Diffusion-weighted imaging of breast invasive lobular carcinoma: comparison with invasive carcinoma of no special type using a histogram analysis
Introduction
Diffusion-weighted imaging (DWI) has been widely integrated into clinical practice for breast imaging. DWI can help distinguish between benign and malignant lesions (1) and could reduce false positives and unnecessary biopsies (2,3). Rahbar et al. reported an apparent diffusion coefficient (ADC) threshold of 1.53×10–3 mm2/s application could reduce the unnecessary biopsy rate by 20.9% without reducing sensitivity (3). DWI can also be used to detect the early response to neoadjuvant chemotherapy (NAC) and in evaluating residual cancer after NAC (4-6).
Recently, there have been increasing concerns over the MRI contrast agents’ side effects, such as nephrogenic systemic fibrosis or deposition of contrast agents in the brain or other tissues. The major strength of DWI is that it can provide quantitative information about the motion of water molecules and biological characteristics of tumors without a contrast agent injection. Therefore, DWI has the potential to be used as an unenhanced magnetic resonance imaging (MRI) for breast cancer screening.
Many studies have demonstrated DWI as an imaging tool for improving breast cancer diagnosis and characterization (7-12). The sensitivity of DWI ranged from 45% to 94% and specificity from 79% to 95% showing lower sensitivity and similar or higher specificity compared to dynamic contrast-enhanced MRI. However, ADC could not reflect a true diffusion restriction in breast tissues with low water content such as fibrosis, scars and some invasive lobular carcinomas (ILCs) and these lesions will demonstrate low signal on DWI (13). Furthermore, low cellularity cancers such as mucinous carcinoma, triple-negative cancer with extensive necrosis and ductal carcinoma in situ (DCIS) could be the causes of false negatives and could be missed by DWI MRI (11,14,15).
ILC accounts for about 10% of cases of invasive breast cancer and second most common subtype of breast cancer. Due to its infiltrative growth pattern, mammography and ultrasonography (US) showed lower sensitivity (16). Although previous study reported that ILC can cause a false-negative diagnosis on DWI (17), there have been few studies on DWI findings of ILC. Therefore, the aims of this study were to investigate the imaging findings and visibility of ILC on DWI and to compare quantitative ADC metrics of ILC and invasive carcinoma of no special type (NST) using histogram analysis.
Methods
The study was conducted in accordance with the Declaration of Helsinki (as revised in 2013). Our institutional review board approved this retrospective study (AJIRB-MED-MDB-21-092) and individual consent for this retrospective analysis was waived.
Study population
We retrospectively searched our hospital medical data to identify patients who met the following inclusion criteria: (I) pathologically proven invasive carcinoma of NST and ILC without intraductal component; and (II) preoperative breast MRI examinations, performed on a 3-T scanner and including DWI, completed in our hospital. Between January 2017 and August 2020, 629 women met the inclusion criteria. Among them, we excluded 23 women who had undergone excisional biopsy, US-guided vacuum-assisted biopsy, or stereotactic vacuum-assisted biopsy before MRI, 6 women who had ILC presented as non-mass enhancement on MRI, 54 women whose histologic or nuclear grade data were not available, and 4 women who had inadequate DWI quality and were excluded. Finally, 542 women (71 patients with ILC and 471 patients with invasive carcinoma of NST) were included in this analysis (Figure 1). Using propensity score matching (PSM), women with ILC were matched with the women with invasive carcinoma of NST according to 7 covariates: age; tumor size; histologic grade; nuclear grade; ER expression; PR expression; and HER2 expression.
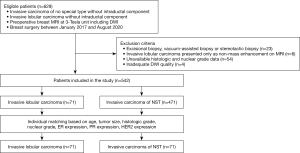
MRI technique
All breast MRI was performed using a 3-T MR scanner (Discovery MR750, GE Healthcare, Milwaukee, WI, USA) with a dedicated 8-channel phased-array breast coil (GE Healthcare). Patients were scanned in the prone position. Breast MRI protocol included axial fat-suppressed T2-weighted imaging (repetition time/echo time =3,800 ms/85 ms, flip angle =124°, matrix =320×256, field of view =300 mm × 300 mm, and slice thickness =3 mm) and axial DWI using a single-shot echo-planar imaging technique with fat suppression (repetition time/echo time =8,500 ms/75 ms, slice thickness =3.0 mm, matrix =256×152, FOV =204 mm ×340 mm, number of excitation =2, no gap). Diffusion-sensitizing gradients were applied sequentially along the three orthogonal directions and images were obtained at b values of 0 and 800 s/mm2.
