Ability of weakly supervised learning to detect acute ischemic stroke and hemorrhagic infarction lesions with diffusion-weighted imaging
Introduction
Acute ischemic stroke (AIS) is the leading cause of death in China. The age-standardized prevalence, incidence, and mortality rates of AIS in China are 111, 4.8, 246.8, and 114.8/100,000 person-years, respectively (1). The latest guidelines recommend imaging evaluations before and after treatment, with particular attention given to the occurrence of hemorrhagic transformation (2). For spontaneous or therapy-related hemorrhagic transformation, missed diagnosis should be avoided to improve the prognosis of patients (3).
Diffusion-weighted imaging (DWI) should be routinely performed for patients who are clinically suspected of having AIS lesions. DWI allows for sensitive visualization of AIS lesions; however, it may prevent the accurate identification of small-sized lesions or those disturbed by various artifacts due to the location (4). The gradient-recalled echo (GRE) sequence provides an accurate assessment of hemorrhagic transformation and is even superior to computed tomography (CT) (5,6). Unfortunately, GRE is not routinely performed, especially in low- and middle-income cities in China. In addition, GRE normally takes several minutes, which can prolong the examination time for AIS patients. Previous studies have found that b0 images exhibit comparable sensitivities to those of GRE (7). However, due to its lower spatial resolution, b0 images have less ability than does GRE in identifying hemorrhagic transformation by human visual assessment. Moreover, a hemorrhagic infarction (HI) is more difficult to identify than parenchymal hematoma, which indicates the presence of petechiae within the infarcted area without a space-occupying effect (8).
Numerous fully supervised approaches, such as EDD-Net (9), 3D-DenseNet (10), and the residual-structured fully convolutional network (11), have been successfully applied in AIS lesion detection. However, the fully labeled annotation of lesions from a large number of images is tremendously labor and time intensive. Recently, some weakly supervised approaches were proposed to leverage the annotation workload (12-14), such as the wiseDNN (15) and the 3D weakly supervised network (16). The related studies have indicated that weakly supervised approaches can reduce the difficulty of label acquisition while still maintaining high detection efficiency.
We hypothesized that weakly supervised learning may be helpful in detecting and locating small AIS lesions, and can simultaneously identify the concurrent HI lesions based on DWI. To this end, we first developed 2 weakly supervised methods to determine small AIS lesions in different positions. We then chose the better one to identify HI lesions and compared its performance with results from human reading.
Methods
Patient selection and data collection
This study was conducted in accordance with the Declaration of Helsinki (as revised in 2013) and was approved by the Ethics Board of Tianjin Huanhu Hospital. Individual consent for this retrospective analysis was waived. All clinical images of consecutive patients were collected from a retrospective database between January 1, 2017, and October 30, 2018.
The overall flow chart of this study is shown in Figure 1. First, a total of 417 training patients were randomly sampled from the retrospective database, in which eligibility for inclusion was AIS within 3 days from the onset of symptoms and available DWI images. The magnetic resonance imaging (MRI) data were screened for severe motion artifacts. To evaluate the performance of the weakly supervised approaches spatially locating the small lesions in different positions, a total of 240 patients in the test set were carefully selected. Lacunar stroke is a typical type of small stroke lesion, which is regarded as an important marker of cerebral small vessel disease and predicts unfavorable long-term outcomes. According to the current stroke classification systems, the definition of lacunar stroke relies mainly on the lesion radius limit (<7.5 mm) on brain imaging (17). Therefore, a patient was categorized as a test subject if the lesion was singular and its radius was smaller than 7.5 mm. The 240 test subjects were further divided into three groups according to the lesion positions on the basal ganglia, pons, and centrum semiovale, with 80 subjects in each group. We used an AIS patient data set to measure the performance of the weakly supervised approaches spatially locating the small lesions (radius <7.5 mm) in different positions.
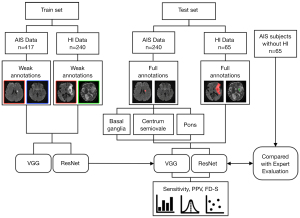
Next, we further identified 305 AIS patients with HI lesions and 65 AIS patients without HI lesions (non-HI group) from the retrospective database. The HI lesions confirmed by two neuroradiologists using GRE sequences were considered to be the gold standard. In each case, GRE images were independently reviewed in conjunction with the isotropic DWI scan. HI was defined as an area of low signal intensity to cortical gray matter on GRE images. Of the 305 AIS subjects with HI lesions, 240 were used to train and 65 were used to test the approach. In other words, the training set included 240 AIS subjects with HI lesions, and the test set included 130 AIS subjects (65 subjects with HI lesions and 65 subjects without HI lesions). There were no significant differences between the HI test group and the non-HI group in baseline characteristics of age, sex, or AIS lesion volume.
