Application value of myocardial work technology by non-invasive echocardiography in evaluating left ventricular function in patients with chronic heart failure
Introduction
Chronic heart failure (CHF) is a severe clinical syndrome of various cardiac diseases and is associated with various interrelated structural and functional alterations of the heart (1,2). Therefore, accurate assessment of left ventricular (LV) systolic function and appropriate intervention are critical in improving the clinical management and long-term survival of patients with CHF in various clinical settings (3).
Numerous techniques have been developed to assess the LV systolic function in CHF patients to guide clinical management and prognosis. Specifically, conventional parameters, such as the LV ejection fraction (LVEF), are often used to stratify and evaluate CHF severity. However, a major limitation of LVEF, measured either by echocardiography or other modalities (such as cardiac magnetic resonance or multidetector computed tomography), is that it is dependent on preload and afterload conditions, which are influenced by heart rate and are insensitive to subtle changes in contractile reserve (4). Furthermore, the LVEF is limited in assessing the increased work of the LV myocardium (5). LV myocardial deformation imaging by Speckle-Tracking Echocardiography (STE) is a superior prognostic marker of cardiac events, providing a comprehensive quantitative assessment of myocardial function beyond the LVEF. However, some of the intrinsic limitations of STE, such as load dependency, will affect the diagnostic accuracy of myocardial function assessment due to the increased LV afterload occurring concomitantly with decreased strain (6,7). LV pressure-volume loop measured by cardiac catheterization is the gold standard in systolic function evaluation, but it is invasive (8).
Myocardial work (MW) by non-invasive echocardiography is a novel method for evaluating systolic cardiac function that accounts for deformation and afterload, potentially offering incremental value to myocardial function assessment (9-11). It is significantly correlated with cardiac metabolism, as assessed by invasive cardiac catheterization measurements (12). Nevertheless, the new MW technology application in patients with CHF has rarely been investigated in the literature.
The purpose of this study was to investigate the incremental value of the echocardiographic MW technique in evaluating LV systolic dysfunction in patients with CHF. This study hypothesizes that the MW parameters could achieve earlier diagnosis than LVEF in distinguishing patients with preserved LVEF (HFpEF) from healthy controls (HC), and the combination of MW parameters and LVEF could complementarily improve the diagnostic accuracy in classifying multiple groups of HC, HFpEF, patients with medium LVEF (HFmrEF), and patients with reduced LVEF (HFrEF).
Methods
This study was conducted following the Declaration of Helsinki (as revised in 2013) and was approved by the Ethics Committee of Henan Provincial People's Hospital. Individual consent for this retrospective analysis was waived.
Study population
A total of 155 patients with a first discharge diagnosis of CHF between December 2017 and March 2020 were consecutively identified. The patients were divided into three groups, including 54 patients with preserved LVEF (HFpEF group, LVEF ≥50%), 50 patients with medium LVEF (HFmrEF group, 40%≤ LVEF <50%), and 51 patients with reduced LVEF (HFrEF group, LVEF <40%). The LVEF used for the grouping was measured using the modified biplane Simpson method according to the guidelines issued by the American College of Cardiology (ACC)/American Heart Association (AHA) in 2017 (13), the European Society of Cardiology (ESC) in 2016 (14), and the American Society of Echocardiography (ASE)/European Association of Cardiovascular Imaging (EACVI) in 2015 (15).
In addition to meeting the relative LVEF values, the diagnostic criteria for CHF should consist of symptoms and/or signs of heart failure. HFpEF/HFmrEF also include increased brain natriuretic peptide [BNP >35 ng/L or N-terminal-proBNP (NT-proBNP) >125 ng/L], and at least one of the following: (I) LV hypertrophy and/or left atrial enlargement, and (II) abnormal diastolic function. The following indicators assessed diastolic dysfunction: left atrial volume index (LAVI) >34 mL/m2 o r E/e′≥13 or tricuspid regurgitation velocity (TRV) >2.8 m/s. Patients with significant valvular heart disease, congenital heart disease, ectopic heart rhythm, severe arrhythmia, or poor ultrasound image quality were excluded. Poor ultrasound image quality was mainly manifested as an unclear display of the endocardium or endocardium located outside the sampling frame. From a combined total of 194 baseline studies received in our center, 155 patients were suitable for quantitative analysis and were included in this report (Figure 1).
One additional group comprising 51 healthy age- and gender-matched volunteers matched in the physical examination center during the same period, referred to as the healthy control (HC) group, was included. All included volunteers were confirmed as having no abnormalities by inquiry of medical history, general physical examination, electrocardiogram, echocardiography, and various laboratory tests. Blood pressure, height, and weight were routinely measured in all subjects.
