Effect of a new deep learning image reconstruction algorithm for abdominal computed tomography imaging on image quality and dose reduction compared with two iterative reconstruction algorithms: a phantom study
Introduction
During the last decade, the reconstruction algorithms used to reconstruct computed tomography (CT) images have evolved considerably (1). For a long time, filtered back projection (FBP) was used to reconstruct images from raw data and presented the advantages of linear properties where spatial resolution was not dependent of the dose and contrast conditions (1,2). However, the images generated with FBP were very noisy, limiting the dose optimization, essential for CT examination. To compensate for this problem, iterative reconstruction (IR) algorithms have been developed by the main CT manufacturers (1).
Two main types of IR algorithms have been developed: IR hybrid/statistical (HIR) algorithms and model-based iterative reconstruction (MBIR) algorithms (1,3). HIR algorithms combine FBP and IR in different proportions to reconstruct CT images. MBIR is based on probabilistic method to decrease noise and artefacts, deriving a statistical cost function that comprises X-ray physics and CT optics modeling (4,5). Using full and advanced MBIR algorithms, the reconstruction time is considerable, limiting its routine use in clinical practice. Differences in terms of image noise, image texture and spatial resolution between the HIR and MBIR algorithms depend on the algorithms studied (3).
Several studies on phantoms and on patients showed noise reduction at a given dose level with these IR algorithms, thus allowing to reduce the dose while maintaining a similar image noise (6-16). However, using IR algorithms and especially the highest IR level, changes the noise texture by smoothing the image, which can impede the radiologist’s interpretation (17). Moreover, IR algorithms have non-linear properties, rendering the spatial resolution dependent on contrast and dose (7,18). As defined by Franck et al., these non-linear properties generate clinical images with locally flat regions in homogeneous tissues and residual noise spikes around sharp edges (bone and vessel boundaries), especially for low-dose CT acquisitions (19).
Recently, new reconstruction algorithms based on deep learning have been developed (20,21). The deep learning image reconstruction (DLR) algorithms developed by GE Healthcare (TrueFidelity™) and Canon Medical Systems [Advanced intelligent Clear-IQ Engine (AiCE)] feature a deep neural network (DNN), trained respectively with high quality FBP (20) and MBIR algorithm [Forward projected model-based Iterative Reconstruction SoluTion (FIRST)] (21) datasets to learn how to differentiate noise from signal. In addition, the TrueFidelity DNN was trained with both patient and phantom data whereas it was trained only with patient data for AiCE. CT images obtained with these algorithms using denoising techniques showed suppressed noise with no change of noise texture or distortion of anatomical and pathological structures (19,22-30).
Recently, three studies have compared the impact of TrueFidelity algorithm on dose reduction and image quality with HIR algorithm (Asir-V, GE Healthcare) using a task-based image quality assessment (31-33). To our knowledge, only one study has compared the impact of the AiCE algorithm on dose reduction and image quality with HIR and/or MBIR algorithms (28). However, this study was performed on a standard phantom with a 20-cm diameter not adapted to the patient’s abdominal morphology. In addition, in that same study, only the iterative level “Standard” was assessed and not the three levels available for each IR algorithms (Mild, Standard and Strong). On another note, many clinical studies have shown the impact of AiCE on dose reduction and image quality for different anatomical locations in both adult and pediatric patients (22,25-27,29,34,35).
The aim of our study was to assess the impact on task-based image quality and the dose reduction potential of the DLR algorithm (AiCE™) compared to a HIR algorithm [Adaptive Iterative Dose Reduction 3D (AIDR 3D) (AIDR 3D)] and an advanced MBIR algorithm (FIRST) on a Canon Medical CT system. To achieve this goal, a task-based IQ assessment was conducted based on the methodology previously used in our institution for the assessment of TrueFidelity™ algorithm (23). This comparison was performed using an image quality phantom placed inside its body insert to most closely simulate the morphology of patients undergoing an abdomen-pelvic CT examination and the three iterative levels available for each algorithm assessed.
Methods
Image reconstruction algorithms used in the CT system
Acquisitions were performed on an Aquilion ONE GENESIS (Canon Medical Systems) CT scan equipped with the HIR, MBIR and DLR algorithms. The HIR algorithm available was the AIDR 3D algorithm, the advanced MBIR was the FIRST algorithm and the DLR algorithm was AiCE. All algorithms had three IR/DLR levels: Mild, Standard and Strong. AIDR 3D had a fourth IR level (enhanced), not studied in the present study. For the IR/DLR algorithms, the Mild level gave slightly noisier images than the Strong level although it gave less noisy images. The current version of AiCE (AiCE 1st version) required 0.5-mm-thick native slices and only the reconstruction kernel “Body” was available for abdominal images. “Lung” and “eCardiac” reconstruction kernels were available for chest/cardiac images.
Data acquisition
A 20-cm-diameter CT ACR 464 phantom (Gammex) placed inside a body ring (diameter of 33 cm and length of 24 cm) was scanned to measure physical metrics (Figure 1A). All acquisitions were performed with a 120-kVp tube voltage. Tube currents (mA) were defined to obtain six volume CT dose indexes (CTDIvol: 15, 10, 7.5, 5, 2.5, and 1 mGy). The pitch factor was of 0.813, the beam collimation of 80 mm × 0.5 mm, the acquisition field-of-view of 500 mm and a rotation time of 0.50 s. All these parameters were used for the abdomen-pelvis CT examinations on this CT system in our Institution. Five acquisitions were performed for each dose level.
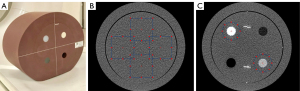
For each acquisition, raw data were reconstructed with each of the three IR or DLR levels (Mild, Standard and Strong) of AIDR 3D, FIRST and AiCE. To take into account AiCE limits on reconstruction parameters, images were reconstructed using a soft tissue reconstruction kernel and a slice thickness of 0.5 mm (with a 0.5 mm increment) for all algorithms. The reconstruction kernel “FC08” was used for AIDR 3D and the reconstruction kernel “Body” for FIRST and AiCE algorithms. A field-of-view of 250 mm was used for all images. The reconstruction time for each set of 271 images was between 10 and 30 s for AIDR 3D and between 30 and 60 s AiCE and from 5 to 10 min for FIRST.
Task-based image quality assessment
Image quality assessment was carried out using imQuest software (Duke) (36,37). Noise power spectrum (NPS) was used to assess the noise texture and the noise magnitude. With the non-linear and non-stationary properties of IR algorithms, the task-based transfer function (TTF) was used to assess the spatial resolution as function of dose level and contrast (2). The detectability index (d') was used to estimate the ability of the radiologist to perform a clinical task such as the detection of simulated lesions.