Image acquisition
MRI measurements were acquired from three MR scanners, with two 3T MR scanners (Skyra and Trio, Siemens, Erlangen, Germany) and one 1.5 T MR scanner (Avanto, Siemens, Erlangen, Germany). DWI images were acquired using a spin-echo type echo-planar (SE-EPI) sequence with b values of 0 and 1,000 s/mm2. Following acquisition, apparent diffusion coefficient (ADC) maps were calculated from the diffusion scan raw data in a pixel-by-pixel manner. The detailed parameters of GRE and DWI are summarized in Table 1.
Table 1
Parameters | Skyra | Trio | Avanto | |||||
---|---|---|---|---|---|---|---|---|
DWI | GRE | DWI | GRE | DWI | GRE | |||
Repetition (ms) | 5,200 | 220 | 3,100 | 566 | 3,800 | 576 | ||
Echo time (ms) | 80 | 2.46 | 99 | 20 | 102 | 20.4 | ||
Number of excitations | 1 | 1 | 3 | 1 | 3 | 1 | ||
Field of view (mm2) | 240×240 | 240×240 | 200×200 | 230×230 | 240×240 | 240×240 | ||
Matrix size | 130×130 | 180×288 | 132×132 | 166×256 | 192×192 | 173×256 | ||
Slice thickness (mm) | 5 | 5 | 6 | 6 | 5 | 5 | ||
Slice spacing (mm) | 1.5 | 1.5 | 1.8 | 1.8 | 1.5 | 1.5 | ||
Number of slices | 21 | 21 | 17 | 17 | 21 | 21 |
MRI, magnetic resonance imaging; DWI, diffusion-weighted imaging; GRE, gradient-recalled echo.
Lesion annotation
The lesions were annotated with different methods. As shown in Figure 2, subjects in the training set were annotated by weak labels, where each slice of the subjects was annotated as “with AIS lesion”, “with AIS and HI lesion”, or “without lesion”. Subjects in the testing set were annotated by full labels, where all of the lesions were annotated in a pixel-by-pixel manner for evaluation only. In our work, we used the slice-level label to perform lesion-level localization and detection. Identification of AIS lesions was performed as regions of ADC <620×10−6 mm2/s. The HI lesions were manually annotated by inspecting the GRE images. The labels were annotated by an experienced expert (Dr. Cao, a radiologist with 8 years of neuroimaging experience). The expert performed the manual annotation of the train and test data twice, and the interobserver agreement was assessed. Manual annotation of the first trial served as the ground truth. Another experienced expert checked the labels (Dr. Jin, a radiologist with 20 years of neuroimaging experience). The full and weak labeling times were recorded. Annotated labels were further tallied for statistical analysis (Tables 2,3).
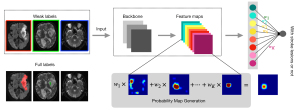
Table 2
Training set | AIS data (n=417) | HI data (n=240) |
---|---|---|
Age, mean [min–max] | 62 [31–86] | 68 [24–93] |
Male sex, n (%) | 218 (52.1) | 124 (51.6) |
Classification, number of slices (%) | ||
Normal | 7,036 (82.0) | 4,101 (76.0) |
AIS | 1,597 (18.0) | 426 (8.0) |
HI | 0 | 840 (16.0) |
Consistency test (manual 1–2) | ||
Kappa coefficient | 0.97 | 0.98 |
AIS, acute ischemic stroke; HI, hemorrhagic infarction.