Echocardiography
Comprehensive transthoracic echocardiography (TTE) was performed in all patients using Vivid E95 ultrasound equipped with M5S 1.5–4.6 MHz transducer (GE Vingmed Ultrasound, Horten, Norway) by experienced ultrasound physicians. Two-dimensional and Dopper echocardiography data were stored on a dedicated workstation for the offline analysis (EchoPAC, GE Healthcare).
The subjects were scanned in the left lateral decubitus position, and an electrocardiogram was attached. Left atrial diameter (LAD), LV end-diastolic diameter (LVDd), inter-ventricular septal wall thickness (IVS), LV posterior wall thickness (LVPW), and LV end systolic diameter (LVDs) were obtained in the parasternal LV long-axis view. Left atrial volume was observed both in the apical four- and two-chamber view as biplane, and the left atrial volume index (LAVI) was calculated. Left ventricular mass was calculated according to the following formula: LVM (g) = {1.04[(IVS + LVDd + LVPW)3-(LVDd)3]}*0.8+0.6 (16), and the left ventricular mass index (LVMI) was obtained. LV end-diastolic volume (EDV), LV end-systolic volume (ESV), and LVEF were acquired using the modified biplane Simpson method. We obtained two-dimensional grayscale images from the apical four-chamber, two-chamber, and three-chamber views at a frame rate of 57–68 frames/s (average of 66.5±6 frames/s) to enable STE analysis of global longitudinal strain (GLS). The probe gain and image size were adjusted to display the endocardium clearly. All images were stored in Digital Imaging and Communication in Medicine (DICOM) format on a mobile hard disk for offline analysis.
All two-dimensional and Doppler recordings and measurements were performed according to the American Society of Echocardiography guidelines (15,17), and all parameters were averaged over three consecutive cardiac cycles.
Two-dimensional speckle-tracking echocardiography (STE)
Semi-automated function imaging from the specific vendor offline analysis software (EchoPAC version 203; GE Vingmed Ultrasound) was used by an observer to calculate the LV global and segmental longitudinal strain. The patients' data were imported into the EchoPAC workstation, and clear images were selected for analysis. After identifying the aortic valve closure from the apical long-axis view using the automated feature, the mitral annulus and LV apex of the end-systolic frame were defined in each of the three apical views. The automated algorithm traced the LV myocardium and adjusted it as needed. The software used a 17-segment model to calculate the GLS of each segment from the weighted average of the peak systolic longitudinal strain and provided the myocardial strain of the whole left ventricle. If one or more areas were not tracked adequately, the patient would be excluded.
Echocardiographic MW analysis
MW and related indices were calculated using a specific vendor module by combining the LV GLS and non-invasive estimation of LV pressure curves within the EchoPAC Version 203 software. Blood pressure was measured from the brachial cuff by sphygmomanometry after the patients had laid down at least 5–10 minutes before TTE, assuming that the peak systolic pressure was equal to the peak arterial pressure. The software then constructed a non-invasive LV pressure curve based on the duration of isovolumetric and ejection periods defined by valve timing events. Pressure-strain loop (PSL) was a curve of the change in LV pressure versus strain; a closed-loop formed along the counterclockwise direction from mitral valve closure to mitral valve opening. The region provided the index of MW within the PSL. The software calculated the following parameters:
- (GWI, mmHg%): The area from mitral valve closure to mitral valve opening within the LV PSL, which was the sum of MW.
- GCW (mmHg%): The sum of the work done by systolic cardiomyocyte shortening and isovolumic diastolic cardiomyocyte elongation, and GCW contributed to the heart.
- GWW (mmHg%): The negative work of the LV segments that do not contribute to LV ejection, consisting of systolic cardiomyocyte elongation and isovolumic diastolic cardiomyocyte shortening.
- GWE (%): GCW/(GCW + GWW), the percentage of GCW to the sum of GCW and GWW.
All MW data (mean values, curves) were exported in an Excel file.
The average time needed to analyze the myocardial work parameters per CHF patient was approximately 154±23 sec.
Statistical analysis
SPSS 18.0 (SPSS Inc., IBM, Chicago, USA) was adopted for statistical analysis, and the enumeration data were expressed as mean ± standard deviation. One-way analysis of variance (ANOVA) was used to compare the demographic data, conventional echocardiographic parameters, and MW parameters between the four groups (HC, HFpEF, HFmrEF, and HFrEF), followed by Fisher’s least significant difference (LSD)-t-test for pairwise comparison.
To further compare the MW parameters with conventional echocardiographic parameters in the multi-classification of the four groups, a random forest machine learning method was developed based on the above statistical analysis results, where the identified indicators with significant differences served as input predictors. The machine learning method ranked the input predictors according to their contributions or importance in the multi-classification task. The highly ranked MW parameters were then used to validate the application value in assessing the LV myocardial systolic function in patients with CHF, including early diagnosis over traditional LVEF and the complementarity with LVEF in improving the diagnostic accuracy in classifying multiple groups (HC, HFpEF, HFmrEF, and HFrEF).