Noise power spectrum
NPS was computed by placing four square regions of interest (ROIs) in the uniform section (module 3) of the ACR phantom (Figure 1B). The ROIs were not placed in the center of the phantom so that the NPS was not affected by the two metallic rods placed inside this phantom section (38). The NPS was computed, as follows:
where Δx and Δy are the pixel size in the x- and y-directions, respectively, Lx and Ly are the ROI lengths in the x- and y-directions, respectively, NROI is the number of ROIs, FT is the Fourier transform and ROIi(x,y) is the mean pixel value measured of a ROI at the position (x,y) and FITi(x,y) is a 2nd order polynomial fit of ROIi(x,y) (39). The combined NPS was computed on 160 ROIs (NROI) of 128×128 pixels (Lx and Ly) each, within 40 consecutive axial slices.
To quantify the changes of magnitude and texture of noise, the NPS peak and average spatial frequency (fav) of the NPS curve were measured respectively. fav values describe the overall frequency content of the NPS and were obtained as follows:
where f is the radial spatial frequency and NPS(f) is the radially re-binned/average 1D NPS (36).
Task-based transfer function
The TTF was assessed using two cylindrical inserts (acrylic and bone, with a diameter of 25 mm and a depth of 4 cm) available in module 1 of the ACR phantom (Figure 1C) from 20 consecutive axial slices according to the methodology previously reported (40). Both inserts were used to assess the spatial resolution in low and high contrast conditions. A circular ROI was placed around the insert, and a circular-edge technique was employed to measure the edge spread function (ESF) by plotting the HU value of each pixel as a function of the distance to the center of the insert. The line spread function (LSF) was then obtained by derivation of the ESF. The TTF was computed from the normalized Fourier transformation of the LSF.
Detectability index
A non-prewhitening observer model with an eye filter (NPWE) was used to calculate the detectability index (d'):
where u and v are the spatial frequencies in the x- and y-directions, respectively, E is the eye filter that models the human visual system’s sensitivity to different spatial frequencies (41-43) and W(u,v) is the task function defined as:
where h1(x,y) and h2(x,y) correspond to the object present and the object absent hypotheses, respectively. The eye filter (E) was modeled according to the visual response function (41).
where is the radial spatial frequency in cycles per degree. C =0.98, γ =0.68 and ρ =1.5.
Two task functions were defined to represent small and large features according to the task defined by Samei et al. (37): a circular signal with a pre-imaged contrast of 500 HU and a diameter of 1.5 mm for the former, and a contrast of 120 HU and a diameter of 25 mm for the latter.
TTF outcomes for the bone insert (995 HU) were used for the small feature while the results from for the acrylic insert (120 HU) were used for the large feature. The small feature was defined to model either the detection of calcifications or the identification of high-contrast tissue boundaries and the large feature was intended to model the detection task of a large mass in the liver (37). As defined by Samei et al, the characteristics (size and contrast) of the small feature were defined to “preferentially weighing low- and mid-spatial frequencies representing more subtle features associated with fine structures in CT images” and those of the large feature to “predominantly weighing low-spatial frequencies, modeling the detection task of larger lesions” (37). “Both features were defined based on the direction from (their) radiologist colleagues to model most relevant tasks” (37).
d' was obtained using a zoom factor of 1.5, a viewing distance of 500 mm, and a field of view of 500 mm.
The combination of the TTF, NPS and task function allowed estimation of d' as function of the CTDIvol, for all detection tasks and reconstruction types. Higher d' values were correlated with a better detection of simulated lesions by the radiologists.
To estimate the increase of d' and the potential dose variation, d' values obtained at 10 mGy using AIDR 3D Standard were used. This dose level corresponds to the classical dose level usually used in our Institution for abdomen-pelvic CT examinations. d' values were compared with those obtained with AIDR 3D Standard at 10 mGy to assess d' potential increase. The CTDIvol obtained with AiCE and FIRST for the same d' value than with AIDR 3D Standard at 10 mGy were compared to assess the potential dose variation.
Results
Noise power spectrum
Table 1 and Figure 2 report the noise magnitude and average NPS spatial frequency (fav) values for all algorithms and dose levels. The NPS peak decreased as the dose level increased and the level of IR/DLR increased (Figure 2A). From the level Mild to Standard, NPS peaks decreased on average by −15%±1% for AIDR 3D, −33%±5% for FIRST and −22%±6% for AiCE and −19%±4%, −38%±8% and −17%±5% from Standard to Strong, respectively. NPS peaks were lower with AiCE than with all AIDR 3D levels (−41%±6%). Compared to FIRST, NPS peaks were lower with AiCE for all IR/DLR levels but the differences were less marked for Strong level (−15%±6% for Strong level and −41%±11% for both other levels). NPS peaks were lower with FIRST than with AIDR 3D for all IR levels, except for Mild level from 1 to 10 mGy and for Standard level at 1 mGy.