For dynamic contrast-enhanced imaging, fat-suppressed axial T1-weighted volumetric scanning was performed (TR =7 ms, TE =2 ms, flip angle =10°, matrix =300×300, FOV =320 mm ×320 mm, slice thickness =2 mm, and phase acquisition time =90 s). For contrast-enhanced examinations, 0.1 mmol/L gadolinium chelate/kg body weight (Gadovist, Bayer Schering Pharma, Berlin, Germany) was power injected (Spectris; Medrad, Pittsburgh, Penn) at a flow rate of 1 mL/sec followed by a 20-mL saline flush.
Image analysis
Two radiologists with 11 and 3 years of experience in breast MRI retrospectively reviewed the images. They were informed only of the diagnosis of breast cancer but were blinded to the clinical-pathologic data. The reviewers identified breast cancers on dynamic contrast-enhanced image and corresponding DWI. Tumor visibility was assessed using a four-point scale (1: no visibility, 2: slight visibility, 3: moderate visibility, 4: excellent visibility). Background parenchymal enhancement (BPE) and diffusion background signals were visually assessed and classified according to a 4-point scale: 1= minimum, 2= mild, 3= moderate, and 4= marked (18).
For histogram analysis of ADC value, we used a semi-automatic software designed by MATLAB (MathWorks, Inc., Natick, MA, USA) (Figure 2). An ADC map of the widest diameter of each lesion was selected and we manually drew a large region of interest (ROI) to encompass the entire cross-section of the tumor avoiding normal parenchymal tissue, areas of necrosis, hemorrhage or fat components. The software automatically calculated mean, median, maximum, minimum, 90th percentile, 10th percentile, ADC difference values, skewness and kurtosis. The ADC difference value was the difference between minimum and maximum ADC values. Skewness is an indicator of the asymmetry in ADC value distribution around the mean. Kurtosis is a measure that defines how heavily the tails of a distribution differ from the tails of a normal distribution.
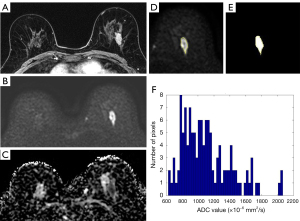
In addition, to measure the ADC value with a conventional ROI method, a ROI was manually drawn on the darkest part of the tumor on the ADC map representing the most suspicious area. The mean size of the ROIs was 96 mm2 (range, 13–217 mm2). Two observers independently measured the ADCs. The ADC measurements were averaged and the mean ADCs were used for statistical analysis.
Statistical analysis
To compare the ILC and invasive carcinoma of NST groups, an individual matching method was used. Individuals were matched in the following covariates: age, tumor size, histologic grade (I or II; III), nuclear grade (I: II or III), ER expression (negative or positive), PR expression (negative or positive), and HER2 expression (negative or positive).
For the analysis of correlation between cancer visibility and tumor size, background signal intensity and tumor ADC value, we used Spearman rank correlation test. After the evaluation of data normality using Shapiro-Wilk test, we used independent t test or Mann-Whitney U test for comparisons of histogram metrics (mean, median, maximum, minimum, 90th percentile, 10th percentile, ADC difference, skewness and kurtosis values) and ROI-based ADC values. The interobserver variability of ROI-based ADC measurement was evaluated using intraclass correlation coefficient (ICC) method.
All statistical analyses were performed using SPSS version 21 (IBM Corp., Armonk, NY, USA). A P value <0.05 was considered to indicate statistical significance.
Results
Clinical and histopathologic characteristics of patients
Among the 542 patients, 471 (86.9%) were invasive carcinoma of NST and 71 (13.1%) were ILC (Table 1). Before PSM, low nuclear grade and low histologic grade were more frequently observed in ILC than invasive carcinoma of NST (P<0.001 and P=0.004, respectively). ER positivity and HER2 negativity were also more frequently observed in ILC (P=0.001 and P=0.003, respectively).