A statistical repeatability test of the MW parameters was also performed by randomly choosing a defined number of studies and calculating the inter-and intra-class correlation coefficient (ICC). P<0.05 was considered statistically significant. The same physician repeatedly measured the MW parameters at 2-day intervals for the intra-class repeatability test. Two physicians repeatedly measured MW parameters for the inter-class repeatability test.
Results
Study participants
A total of 51 HC and 155 patients with CHF (including 55 patients with old myocardial infarction, 39 patients with dilated cardiomyopathy, 22 patients with ischemic cardiomyopathy, three patients with myocardial amyloidosis, and 36 patients with hypertension) were included in our study. The demographic and clinical laboratory data are summarized in Table 1. No significant differences in NT-proBNP, glomerular filtrate rate (GFR), and hemoglobin (HGB) were observed between the HC and HFpEF groups. Compared to the HC and HFpEF groups, the NT-proBNP was higher, while GFR and HGB were significantly decreased in the HFmrEF and HFrEF groups (P<0.05). Patients with HFmrEF and HFrEF were associated with diabetes, renal disease, and coronary artery disease (Table 1, Parameter group A). There were no significant differences between the groups in gender, age, height, weight, body surface area (BSA), systolic blood pressure (BP), diastolic BP, and heart rate (P>0.05).
Table 1
Variable | HC group (n=51) | HFpEF group (n=54) | HFmrEF group (n=50) | HFrEF group (n=51) |
---|---|---|---|---|
Parameter group A | ||||
Gender (M/F) | 26/25 | 27/27 | 28/22 | 27/24 |
Age (years) | 56.75±9.92 | 57.06±10.82 | 57.20±7.89 | 59.15±8.93 |
Height (cm) | 164.94±6.26 | 165.37±7.36 | 167.30±5.53 | 164.35±7.93 |
Weight (kg) | 68.32±8.63 | 66.70±9.54 | 68.86±13.99 | 67.74±11.75 |
BSA (m2) | 1.74±0.12 | 1.73±0.14 | 1.77±0.17 | 1.73±0.17 |
Systolic BP (mmHg) | 116.47±4.74 | 123.48±11.82 | 121.54±11.64 | 114.04±19.06 |
Diastolic BP (mmHg) | 70.73±6.19 | 76.93±11.42 | 71.73±8.84 | 71.78±18.84 |
Heart rate (beats/min) | 65.86±6.54 | 68.33±7.70 | 67.56±6.20 | 70.76±6.93 |
NT-proBNP (pg/mL) | 66.57±25.06 | 197.81±184.97 | 2935.71±5265.57ab | 5858.94±6803.62abc |
GFR (mL/min) | 104.02±10.10 | 102.82±8.69 | 67.33±14.46ab | 55.19±13.44abc |
HGB (g/L) | 140.29±6.54 | 137.63±8.87 | 125.06±15.75ab | 95.88±19.06abc |
Comorbidity | ||||
Hypertension | 0 | 10 | 4 | 6 |
Diabetes mellitus | 0 | 3 | 10 | 16 |
Renal disease | 0 | 0 | 30 | 35 |
Coronary artery disease | 0 | 15 | 19 | 28 |
Peripheral arterial disease | 0 | 2 | 4 | 6 |
Parameter group B | ||||
LAD (mm) | 33.51±3.83 | 37.13±4.77a | 39.04±4.52ab | 43.14±3.13abc |
LAVI (mL/m2) | 28.86±4.32 | 36.57±5.21a | 44.38±5.57ab | 52.14±4.97abc |
E/e’ (septal) | 6.74±1.32 | 13.15±2.29a | 14.90±1.99ab | 16.59±2.05abc |
TRVmax (m/s) | 2.44±0.25 | 2.47±0.31 | 2.46±0.29 | 2.50±0.32 |
LVDd (mm) | 45.86±2.98 | 48.13±3.72 | 53.50±4.65ab | 65.80±7.97abc |
EDV (mL) | 97.73±14.06 | 110.89±20.48 | 142.04±28.45ab | 224.27±70.31abc |
LVDs (mm) | 29.96±2.07 | 32.15±3.41 | 41.80±3.72ab | 55.49±7.07abc |
ESV (mL) | 35.35±5.47 | 43.57±11.01 | 79.00±17.03ab | 155.31±55.79abc |
LVEF (%) | 63.35±2.92 | 60.91±4.07 | 44.10±1.78ab | 32.67±5.42abc |
LVMI (g/m2) | 69.77±13.30 | 70.21±14.45 | 72.89±14.59 | 102.86±15.43abc |
Data are expressed as mean ± standard deviation (SD). Compared with control group, aP<0.05; Compared with the HFpEF group, bP<0.05; Compared with the HFmrEF group, cP<0.05. HC, Healthy control; HFpEF, heart failure with preserve ejection fraction; HFmrEF, heart failure with mid-range ejection fraction; HFrEF, heart failure with reduced ejection fraction; GFR, glomerular filtration rate; HGB, hemoglobin; BSA, body surface area; NT-proBNP, N-terminal-pro-brain natriuretic peptide; GFR, glomerular filtration rate; LAD, left atrial diameter; LAVI, left atrial volume index; TRVmax, maximum tricuspid regurgitation velocity; LVMI, left ventricular mass index; LVDd, left ventricular end-diastolic dimension; EDV, end-diastolic volume; LVDs, left ventricular end-systolic dimension; ESV, end-systolic volume; LVEF, left ventricular ejection fraction; BSA, body surface area; BP, blood pressure. 1 mmHg=0.133 kPa.