Table 1
NPS outcomes | Algorithms | Levels | CTDIvol (mGy) | |||||
---|---|---|---|---|---|---|---|---|
1.0 | 2.5 | 5.0 | 7.5 | 10.0 | 15.0 | |||
NPS peak (HU2·mm2) | AIDR 3D | Mild | 4,652±85 | 3,106±35 | 1,972±14 | 1,541±25 | 1,261±10 | 1,030±5 |
Standard | 3,914±80 | 2,617±25 | 1,665±13 | 1,335±23 | 1,086±11 | 854±4 | ||
Strong | 2,965±76 | 2,044±17 | 1,362±13 | 1,082±18 | 917±10 | 725±4 | ||
FIRST | Mild | 7,938±749 | 3,757±302 | 2,289±101 | 1,703±108 | 1,293±74 | 843±51 | |
Standard | 4,643±758 | 2,384±196 | 1,568±122 | 1,181±53 | 923±79 | 607±30 | ||
Strong | 2,454±424 | 1,420±353 | 931±127 | 714±78 | 590±81 | 468±18 | ||
AiCE | Mild | 3,128±83 | 1,921±15 | 1,179±11 | 944±15 | 778±10 | 602±4 | |
Standard | 2,644±81 | 1,433±13 | 841±7 | 696±7 | 614±9 | 508±4 | ||
Strong | 2,128±87 | 1,245±14 | 705±5 | 596±4 | 545±9 | 382±4 | ||
Average NPS spatial frequency (mm−1) | AIDR 3D | Mild | 0.18±0.00 | 0.22±0.00 | 0.24±0.00 | 0.25±0.00 | 0.26±0.00 | 0.27±0.00 |
Standard | 0.17±0.00 | 0.21±0.00 | 0.23±0.00 | 0.24±0.00 | 0.25±0.00 | 0.25±0.00 | ||
Strong | 0.16±0.00 | 0.19±0.00 | 0.22±0.00 | 0.23±0.00 | 0.23±0.00 | 0.24±0.00 | ||
FIRST | Mild | 0.18±0.00 | 0.21±0.01 | 0.24±0.00 | 0.25±0.00 | 0.25±0.00 | 0.25±0.01 | |
Standard | 0.15±0.01 | 0.18±0.00 | 0.21±0.00 | 0.22±0.00 | 0.22±0.00 | 0.22±0.00 | ||
Strong | 0.12±0.01 | 0.15±0.01 | 0.17±0.01 | 0.18±0.00 | 0.19±0.00 | 0.18±0.00 | ||
AiCE | Mild | 0.16±0.00 | 0.20±0.00 | 0.23±0.00 | 0.24±0.00 | 0.24±0.00 | 0.24±0.00 | |
Standard | 0.15±0.00 | 0.19±0.00 | 0.22±0.00 | 0.23±0.00 | 0.23±0.00 | 0.24±0.00 | ||
Strong | 0.15±0.00 | 0.18±0.00 | 0.21±0.00 | 0.22±0.00 | 0.23±0.00 | 0.24±0.00 |
Values are expressed as mean ± standard deviation. AIDR 3D, Adaptive Iterative Dose Reduction 3D; AiCE, Advanced intelligent Clear-IQ Engine; CTDIvol, volume computed tomography dose index; FIRST, Forward projected model-based Iterative Reconstruction SoluTion; NPS, noise power spectrum.
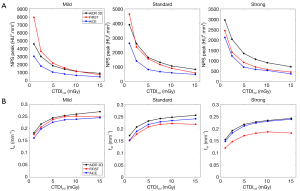
The NPS average spatial frequency shifted towards higher frequencies as the dose increased and the level of IR/DLR decreased (Figure 2B). The highest fav values were found with AIDR 3D and the lowest for FIRST, except for Mild level. Differences of fav values between AiCE and AIDR 3D decreased as the IR/DLR level increased (−9%±2% using Mild and −3%±2% using Strong). The opposite pattern was found between FIRST and AIDR 3D (−4%±2% using Mild and −22%±2% using Strong). For Mild level, fav values of AiCE were on average −5%±2% lower than FIRST but were higher by an average of 6%±3% and 25%±3% for Standard and Strong levels, respectively.
Task-based transfer function
Table 2 and Figure 3 report the TTF50% values for both inserts, all algorithms and dose levels. The values of TTF50% decreased as the dose level decreased. The values of TTF50% shifted to lower frequencies as the AIDR 3D and FIRST level increased. The opposite pattern was found for AiCE.
Table 2
TTF outcomes | Algorithms | Levels | CTDIvol (mGy) | |||||
---|---|---|---|---|---|---|---|---|
1.0 | 2.5 | 5.0 | 7.5 | 10.0 | 15.0 | |||
TTF50% (mm−1), acrylic insert | AIDR 3D | Mild | 0.24±0.01 | 0.28±0.01 | 0.33±0.03 | 0.34±0.02 | 0.34±0.01 | 0.36±0.02 |
Standard | 0.23±0.01 | 0.26±0.01 | 0.32±0.03 | 0.33±0.02 | 0.33±0.01 | 0.35±0.02 | ||
Strong | 0.21±0.01 | 0.24±0.01 | 0.31±0.03 | 0.31±0.02 | 0.31±0.01 | 0.33±0.03 | ||
FIRST | Mild | 0.26±0.01 | 0.29±0.01 | 0.35±0.03 | 0.38±0.05 | 0.38±0.02 | 0.40±0.02 | |
Standard | 0.21±0.01 | 0.25±0.01 | 0.34±0.03 | 0.34±0.04 | 0.34±0.02 | 0.37±0.03 | ||
Strong | 0.16±0.00 | 0.20±0.02 | 0.28±0.03 | 0.29±0.03 | 0.29±0.00 | 0.31±0.01 | ||
AiCE | Mild | 0.22±0.01 | 0.26±0.01 | 0.28±0.03 | 0.33±0.02 | 0.33±0.01 | 0.36±0.05 | |
Standard | 0.22±0.01 | 0.26±0.01 | 0.33±0.03 | 0.34±0.02 | 0.34±0.01 | 0.37±0.03 | ||
Strong | 0.23±0.01 | 0.26±0.01 | 0.34±0.03 | 0.34±0.01 | 0.35±0.01 | 0.37±0.03 | ||
TTF50% (mm−1), bone insert | AIDR 3D | Mild | 0.24±0.01 | 0.30±0.00 | 0.33±0.01 | 0.34±0.00 | 0.35±0.00 | 0.36±0.00 |
Standard | 0.23±0.00 | 0.29±0.01 | 0.32±0.01 | 0.34±0.00 | 0.34±0.00 | 0.35±0.00 | ||
Strong | 0.21±0.00 | 0.27±0.00 | 0.31±0.01 | 0.32±0.00 | 0.32±0.00 | 0.33±0.00 | ||
FIRST | Mild | 0.43±0.04 | 0.53±0.02 | 0.60±0.02 | 0.62±0.04 | 0.64±0.04 | 0.64±0.01 | |
Standard | 0.39±0.03 | 0.50±0.02 | 0.58±0.02 | 0.60±0.04 | 0.63±0.03 | 0.63±0.01 | ||
Strong | 0.35±0.01 | 0.45±0.01 | 0.52±0.01 | 0.56±0.02 | 0.59±0.02 | 0.59±0.01 | ||
AiCE | Mild | 0.26±0.01 | 0.35±0.01 | 0.37±0.01 | 0.42±0.00 | 0.43±0.00 | 0.44±0.05 | |
Standard | 0.29±0.01 | 0.41±0.00 | 0.47±0.01 | 0.49±0.00 | 0.51±0.01 | 0.53±0.00 | ||
Strong | 0.31±0.00 | 0.46±0.01 | 0.51±0.01 | 0.55±0.01 | 0.57±0.01 | 0.58±0.00 |
Values are expressed as mean ± standard deviation. AIDR 3D, Adaptive Iterative Dose Reduction 3D; AiCE, Advanced intelligent Clear-IQ Engine; CTDIvol, volume computed tomography dose index; FIRST, Forward projected model-based Iterative Reconstruction SoluTion; TTF50%, values of Task-based transfer function at fifty percent.