Table 3
Test set | AIS data | HI data | |||||
---|---|---|---|---|---|---|---|
Basal ganglia (n=80) | Pons (n=80) | Centrum semiovale (n=80) | HI group (n=65) | Non-HI group (n=65) | P | ||
Age, years | 61 [23–71] | 66 [45–88] | 65 [39–73] | 58 [25–79] | 64 [23–70] | 0.28 | |
Male sex, n (%) | 42 (52.5) | 38 (47.5) | 47 (58.6) | 38 (58.5) | 36 (55.4) | 0.64 | |
Lesion volume | |||||||
AIS, mm3 | 58.3 [8–313] | 35.0 [6–150] | 31.4 [10–62] | 5,940.6 [215–28,701] | 4261.7 [638–27,365] | 0.08 | |
HI, mm3 | 0 | 0 | 0 | 925.4 [26–12,420] | 0 | ||
Consistency test | |||||||
ICC | 0.96 | 0.97 | 0.96 | 0.97 | 0.96 |
Data are given as median [min–max]. AIS, acute ischemic stroke; HI, hemorrhagic infarction; ICC, intraclass correlation coefficient.
Visual assessment of HI lesions in DWI by 2 experienced neuroradiologists
Concurrent HI lesions for each patient in the HI test set and non-HI group were visually assessed independently by two neuroradiologists (Dr. Liu and Dr. Zhang, with 15 and 25 years of experience in neuroradiology, respectively) who were blinded to the GRE. Any disagreements were resolved by consensus (18). Visual assessment and lesion annotation were performed in two different groups. As image analysis for DWI included both ADC and b0 images, the presence of HI lesions on b0 images was determined by investigators with knowledge of lesion presence on DWI. A HI lesion was judged as visible on b0 imaging when hypointensity was present in a region corresponding to the AIS lesion on DWI. The human and weakly supervised approach reading times were recorded.
Weakly supervised model architecture
In our experiments, the images were first normalized to zero mean and unit variance, and then concatenated in channel-wise fashion. We chose to use the visual geometry group network with 16 layers (VGG-16) (19) and the residual neural network with 50 layers (ResNet-50) (11,20) as the convolutional neural network (CNN) backbone. The backbone was used to extract the features (21)1. Conventionally, in a CNN that is designed for classification, the last convolution layer is usually followed by a fully connected layer to output the classification result (22). In this study, since the objective was to detect the lesion while the labels were the manual annotation denoting whether or not a slice contained a stroke lesion, we replaced the 4096-dimension fully connected layers in the original VGG-16 and ResNet-50 by a global average pooling layer followed by an output layer (i.e., a fully connected layer). The global average pooling layer output the mean value of each feature map, and the mean values were further processed by a fully connected layer which output the classification result. In the training stage, the CNN was trained as a classifier with binary cross entropy being used as the loss function. Notably, although only AIS subjects were included in our work, as each AIS subject includes slices both with and without AIS lesions, the CNN was trained to classify AIS slices and non-AIS slices as well as HI slices and non-HI slices. In the testing stage, as our objective was to localize the stroke lesions, we needed to convert the trained CNN classifier such that the network could output an attention map that showed where the lesions were located. We directly output the feature maps of the last convolutional layer and used the weighted sum as the localization result to generate a class activation map (CAM) (23), where the weights were copied from the last fully connected layer in the training stage. The CAMs were then normalized and used to localize the lesions. The CNN architecture is detailed in the Supplementary file.
The output of the CNN indicated the probability that a pixel should be labeled as lesion tissue, and a threshold δ was required to convert the probability score map to a binary segmentation. The threshold was selected by maximizing the F1 score on the validation set.
The experiments were performed on a computer with an Intel Core i7-7800K CPU, 64GB RAM and Nvidia GeForce 1080Ti GPU with 11GB memory. The computer operated on Ubuntu Linux 16.04 LTS with CUDA 9.0. The network was implemented on Keras 2.0.2 (www.keras.io). The MR image files were stored as Neuroimaging Informatics Technology Initiative format, and processed using Simple Insight ToolKit (SimpleITK, www.simpleitk.org) [1.2.0]. We used ITK-SNAP [3.8.0] (www.itksnap.org) (21) for image annotation and the visualization of results.
Statistical analysis
Statistical analyses were performed using SPSS (version 22.0, IBM Corporation, Armonk, NY, USA). Receiver operating characteristic (ROC) curves were constructed and the area under the curve (AUC) was calculated to compare the efficacy of each model. First, we proposed several lesion-wise metrics using three-dimensional (3D) connected component analysis to evaluate the performance of the networks. We measured the lesion-wise sensitivity and positive predictive value. The number of failure-to-detect subjects (FD-S) was used to evaluate the subject-level performance.
