A nomogram based on bi-regional radiomics features from multimodal magnetic resonance imaging for preoperative prediction of microvascular invasion in hepatocellular carcinoma
Introduction
Hepatocellular carcinoma (HCC) is the sixth most prevalent cancer worldwide and one of the leading causes of cancer-related death (1). Hepatectomy and liver transplantation (LT) are potential curative modalities for HCC patients. Although several clinically based classification schemes have been used to guide the selection of therapeutic strategies, the 5-year recurrence rates can be as high as 70% after hepatectomy and 35% after LT (2-4). Studies have demonstrated that microvascular invasion (MVI) is the strongest independent predictor of early tumor recurrence (5). Alternative treatment strategies and preoperative adjuvant therapy should be considered for HCC patients who are at high risk of MVI (6,7). Unfortunately, MVI diagnosis can only reliably be made by histopathology of surgical or biopsy specimens, which is when its clinical utility is marginal. To minimize the risk of recurrence, a non-invasive method capable of accurately identifying MVI preoperatively is urgently needed to better stratify HCC patients for treatment decisions.
Over the past decade, many efforts have been made to preoperatively predict MVI. Current practice uses two clinical surrogate biomarkers, the Milan criteria, and serum alpha fetoprotein (AFP) levels, as predictors (8). LT is generally recommended in patients with tumors that meet the Milan criteria (a single tumor ≤5 cm or up to 3 tumors, each ≤3 cm). However, 30% of HCCs selected by the Milan criteria for LT has MVI and, conversely, 50% of tumors outside the Milan criteria have no MVI and would potentially benefit from LT (9,10). Serum AFP lacks sensitivity for the prediction of MVI, and even the model that combines both the Milan criteria and serum AFP has a sensitivity of only 50% (11). In addition to clinical predictors of MVI, several studies have reported that certain imaging findings, including the gross type of HCC (12), arterial rim enhancement or peritumoral enhancement (13,14), internal arteries (15), incomplete capsule (16), washout (17), and peritumoral hypointensity during the hepatobiliary phase (HBP) (18), are useful for predicting MVI of HCC. However, such qualitative radiographic descriptors suffer from limitations, including inter-reader variability and lack of external validation (19).
Recently, the emergence of radiomics has allowed the automated, high-throughput extraction of quantitative imaging features from regions of interest (ROIs) in routinely acquired radiologic images, which provide crucial insights into tumor heterogeneity, phenotype, and microenvironment (20). Few investigations have been published on the utility of radiomics for MVI prediction in HCC patients (8,19,21-24), and most of them are based on CT images. However, magnetic resonance imaging (MRI) carries an advantage of depicting more soft-tissue characteristics, and dynamic contrast-enhanced (DCE) MRI and diffusion-weighted imaging (DWI) has the potential to assess the tumor’s metabolism and proliferation with higher accuracy (25). Consequently, multimodal MRI may allow more effective radiomics. In addition, due to the multiregional and microenvironmental heterogeneity in HCC, it is reasonable to hypothesize that quantitative features from the whole tumor and peritumoral region could have the best accuracy in predicting MVI than those features from a single region.
Therefore, in this study, we aimed to investigate the use of bi-regional radiomics parameters derived from multimodal MR images to predict MVI of HCC, as well as to establish and validate a combined nomogram model that incorporates the developed radiomics signature with radiologic features and clinical risk factors for the preoperative prediction of MVI in HCC patients.
Methods
Patients
This study was approved by our Institutional Review Board, and the requirement for informed consent was waived. We retrospectively searched our institutional database between September 2016 and September 2018 and identified 459 patients who had undergone preoperative multiparameter MRI for HCC. The inclusion criteria were as follows: (I) histologically confirmed HCC; (II) single tumor with or without satellite nodules, which are lesions having a tumor diameter ≤2 cm and a distance from the main tumor ≤2 cm; (III) no preoperative cancer-related treatments, including transarterial chemoembolization and radiofrequency ablation; (IV) no macrovascular invasion on MRI; (V) an MR scan received within 1 month before curative hepatic resection or transplantation; and (VI) MR images with sufficient image quality. Patients who met the following criteria were excluded: (I) combined hepatocellular-cholangiocarcinoma, (II) recurrent HCC and (III) a history of other malignancies. The patient recruitment process is shown in Figure 1. The final cohort consisted of 267 consecutive patients (229 males and 38 females; range, 31–83 years; mean age, 57.93±10.65 years). The cohort was divided into a training set (n=194; 165 males and 29 females; range, 31–83 years; mean age, 58.35±10.85 years; from September 2016 to February 2018) and a validation set (n=73; 64 males and 9 females; range, 40–77 years; mean age, 56.82±9.40 years; from March 2018 to September 2018) according to the MR examination date.