Table 1
Variable | Entire population | Propensity-matched population (1:1) | |||||||
---|---|---|---|---|---|---|---|---|---|
ILC (N=71) | Invasive carcinoma NST (N=471) | P value | Standardized difference | ILC (N=71) | Invasive carcinoma NST (N=71) | P value | Standardized difference | ||
Size | 0.212 | 0.17 | 0.919 | 0.02 | |||||
Mean (SD) | 2.36 (1.75) | 2.09 (1.33) | 2.36 (1.75) | 2.33 (1.7) | |||||
Median (Q1, Q3) | 2 (1.2, 2.7) | 1.8 (1.2, 2.5) | 2 (1.2, 2.7) | 1.9 (1.35, 2.6) | |||||
Age | 0.435 | 0.1 | 0.449 | 0.13 | |||||
Mean (SD) | 51.66 (8.91) | 50.73 (9.39) | 51.66 (8.91) | 50.45 (10.07) | |||||
Median (Q1, Q3) | 50 (45, 57) | 50 (44, 57) | 50 (45, 57) | 50 (43.5, 57) | |||||
NG group, n (%) | <0.001 | −0.61 | >0.999 | −0.04 | |||||
Low | 59 (83.1) | 266 (56.48) | 59 (83.1) | 58 (81.69) | |||||
High | 12 (16.9) | 205 (43.52) | 12 (16.9) | 13 (18.31) | |||||
HG group, n (%) | 0.004 | −0.42 | 0.823 | −0.08 | |||||
Low | 60 (84.51) | 314 (66.67) | 60 (84.51) | 58 (81.69) | |||||
High | 11 (15.49) | 157 (33.33) | 11 (15.49) | 13 (18.31) | |||||
ER, n (%) | 0.001 | 0.53 | >0.999 | 0 | |||||
Negative | 2 (2.82) | 89 (18.9) | 2 (2.82) | 2 (2.82) | |||||
Positive | 69 (97.18) | 382 (81.1) | 69 (97.18) | 69 (97.18) | |||||
PR, n (%) | 0.066 | 0.28 | >0.999 | 0 | |||||
Negative | 8 (11.27) | 101 (21.44) | 8 (11.27) | 8 (11.27) | |||||
Positive | 63 (88.73) | 370 (78.56) | 63 (88.73) | 63 (88.73) | |||||
HER2, n (%) | 0.003 | −0.48 | >0.999 | 0 | |||||
Negative | 68 (95.77) | 380 (80.68) | 68 (95.77) | 68 (95.77) | |||||
Positive | 3 (4.23) | 91 (19.32) | 3 (4.23) | 3 (4.23) |
ILC, invasive lobular carcinoma; NST, no special type; NG, nuclear grade; HG, histologic grade; SD, standard deviation; ER, estrogen receptor; PR, progesterone receptor; HER2, human epidermal growth factor receptor 2.
After PSM with age, tumor size, histologic grade, nuclear grade, ER expression, PR expression and HER2 expression, 71 women were matched in each group of ILC and invasive carcinoma of NST groups. The tumor size was 2.36±1.75 cm (median, 2 cm: range, 0.8–11.7 cm) in ILC and 2.09±1.33 cm (median, 1.8 cm: range, 0.5–12 cm) in invasive carcinoma of NST.
Assessment of cancer visibility and background signal on contrast-enhanced imaging and DWI
Table 2 summarizes the cancer visibility on mammography, ultrasonography and MRI. Sixteen (22.5%) of 71 ILC were not visualized on mammography, whereas 6 (8.5%) of 71 invasive carcinoma of NST were not detected. All ILC and invasive carcinoma of NST were visualized on ultrasonography.
Table 2
Imaging findings | Invasive lobular carcinoma, n (%) | Invasive carcinoma of NST, n (%) |
---|---|---|
Visibility on mammography | ||
Visible | 55 (77.5) | 65 (91.5) |
Not visible | 16 (22.5) | 6 (8.5) |
Calcification on mammography | ||
Present | 11 (15.5) | 1 (1.4) |
Absent | 60 (84.5) | 70 (98.6) |
Visibility on ultrasonography | ||
Visible | 71 (100.0) | 71 (100.0) |
Not visible | 0 (0.0) | 0 (0.0) |
Visibility score on contrast-enhanced MRI | ||
1 | 0 (0.0) | 0 (0.0) |
2 | 2 (2.8) | 1 (1.4) |
3 | 9 (12.7) | 5 (7.0) |
4 | 60 (84.5) | 65 (91.6) |
Visibility score on DWI | ||
1 | 9 (12.7) | 4 (5.6) |
2 | 9 (12.7) | 3 (4.2) |
3 | 19 (26.7) | 13 (18.3) |
4 | 34 (47.9) | 51 (71.9) |
Qualitative BPE grade | ||
1 | 31 (43.6) | 30 (42.3) |
2 | 11 (15.5) | 13 (18.3) |
3 | 20 (28.2) | 22 (31.0) |
4 | 9 (12.7) | 6 (8.4) |
Qualitative background diffusion grade | ||
1 | 7 (9.8) | 7 (9.8) |
2 | 25 (35.2) | 21 (29.6) |
3 | 31 (43.7) | 31 (43.7) |
4 | 8 (11.3) | 12 (16.9) |
Background ADC value |
1.791±0.322 | 1.778±0.326 |
a, data are means ± standard deviations. MRI, magnetic resonance imaging; DWI, diffusion-weighted imaging; BPE, background parenchymal enhancement.
On contrast-enhanced images, 60 (84.5%) lesions of ILC and 65 (91.6%) of invasive carcinoma of NST were scored as 4 and there was no lesion scored as 1. On diffusion-weighted images, 9 (12.7%) lesions of ILC were scored as 1 and 4 (5.6%) invasive carcinoma of NST were scored as 1. 34 (47.9%) lesions of ILC were scored as 4 and 51 (71.9%) of invasive carcinoma of NST were scored as 4.
