzheimer's disease. Alzheimers Dement 2018;14:535-62. [Crossref] [PubMed]
- Buckley CJ, Sherwin PF, Smith AP, Wolber J, Weick SM, Brooks DJ. Validation of an electronic image reader training programme for interpretation of 18Fflutemetamol β-amyloid PET brain images. Nucl Med Commun 2017;38:234-41. [Crossref] [PubMed]
- Klunk WE, Koeppe RA, Price JC, Benzinger TL, Devous MD, Sr., Jagust WJ, Johnson KA, Mathis CA, Minhas D, Pontecorvo MJ, Rowe CC, Skovronsky DM, Mintun MA. The Centiloid Project: standardizing quantitative amyloid plaque estimation by PET. Alzheimers Dement 2015;11:1-15.e1-4.
- Fleisher AS, Chen K, Liu X, Roontiva A, Thiyyagura P, Ayutyanont N, Joshi AD, Clark CM, Mintun MA, Pontecorvo MJ, Doraiswamy PM, Johnson KA, Skovronsky DM, Reiman EM. Using positron emission tomography and florbetapir F18 to image cortical amyloid in patients with mild cognitive impairment or dementia due to Alzheimer disease. Arch Neurol 2011;68:1404-11. [Crossref] [PubMed]
- Thurfjell L, Lilja J, Lundqvist R, Buckley C, Smith A, Vandenberghe R, Sherwin P. Automated quantification of 18F-flutemetamol PET activity for categorizing scans as negative or positive for brain amyloid: concordance with visual image reads. J Nucl Med 2014;55:1623-8. [Crossref] [PubMed]
- Lilja J, Thurfjell L, Sörensen J. Visualization and Quantification of 3-Dimensional Stereotactic Surface Projections for 18F-flutemetamol PET Using Variable Depth. J Nucl Med 2016;57:1078-83. [Crossref] [PubMed]
- Lilja J, Leuzy A, Chiotis K, Savitcheva I, Sörensen J, Nordberg A. Spatial Normalization of 18F-flutemetamol PET Images Using an Adaptive Principal-Component Template J Nucl Med 2019;60:285-91. [Crossref] [PubMed]
- Lundqvist R, Lilja J, Thomas BA, Lötjönen J, Villemagne VL, Rowe CC, Thurfjell L. Implementation and validation of an adaptive template registration method for 18F-flutemetamol imaging data. J Nucl Med 2013;54:1472-8. [Crossref] [PubMed]
- Cho SH, Choe YS, Kim HJ, Jang H, Kim Y, Kim SE, Kim SJ, Kim JP, Jung YH, Kim BC, Baker SL, Lockhart SN, Na DL, Park S, Seo SW. A new Centiloid method for 18F-florbetaben and 18F-flutemetamol PET without conversion to PiB. Eur J Nucl Med Mol Imaging 2020;47:1938-48. [Crossref] [PubMed]
- Battle MR, Pillay LC, Lowe VJ, Knopman D, Kemp B, Rowe CC, Doré V, Villemagne VL, Buckley CJ. Centiloid scaling for quantification of brain amyloid with 18Fflutemetamol using multiple processing methods. EJNMMI Res 2018;8:107. [Crossref] [PubMed]
- Hammers DB, Atkinson TJ, Dalley BCA, Suhrie KR, Horn KP, Rasmussen KM, Beardmore BE, Burrell LD, Duff K, Hoffman JM. Amyloid Positivity Using 18FFlutemetamol-PET and Cognitive Deficits in Nondemented Community-Dwelling Older Adults. Am J Alzheimers Dis Other Demen 2017;32:320-8. [Crossref] [PubMed]
- Chincarini A, Peira E, Morbelli S, Pardini M, Bauckneht M, Arbizu J, et al. Semi-quantification and grading of amyloid PET: A project of the European Alzheimer's Disease Consortium (EADC). Neuroimage Clin 2019;23:101846 [Crossref] [PubMed]
- PMOD Technologies LLC. PMOD Neuro Tool (PNEURO), User manual Version 4.0. In: User Manual Version 4.0. Available online: https://www.pmod.com/web/?page_id=702
- Thomas BA, Erlandsson K, Modat M, Thurfjell L, Vandenberghe R, Ourselin S, Hutton BF. The importance of appropriate partial volume correction for PET quantification in Alzheimer's disease. Eur J Nucl Med Mol Imaging 2011;38:1104-19. [Crossref] [PubMed]