In implementing the machine learning method, 80% of the studies randomly selected from each group of HC, HFpEF, HFmrEF, and HFrEF were used as training data, while the remaining 20% of the studies were used as training used for testing data. The out-of-bag observations that occurred during the training process of random forests were used for validation data.
Statistical comparison of conventional echocardiographic parameters between the four groups
Compared to the HC group, no notable differences in LVDd, EDV, LVDs, ESV, LVEF, LVMI, and TRV (P>0.05) were observed, except for LAD, LAVI, and E/e' in the HFpEF group (P<0.05, Table 1, Parameter group B).
Also, compared to the HC and HFpEF groups, significantly increased LAD, LAVI, E/e', LVDd, LVDs, EDV, and ESV and markedly decreased LVEF were observed in the HFmrEF group (P<0.05, Table 1, Parameter group B).
Furthermore, compared to the HFmrEF group, significantly increased LAD, LAVI, E/e', LVDd, LVDs, EDV, ESV, and LVMI and notably decreased LVEF was observed in the HFrEF group (P<0.05, Table 1, Parameter group B).
Statistical comparison of myocardial work parameters between the four groups
As for the MW parameters, markedly decreased GWI, GCW, and GWE and significantly increased GWW were observed in the HFpEF, HFmrEF, and HFrEF groups, compared to the HC group (Table 2).
Table 2
Variable | HC group (n=51) | HFpEF group (n=54) | HFmrEF group (n=50) | HFrEF group (n=51) |
---|---|---|---|---|
GWI (mmHg%) | 1,992.86±176.50 | 1,506.91±148.97a | 1,267.04±150.66ab | 622.02±302.10abc |
GCW (mmHg%) | 2,227.55±206.68 | 1,758.96±202.53a | 1,532.38±230.11ab | 768.45±371.01abc |
GWW (mmHg%) | 58.37±45.97 | 107.78±73.55a | 150.60±96.23ab | 201.10±97.84abc |
GWE (%) | 96.41±2.75 | 90.89±6.26a | 85.24±7.82ab | 78.86±8.91abc |
Data are expressed as mean ± SD. Compared with control group, aP<0.05; Compared with HFpEF group, bP<0.05; Compared with HFmrEF group, cP<0.05. GWI, global myocardial work index; GCW, global constructive work; GWW, global wasted work; GWE, myocardial work efficiency; HC, healthy control; HFpEF, heart failure with preserve ejection fraction; HFmrEF, heart failure with mid-range ejection fraction; HFrEF, heart failure with reduced ejection fraction.
There were also statistically significant differences between the HFpEF, HFmrEF, and HFrEF groups in all MW parameters (GWI, GCW, GWE, and GWW) (Figure 2 and Table 2).
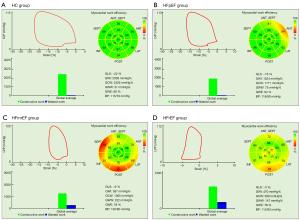
Machine learning-based integration of myocardial work and conventional echocardiographic parameters for multi-group diagnosis
Based on the above statistical analysis, the conventional echocardiographic parameters, LAD, LVDd, LVDs, EDV, ESV, and LVEF, as well as the MW parameters, GWI, GCW, GWE, and GWW, were used for multi-group diagnosis of the HC, HFpEF, HFmrEF, and HFrEF groups by establishing a multi-classification machine learning model. The histogram of each parameter was a plot in Figure 3, with the different colors corresponding to each of the above groups.