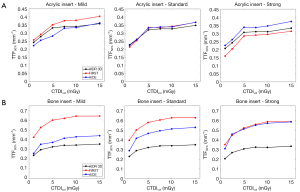
For acrylic insert (Figure 3A), TTF50% were higher with AiCE than with AIDR 3D and FIRST for Strong level but lower for the level Mild (except at 15 mGy). The opposite pattern was found for FIRST compared to AIDR 3D. Similar TTF50% values were found for the Standard level for all algorithms.
Higher TTF50% differences as function of IR algorithms were found for bone insert than for acrylic insert (Figure 3B). Highest values of TTF50% were found for FIRST except for the level Strong and lowest values were found for AIDR 3D. Differences of TTF50% values between AiCE and AIDR 3D increased as the IR/DLR level increased (18%±6% using Mild and 68%±10% using Strong). The opposite pattern was found between AiCE and FIRST (−35%±3% using Mild and −3%±5% using Strong). Compared to AIDR 3D, values of TTF50% were higher with FIRST by an average of 77%±6%.
Detectability index
Table 3 and Figure 4 depicts the values of detectability index (d') for both features, using all algorithms and at all dose levels. d' increased as dose increased and the IR/DLR levels increased.
Table 3
Detectability index outcomes | Algorithms | Levels | CTDIvol (mGy) | |||||
---|---|---|---|---|---|---|---|---|
1.0 | 2.5 | 5.0 | 7.5 | 10.0 | 15.0 | |||
Large feature (25 mm and 500 HU) | AIDR 3D | Mild | 4.7±0.2 | 5.9±0.3 | 7.6±0.5 | 8.7±0.6 | 9.6±0.7 | 11.0±0.8 |
Standard | 5.2±0.2 | 6.4±0.3 | 8.1±0.6 | 9.2±0.6 | 10.2±0.7 | 11.9±0.9 | ||
Strong | 6.0±0.2 | 7.1±0.4 | 8.9±0.6 | 10.1±0.6 | 10.9±0.8 | 12.7±0.9 | ||
FIRST | Mild | 3.7±0.4 | 5.1±0.4 | 6.6±0.5 | 7.6±0.6 | 8.7±0.8 | 10.9±0.9 | |
Standard | 5.1±0.7 | 6.5±0.5 | 8.0±0.6 | 9.1±0.8 | 10.4±1.1 | 12.9±1.1 | ||
Strong | 6.7±1.4 | 8.9±1.3 | 11.0±1.0 | 12.3±1.3 | 13.1±1.6 | 14.8±1.4 | ||
AiCE | Mild | 5.8±0.3 | 7.3±0.4 | 9.4±0.6 | 10.6±0.7 | 11.6±0.8 | 13.9±1.1 | |
Standard | 6.4±0.3 | 8.3±0.4 | 10.8±0.6 | 12.1±0.7 | 12.8±0.9 | 14.4±1.0 | ||
Strong | 8.5±0.3 | 9.4±0.4 | 11.8±0.7 | 12.9±0.8 | 13.5±0.9 | 16.7±1.0 | ||
Small feature (1.5 mm and 120 HU) | AIDR 3D | Mild | 2.1±0.0 | 3.1±0.0 | 4.2±0.0 | 5.0±0.0 | 5.7±0.0 | 6.1±0.0 |
Standard | 2.3±0.0 | 3.3±0.0 | 4.6±0.1 | 5.3±0.0 | 6.0±0.0 | 6.5±0.0 | ||
Strong | 2.4±0.0 | 3.5±0.0 | 4.8±0.1 | 5.6±0.0 | 6.3±0.0 | 6.9±0.0 | ||
FIRST | Mild | 3.2±0.2 | 4.7±0.2 | 5.8±0.1 | 6.8±0.3 | 7.9±0.2 | 8.6±0.4 | |
Standard | 4.2±0.6 | 6.4±0.4 | 7.6±0.3 | 8.6±0.1 | 10±0.4 | 10.8±0.5 | ||
Strong | 6.5±2.0 | 8.4±1.5 | 11.2±0.9 | 12.6±0.7 | 14±0.9 | 15.7±0.7 | ||
AiCE | Mild | 3.2±0.0 | 4.9±0.1 | 6.7±0.1 | 7.6±0.0 | 8.6±0.0 | 8.8±0.8 | |
Standard | 4.5±0.1 | 6.5±0.1 | 8.9±0.1 | 9.8±0.0 | 10.6±0.1 | 11.1±0.0 | ||
Strong | 4.9±0.1 | 7.6±0.1 | 10.4±0.1 | 11.3±0.0 | 11.9±0.1 | 11.8±0.0 |
Values are expressed as mean ± standard deviation. AIDR 3D, Adaptive Iterative Dose Reduction 3D; AiCE, Advanced intelligent Clear-IQ Engine; CTDIvol, volume computed tomography dose index; FIRST, Forward projected model-based Iterative Reconstruction SoluTion.
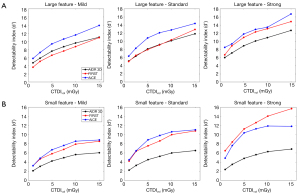
For the large feature (Figure 4A), d' values were higher with AiCE than with AIDR 3D (27%±6%) and with FIRST (33%±12%) for all IR/DLR levels. Differences of d' values between AiCE and FIRST decreased as the IR/DLR level increased. Variations of d' values between FIRST and AIDR 3D were different as function of the IR level: −12%±7% for Mild, 1%±4% for Standard and 20%±5% for Strong.
For the small feature (Figure 4B), d' values were higher with AiCE than with AIDR 3D and with FIRST (except for the level Strong). Differences of d' values between AiCE and AIDR 3D increased as the IR/DLR level increased. A similar pattern was found between FIRST and AIDR 3D.
Potential increase in d' and potential dose variation
Table 4 shows that the detectability of all features increased as AiCE level increased. For the small feature, the increase in d' was in the same range for FIRST and AiCE. For the large feature, the increase in d' with FIRST only occurred when the level Strong was used.