- PMOD Technologies LLC. Centiloid Analysis. In: PMOD application note, version 3.9. PMOD Technologies LLC. Available online: https://www.pmod.com/files/pdf/applications/Centiloid_AppNote.pdf
- Müller EG, Edwin TH, Stokke C, Navelsaker SS, Babovic A, Bogdanovic N, Knapskog AB, Revheim ME. Amyloid-β PET-Correlation with cerebrospinal fluid biomarkers and prediction of Alzheimer’s disease diagnosis in a memory clinic. PLoS One 2019;14:e0221365 [Crossref] [PubMed]
- Brækhus A, Ulstein I, Wyller TB, Engedal K. The Memory Clinic--outpatient assessment when dementia is suspected. Tidsskr Nor Laegeforen 2011;131:2254-7. [PubMed]
- Folstein MF, Folstein SE, McHugh PR. "Mini-mental state". A practical method for grading the cognitive state of patients for the clinician. J Psychiatr Res 1975;12:189-98. [Crossref] [PubMed]
- Morris JC, Heyman A, Mohs RC, Hughes JP, van Belle G, Fillenbaum G, Mellits ED, Clark C. The Consortium to Establish a Registry for Alzheimer's Disease (CERAD). Part I. Clinical and neuropsychological assessment of Alzheimer's disease. Neurology 1989;39:1159-65. [Crossref] [PubMed]
- Shulman KI. Clock-drawing: is it the ideal cognitive screening test? Int J Geriatr Psychiatry 2000;15:548-61. [Crossref] [PubMed]
- Reitan RM. Validity of the Trail Making Test as an indicator of organic brain damage. Percept Mot Skills 1958;8:271-6. [Crossref]
- Benton A, Hamsher K. Multilingual aphasia examination manual. Iowa City: University of Iowa, 1978.
- Lezak MD, Howieson DB, Loring DW, Fischer JS. Neuropsychological assessment. USA: Oxford University Press, 2004.
- Malek-Ahmadi M, Small BJ, Raj A. The diagnostic value of controlled oral word association test-FAS and category fluency in single-domain amnestic mild cognitive impairment. Dement Geriatr Cogn Disord 2011;32:235-40. [Crossref] [PubMed]
- Goodglass H, Kaplan E, Weintraub S. Boston naming test. Philadelphia, PA: Lea & Febiger, 1983.
- Hughes CP, Berg L, Danziger WL, Coben LA, Martin RL. A new clinical scale for the staging of dementia. Br J Psychiatry 1982;140:566-72. [Crossref] [PubMed]
- Jessen F, Amariglio RE, van Boxtel M, Breteler M, Ceccaldi M, Chételat G, et al. A conceptual framework for research on subjective cognitive decline in preclinical Alzheimer's disease. Alzheimers Dement 2014;10:844-52. [Crossref] [PubMed]
- Sachdev P, Kalaria R, O'Brien J, Skoog I, Alladi S, Black SE, Blacker D, Blazer DG, Chen C, Chui H, Ganguli M, Jellinger K, Jeste DV, Pasquier F, Paulsen J, Prins N, Rockwood K, Roman G, Scheltens PInternationlal Society for Vascular Behavioral and Cognitive Disorders. Diagnostic criteria for vascular cognitive disorders: a VASCOG statement. Alzheimer Dis Assoc Disord 2014;28:206-18. [Crossref] [PubMed]
- Rascovsky K, Hodges JR, Knopman D, Mendez MF, Kramer JH, Neuhaus J, et al. Sensitivity of revised diagnostic criteria for the behavioural variant of frontotemporal dementia. Brain 2011;134:2456-77. [Crossref] [PubMed]
- Gorno-Tempini ML, Hillis AE, Weintraub S, Kertesz A, Mendez M, Cappa SF, Ogar JM, Rohrer JD, Black S, Boeve BF, Manes F, Dronkers NF, Vandenberghe R, Rascovsky K, Patterson K, Miller BL, Knopman DS, Hodges JR, Mesulam MM, Grossman M. Classification of primary progressive aphasia and its variants. Neurology 2011;76:1006-14. [Crossref] [PubMed]
- Emre M, Aarsland D, Brown R, Burn DJ, Duyckaerts C, Mizuno Y, et al. Clinical diagnostic criteria for dementia associated with Parkinson's disease. Mov Disord 2007;22:1689-707; quiz 1837. [Crossref] [PubMed]