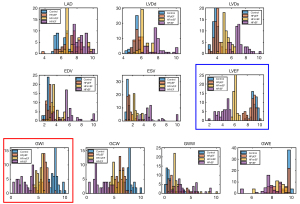
The accuracy of multi-classification of the four groups was as high as 98% (Figure 4A), with LVEF and GWI being more important than the other predictors (Figure 4B). Three additional machine learning experiments were performed for multi-classification of the four groups, with the inputs being LVEF, GWI, and a combination of both, respectively. Multi-classification accuracies of 0.82, 0.88, and 0.98 were obtained, with the combination of LVEF and GWI exhibiting the highest accuracy.

GWI was positively correlated with LVEF, with a correlation coefficient of 0.86 (Figure 5). The receiver operating characteristic (ROC) curves of the multi-classification machine learning models for each group (Figure 6) were also plotted and revealed the complementarity of LVEF and GWI in improving the accuracy of multi-classification. Specifically, for the HC and HFpEF groups, the area under the ROC curve (AUC) using GWI was significantly greater than that by LVEF, while for the HFmrEF and HFrEF groups, the AUC using GWI was smaller than that by LVEF. The combination of LVEF and GWI obtained the highest AUC of all four groups.
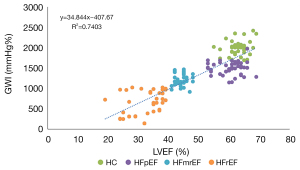
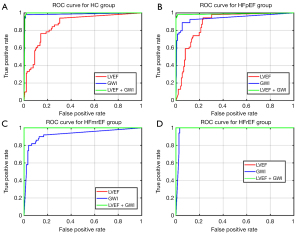
Additionally, the histograms of LVEF (Figure 3, blue bounding box) and GWI (Figure 3, red bounding box) further demonstrated their complementarity in improving the multi-classification accuracy compared to each individually. The overlap in LVEF between the HC and HFpEF groups was greater than that of the counterpart in GWI, while LVEF demonstrated a better separation between the HFpEF, HFmrEF and HFrEF groups.
Repeatability test
Regarding the MW parameters, a repeatability test was performed on 20 studies, which showed statistically significant results and indicated good repeatability (P<0.05, Table 3).
Table 3
Variable | Intra-group | Inter-group | |||
---|---|---|---|---|---|
r | 95% CI | r | 95% CI | ||
GWI (mmHg%) | 0.912 | 0.836–0.963 | 0.911 | 0.857–0.979 | |
GCW (mmHg%) | 0.911 | 0.822–0.976 | 0.925 | 0.855–0.979 | |
GWW (mmHg%) | 0.918 | 0.846–0.992 | 0.921 | 0.836–0.979 | |
GWE (%) | 0.921 | 0.859–0.987 | 0.922 | 0.845–0.978 |
GWI, global myocardial work index; GCW, global constructive work; GWW, global wasted work; GWE, myocardial work efficiency.
Discussion
CHF is a clinical syndrome caused by abnormal structure and/or function (systolic or diastolic dysfunction), resulting in increased intracardiac pressure and/or reduced cardiac output at rest or during stress, with a high mortality rate (18,19). Myocardial systolic function assessments followed by relevant treatments important for CHF patients to prevent and delay myocardial remodeling, improve symptoms, reduce mortality, and improve quality of life (20-22). The present study investigated the value of the echocardiographic MW technique in the application of assessing LV systolic function in patients with CHF. Specifically, the MW parameters were significantly different between all four groups (HC, HFpEF, HFmrEF, and HFrEF), while the traditional and most commonly used LVEF was insufficient in distinguishing the HC and HFpEF groups. Also, the combination of GWI and LVEF could complementarily improve the diagnostic accuracy of classifying multiple groups (HC, HFpEF, HFmrEF, and HFrEF), with an accuracy of as high as 0.98, compared to 0.82 and 0.88 when using each individually.
The clinical diagnosis of HFpEF, which was previously known as diastolic heart failure with normal LVEF, is more challenging than HFrEF (23). Statistically significance in the MW parameters indicated some degree of impaired myocardial systolic function in HFpEF patients. Considering that patients with HFpEF generally have an increased left atrial size and additional "evidence" of impaired LV filling or suction capacity, myocardial cell elongation was affected during isovolumic relaxation, resulting in significantly reduced GCW and GWE. The reduction of GWI indicated an overall decreased MW in HFpEF patients. Therefore, despite the normal LVEF in HFpEF patients, the MW parameters could be more sensitive measures of intrinsic myocardial systolic function, particularly in distinguishing HFpEF from HC patients (24).
Early population-level studies have shown that patients with HFmrEF have similar clinical characteristics and prognoses to those with HFpEF (25-27). The results of the present study appeared to paint a different picture. In terms of MW parameters, the HFmrEF group was found to be intermediate to HFpEF and HFrEF. Also, GWI, GCW, and GWE progressively decreased, and GWW gradually increased from HFpEF through HFmrEF to HFrEF. The MW parameters were statistically different between the groups (P<0.05), and all three groups demonstrated evidence of LV systolic dysfunction. Considering the lack of formal studies assessing LV systolic function in patients with HFmrEF (28), the management of HFmrEF patients is challenging. Despite significant progress (29), our study suggests that the MW parameters could be used to better elucidate the HFmrEF group and therapeutic assessment.