Table 4
Algorithms | Levels | Increase in d’ (%) | Dose variation (%) | |||
---|---|---|---|---|---|---|
Small | Large | Small | Large | |||
FIRST | Mild | 32 | −14 | −46 | 33 | |
Standard | 67 | 2 | −78 | −4 | ||
Strong | 134 | 29 | −94 | −60 | ||
AiCE | Mild | 43 | 14 | −59 | −33 | |
Standard | 77 | 26 | −79 | −57 | ||
Strong | 98 | 32 | −84 | −67 |
The small feature represented a circular signal with a pre-imaged contrast of 500 HU and a diameter of 1.5 mm, while the large feature was defined with a contrast of 120 HU and a diameter of 25 mm. AIDR 3D, Adaptive Iterative Dose Reduction 3D; AiCE, Advanced intelligent Clear-IQ Engine; d’, detectability index; FIRST, Forward projected model-based Iterative Reconstruction SoluTion; CTDIvol, volume computed tomography dose index.
Using AiCE indicates a potential to reduce the CTDIvol for all features. Potential dose variation was slightly higher for the small than for the large feature. Using FIRST indicates a potential to reduce the CTDIvol for all features, except for the large feature with Mild and Standard levels.
Visual assessment of image quality
Figure 5 depicts the image quality obtained with the acrylic insert at each dose level and with all IR algorithms.
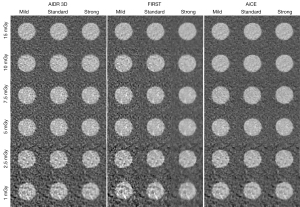
For all algorithms, the image noise increased as the dose decreased. For all dose levels, the image noise decreased but the images were more smoothed when the IR/DLR level increased, especially with FIRST. Images obtained with AiCE were less noisy than AIDR 3D images and were less smooth than FIRST images, which improved the insert detectability
Additional results obtained for NPS, TTF and d' values for FBP and for AIDR 3D and FIRST algorithms using the Standard level at 1-mm slice thickness are presented in the Supplementary file. In addition, outcomes of HU values for both inserts and NPS graphs for all reconstruction types, dose and IR/DLR levels are presented within this file.
Discussion
We compared the new DLR algorithm AiCE with the hybrid IR algorithm AIDR 3D and the model-based IR FIRST algorithms and demonstrated that AiCE reduced image noise substantially. Compared to AIDR 3D, AiCE either improved or maintained the spatial resolution and improved the signal detectability without perceptible alteration of the noise/image texture. Also, compared to FIRST, AiCE improved the detectability and the image texture avoiding overly enforced smoothness, which is usually high with FIRST algorithm in a uniform background phantom.
Our NPS outcomes as function of dose levels and IR/DLR levels were similar to those previously reported for HIR and MBIR algorithms (3). The dose increase reduced the image noise (NPS peak) and shifted the NPS average spatial frequency (fav) towards higher frequencies. For all algorithms, the image noise (NPS peak) decreased and the fav shifted towards lower frequencies as the IR/DLR level increased. More the fav shifted towards lower frequencies, more the image texture change (such as image smoothness). Compared to AIDR 3D and FIRST, AiCE reduced the image noise for all IR/DLR levels. Similar variations between AIDR 3D and AiCE were found by Higaki et al. (28) but a different reconstruction kernel was used with AIDR 3D (FC08 vs. FC13). However, they found that image noise was more reduced using AiCE than FIRST only for low dose levels (CTDIvol <6 mGy) for the same reconstruction level (Standard). In addition, Higaki et al. (28) computed their NPS on a cylindrical 200-mm diameter phantom created with a 3D printer and made of acrylic as background material with a higher HU value than the water equivalent as background material used in our study. Compared to AIDR 3D, the fav shifted towards lower frequencies but the differences between both algorithms decreased as the IR/DLR level increased. Indeed, using AiCE, fav values were in the same range for the three DLR levels (−6%±2% from Mild to Strong) while these values are lower when IR level increased with AIDR 3D (−11%±2%, respectively). Compared to FIRST, fav values were higher for Standard and Strong levels but the opposite for Mild level. Using FIRST, the fav values variations as function of IR level were higher than other algorithms (−28%±2%). The lack of the fav values in the Higaki et al. (28) study does not allow putting our results in perspective. Visual assessment of image quality confirmed our NPS outcomes for noise magnitude and noise texture. Images obtained with AiCE were less noisy than AIDR 3D images and were less smooth than FIRST images.
TTF outcomes shows that TTF values shifted towards higher frequencies as the dose increased. For AIDR 3D and FIRST algorithms, TTF values shifted towards lower frequencies as the IR level increased in particular for MBIR. Similar TTF variations as function of dose and IR levels were previously reported for HIR and MBIR algorithms (3). With AiCE, TTF values shifted towards higher frequencies as the DLR level increased. The variations of TTF values as function of IR or DLR levels were greater with the bone insert than the acrylic insert. These results were related to the non-linear properties of IR/MBIR and probably DLR algorithms, which make the spatial resolution dependent on dose (and also image noise) and contrast. With a lower noise level, TTF outcomes obtained with DLR algorithm was less marked by the dose reduction than with IR algorithms. Otherwise, TTF values of AiCE were higher than those obtained with AIDR 3D, except for Mild level with acrylic insert. The decrease of TTF values using FIRST and the increase of TTF values as the IR or DLR level increased leads to insert-dependent variations between the two algorithms. For bone insert, TTF values with FIRST were higher than AiCE but the differences between both algorithms decreased as the IR or DLR level increased. For acrylic insert, TTF values were higher with AiCE than FIRST for Standard and Strong levels. Higaki et al. found that the TTF values obtained with AiCE for the task contrast of 100 HU close to the acrylic insert were higher than AIDR 3D but lower than FIRST (28). These differences between AiCE and FIRST may be explained by the differences between the phantoms used in particular material of the bottom. Indeed, Higaki et al. used a phantom with inserts placed inside an acrylic background material while we used in the present study inserts placed inside a water equivalent as background material. Lower contrast difference between the insert and the background material impacted the slope of the ESF and the TTF outcomes. The calculated outcomes of TTF values for acrylic insert were visually confirmed on images. The visual image quality assessment showed that with a lowest noise level, the visual border detection (spatial resolution) of acrylic insert was higher for AiCE than AIDR 3D and for AiCE than FIRST, particularly for the lowest dose level and the highest IR/DLR levels.