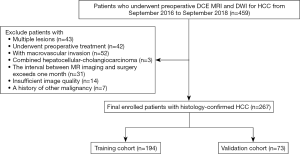
Clinical and pathological variables
The demographic characteristics, including sex, age, and hepatitis status (presence or absence), were gathered from the electronic medical record system. Data on cirrhosis, MVI, and Edmondson-Steiner (E-S) grade were obtained from pathology reports. MVI was defined as a tumor within a vascular space lined by endothelium that was visible only by microscopy (5). Specimens from hepatectomy or LT were sampled by using the “7 points” baseline sampling method (26). Laboratory data, including albumin, gamma-glutamyl transferase (GGT), and AFP were obtained via preoperative routine blood tests. Patients were classified by the Barcelona clinic liver cancer (BCLC) staging system and Child-Pugh score based on MRI findings and medical history. Clinical and pathological descriptions of these two cohorts are fully detailed in Table 1.
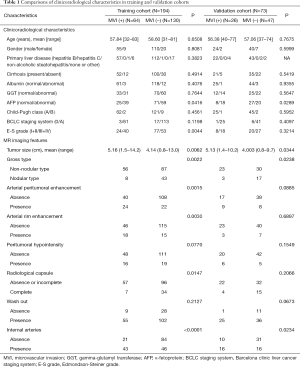
Full table
MR image acquisition
MR examinations were performed using a 3.0 T scanner (GE Medical Systems, Milwaukee, WI, USA). Our liver MRI protocol consisted of axial T2-weighted imaging with fat suppression, dual-echo (in-phase and opposed-phase) T1-weighted imaging, DWI, and pre-contrast and post-contrast dynamic three-dimensional fast-spoiled gradient-recalled echo sequence (liver acceleration volume acquisition, LAVA). DWI was obtained using a respiratory triggering, a single-shot echo-planar imaging pulse sequence with b values of 0 and 1,000 mm2/s. The acquisition parameters for LAVA were as follows: repetition time (TR) of 3.2 ms; echo time (TE) of 1.5 ms; reverse time of 5 ms; field of view of 380×304 mm2; flip angle of 10°; bandwidth of 100 kHz; and image resolution of 1.19×1.19×5 mm3. Post-contrast dynamic LAVA was performed at the arterial phase (20 s), portal venous phase (60 s), and equilibrium phase (180 s) after a rapid bolus injection of 0.1 mmol/kg of gadopentetate dimeglumine (magnevistt®, Bayer Schering Pharma, Berlin, Germany) into the cubital vein at a rate of 2.5 mL/s, followed by a 15-mL saline flush.
Qualitative radiographic descriptors
Image analysis was performed by two abdominal radiologists (R. Z. and J. Z., with 10 and 15 years of experience in liver MRI, respectively) who were blinded to clinical, laboratory, and pathologic information. The two radiologists independently evaluated the following eight MR imaging features for each HCC including tumor size, the gross type of HCC (including nodule and non-nodule types), arterial rim enhancement, arterial peritumoral parenchymal enhancement, washout, and peritumoral hypointensity in the later phase, radiological capsule, and internal arteries, as previously described (12-18).
Image segmentation and radiomics feature extraction
The whole tumor was manually depicted along with the lesion outline on each axial slice of the T1-weighted images, T2-weighted images, DWI images, and three-phase DCE images by a radiologist with ten years of professional experience using ITK-SNAP (http://www.nitrc.org/projects/itk-snap/). The delineated tumor boundary was independently validated by another radiologist to reduce possible bias.