In clinical practice, a non-negligible number of CHF patients experience LVEF recovery or exacerbation due to advances in drug therapy, devices, coronary revascularization, or valvular repair (30). MW is expected to be a new and more sensitive method with potential application value in assessing LV myocardial systolic function after clinical treatment (31,32). Decreased GWI demonstrated significantly impaired contractile performance of the cardiomyocytes. The reason may be attributed to severe ischemia, degeneration, necrosis, disordered arrangement of myocardial fibers, myocardial interstitial fibrosis, and other pathological changes in myocardial cells (33). The LVEF and GWI, which were highly ranked regarding importance in the multi-classification model, were complementary in improving the multi-classification of HC, HFpEF, HFmrEF, and HFrEF groups to the machine learning model. In the present study, the complementarity of both was further analyzed and confirmed by the histograms and ROC curves. Therefore, GWI has significant clinical application value in promoting the traditional LVEF.
However, this study had some limitations that should be noted. Firstly, this study only evaluated the global work of the LV myocardium in CHF patients and did not distinguish the work of each segment among 17 segments. Secondly, brachial artery blood pressure was used to replace LV pressure. Thirdly, CHF patients may have received medication before making a definite diagnosis. Fourthly, follow-up of the study population lacked to evaluate further MW, which will be encompassed in the next phase of the study. Lastly, we did not compare the MW index with LVEF determined by cardiac magnetic resonance, with higher reproducibility, and this was only a single center study.
In summary, this study demonstrated that GWI could play a complementary role to LVEF in the early diagnosis of HFpEF patients from the HC group, as well as improving the accuracy of clinical evaluation of CHF patients, highlighting the value of echocardiographic MW in the assessment of LV myocardial systolic function in patients with CHF. Echocardiographic MW is a promising tool and should be utilized along with conventional LVEF to evaluate CHF in clinical practice.
Acknowledgments
We would like to thank all study participants for their interest in our study.
Funding: This work was supported by National Natural Science Foundation of China (82071950, 61802330), National Natural Science Foundation of Henan for Excellet Young Scientists (202300410364) and Medical Science and Technology Project of Henan Province (SB201901099, 2018020452, LHGJ20190805).
Footnote
Conflicts of Interest: All authors have completed the ICMJE uniform disclosure form (available at https://dx.doi.org/10.21037/qims-20-1038). The authors have no conflicts of interest to declare.
Ethical Statement: The authors are accountable for all aspects of the work in ensuring that questions related to the accuracy or integrity of any part of the work are appropriately investigated and resolved. The study was conducted in accordance with the Declaration of Helsinki (as revised in 2013), and it was approved by the Ethics Committee of Henan Provincial People’s Hospital. Individual consent for this retrospective analysis was waived.
Open Access Statement: This is an Open Access article distributed in accordance with the Creative Commons Attribution-NonCommercial-NoDerivs 4.0 International License (CC BY-NC-ND 4.0), which permits the non-commercial replication and distribution of the article with the strict proviso that no changes or edits are made and the original work is properly cited (including links to both the formal publication through the relevant DOI and the license). See: https://creativecommons.org/licenses/by-nc-nd/4.0/.