Next, we compared the performance of the weakly supervised approach with human visual assessment by analyzing their sensitivities, specificities, positive predictive values, negative predictive values, and AUCs in detecting concurrent HI lesions. Sensitivity and specificity were compared using McNemar tests, while positive and negative predictive values were compared using the Generalized Score Statistics method, as appropriate. The Z-test was used to compare the AUC. P values <0.05 were considered to indicate statistical significance. Additional statistical comparisons are described in the Supplementary file.
Results
Patients
Of the 1,027 enrolled patients, 657 were used to train and 370 patients were used to test the methods. There were no significant differences between the training and test sets in baseline characteristics of age or sex. Of the 1,027 patients, 657 belonged to the AIS database, including 417 in the training set and 240 in the test set, and 370 belonged to the HI database, including 240 in the training set and 130 in the test set. The patients’ characteristics are summarized in Tables 2,3.
Network performance in detecting AIS lesions
We evaluated the network slice-wise classification performance by evaluating the ROC curves, as shown in Figure S1. It is clear in Figure S1 that both classifiers possessed superior performance in identifying image slices with stroke lesions. In particular, the ResNet-50 achieved an AUC score of 0.966, which highlighted the considerable potential of this deep learning method to aid clinicians’ diagnoses. The confusion matrix for slice-wise classification performance is displayed in Figure 3. ResNet-50 showed significantly higher lesion-wise sensitivity and positive predictive value of the pons group than did VGG-16 (P<0.001), as shown in Table 4. All of the FD-S appeared in the pons group, and 14 subjects were missed by VGG-16 compared with 1 subject by ResNet-50. Although the lesion-wise positive predictive values of ResNet-50 tended to be superior to those of VGG-16 in the centrum semiovale group (P<0.001) and the basal ganglia group (P=0.03), the sensitivities were similar.
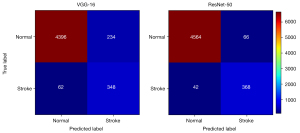
Table 4
Indicators | Acute ischemic stroke | Hemorrhagic infarction | |||||||||||||
---|---|---|---|---|---|---|---|---|---|---|---|---|---|---|---|
Basal ganglia | Pons | Centrum semiovale | |||||||||||||
VGG | ResNet | P | VGG | ResNet | P | VGG | ResNet | P | VGG | ResNet | P | ||||
Sensitivity | 0.954 | 0.965 | >0.99 | 0.795 | 0.964 | <0.001 | 1 | 1 | >0.99 | 0.725 | 0.847 | <0.001 | |||
PPV | 0.643 | 0.804 | 0.03 | 0.504 | 0.889 | <0.001 | 0.541 | 0.851 | <0.001 | 0.772 | 0.879 | 0.04 | |||
FD-S | 0 | 0 | – | 14 | 1 | – | 0 | 0 | – | 5 | 1 | – |
VGG-16, visual geometry group network-16; ResNet-50, residual neural network-50; PPV, positive predictive value; FD-S, the number of failure-to-detect subjects.
Identification of concurrent HI lesions in AIS patients
The lesion-level sensitivities and positive predictive values of VGG-16 were 0.834 and 0.912, respectively, for AIS detection, and 0.725 and 0.772, respectively, for HI detection. With ResNet-50, the lesion-level sensitivities and positive predictive values were 0.877 and 0.914, respectively, for AIS detection, and 0.847 and 0.879, respectively, for HI detection. As shown in Table 4, the lesion-level performance of ResNet-50 was better than that of VGG-16 (P<0.05). Figure 4 shows that the trained ResNet-50 could spatially locate the AIS and HI lesions. Based on the visual assessment of concurrent HI lesions from neuroradiologist (initial human interobserver agreement: 75.3% with a κ of 0.48), HI lesions were identified with a patient-level sensitivity of 0.862 and a specificity of 0.969. ResNet-50 exhibited a significantly higher sensitivity in detecting concurrent HI lesions compared to neuroradiologist visual assessment (Table 5). Although the specificities of the human results in identifying concurrent HI lesions were high, ResNet-50 demonstrated comparable specificity. Furthermore, ResNet-50 had a higher AUC in differentiating AIS patients with or without HI compared to visual assessment [95% confidence interval (CI): 0.003–0.105; Z=2.073; P=0.038].