Detectability index outcomes show that d' values were higher with AiCE than AIDR 3D for all features and all IR/DLR levels. A similar pattern was found between AiCE and FIRST, except for the small feature with the level Strong. These outcomes were directly correlated with the NPS and TTF outcomes, in particular the NPS peak variations. Highest d' values were obtained using AiCE because the lowest values of NPS peak were found with this algorithm, despite fav and TTF50% values not always being highest. The highest d' values obtained using FIRST than AiCE for the small feature with the level Strong were related to the highest TTF values for the bone insert of both algorithms. Higaki et al. found that the d' values obtained with AiCE for the task contrast of 100 HU close to the contrast of the large feature were higher than AIDR 3D for all dose levels but lower than with FIRST for high dose levels, and the opposite for low dose levels (28). These differences can be explained by differences between the phantoms used as defined previously but also the differences between the defined clinical tasks. Higaki et al. used a smaller size (3–5 vs. 25 mm) and lower contrast (100 vs. 120 HU) clinical tasks than the characteristics of the large feature. The orders of magnitude of the d' values between our study and the Higaki study are also very different. Higaki et al. used the same methodology as that used in our study, i.e., that developed by Samei et al. (37), however, they do not give detailed information on the calculation method of d' (observer model used, interpretation conditions defined, diameter of the clinical tasks, software used). This therefore limits the comparison of the d' values.
Finally, the results presented in this study show that AiCE corrects the limitations of the AIDR 3D and FIRST algorithms. Indeed, the use of AiCE makes it possible to greatly reduce noise compared to AIDR 3D without compromising image quality, except for the small feature and Strong level. This improvement in image quality leads to better detectability of low- and high-contrast lesions and offers good prospects for optimization in clinical routine. However, many studies have shown that the potential dose reduction obtained with IR algorithms was limited to low contrast liver lesions and the outcomes found on phantoms overestimated the real dose reduction in patients (44-46). Nevertheless, the first patient studies published on pulmonary or cardiac CT angiography and chest and abdominal CT have also confirmed that AiCE improved both image quality and lesion detection as compared with AIDR 3D for a given dose level (22,25,26,30) or with a dose reduction (26,29,34,35). Singh et al. found that the dose could be reduced by −84% between a standard protocol (CTDIvol: 13.0±4.4 mGy) with AIDR 3D and a low-dose protocol (CTDIvol: 2.1±0.8 mGy) with AiCE for the detection of the same abdominal lesions and an overall image quality scored acceptable for more than 95% of patients (34). We found a lower dose reduction compared to this study for the large feature using the Standard (CTDIvol: 4.4 mGy) and Strong (CTDIvol: 3.3 mGy) AiCE levels. In addition to being less noisy, the images obtained with AiCE are less smooth compared to FIRST (except for the Mild level), which should facilitate its use in clinical routine. Indeed, in addition to having a long reconstruction time, AIDR 3D is often preferred to FIRST in clinical practice because of a greater smoothing of the image which impedes the radiologist in interpreting the images (17). The use of AiCE therefore improves the overall image appearance qualitatively but also quantitatively, since the detectability of simulated lesions at low and high contrast was improved. This could facilitate clinical practice, and be used to optimize protocols or to reduce patient dose. Furthermore, the results of the potential dose reduction in this study should be toned down because we used a simple phantom that did not accurately represent the patient morphology or tissue attenuation for the abdomen. In addition, as the DNN of AiCE is trained with patient data only, our outcomes must be validated in patients. Further patient studies on abdominal CT images could be performed to confirm these results found on phantom.
This study has several limitations. It was performed on only one CT scan with specific acquisition and reconstruction parameters. Based on the AiCE limits for abdominal images, only a soft tissue kernel and a slice thickness 0.5-mm thick were used. All body regions kernels could be proposed by the manufacturer for the next version of AiCE (2nd version). AIDR 3D images were reconstructed with a different reconstruction kernel compared to that of FIRST and AiCE algorithms. Specific parameters for each clinical indication are necessary to further evaluate this DLR algorithm and compare it with the other DLR algorithm available (23). Also, the visual image quality assessment was limited and could be improved in further studies with true assessment of image quality by human observers. In addition, the phantom used in this study did not take into account the variety of patient’s body morphologies or tissue textures. The phantom materials do not represent the composition of human tissue which may be a handicap for the assessment of the DLR method under study, as it was only trained on patient data and was limited to low contrast resolution detectability studies (HU values lower than 120 HU). Last, further research could be performed to assess the NPS in the z direction or using an anthropomorphic phantom including 3D printing options in order to be closer to the patient's body morphology or tissue texture for image quality assessment of DLR algorithms (47,48).
Conclusions
We found that the new DLR algorithm AiCE generates images with less noise compared to AIDR 3D or FIRST algorithms while simultaneously avoiding unrealistically smooth images provided by FIRST algorithm. When used in a phantom, it also demonstrated higher detectability than two other algorithms (except for the small feature and Strong level using AIDR 3D). This shows that this DLR algorithm has potential to be used in clinical practice, especially in dose optimization. Further patient studies need to be conducted under clinical conditions to confirm these findings on phantom.
Acknowledgments
We thank Sarah Kabani and Hélène de Forges for their help in editing the manuscript. We thank Karim Haioun, Christiana Balta and Yannick Fuamba for their support.
Funding: None.
Footnote
Conflicts of Interest: All authors have completed the ICMJE uniform disclosure form (available at https://dx.doi.org/10.21037/qims-21-215). The authors have no conflicts of interest to declare.
Ethical Statement: The authors are accountable for all aspects of the work in ensuring that questions related to the accuracy or integrity of any part of the work are appropriately investigated and resolved. This is a phantom study, ethical approval and written informed consent are not required.
Open Access Statement: This is an Open Access article distributed in accordance with the Creative Commons Attribution-NonCommercial-NoDerivs 4.0 International License (CC BY-NC-ND 4.0), which permits the non-commercial replication and distribution of the article with the strict proviso that no changes or edits are made and the original work is properly cited (including links to both the formal publication through the relevant DOI and the license). See: https://creativecommons.org/licenses/by-nc-nd/4.0/.