References
- Lüscher TF. Heart failure: the cardiovascular epidemic of the 21st century. Eur Heart J 2015;36:395-7. [Crossref] [PubMed]
- Mosterd A, Hoes AW. Clinical epidemiology of heart failure. Heart 2007;93:1137-46. [Crossref] [PubMed]
- Hopper I, Easton K. Chronic heart failure. Aust Prescr 2017;40:128-36. [Crossref] [PubMed]
- Kinno M, Nagpal P, Horgan S, Waller AH. Comparison of Echocardiography, Cardiac Magnetic Resonance, and Computed Tomographic Imaging for the Evaluation of Left Ventricular Myocardial Function: Part 1 (Global Assessment). Curr Cardiol Rep 2017;19:9. [Crossref] [PubMed]
- Chan J, Edwards NFA, Khandheria BK, Shiino K, Sabapathy S, Anderson B, Chamberlain R, Scalia GM. A new approach to assess myocardial work by non-invasive left ventricular pressure-strain relations in hypertension and dilated cardiomyopathy. Eur Heart J Cardiovasc Imaging 2019;20:31-9. [Crossref] [PubMed]
- Yingchoncharoen T, Agarwal S, Popović ZB, Marwick TH. Normal ranges of left ventricular strain: a meta-analysis. J Am Soc Echocardiogr 2013;26:185-91. [Crossref] [PubMed]
- Mor-Avi V, Patel MB, Maffessanti F, Singh A, Medvedofsky D, Zaidi SJ, Mediratta A, Narang A, Nazir N, Kachenoura N, Lang RM, Patel AR. Fusion of Three-Dimensional Echocardiographic Regional Myocardial Strain with Cardiac Computed Tomography for Noninvasive Evaluation of the Hemodynamic Impact of Coronary Stenosis in Patients with Chest Pain. J Am Soc Echocardiogr 2018;31:664-73. [Crossref] [PubMed]
- Tulner SA, Klautz RJ, van Rijk-Zwikker GL, Engbers FH, Bax JJ, Baan J, van der Wall EE, Dion RA, Steendijk P. Perioperative assessment of left ventricular function by pressure-volume loops using the conductance catheter method. Anesth Analg 2003;97:950-7. table of contents. [Crossref] [PubMed]
- Oberhoffer FS, Abdul-Khaliq H, Jung AM, Zemlin M, Rohrer TR, Abd El Rahman M. Assessment of left ventricular myocardial work in Turner syndrome patients: insights from the novel non-invasive pressure-strain loop analysis method. Quant Imaging Med Surg 2020;10:15-25. [Crossref] [PubMed]
- Edwards NFA, Scalia GM, Shiino K, Sabapathy S, Anderson B, Chamberlain R, Khandheria BK, Chan J. Global Myocardial Work Is Superior to Global Longitudinal Strain to Predict Significant Coronary Artery Disease in Patients With Normal Left Ventricular Function and Wall Motion. J Am Soc Echocardiogr 2019;32:947-57. [Crossref] [PubMed]
- Zhu M, Wang Y, Cheng Y, Su Y, Chen H, Shu X. The value of non-invasive myocardial work indices derived from left ventricular pressure-strain loops in predicting the response to cardiac resynchronization therapy. Quant Imaging Med Surg 2021;11:1406-20. [Crossref] [PubMed]
- Hubert A, Le Rolle V, Leclercq C, Galli E, Samset E, Casset C, Mabo P, Hernandez A, Donal E. Estimation of myocardial work from pressure-strain loops analysis: an experimental evaluation. Eur Heart J Cardiovasc Imaging 2018;19:1372-9. [Crossref] [PubMed]
- Yancy CW, Jessup M, Bozkurt B, Butler J, Casey DE, Colvin MM, Drazner MH, Filippatos GS, Fonarow GC, Givertz MM, Hollenberg SM, Lindenfeld JA, Masoudi FA, McBride PE, Peterson PN, Stevenson LW, Westlake C. 2017 ACC/AHA/HFSA Focused Update of the 2013 ACCF/AHA Guideline for the Management of Heart Failure: A Report of the American College of Cardiology/American Heart Association Task Force on Clinical Practice Guidelines and the Heart Failure Society of America. Circulation 2017;136:e137-61. [PubMed]
- Ponikowski P, Voors AA, Anker SD, Bueno H, Cleland JGF, Coats AJS, et al. 2016 ESC Guidelines for the diagnosis and treatment of acute and chronic heart failure: The Task Force for the diagnosis and treatment of acute and chronic heart failure of the European Society of Cardiology (ESC)Developed with the special contribution of the Heart Failure Association (HFA) of the ESC. Eur Heart J 2016;37:2129-200. [Crossref] [PubMed]
- Lang RM, Badano LP, Mor-Avi V, Afilalo J, Armstrong A, Ernande L, Flachskampf FA, Foster E, Goldstein SA, Kuznetsova T, Lancellotti P, Muraru D, Picard MH, Rietzschel ER, Rudski L, Spencer KT, Tsang W, Voigt JU. Recommendations for cardiac chamber quantification by echocardiography in adults: an update from the American Society of Echocardiography and the European Association of Cardiovascular Imaging. J Am Soc Echocardiogr 2015;28:1-39.e14. [Crossref] [PubMed]