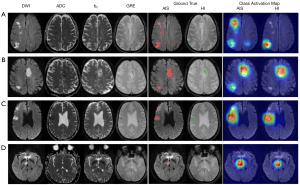
Table 5
Methods | Sensitivity | P | Specificity | P | PPV | P | NPV | P | AUC | P |
---|---|---|---|---|---|---|---|---|---|---|
Human | 0.846 | – | 0.969 | – | 0.945 | – | 0.863 | – | 0.908 | – |
ResNet-50 | 0.984 | 0.004 | 0.954 | >0.99 | 0.955 | 0.80 | 0.984 | 0.01 | 0.969 | 0.02 |
VGG-16 | 0.923 | 0.06 | 0.938 | 0.50 | 0.938 | 0.52 | 0.924 | 0.26 | 0.931 | 0.36 |
P value denotes the P value derived from comparisons with human results. DWI, diffusion-weighted imaging; ResNet-50, residual neural network-50; VGG-16, visual geometry group network-16; PPV, positive predictive value; NPV, negative predictive value; AUC, area under the curve.
Costs and benefits of the weakly supervised learning
In the training stage, VGG-16 and ResNet-50 took 2.2 hours and 2.5 hours, respectively. In the testing stage, VGG-16 and ResNet-50 took 0.46 and 0.87 s, respectively. The weakly supervised approach significantly reduced the labeling time compared to the fully supervised approach (10.115 vs. 2.663 min; P<0.001), as shown in Figure 5. Also, ResNet-50 involved less reading time than did human visual assessment in identifying concurrent HI lesions in AIS patients (39.921 vs. 0.873 s; P<0.001).
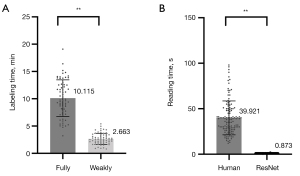
Discussion
The contributions of this paper are summarized as follows. First, ResNet-50 improved the sensitivity of detecting small AIS lesions in different positions, especially for pontine lesions. Next, a weakly supervised method was proposed, which could sensitively identify concurrent HI lesions in AIS patients using DWI and effectively reduce the time of lesion labeling and film reading. Our algorithm is capable of rapidly and accurately identifying AIS and concurrent HI lesions, which can minimize the occurrence of misdiagnosis, avoid inappropriate treatment, and improve the quality of medical care.
We trained and validated two neural networks to detect small stroke lesions in different regions. ResNet-50 showed superior sensitivities compared to VGG-16, which highlighted the importance of using a residual structure. The residual structure can make the network much deeper and avoid the vanishing gradient problem (24). Notably, an overall several times increase of FD-S in the pons group detected by VGG-16 (versus ResNet-50) should be emphasized in terms of patient safety because false negative cases may have an increased risk of inappropriate treatment. There are several possible explanations for why all of the failed detections for AIS regions were in the pons. It may be due to a small sample size of pons infractions in the data set. In the training set, 103 cases (24.7%) had posterior circulation cerebral infarction, of which 72 cases (17.2%) had pontine infarction. A total of 128 AIS image sets from 72 patients with pontine infarction were included for network training, slightly lower than the number of some other artificial intelligence studies (25,26). Furthermore, an epidemiological study showed that approximately 20–25% of ischemic strokes affect posterior circulation brain structures. The number of pontine infarctions in the training set was consistent with epidemiological characteristics. Second, magnetically susceptible artifacts may be the main cause of this issues; these artifacts originate from the inhomogeneous magnetic field and the magnetic susceptibility differences between brain tissue and the air-containing areas of the skull (Figure S2) (27).
In addition, the positive predictive value of the centrum semiovale group was lower than that of the basal ganglia group in VGG-16. This result may be due to T2 shine-through that is caused by the demyelination of white matter. ResNet-50 has solved the above problems remarkably well. Although the CAM did not use the correct regions of images in some case, we would like to mention that this problem usually occurs in the ImageNet data set with millions of images of 1,000 classes. Our study involved thousands of image slices with significantly fewer classes; that is, 2 classes for the AIS task and 3 classes for the HI task. The images were all brain MR images, which were not as diverse as those in the ImageNet data set. In addition, a total of 1,027 patients were included in this study for analysis; compared to previous artificial intelligence studies related to stroke (Table S1), the sample size of this study was sufficient.