- McKeith IG, Boeve BF, Dickson DW, Halliday G, Taylor JP, Weintraub D, et al. Diagnosis and management of dementia with Lewy bodies: Fourth consensus report of the DLB Consortium. Neurology 2017;89:88-100. [Crossref] [PubMed]
- Armstrong MJ, Litvan I, Lang AE, Bak TH, Bhatia KP, Borroni B, Boxer AL, Dickson DW, Grossman M, Hallett M, Josephs KA, Kertesz A, Lee SE, Miller BL, Reich SG, Riley DE, Tolosa E, Tröster AI, Vidailhet M, Weiner WJ. Criteria for the diagnosis of corticobasal degeneration. Neurology 2013;80:496-503. [Crossref] [PubMed]
- Gilman S, Wenning GK, Low PA, Brooks DJ, Mathias CJ, Trojanowski JQ, Wood NW, Colosimo C, Dürr A, Fowler CJ, Kaufmann H, Klockgether T, Lees A, Poewe W, Quinn N, Revesz T, Robertson D, Sandroni P, Seppi K, Vidailhet M. Second consensus statement on the diagnosis of multiple system atrophy. Neurology 2008;71:670-6. [Crossref] [PubMed]
- Litvan I. Diagnosis and management of progressive supranuclear palsy. Semin Neurol 2001;21:41-8. [Crossref] [PubMed]
- Litvan I, Agid Y, Calne D, Campbell G, Dubois B, Duvoisin RC, Goetz CG, Golbe LI, Grafman J, Growdon JH, Hallett M, Jankovic J, Quinn NP, Tolosa E, Zee DS. Clinical research criteria for the diagnosis of progressive supranuclear palsy (Steele-Richardson-Olszewski syndrome): report of the NINDS-SPSP international workshop. Neurology 1996;47:1-9. [Crossref] [PubMed]
- Mesulam M, Wicklund A, Johnson N, Rogalski E, Léger GC, Rademaker A, Weintraub S, Bigio EH. Alzheimer and frontotemporal pathology in subsets of primary progressive aphasia. Ann Neurol 2008;63:709-19. [Crossref] [PubMed]
- Santos-Santos MA, Rabinovici GD, Iaccarino L, Ayakta N, Tammewar G, Lobach I, et al. Rates of Amyloid Imaging Positivity in Patients With Primary Progressive Aphasia. JAMA Neurol 2018;75:342-52. [Crossref] [PubMed]
- Jack CR Jr, Wiste HJ, Weigand SD, Therneau TM, Lowe VJ, Knopman DS, Gunter JL, Senjem ML, Jones DT, Kantarci K, Machulda MM, Mielke MM, Roberts RO, Vemuri P, Reyes DA, Petersen RC. Defining imaging biomarker cut points for brain aging and Alzheimer's disease. Alzheimers Dement 2017;13:205-16. [Crossref] [PubMed]
- Su Y, Flores S, Wang G, Hornbeck RC, Speidel B, Joseph-Mathurin N, et al. Comparison of Pittsburgh compound B and florbetapir in cross-sectional and longitudinal studies. Alzheimers Dement (Amst) 2019;11:180-90. [Crossref] [PubMed]
- Grothe MJ, Barthel H, Sepulcre J, Dyrba M, Sabri O, Teipel SJAlzheimer's Disease Neuroimaging Initiative. In vivo staging of regional amyloid deposition. Neurology 2017;89:2031-8. [Crossref] [PubMed]
- Hanseeuw BJ, Betensky RA, Mormino EC, Schultz AP, Sepulcre J, Becker JA, Jacobs HIL, Buckley RF, LaPoint MR, Vannini P, Donovan NJ, Chhatwal JP, Marshall GA, Papp KV, Amariglio RE, Rentz DM, Sperling RA, Johnson KAHarvard Aging Brain Study. PET staging of amyloidosis using striatum. Alzheimers Dement 2018;14:1281-92. [Crossref] [PubMed]
- Thal DR, Beach TG, Zanette M, Lilja J, Heurling K, Chakrabarty A, Ismail A, Farrar G, Buckley C, Smith APL. Estimation of amyloid distribution by 18Fflutemetamol PET predicts the neuropathological phase of amyloid β-protein deposition. Acta Neuropathol 2018;136:557-67. [Crossref] [PubMed]
- Mattsson N, Palmqvist S, Stomrud E, Vogel J, Hansson O. Staging β-Amyloid Pathology With Amyloid Positron Emission Tomography. JAMA Neurol 2019;76:1319-29. [Crossref] [PubMed]