The pre-processing procedure was undertaken before feature extraction, including image resampling and grey level normalization. The voxel resample was performed using three-dimensional Lagrangian polygon interpolation on MATLAB software (27). All voxel sizes of all images were resampled with the same size of 1×1×1 mm3. The image grey level was normalized to a scale of 1 to 64. To capture quantitative features from the area around the tumor, a surrounding tissue area of approximately 10 mm was created using MATLAB 2017b (MathWorks Inc., Natick, MA, USA) (http://www.mathworks.com/products/matlab/), the lesion segmentation is shown in Figure 2. A total of 484 radiomics features were extracted from each of the 2 ROIs (tumor and surrounding tissue), which included three types: (I) 7 intensity features; (II) 53 texture features; and (III) 424 wavelet features. For triphasic DCE MR images, 14 inside-outside co-occurrence features were further calculated for each of the 2 ROIs, including the intensity changes and the corresponding intensity change ratios. The intensity changes were calculated by subtracting the signal intensity of the lesion in the first/third phase from that in the second phase. The corresponding intensity change ratios were calculated by dividing the signal intensity of the lesion in the second phase by the intensity changes of the second phase. During the liver DCE MR scanning, the diaphragm was hardly assured to be in the same position for each scan. Thus, the intensities of the lesion in the three phases were calculated using the mean value of the intensities within ROIs. The detailed descriptions of these features are presented in Figure S1. By performing stability and reproducibility test for radiomcis features, a number of 2,516 features showed desirable reproducibility. The detailed results of reproducibility test were provided in Supplementary I.
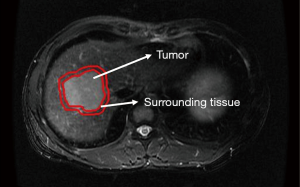
Radiomics feature selection and signature construction
The extracted radiomics features were normalized to eliminate the value scales of the data both in the training and validation cohorts. The training cohort was used to build a radiomics signature as the MVI classifier. More features may improve the classifiers performance; however, more features may cause the model overfitting. The minimum redundancy maximum relevance (mRMR) algorithm was applied to identify the optimal radiomics features. The mRMR ranks the input radiomics features by maximizing the mutual information (MI) with respect to the class labels and minimizing the average MI of selected features (28). After the mRMR feature selection, the optimal feature subset was generated. Then, a radiomics signature was built with multivariable logistic regression analysis using the selected features. The performances of the radiomics signatures from single MR sequences and fusion radiomics signatures from multiple sequences were evaluated using the area under the receiver operating characteristic (ROC) curve (AUC) both in the training and validation cohort. Multiple comparisons of the curves were performed using the Delong test to identify the optimal signature.
To minimize the impact of established predictors of MVI in HCC (tumor size and AFP level) (29,30), all HCC patients were divided into two subgroups defined according to two risk factors. The predictive performance of the optimal radiomics signature was evaluated within subgroups using the AUCs from both the training and validation cohorts.
Construction and evaluation of MVI prediction models
Clinicoradiological risk factors were analyzed for significant differences between the MVI and non-MVI groups using the Mann-Whitney U test, and Chi-square test, as appropriate. In the training cohort, a clinicoradiological model was developed with stepwise multivariable logistic regression using a backward search method.
In the training cohort, a combination model incorporating the optimal radiomics and clinicoradiological characteristics were developed using multivariable logistic regression analysis. The backward step-wise selection was applied using the likelihood ratio test with Akaike’s information criterion (AIC) as the stopping rule. The model with the minimum AIC value was selected as the final model.
Comparisons of the predictive performances among the optimal radiomics signature, the clinicoradiological model, and the combined radiomics model were performed using the Delong test.
Construction and validation of the radiomics nomogram
A radiomics nomogram was generated on the prediction model as a graphical presentation (31). The performance of the nomogram was evaluated based on its capabilities in discrimination, calibration, and clinical utility in both the training and validation cohorts. The discrimination capability was measured using the AUC. Calibration curves were plotted to intuitively evaluate the predictive accuracy of the nomogram (32). The calibration curve takes the nomogram-predicted probability of MVI as the abscissa and the actual rate obtained by the bootstrapping method as the ordinate. The closer the calibration curve is to the diagonal line, the higher the prediction accuracy of the model. The Hosmer-Lemeshow test was used to test the goodness-of-fit of the nomogram. Decision curve analysis was conducted to evaluate the clinical utility of the nomogram by quantifying the probabilities of net benefits at a threshold across 0.0 to 1.0 (33). The decision curve takes the threshold of nomogram-predicted probability for MVI as the abscissa and clinical decision net benefit as the ordinate. The two extreme curves of the treat-all strategy and the treat-none strategy are used as references; the farther the decision curve is from the two extreme curves, the higher the clinical decision net benefit of the model.
Statistical analysis
All statistical analyses were performed in R software (version 3.4.1; http://www.Rproject.org) and MedCalc software (Version 15.2.2; https://www.medcalc.org). The mRMR feature selection was performed using the “mRMRe” package. Nomogram and calibration curves were plotted using the “rms” package. The Hosmer-Lemeshow test was performed using the “generalhoslem” package. The ROC curves were plotted using MedCalc software. A two-sided P value of 0.05 was used to determine the statistical significance.