This study was designed to examine the value of a weakly supervised method to automatically identify the HI lesions in AIS patients. HI may occur as part of the spontaneous evolution of AIS, or is precipitated by the use of thrombolytic therapy. A spontaneous HI can occur prior to any treatment and may seriously influence patient prognosis if it is not promptly detected (28). In addition, therapy-related HI, or post-therapy HI, is a medical emergency, with deterioration typically occurring quickly after onset. As shown in Figure 4, it was difficult for us to determine the existence of HI lesions in DWI by human visual assessment. Subject C had an old hemorrhagic lesion in the contralateral hemisphere of the AIS lesion, which was not misdiagnosed as a HI. Although the AIS and HI lesion volume of subject D were only 327 and 302 mm3, respectively (Figure S3), ResNet-50 accurately located the lesion and was not affected by the magnetic susceptibility artifact. Moreover, weakly supervised learning has the potential to reduce the labeling workload. Full annotation of lesion outlines takes up to approximately 10 minutes per subject, while weak labeling can be completed in approximately 2 minutes (29). This indicates that the algorithm can be perfectly trained on more patients, which makes it possible to provide more consistency in larger data sets.
There are several limitations in the present study that should be noted. First, the lesion segmentation accuracy needs to be further improved. In this paper, due to the data training of weakly supervised learning, the information that was provided by weakly supervised labels was significantly less than that provided by fully supervised labels. Although this method can accurately mark the locations of lesions, it is less able to provide detailed parameters, such as the size of a lesion. Second, although some examples demonstrated that this method can distinguish HI lesions from hemosiderin deposits, this has not been systematically confirmed and requires further verification. This method still depends on the clinical history and changes in the patients’ symptoms. Third, the lesion-wise specificity was not included in our analysis due to the fact that specificity is a true-negative–related metric, and it was difficult to define a true-negative lesion in our task. Fourth, in order to further evaluate the detection accuracy of the proposed method, multicenter studies that standardize the imaging protocols and the post-processing procedures are warranted.
Conclusions
ResNet-50 offers superior performance to VGG-16 for identifying small AIS lesions in different positions. Our study provides a weakly supervised approach based on ResNet-50 to identify sensitively concurrent HI lesions in AIS patients. The proposed approach helps to reduce the difficulty in obtaining expert labels and has the potential to minimize the occurrence of misdiagnosis when GRE is not routinely performed.
Acknowledgments
We would like to thank the study team and all the study participants, and Dr. Zhang and Dr. Liu, especially, for their assessment of the disease.
Funding: This work was supported by the Natural Science Foundation of China (grant number 81771806, 81871342, 61871235), the National Key Research and Development Project (grant number 2019YFC0120903), the Natural Science Foundation of Tianjin (grant number 20JCQNJC01250), and the Tianjin Key Laboratory of Optoelectronic Sensor and Sensing Network Technology.
Footnote
Conflicts of Interest: All authors have completed the ICMJE uniform disclosure form (available at https://dx.doi.org/10.21037/qims-21-324). The authors have no conflicts of interest to declare.
Ethical Statement: The authors are accountable for all aspects of the work in ensuring that questions related to the accuracy or integrity of any part of the work are appropriately investigated and resolved. This study was conducted in accordance with the Declaration of Helsinki (as revised in 2013) and was approved by the Ethics Board of Tianjin Huanhu Hospital. Individual consent for this retrospective analysis was waived.
Open Access Statement: This is an Open Access article distributed in accordance with the Creative Commons Attribution-NonCommercial-NoDerivs 4.0 International License (CC BY-NC-ND 4.0), which permits the non-commercial replication and distribution of the article with the strict proviso that no changes or edits are made and the original work is properly cited (including links to both the formal publication through the relevant DOI and the license). See: https://creativecommons.org/licenses/by-nc-nd/4.0/.
1As the VGG-16 and ResNet-50 were applied in their original form, please refer to (Simonyan K, Zisserman A. Very Deep Convolutional Networks for Large-Scale Image Recognition. arXiv 14091556. 2014) and (He K, Zhang X, Ren S, Sun J, editors. Deep Residual Learning for Image Recognition. IEEE Conference on Computer Vision & Pattern Recognition; 2016) for detailed network architectures.