Associations of cancer visibility with tumor size and background signal intensity
Table 3 shows the associations of cancer visibility with tumor size, tumor ADC value, BPE grade, background diffusion grade, and mean background ADC values. For the visibility of ILC on contrast-enhanced imaging, the visibility score was significantly associated with tumor size (rho =0.245, P=0.039) and BPE grade (rho =−0.362, P=0.002). However, in case of invasive carcinoma of NST, only BPE grade was significantly associated with cancer visibility (rho =−0.402, P<0.001), and tumor size was not (rho =0.205, P=0.086).
Table 3
Factors affecting cancer visibility | Invasive lobular carcinoma | Invasive carcinoma of NST |
---|---|---|
Visibility on contrast-enhanced imaging | ||
Tumor size | rho =0.245 (P=0.039) | rho =0.205 (P=0.086) |
BPE grade | rho =−0.362 (P=0.002) | rho =−0.402 (P<0.001) |
Visibility on diffusion-weighted imaging | ||
Tumor size | rho =0.354 (P=0.002) | rho =0.322 (P=0.006) |
Tumor mean ADC value | rho =−0.322 (P=0.006) | rho =−0.401 (P=0.023) |
Qualitative background diffusion grade | rho =−0.301 (P=0.011) | rho =−0.321 (P=0.006) |
Background ADC value | rho =−0.166 (P=0.166) | rho =−0.074 (P=0.542) |
ADC, apparent diffusion coefficient; BPE, background parenchymal enhancement.
For the visibility of ILC on DWI, tumor size (rho =0.354, P=0.002), tumor ADC value (rho =−0.322, P=0.006), and background diffusion grade (rho =−0.301, P=0.011) were significantly associated with the visibility score, whereas the mean background ADC value was not (rho =−0.166, P=0.166). For invasive carcinoma of NST, tumor size (rho =0.322, P=0.006), tumor ADC value (rho =−0.401, P=0.023), and background diffusion grade (rho =−0.321, P=0.006) were significantly associated with the visibility score, whereas the mean background ADC value was not (rho =−0.074, P=0.542).
Comparison of ADC metrics between ILC and invasive carcinoma of NST
Table 4 shows the comparison of quantitative ADC metrics between ILC and invasive carcinoma of NST using histogram analysis. Mean ADC (1.226×10−3 vs. 1.052×10−3 mm2/s, P<0.001), median ADC (1.222×10−3 vs. 1.051×10−3 mm2/s, P=0.002), minimum ADC (0.717×10−3 vs. 0.649×10−3 mm2/s, P=0.003), maximum ADC (1.758×10−3 vs. 1.504×10−3 mm2/s, P<0.001), 10th percentile ADC (0.956×10−3 vs. 0.818×10−3 mm2/s, P=0.024) and 90th percentile ADC (1.506×10−3 vs. 1.292×10−3 mm2/s, P<0.001) were higher in ILC compared to invasive carcinoma of NST. Also ADC difference value of the ILC was higher than that of invasive carcinoma of NST (1.04×10−3 vs. 0.855×10−3 mm2/s, P=0.026). Skewness and Kurtosis did not show any differences between two groups (Table 4).
Table 4
Variable | Invasive lobular carcinoma (n=71) | Invasive carcinoma of NST (n=71) | P value |
---|---|---|---|
Histogram analysis | |||
Mean ADC (×10−3 mm2/s) | 1.226±0.335 | 1.052±0.272 | <0.001 |
Median ADC (×10−3 mm2/s) | 1.222±0.348 | 1.051±0.286 | 0.002 |
Minimum ADC (×10−3 mm2/s) | 0.717±0.43 | 0.649±0.302 | 0.003 |
Maximum ADC (×10−3 mm2/s) | 1.758±0.428 | 1.504±0.387 | <0.001 |
10th percentile ADC (×10−3 mm2/s) | 0.956±0.341 | 0.818±0.262 | 0.024 |
90th percentile ADC (×10−3 mm2/s) | 1.506±0.381 | 1.292±0.321 | <0.001 |
ADC difference (×10−3 mm2/s) | 1.04±0.53 | 0.855±0.457 | 0.026 |
ADC Skewness | 0.093±0.736 | 0.193±0.718 | 0.397 |
ADC Kurtosis | 3.415±1.485 | 3.269±1.341 | 0.418 |
ROI-based measurement | |||
ADCmean (×10−3 mm2/s) | 1.026±0.305 | 0.926±0.247 | 0.034 |
ADC difference is the difference between maximum and minimum ADCs. ADC, apparent diffusion coefficient; NST, no special type.