- Collij LE, Salvadó G, Shekari M, Lopes Alves I, Reimand J, Wink AM, Zwan M, Niñerola-Baizán A, Perissinotti A, Scheltens P, Ikonomovic MD, Smith APL, Farrar G, Molinuevo JL, Barkhof F, Buckley CJ, van Berckel BNM, Gispert JDAMYPAD consortium. Visual assessment of 18Fflutemetamol PET images can detect early amyloid pathology and grade its extent. Eur J Nucl Med Mol Imaging 2021;48:2169-82. [Crossref] [PubMed]
- Beach TG, Thal DR, Zanette M, Smith A, Buckley C. Detection of Striatal Amyloid Plaques with 18Fflutemetamol: Validation with Postmortem Histopathology. J Alzheimers Dis 2016;52:863-73. [Crossref] [PubMed]
- Cohen AD, Landau SM, Snitz BE, Klunk WE, Blennow K, Zetterberg H. Fluid and PET biomarkers for amyloid pathology in Alzheimer's disease. Mol Cell Neurosci 2019;97:3-17. [Crossref] [PubMed]
- Ikonomovic MD, Buckley CJ, Heurling K, Sherwin P, Jones PA, Zanette M, Mathis CA, Klunk WE, Chakrabarty A, Ironside J, Ismail A, Smith C, Thal DR, Beach TG, Farrar G, Smith AP. Post-mortem histopathology underlying β-amyloid PET imaging following flutemetamol F 18 injection. Acta Neuropathol Commun 2016;4:130. [Crossref] [PubMed]
- Ikonomovic MD, Fantoni ER, Farrar G, Salloway S. Infrequent false positive 18Fflutemetamol PET signal is resolved by combined histological assessment of neuritic and diffuse plaques. Alzheimers Res Ther 2018;10:60. [Crossref] [PubMed]
- Amadoru S, Doré V, McLean CA, Hinton F, Shepherd CE, Halliday GM, Leyton CE, Yates PA, Hodges JR, Masters CL, Villemagne VL, Rowe CC. Comparison of amyloid PET measured in Centiloid units with neuropathological findings in Alzheimer's disease. Alzheimers Res Ther 2020;12:22. [Crossref] [PubMed]
- La Joie R, Ayakta N, Seeley WW, Borys E, Boxer AL, DeCarli C, et al. Multisite study of the relationships between antemortem 11CPIB-PET Centiloid values and postmortem measures of Alzheimer's disease neuropathology. Alzheimers Dement 2019;15:205-16. [Crossref] [PubMed]
- Navitsky M, Joshi AD, Kennedy I, Klunk WE, Rowe CC, Wong DF, Pontecorvo MJ, Mintun MA, Devous MD Sr. Standardization of amyloid quantitation with florbetapir standardized uptake value ratios to the Centiloid scale. Alzheimers Dement 2018;14:1565-71. [Crossref] [PubMed]
- Doré V, Bullich S, Rowe CC, Bourgeat P, Konate S, Sabri O, Stephens AW, Barthel H, Fripp J, Masters CL, Dinkelborg L, Salvado O, Villemagne VL, De Santi S. Comparison of 18F-florbetaben quantification results using the standard Centiloid, MR-based, and MR-less CapAIBL® approaches: Validation against histopathology. Alzheimers Dement 2019;15:807-16. [Crossref] [PubMed]
- Hanseeuw BJ, Malotaux V, Dricot L, Quenon L, Sznajer Y, Cerman J, Woodard JL, Buckley C, Farrar G, Ivanoiu A, Lhommel R. Defining a Centiloid scale threshold predicting long-term progression to dementia in patients attending the memory clinic: an 18F flutemetamol amyloid PET study. Eur J Nucl Med Mol Imaging 2021;48:302-10. [Crossref] [PubMed]
- Thal DR, Rüb U, Orantes M, Braak H. Phases of A beta-deposition in the human brain and its relevance for the development of AD. Neurology 2002;58:1791-800. [Crossref] [PubMed]
- Johnson KA, Minoshima S, Bohnen NI, Donohoe KJ, Foster NL, Herscovitch P, Karlawish JH, Rowe CC, Carrillo MC, Hartley DM, Hedrick S, Pappas V, Thies WH. Amyloid Imaging Taskforce. Appropriate use criteria for amyloid PET: a report of the Amyloid Imaging Task Force, the Society of Nuclear Medicine and Molecular Imaging, and the Alzheimer's Association. Alzheimers Dement 2013;9:e-1-16. [Crossref]