References
- Willemink MJ, Noël PB. The evolution of image reconstruction for CT-from filtered back projection to artificial intelligence. Eur Radiol 2019;29:2185-95. [Crossref] [PubMed]
- Verdun FR, Racine D, Ott JG, Tapiovaara MJ, Toroi P, Bochud FO, Veldkamp WJH, Schegerer A, Bouwman RW, Giron IH, Marshall NW, Edyvean S. Image quality in CT: From physical measurements to model observers. Phys Med 2015;31:823-43. [Crossref] [PubMed]
- Greffier J, Frandon J, Larbi A, Beregi JP, Pereira F. CT iterative reconstruction algorithms: a task-based image quality assessment. Eur Radiol 2020;30:487-500. [Crossref] [PubMed]
- Thibault JB, Sauer KD, Bouman CA, Hsieh J. A three-dimensional statistical approach to improved image quality for multislice helical CT. Med Phys 2007;34:4526-44. [Crossref] [PubMed]
- McCollough CH, Yu L, Kofler JM, Leng S, Zhang Y, Li Z, Carter RE. Degradation of CT Low-Contrast Spatial Resolution Due to the Use of Iterative Reconstruction and Reduced Dose Levels. Radiology 2015;276:499-506. [Crossref] [PubMed]
- Beregi JP, Greffier J. Low and ultra-low dose radiation in CT: Opportunities and limitations. Diagn Interv Imaging 2019;100:63-4. [Crossref] [PubMed]
- Greffier J, Frandon J, Pereira F, Hamard A, Beregi JP, Larbi A, Omoumi P. Optimization of radiation dose for CT detection of lytic and sclerotic bone lesions: a phantom study. Eur Radiol 2020;30:1075-8. [Crossref] [PubMed]
- Larbi A, Orliac C, Frandon J, Pereira F, Ruyer A, Goupil J, Macri F, Beregi JP, Greffier J. Detection and characterization of focal liver lesions with ultra-low dose computed tomography in neoplastic patients. Diagn Interv Imaging 2018;99:311-20. [Crossref] [PubMed]
- Macri F, Greffier J, Khasanova E, Claret PG, Bastide S, Larbi A, Bobbia X, Pereira FR, de la Coussaye JE, Beregi JP. Minor Blunt Thoracic Trauma in the Emergency Department: Sensitivity and Specificity of Chest Ultralow-Dose Computed Tomography Compared With Conventional Radiography. Ann Emerg Med 2019;73:665-70. [Crossref] [PubMed]
- Ott JG, Becce F, Monnin P, Schmidt S, Bochud FO, Verdun FR. Update on the non-prewhitening model observer in computed tomography for the assessment of the adaptive statistical and model-based iterative reconstruction algorithms. Phys Med Biol 2014;59:4047-64. [Crossref] [PubMed]
- Yamada Y, Jinzaki M, Hosokawa T, Tanami Y, Sugiura H, Abe T, Kuribayashi S. Dose reduction in chest CT: comparison of the adaptive iterative dose reduction 3D, adaptive iterative dose reduction, and filtered back projection reconstruction techniques. Eur J Radiol 2012;81:4185-95. [Crossref] [PubMed]
- Maamoun I, Khalil MM. Assessment of iterative image reconstruction on kidney and liver donors: Potential role of adaptive iterative dose reduction 3D (AIDR 3D) technology. Eur J Radiol 2018;109:124-9. [Crossref] [PubMed]
- Nakamoto A, Yamamoto K, Sakane M, Nakai G, Higashiyama A, Juri H, Yoshikawa S, Narumi Y. Reduction of the radiation dose and the amount of contrast material in hepatic dynamic CT using low tube voltage and adaptive iterative dose reduction 3-dimensional. Medicine (Baltimore) 2018;97:e11857 [Crossref] [PubMed]
- Paruccini N, Villa R, Pasquali C, Spadavecchia C, Baglivi A, Crespi A. Evaluation of a commercial Model Based Iterative reconstruction algorithm in computed tomography. Phys Med 2017;41:58-70. [Crossref] [PubMed]
- Rotzinger DC, Racine D, Beigelman-Aubry C, Alfudhili KM, Keller N, Monnin P, Verdun FR, Becce F. Task-Based Model Observer Assessment of A Partial Model-Based Iterative Reconstruction Algorithm in Thoracic Oncologic Multidetector CT. Sci Rep 2018;8:17734. [Crossref] [PubMed]
- Solomon J, Wilson J, Samei E. Characteristic image quality of a third generation dual-source MDCT scanner: Noise, resolution, and detectability. Med Phys 2015;42:4941-53. [Crossref] [PubMed]
- Laurent G, Villani N, Hossu G, Rauch A, Noël A, Blum A, Gondim Teixeira PA. Full model-based iterative reconstruction (MBIR) in abdominal CT increases objective image quality, but decreases subjective acceptance. Eur Radiol 2019;29:4016-25. [Crossref] [PubMed]
- Geyer LL, Schoepf UJ, Meinel FG, Nance JW Jr, Bastarrika G, Leipsic JA, Paul NS, Rengo M, Laghi A, De Cecco CN. State of the Art: Iterative CT Reconstruction Techniques. Radiology 2015;276:339-57. [Crossref] [PubMed]
- Franck C, Zhang G, Deak P, Zanca F. Preserving image texture while reducing radiation dose with a deep learning image reconstruction algorithm in chest CT: A phantom study. Phys Med 2021;81:86-93. [Crossref] [PubMed]
- Hsieh J, Liu E, Nett B, Tang J, Thibault JB, Sahney S. A new era of image reconstruction: TrueFidelity™. Technical white paper on deep learning image reconstruction, 2019.
- Boedeker K. AiCE Deep Learning Reconstruction: Bringing the power of Ultra-High Resolution CT to routine imaging. Technical white paper on deep learning reconstruction Canon Medical system, 2019.