- Milan A, Puglisi E, Magnino C, Naso D, Abram S, Avenatti E, Rabbia F, Mulatero P, Veglio F. Left atrial enlargement in essential hypertension: role in the assessment of subclinical hypertensive heart disease. Blood Press 2012;21:88-96. [Crossref] [PubMed]
- Nagueh SF, Smiseth OA, Appleton CP, Byrd BF 3rd, Dokainish H, Edvardsen T, Flachskampf FA, Gillebert TC, Klein AL, Lancellotti P, Marino P, Oh JK, Popescu BA, Waggoner AD. Recommendations for the Evaluation of Left Ventricular Diastolic Function by Echocardiography: An Update from the American Society of Echocardiography and the European Association of Cardiovascular Imaging. J Am Soc Echocardiogr 2016;29:277-314. [Crossref] [PubMed]
- Nieminen MS, Dickstein K, Fonseca C, Serrano JM, Parissis J, Fedele F, et al. The patient perspective: Quality of life in advanced heart failure with frequent hospitalisations. Int J Cardiol 2015;191:256-64. [Crossref] [PubMed]
- Gajarsa JJ, Kloner RA. Left ventricular remodeling in the post-infarction heart: a review of cellular, molecular mechanisms, and therapeutic modalities. Heart Fail Rev 2011;16:13-21. [Crossref] [PubMed]
- Distefano G, Sciacca P. Molecular pathogenesis of myocardial remodeling and new potential therapeutic targets in chronic heart failure. Ital J Pediatr 2012;38:41. [Crossref] [PubMed]
- Cameli M, Mondillo S, Solari M, Righini FM, Andrei V, Contaldi C, De Marco E, Di Mauro M, Esposito R, Gallina S, Montisci R, Rossi A, Galderisi M, Nistri S, Agricola E, Mele D. Echocardiographic assessment of left ventricular systolic function: from ejection fraction to torsion. Heart Fail Rev 2016;21:77-94. [Crossref] [PubMed]
- Konstam MA, Abboud FM. Ejection Fraction: Misunderstood and Overrated (Changing the Paradigm in Categorizing Heart Failure). Circulation 2017;135:717-9. [Crossref] [PubMed]
- Tromp J, MacDonald MR, Tay WT, Teng TK, Hung CL, Narasimhan C, Shimizu W, Ling LH, Ng TP, Yap J, McMurray JJV, Zile MR, Richards AM, Anand IS, Lam CSP. Heart Failure With Preserved Ejection Fraction in the Young. Circulation 2018;138:2763-73. [Crossref] [PubMed]
- Lam CSP, Voors AA, de Boer RA, Solomon SD, van Veldhuisen DJ. Heart failure with preserved ejection fraction: from mechanisms to therapies. Eur Heart J 2018;39:2780-92. [Crossref] [PubMed]
- Shah KS, Xu H, Matsouaka RA, Bhatt DL, Heidenreich PA, Hernandez AF, Devore AD, Yancy CW, Fonarow GC. Heart Failure With Preserved, Borderline, and Reduced Ejection Fraction: 5-Year Outcomes. J Am Coll Cardiol 2017;70:2476-86. [Crossref] [PubMed]
- Fonarow GC, Stough WG, Abraham WT, Albert NM, Gheorghiade M, Greenberg BH, O'Connor CM, Sun JL, Yancy CW, Young JBOPTIMIZE-HF Investigators and Hospitals. Characteristics, treatments, and outcomes of patients with preserved systolic function hospitalized for heart failure: a report from the OPTIMIZE-HF Registry. J Am Coll Cardiol 2007;50:768-77. [Crossref] [PubMed]
- Sweitzer NK, Lopatin M, Yancy CW, Mills RM, Stevenson LW. Comparison of clinical features and outcomes of patients hospitalized with heart failure and normal ejection fraction (> or =55%) versus those with mildly reduced (40% to 55%) and moderately to severely reduced (<40%) fractions. Am J Cardiol 2008;101:1151-6. [Crossref] [PubMed]
- Yancy CW, Jessup M, Bozkurt B, Butler J, Casey DE Jr, Drazner MH, et al. 2013 ACCF/AHA guideline for the management of heart failure: executive summary: a report of the American College of Cardiology Foundation/American Heart Association Task Force on practice guidelines. Circulation 2013;128:1810-52. [Crossref] [PubMed]
- Srivastava PK, Hsu JJ, Ziaeian B, Fonarow GC. Heart Failure With Mid-range Ejection Fraction. Curr Heart Fail Rep 2020;17:1-8. [Crossref] [PubMed]
- Hellawell JL, Margulies KB. Myocardial reverse remodeling. Cardiovasc Ther 2012;30:172-81. [Crossref] [PubMed]
- Smiseth OA, Donal E, Penicka M, Sletten OJ. How to measure left ventricular myocardial work by pressure-strain loops. Eur Heart J Cardiovasc Imaging 2021;22:259-61. [Crossref] [PubMed]
- Wang RR, Tian T, Li SQ, Leng XP, Tian JW. Assessment of Left Ventricular Global Myocardial Work in Patients With Different Degrees of Coronary Artery Stenosis by Pressure-Strain Loops Analysis. Ultrasound Med Biol 2021;47:33-42. [Crossref] [PubMed]
- De Jong KA, Lopaschuk GD. Complex Energy Metabolic Changes in Heart Failure With Preserved Ejection Fraction and Heart Failure With Reduced Ejection Fraction. Can J Cardiol 2017;33:860-71. [Crossref] [PubMed]