Using the conventional ROI-based measurement, mean ADC was higher in ILC than that of invasive carcinoma NST (1.026×10−3 vs. 0.926×10−3 mm2/s, P=0.034). The ICC for interobserver variability was 0.913 (95% confidence interval, 0.891–0.929). A representative case is presented in Figure 3.
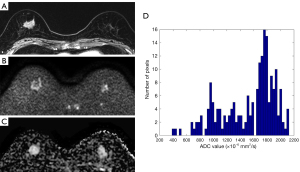
Discussion
Our results showed that the visibility of ILC was lower than invasive carcinoma of NST on DWI and the visibility was affected by tumor size, tumor ADC value and qualitative background diffusion grade. ILC showed higher quantitative ADC and higher ADC difference values compared to invasive carcinoma of NST.
Several studies reported the associations of ADC value and pathologic biomarkers of breast cancer. ADC values extracted by the histogram were lower in cancers with high Ki-67 proliferation index compared to low Ki-67 group (19). ADC value was lower in ER-positive breast cancer compared to ER-negative cancer, whereas the ADC value was higher in breast cancer with positive human epidermal growth factor receptor type 2 (HER2) (15,20,21). Therefore, for the exact comparison between ILC and invasive carcinoma of NST, we used propensity score matching for tumor size and other pathologic biomarkers. Better pathologic prognostic factors such as low nuclear and histologic grade, ER positivity and HER2 negativity were more frequently observed in ILC before PSM.
Several recent studies reported that ADC value of DCIS was higher than invasive cancers at 3T MRI (22,23). Using b values of 50 and 850 s/mm2, mean ADC of DCIS (1.24×10 mm2/s) was higher than invasive cancer (0.9×10 mm2/s) (22). Because ADC value could be measured variously depending on the quantity of DCIS component, we included invasive cancers without intraductal component in both ILC and invasive cancer of NST groups.
There have been several blind studies reporting the sensitivity of DWI. In the study of Trimboli et al. (9), the sensitivity of DWI with T1-weighted imaging (T1WI) and T2-weighted imaging (T2WI) was 76–78%. In the study by Kang et al., the readers detected the sensitivity of DWI fused with T1-weighted imaging (T1WI) to be 89% and 100% (12). Kazama et al. reported that the sensitivity was 74% for DWI alone and 93% for DWI with mammography in patients with T1 breast cancer (8), and McDonald et al. reported the sensitivity of DWI was 45% (11).
When using DWI for breast cancer screening without contrast-enhanced imaging, cancer visibility is the most important issue. Our results showed that ILC was less visualized on DWI compared to invasive carcinoma of NST. 18 (25.4%) of 71 ILC were not well visualized on DWI and acquired score 1 or score 2, whereas only 9 (9.8%) invasive carcinoma of NST were score 1 or 2.
Previous studies demonstrated that the tumor size, diffusion background signal and histologic grade could affect the cancer visibility on DWI (17,18). Similar to previous results, our study also showed that the cancer visibility was affected by tumor size, tumor ADC value and qualitative background diffusion signal and these findings were observed in both ILC and invasive carcinoma of NST. However, ADC values measured from the parenchyma was not associated with cancer visibility.
Various ADC measurement methods and ADC parameters have been proposed (24-27). Hirano et al. placed multiple ROIs within the mass and found that the combination of minimum ADC and ADC difference value showed best diagnostic performance compared to mean or maximum ADC values. Given the size of the ROI to be used, the lowest ADC value within the lesion was useful for the accurate discrimination between malignant and benign breast lesions (28,29). We measured the ADC values using both histogram analysis and the ROI-based method in the darkest part on ADC map. All ADC metrics obtained from histogram provided more significant differences between ILC and invasive carcinoma of NST compared to ROI-based method.
Recent studies demonstrated the clinical significance of ADC difference value in breast cancer patients (30,31). Higher ADC difference value was related with poorer distant metastasis-free survival in patients with invasive breast cancer (30) and a high risk of recurrence risk stratified using the Oncotype DX recurrence score in patients with ER-positive, HER2-negative and node-negative breast cancer (31). In our study, ADC difference value of ILC was higher than invasive carcinoma of NST and we presumed that infiltrative growth pattern of ILC made low cellularity area within the tumor and it would cause high ADC area.