- Akagi M, Nakamura Y, Higaki T, Narita K, Honda Y, Zhou J, Yu Z, Akino N, Awai K. Deep learning reconstruction improves image quality of abdominal ultra-high-resolution CT. Eur Radiol 2019;29:6163-71. [Crossref] [PubMed]
- Wu D, Kim K, Li Q. Computationally efficient deep neural network for computed tomography image reconstruction. Med Phys 2019;46:4763-76. [Crossref] [PubMed]
- Nakamura Y, Higaki T, Tatsugami F, Zhou J, Yu Z, Akino N, Ito Y, Iida M, Awai K. Deep Learning-based CT Image Reconstruction: Initial Evaluation Targeting Hypovascular Hepatic Metastases. Radiol Artif Intell 2019;1:e180011 [Crossref] [PubMed]
- Tatsugami F, Higaki T, Nakamura Y, Yu Z, Zhou J, Lu Y, Fujioka C, Kitagawa T, Kihara Y, Iida M, Awai K. Deep learning-based image restoration algorithm for coronary CT angiography. Eur Radiol 2019;29:5322-9. [Crossref] [PubMed]
- Lenfant M, Chevallier O, Comby PO, Secco G, Haioun K, Ricolfi F, Lemogne B, Loffroy R. Deep Learning Versus Iterative Reconstruction for CT Pulmonary Angiography in the Emergency Setting: Improved Image Quality and Reduced Radiation Dose. Diagnostics (Basel) 2020;10:558. [Crossref] [PubMed]
- Akagi M, Nakamura Y, Higaki T, Narita K, Honda Y, Awai K. Deep learning reconstruction of equilibrium phase CT images in obese patients. Eur J Radiol 2020;133:109349 [Crossref] [PubMed]
- Higaki T, Nakamura Y, Zhou J, Yu Z, Nemoto T, Tatsugami F, Awai K. Deep Learning Reconstruction at CT: Phantom Study of the Image Characteristics. Acad Radiol 2020;27:82-7. [Crossref] [PubMed]
- Bernard A, Comby PO, Lemogne B, Haioun K, Ricolfi F, Chevallier O, Loffroy R. Deep learning reconstruction versus iterative reconstruction for cardiac CT angiography in a stroke imaging protocol: reduced radiation dose and improved image quality. Quant Imaging Med Surg 2021;11:392-401. [Crossref] [PubMed]
- Bernard A, Leclercq T, Comby PO, Duloquin G, Ricolfi F, Béjot Y, Guenancia C. High rate of cardiac thrombus diagnosed by adding cardiac imaging in acute stroke computed tomography protocol. Int J Stroke 2021;16:692-700. [Crossref] [PubMed]
- Greffier J, Hamard A, Pereira F, Barrau C, Pasquier H, Beregi JP, Frandon J. Image quality and dose reduction opportunity of deep learning image reconstruction algorithm for CT: a phantom study. Eur Radiol 2020;30:3951-9. [Crossref] [PubMed]
- Racine D, Becce F, Viry A, Monnin P, Thomsen B, Verdun FR, Rotzinger DC. Task-based characterization of a deep learning image reconstruction and comparison with filtered back-projection and a partial model-based iterative reconstruction in abdominal CT: A phantom study. Phys Med 2020;76:28-37. [Crossref] [PubMed]
- Solomon J, Lyu P, Marin D, Samei E. Noise and spatial resolution properties of a commercially available deep learning-based CT reconstruction algorithm. Med Phys 2020;47:3961-71. [Crossref] [PubMed]
- Singh R, Digumarthy SR, Muse VV, Kambadakone AR, Blake MA, Tabari A, Hoi Y, Akino N, Angel E, Madan R, Kalra MK. Image Quality and Lesion Detection on Deep Learning Reconstruction and Iterative Reconstruction of Submillisievert Chest and Abdominal CT. AJR Am J Roentgenol 2020;214:566-73. [Crossref] [PubMed]
- Brady SL, Trout AT, Somasundaram E, Anton CG, Li Y, Dillman JR. Improving Image Quality and Reducing Radiation Dose for Pediatric CT by Using Deep Learning Reconstruction. Radiology 2021;298:180-8. [Crossref] [PubMed]
- Samei E, Bakalyar D, Boedeker KL, Brady S, Fan J, Leng S, Myers KJ, Popescu LM, Ramirez Giraldo JC, Ranallo F, Solomon J, Vaishnav J, Wang J. Performance evaluation of computed tomography systems: Summary of AAPM Task Group 233. Med Phys 2019;46:e735-56. [Crossref] [PubMed]
- Samei E, Richard S. Assessment of the dose reduction potential of a model-based iterative reconstruction algorithm using a task-based performance metrology. Med Phys 2015;42:314-23. [Crossref] [PubMed]
- Christianson O, Chen JJ, Yang Z, Saiprasad G, Dima A, Filliben JJ, Peskin A, Trimble C, Siegel EL, Samei E. An Improved Index of Image Quality for Task-based Performance of CT Iterative Reconstruction across Three Commercial Implementations. Radiology 2015;275:725-34. [Crossref] [PubMed]
- Solomon JB, Christianson O, Samei E. Quantitative comparison of noise texture across CT scanners from different manufacturers. Med Phys 2012;39:6048-55. [Crossref] [PubMed]
- Richard S, Husarik DB, Yadava G, Murphy SN, Samei E. Towards task-based assessment of CT performance: system and object MTF across different reconstruction algorithms. Med Phys 2012;39:4115-22. [Crossref] [PubMed]
- Eckstein M, Bartroff J, Abbey C, Whiting J, Bochud F. Automated computer evaluation and optimization of image compression of x-ray coronary angiograms for signal known exactly detection tasks. Opt Express 2003;11:460-75. [Crossref] [PubMed]
- Burgess AE, Li X, Abbey CK. Visual signal detectability with two noise components: anomalous masking effects. J Opt Soc Am A Opt Image Sci Vis 1997;14:2420-42. [Crossref] [PubMed]
- Burgess AE, Wagner RF, Jennings RJ, Barlow HB. Efficiency of human visual signal discrimination. Science 1981;214:93-4. [Crossref] [PubMed]
- Baker ME, Dong F, Primak A, Obuchowski NA, Einstein D, Gandhi N, Herts BR, Purysko A, Remer E, Vachhani N. Contrast-to-noise ratio and low-contrast object resolution on full- and low-dose MDCT: SAFIRE versus filtered back projection in a low-contrast object phantom and in the liver. AJR Am J Roentgenol 2012;199:8-18. [Crossref] [PubMed]
- McCollough CH, Bartley AC, Carter RE, Chen B, Drees TA, Edwards P, Holmes DR 3rd, Huang AE, Khan F, Leng S, McMillan KL, Michalak GJ, Nunez KM, Yu L, Fletcher JG. Low-dose CT for the detection and classification of metastatic liver lesions: Results of the 2016 Low Dose CT Grand Challenge. Med Phys 2017;44:e339-52. [Crossref] [PubMed]
- Mileto A, Zamora DA, Alessio AM, Pereira C, Liu J, Bhargava P, et al. CT Detectability of Small Low-Contrast Hypoattenuating Focal Lesions: Iterative Reconstructions versus Filtered Back Projection. Radiology 2018;289:443-54. [Crossref] [PubMed]
- Filippou V, Tsoumpas C. Recent advances on the development of phantoms using 3D printing for imaging with CT, MRI, PET, SPECT, and ultrasound. Med Phys 2018; Epub ahead of print. [Crossref] [PubMed]
- Valladares A, Beyer T, Rausch I. Physical imaging phantoms for simulation of tumor heterogeneity in PET, CT, and MRI: An overview of existing designs. Med Phys 2020;47:2023-37. [Crossref] [PubMed]