References
- Wang W, Jiang B, Sun H, Ru X, Sun D, Wang L, Wang L, Jiang Y, Li Y, Wang Y, Chen Z, Wu S, Zhang Y, Wang D, Wang Y, Feigin VL. NESS-China Investigators. Prevalence, Incidence, and Mortality of Stroke in China: Results from a Nationwide Population-Based Survey of 480 687 Adults. Circulation 2017;135:759-71. [Crossref] [PubMed]
- Powers WJ, Rabinstein AA, Ackerson T, Adeoye OM, Bambakidis NC, Becker K, Biller J, Brown M, Demaerschalk BM, Hoh B, Jauch EC, Kidwell CS, Leslie-Mazwi TM, Ovbiagele B, Scott PA, Sheth KN, Southerland AM, Summers DV, Tirschwell DL. Guidelines for the Early Management of Patients With Acute Ischemic Stroke: 2019 Update to the 2018 Guidelines for the Early Management of Acute Ischemic Stroke: A Guideline for Healthcare Professionals From the American Heart Association/American Stroke Association. Stroke 2019;50:e344-418. [Crossref] [PubMed]
- Stone JA, Willey JZ, Keyrouz S, Butera J, McTaggart RA, Cutting S, Silver B, Thompson B, Furie KL, Yaghi S. Therapies for Hemorrhagic Transformation in Acute Ischemic Stroke. Curr Treat Options Neurol 2017;19:1. [Crossref] [PubMed]
- Jiang L, Peng M, Chen H, Geng W, Zhao B, Yin X, Chen YC, Su H. Diffusion-weighted imaging (DWI) ischemic volume is related to FLAIR hyperintensity-DWI mismatch and functional outcome after endovascular therapy. Quant Imaging Med Surg 2020;10:356-67. [Crossref] [PubMed]
- Kidwell CS, Chalela JA, Saver JL, Starkman S, Hill MD, Demchuk AM, et al. Comparison of MRI and CT for detection of acute intracerebral hemorrhage. JAMA 2004;292:1823-30. [Crossref] [PubMed]
- Arnould MC, Grandin CB, Peeters A, Cosnard G, Duprez TP. Comparison of CT and three MR sequences for detecting and categorizing early (48 hours) hemorrhagic transformation in hyperacute ischemic stroke. AJNR Am J Neuroradiol 2004;25:939-44. [PubMed]
- Lu CY, Chiang IC, Lin WC, Kuo YT, Liu GC. Detection of intracranial hemorrhage: comparison between gradient-echo images and b0 images obtained from diffusion-weighted echo-planar sequences on 3.0T MRI. Clin Imaging 2005;29:155-61. [Crossref] [PubMed]
- Zhu F, Labreuche J, Haussen DC, Piotin M, Steglich-Arnholm H, Taschner C, Papanagiotou P, Lapergue B, Dorn F, Cognard C, Killer M, Psychogios MN, Spiotta A, Mazighi M, Bracard S, Turjman F, Richard S, Gory B. TITAN (Thrombectomy in Tandem Lesions) Investigators. Hemorrhagic Transformation After Thrombectomy for Tandem Occlusions. Stroke 2019;50:516-9. [Crossref] [PubMed]
- Chen L, Bentley P, Rueckert D. Fully automatic acute ischemic lesion segmentation in DWI using convolutional neural networks. Neuroimage Clin 2017;15:633-43. [Crossref] [PubMed]
- Zhang R, Zhao L, Lou W, Abrigo JM, Mok VCT, Chu WCW, Wang D, Shi L. Automatic Segmentation of Acute Ischemic Stroke From DWI Using 3-D Fully Convolutional DenseNets. IEEE Trans Med Imaging 2018;37:2149-60. [Crossref] [PubMed]
- Liu Z, Cao C, Ding S, Liu Z, Han T, Liu S. Towards Clinical Diagnosis: Automated Stroke Lesion Segmentation on Multi-Spectral MR Image Using Convolutional Neural Network. IEEE Access 2018;6:57006-16.