The most important advantage of DWI is that it could diagnose breast cancer without contrast agent injection. Not only for differential diagnosis, but also for screening of high-risk women or women with dense breasts, several clinical trials are in progress. For successful cancer screening, it is of utmost importance to find and discriminate small-sized lesions. Conventional DWI is performed using single-shot echo planar imaging (EPI) and there are well known limitations such as susceptibility artifacts, image distortions, low signal-to-noise ratio and spatial blurring. Especially in case of small lesions less than 1cm, the conventional DWI axial in-plane spatial resolution of 2×2 mm2 and section thickness of 4 mm could cause a partial volume effect. For higher spatial resolution and reduced susceptibility artifacts, advanced DWI techniques such as readout-segmented EPI or reduced field-of view techniques have been developed (32,33). However, even though breast DWI shows better sensitivity compared to conventional screening techniques such as mammography or ultrasonography, its sensitivity is lower than the sensitivity of contrast-enhanced MRI (34). Given the high sensitivity and high negative predictive value of contrast-enhanced MRI, it seems to be too early to use only DWI for the cancer screening. Screening DWI could be useful in patients for whom contrast-enhanced MRI is not accessible or not appropriate. Larger prospective studies are needed to prove the effectiveness of DWI for breast cancer screening.
There are some limitations in our study. First, this study was performed from a single tertiary academic center and there could be selection bias because we included only women who had undergone breast MRI before surgery. Second, the quantitative ADC values were obtained from the representative single section of the ADC map. Data acquisition from the whole tumor volume may be better for comparison between two groups. Third, breast MRI was performed after biopsy and biopsy-related changes could affect the visibility and ADC values of the tumors. However, the average time interval between biopsy and MRI was 12 days ranging from 9 to 17 days, which may reduce the post-biopsy effect on DWI.
In conclusion, the visibility of ILC was lower and ILC showed higher quantitative ADC and higher ADC difference values compared to invasive carcinoma of NST. Small ILC can cause a false-negative diagnosis on DWI.
Acknowledgments
Funding: None.
Footnote
Conflicts of Interest: Both authors have completed the ICMJE uniform disclosure form (available at https://dx.doi.org/10.21037/qims-21-355). The authors have no conflicts of interest to declare.
Ethical Statement: The authors are accountable for all aspects of the work in ensuring that questions related to the accuracy or integrity of any part of the work are appropriately investigated and resolved. The study was conducted in accordance with the Declaration of Helsinki (as revised in 2013). Our institutional review board approved this retrospective study (AJIRB-MED-MDB-21-092) and individual consent for this retrospective analysis was waived.
Open Access Statement: This is an Open Access article distributed in accordance with the Creative Commons Attribution-NonCommercial-NoDerivs 4.0 International License (CC BY-NC-ND 4.0), which permits the non-commercial replication and distribution of the article with the strict proviso that no changes or edits are made and the original work is properly cited (including links to both the formal publication through the relevant DOI and the license). See: https://creativecommons.org/licenses/by-nc-nd/4.0/.
References
- Dorrius MD, Dijkstra H, Oudkerk M, Sijens PE. Effect of b value and pre-admission of contrast on diagnostic accuracy of 1.5-T breast DWI: a systematic review and meta-analysis. Eur Radiol 2014;24:2835-47. [Crossref] [PubMed]
- Spick C, Pinker-Domenig K, Rudas M, Helbich TH, Baltzer PA. MRI-only lesions: application of diffusion-weighted imaging obviates unnecessary MR-guided breast biopsies. Eur Radiol 2014;24:1204-10. [Crossref] [PubMed]
- Rahbar H, Zhang Z, Chenevert TL, Romanoff J, Kitsch AE, Hanna LG, et al. Utility of Diffusion-weighted Imaging to Decrease Unnecessary Biopsies Prompted by Breast MRI: A Trial of the ECOG-ACRIN Cancer Research Group (A6702). Clin Cancer Res 2019;25:1756-65. [Crossref] [PubMed]
- Park SH, Moon WK, Cho N, Song IC, Chang JM, Park IA, Han W, Noh DY. Diffusion-weighted MR imaging: pretreatment prediction of response to neoadjuvant chemotherapy in patients with breast cancer. Radiology 2010;257:56-63. [Crossref] [PubMed]
- Wu LM, Hu JN, Gu HY, Hua J, Chen J, Xu JR. Can diffusion-weighted MR imaging and contrast-enhanced MR imaging precisely evaluate and predict pathological response to neoadjuvant chemotherapy in patients with breast cancer? Breast Cancer Res Treat 2012;135:17-28. [Crossref] [PubMed]
- Liu S, Ren R, Chen Z, Wang Y, Fan T, Li C, Zhang P. Diffusion-weighted imaging in assessing pathological response of tumor in breast cancer subtype to neoadjuvant chemotherapy. J Magn Reson Imaging 2015;42:779-87. [Crossref] [PubMed]
- Yabuuchi H, Matsuo Y, Sunami S, Kamitani T, Kawanami S, Setoguchi T, Sakai S, Hatakenaka M, Kubo M, Tokunaga E, Yamamoto H, Honda H. Detection of non-palpable breast cancer in asymptomatic women by using unenhanced diffusion-weighted and T2-weighted MR imaging: comparison with mammography and dynamic contrast-enhanced MR imaging. Eur Radiol 2011;21:11-7. [Crossref] [PubMed]
- Kazama T, Kuroki Y, Kikuchi M, Sato Y, Nagashima T, Miyazawa Y, Sakakibara M, Kaneoya K, Makimoto Y, Hashimoto H, Motoori K, Takano H. Diffusion-weighted MRI as an adjunct to mammography in women under 50 years of age: an initial study. J Magn Reson Imaging 2012;36:139-44. [Crossref] [PubMed]
- Trimboli RM, Verardi N, Cartia F, Carbonaro LA, Sardanelli F. Breast cancer detection using double reading of unenhanced MRI including T1-weighted, T2-weighted STIR, and diffusion-weighted imaging: a proof of concept study. AJR Am J Roentgenol 2014;203:674-81. [Crossref] [PubMed]
- Telegrafo M, Rella L, Stabile Ianora AA, Angelelli G, Moschetta M. Unenhanced breast MRI (STIR, T2-weighted TSE, DWIBS): An accurate and alternative strategy for detecting and differentiating breast lesions. Magn Reson Imaging 2015;33:951-5. [Crossref] [PubMed]
- McDonald ES, Hammersley JA, Chou SH, Rahbar H, Scheel JR, Lee CI, Liu CL, Lehman CD, Partridge SC. Performance of DWI as a Rapid Unenhanced Technique for Detecting Mammographically Occult Breast Cancer in Elevated-Risk Women With Dense Breasts. AJR Am J Roentgenol 2016;207:205-16. [Crossref] [PubMed]
- Kang JW, Shin HJ, Shin KC, Chae EY, Choi WJ, Cha JH, Kim HH. Unenhanced magnetic resonance screening using fused diffusion-weighted imaging and maximum-intensity projection in patients with a personal history of breast cancer: role of fused DWI for postoperative screening. Breast Cancer Res Treat 2017;165:119-28. [Crossref] [PubMed]
- Baltzer P, Mann RM, Iima M, Sigmund EE, Clauser P, Gilbert FJ, Martincich L, Partridge SC, Patterson A, Pinker K, Thibault F, Camps-Herrero J, Le Bihan D. EUSOBI international Breast Diffusion-Weighted Imaging working group. Diffusion-weighted imaging of the breast-a consensus and mission statement from the EUSOBI International Breast Diffusion-Weighted Imaging working group. Eur Radiol 2020;30:1436-50. [Crossref] [PubMed]
- Woodhams R, Matsunaga K, Kan S, Hata H, Ozaki M, Iwabuchi K, Kuranami M, Watanabe M, Hayakawa K. ADC mapping of benign and malignant breast tumors. Magn Reson Med Sci 2005;4:35-42. [Crossref] [PubMed]
- Youk JH, Son EJ, Chung J, Kim JA, Kim EK. Triple-negative invasive breast cancer on dynamic contrast-enhanced and diffusion-weighted MR imaging: comparison with other breast cancer subtypes. Eur Radiol 2012;22:1724-34. [Crossref] [PubMed]
- Berg WA, Gutierrez L. NessAiver MS, Carter WB, Bhargavan M, Lewis RS, Ioffe OB. Diagnostic accuracy of mammography, clinical examination, US, and MR imaging in preoperative assessment of breast cancer. Radiology 2004;233:830-49. [Crossref] [PubMed]
- Jung Y, Jeong S, Kim JY, Kang DK, Kim TH. Correlations of female hormone levels with background parenchymal enhancement and apparent diffusion coefficient values in premenopausal breast cancer patients: Effects on cancer visibility. Eur J Radiol 2020;124:108818 [Crossref] [PubMed]
- Hahn SY, Ko ES, Han BK, Lim Y, Gu S, Ko EY. Analysis of factors influencing the degree of detectability on diffusion-weighted MRI and diffusion background signals in patients with invasive breast cancer. Medicine (Baltimore) 2016;95:e4086 [Crossref] [PubMed]