- Meng Q, Sinclair M, Zimmer V, Hou B, Rajchl M, Toussaint N, Oktay O, Schlemper J, Gomez A, Housden J, Matthew J, Rueckert D, Schnabel JA, Kainz B. Weakly Supervised Estimation of Shadow Confidence Maps in Fetal Ultrasound Imaging. IEEE Trans Med Imaging 2019;38:2755-67. [Crossref] [PubMed]
- Zhao B, Ding S, Wu H, Liu G, Cao C, Jin S, Liu Z. Automatic acute ischemic stroke lesion segmentation using semi-supervised learning. Int J Comput Intell 2021;14:723-33. [Crossref]
- Zhao B, Liu Z, Liu G, Cao C, Jin S, Wu H, Ding S. Deep Learning-Based Acute Ischemic Stroke Lesion Segmentation Method on Multimodal MR Images Using a Few Fully Labeled Subjects. Comput Math Methods Med 2021;2021:3628179 [Crossref] [PubMed]
- Liu M, Zhang J, Lian C, Shen D. Weakly Supervised Deep Learning for Brain Disease Prognosis Using MRI and Incomplete Clinical Scores. IEEE Trans Cybern 2020;50:3381-92. [PubMed]
- Zhou J, Luo LY, Dou Q, Chen H, Chen C, Li GJ, Jiang ZF, Heng PA. Weakly supervised 3D deep learning for breast cancer classification and localization of the lesions in MR images. J Magn Reson Imaging 2019;50:1144-51. [Crossref] [PubMed]
- Jiang S, Wu S, Zhang S, Wu B. Advances in Understanding the Pathogenesis of Lacunar Stroke: From Pathology and Pathophysiology to Neuroimaging. Cerebrovasc Dis 2021;50:588-96. [PubMed]
- Lee H, Lee EJ, Ham S, Lee HB, Lee JS, Kwon SU, Kim JS, Kim N, Kang DW. Machine Learning Approach to Identify Stroke Within 4.5 Hours. Stroke 2020;51:860-6. [Crossref] [PubMed]
-
.Simonyan K Zisserman A Very deep convolutional networks for large-scale image recognition. arXiv 14091556.2014 . - He K, Zhang X, Ren S, Sun J, editors. Deep Residual Learning for Image Recognition. IEEE Conference on Computer Vision & Pattern Recognition 2016.
- Duong MT, Rudie JD, Wang J, Xie L, Mohan S, Gee JC, Rauschecker AM. Convolutional Neural Network for Automated FLAIR Lesion Segmentation on Clinical Brain MR Imaging. AJNR Am J Neuroradiol 2019;40:1282-90. [Crossref] [PubMed]
- Zhou T, Tan T, Pan X, Tang H, Li J. Fully automatic deep learning trained on limited data for carotid artery segmentation from large image volumes. Quant Imaging Med Surg 2021;11:67-83. [Crossref] [PubMed]
- Zhou B, Khosla A, Lapedriza A, Oliva A, Torralba A, editors. Learning Deep Features for Discriminative Localization. 2016 IEEE Conference on Computer Vision and Pattern Recognition (CVPR) 2016.
- Wang EK, Zhang X, Pan L, Cheng C, Dimitrakopoulou-Strauss A, Li Y, Zhe N. Multi-Path Dilated Residual Network for Nuclei Segmentation and Detection. Cells 2019;8:499. [Crossref] [PubMed]
- Wang K, Shou Q, Ma SJ, Liebeskind D, Qiao XJ, Saver J, Salamon N, Kim H, Yu Y, Xie Y, Zaharchuk G, Scalzo F, Wang DJJ. Deep Learning Detection of Penumbral Tissue on Arterial Spin Labeling in Stroke. Stroke 2020;51:489-97. [Crossref] [PubMed]
- Qiu W, Kuang H, Teleg E, Ospel JM, Sohn SI, Almekhlafi M, Goyal M, Hill MD, Demchuk AM, Menon BK. Machine Learning for Detecting Early Infarction in Acute Stroke with Non-Contrast-enhanced CT. Radiology 2020;294:638-44. [Crossref] [PubMed]
- Lu J, Wang X, Qing Z, Li Z, Zhang W, Liu Y, Yuan L, Cheng L, Li M, Zhu B, Zhang X, Yang QX, Zhang B. Detectability and reproducibility of the olfactory fMRI signal under the influence of magnetic susceptibility artifacts in the primary olfactory cortex. Neuroimage 2018;178:613-21. [Crossref] [PubMed]
- D'Amelio M, Terruso V, Famoso G, Di Benedetto N, Realmuto S, Valentino F, Ragonese P, Savettieri G, Aridon P. Early and late mortality of spontaneous hemorrhagic transformation of ischemic stroke. J Stroke Cerebrovasc Dis 2014;23:649-54. [Crossref] [PubMed]
- Ng D, Du H, Yao MM, Kosik RO, Chan WP, Feng M. Today's radiologists meet tomorrow's AI: the promises, pitfalls, and unbridled potential. Quant Imaging Med Surg 2021;11:2775-9. [Crossref] [PubMed]