- Xu M, Tang Q, Li M, Liu Y, Li F. An analysis of Ki-67 expression in stage 1 invasive ductal breast carcinoma using apparent diffusion coefficient histograms. Quant Imaging Med Surg 2021;11:1518-31. [Crossref] [PubMed]
- Martincich L, Deantoni V, Bertotto I, Redana S, Kubatzki F, Sarotto I, Rossi V, Liotti M, Ponzone R, Aglietta M, Regge D, Montemurro F. Correlations between diffusion-weighted imaging and breast cancer biomarkers. Eur Radiol 2012;22:1519-28. [Crossref] [PubMed]
- Jeh SK, Kim SH, Kim HS, Kang BJ, Jeong SH, Yim HW, Song BJ. Correlation of the apparent diffusion coefficient value and dynamic magnetic resonance imaging findings with prognostic factors in invasive ductal carcinoma. J Magn Reson Imaging 2011;33:102-9. [Crossref] [PubMed]
- Bickel H, Pinker-Domenig K, Bogner W, Spick C, Bagó-Horváth Z, Weber M, Helbich T, Baltzer P. Quantitative apparent diffusion coefficient as a noninvasive imaging biomarker for the differentiation of invasive breast cancer and ductal carcinoma in situ. Invest Radiol 2015;50:95-100. [Crossref] [PubMed]
- Mori N, Ota H, Mugikura S, Takasawa C, Tominaga J, Ishida T, Watanabe M, Takase K, Takahashi S. Detection of invasive components in cases of breast ductal carcinoma in situ on biopsy by using apparent diffusion coefficient MR parameters. Eur Radiol 2013;23:2705-12. [Crossref] [PubMed]
- Wielema M, Dorrius MD, Pijnappel RM, De Bock GH, Baltzer PAT, Oudkerk M, Sijens PE. Diagnostic performance of breast tumor tissue selection in diffusion weighted imaging: A systematic review and meta-analysis. PLoS One 2020;15:e0232856 [Crossref] [PubMed]
- Marini C, Iacconi C, Giannelli M, Cilotti A, Moretti M, Bartolozzi C. Quantitative diffusion-weighted MR imaging in the differential diagnosis of breast lesion. Eur Radiol 2007;17:2646-55. [Crossref] [PubMed]
- Guo Y, Cai YQ, Cai ZL, Gao YG, An NY, Ma L, Mahankali S, Gao JH. Differentiation of clinically benign and malignant breast lesions using diffusion-weighted imaging. J Magn Reson Imaging 2002;16:172-8. [Crossref] [PubMed]
- Hirano M, Satake H, Ishigaki S, Ikeda M, Kawai H, Naganawa S. Diffusion-weighted imaging of breast masses: comparison of diagnostic performance using various apparent diffusion coefficient parameters. AJR Am J Roentgenol 2012;198:717-22. [Crossref] [PubMed]
- Bickel H, Pinker K, Polanec S, Magometschnigg H, Wengert G, Spick C, Bogner W, Bago-Horvath Z, Helbich TH, Baltzer P. Diffusion-weighted imaging of breast lesions: Region-of-interest placement and different ADC parameters influence apparent diffusion coefficient values. Eur Radiol 2017;27:1883-92. [Crossref] [PubMed]
- Arponen O, Sudah M, Masarwah A, Taina M, Rautiainen S, Könönen M, Sironen R, Kosma VM, Sutela A, Hakumäki J, Vanninen R. Diffusion-Weighted Imaging in 3.0 Tesla Breast MRI: Diagnostic Performance and Tumor Characterization Using Small Subregions vs. Whole Tumor Regions of Interest. PLoS One 2015;10:e0138702 [Crossref] [PubMed]
- Kim JY, Kim JJ, Hwangbo L, Kang T, Park H. Diffusion-weighted Imaging of Invasive Breast Cancer: Relationship to Distant Metastasis-free Survival. Radiology 2019;291:300-7. [Crossref] [PubMed]
- Kim JY, Kim JJ, Hwangbo L, Lee JW, Lee NK, Nam KJ, Choo KS, Kang T, Park H, Son Y, Grimm R. Diffusion-weighted MRI of estrogen receptor-positive, HER2-negative, node-negative breast cancer: association between intratumoral heterogeneity and recurrence risk. Eur Radiol 2020;30:66-76. [Crossref] [PubMed]
- Porter DA, Heidemann RM. High resolution diffusion-weighted imaging using readout-segmented echo-planar imaging, parallel imaging and a two-dimensional navigator-based reacquisition. Magn Reson Med 2009;62:468-75. [Crossref] [PubMed]
- Baltzer PAT, Bickel H, Spick C, Wengert G, Woitek R, Kapetas P, Clauser P, Helbich TH, Pinker K. Potential of Noncontrast Magnetic Resonance Imaging With Diffusion-Weighted Imaging in Characterization of Breast Lesions: Intraindividual Comparison With Dynamic Contrast-Enhanced Magnetic Resonance Imaging. Invest Radiol 2018;53:229-35. [Crossref] [PubMed]
- Pinker K, Moy L, Sutton EJ, Mann RM, Weber M, Thakur SB, Jochelson MS, Bago-Horvath Z, Morris EA, Baltzer PA, Helbich TH. Diffusion-Weighted Imaging With Apparent Diffusion Coefficient Mapping for Breast Cancer Detection as a Stand-Alone Parameter: Comparison With Dynamic Contrast-Enhanced and Multiparametric Magnetic Resonance Imaging. Invest Radiol 2018;53:587-95. [Crossref] [PubMed]