Results
Clinicoradiological characteristics
Clinicoradiological characteristics for patients in the training and validation cohorts are listed in Table 1. The univariate analyses showed that four clinicoradiological characteristics including AFP level, tumor size, gross tumor type, and presence of internal arteries had significant differences between the patients with and without MVI in both the training and validation cohorts.
Based on the multivariate analysis that used the minimum Akaike information criterion, an optimal clinicoradiological model was constructed using gross tumor type, arterial peritumoral enhancement, arterial rim enhancement, internal arteries, and AFP level. The formula of the clinicoradiological model is provided in Figure S2. The AUCs of the clinicoradiological model was 0.753 (95% CI: 0.686–0.812) for the training cohort and 0.729 (95% CI: 0.612–0.827) for the validation cohort (Table 2).
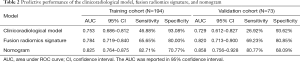
Full table
Construction and validation of the radiomics signatures
The performances of radiomics signatures using a single MR sequence and the fusion radiomics signatures using multi-sequence are shown in Table S2. Obviously, the fusion radiomics signature based on triphasic DCE MR images showed the best predictive performance, with an AUC of 0.784 (95% CI: 0.719–0.840) in the training cohort and 0.820 (95% CI: 0.713–0.900) in the validation cohort. The formula for the fusion radiomics signature is provided as a Figure S2.
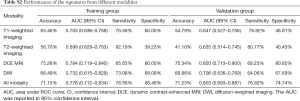
Full table
According to tumor size, all HCC patients were divided into two sub-groups: tumor size ≤5 cm (n=182) and tumor size >5 cm (n=85). According to the AFP level, all patients were divided into two groups: AFP level ≤20 ng/mL (n=131) and AFP level >20 ng/mL (n=136). The predictive performance of the fusion radiomics signature within subgroups defined by tumor size and AFP level is summarized in Table 3.
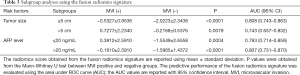
Full table
Construction and comparison of MVI prediction models
The combined radiomics model that added the fusion radiomics signature into the clinicoradiological factors exhibited an AUC of 0.825 (95% CI: 0.764–0.875) with a sensitivity of 82.71% and a specificity of 70.77% in the training cohort. The nomogram yielded an AUC of 0.858 (95% CI: 0.756–0.928) with a sensitivity of 80.77% and a specificity of 68.09% in the validation cohort (Table 2). For MVI prediction, the combined radiomics model showed the best performance compared with the clinicoradiological model (AUCs: 0.858 vs. 0.729; P=0.022) in the validation cohort, although the training did not have statistical significance (AUCs: 0.825 vs. 0.753; P=0.055). ROC curves for the prediction for MVI were compared among the optimal radiomics signatures, and the best clinicoradiological model and the combined radiomics model are shown in Figure 3A,B, respectively.
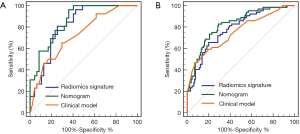
Development and validation of the radiomics nomogram
The nomogram based on the combined radiomics model is presented in Figure 4. Calibration curves (Figure 5A,B) showed good consistency between the nomogram-predicted probability of MVI and the actual MVI rate. The Hosmer-Lemeshow test yielded nonsignificant statistics for both the training cohort (P=0.7541) and the validation cohort (P=0.7916). The decision curve (Figure 5C,D) demonstrated a higher net benefit of the nomogram than that of the treat-all strategy and the treat-none strategy. This result suggests that a therapy strategy based on the nomogram will improve the clinical outcome. An example of representative multimodal MR and relevant pathological images of two patients is given in Figure 6.
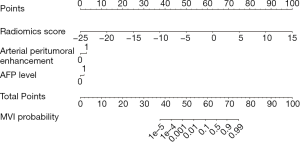
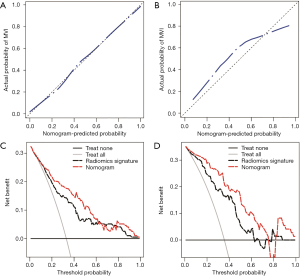
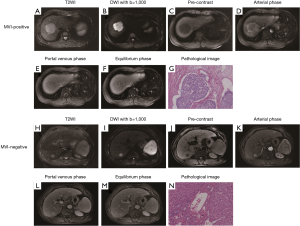
Discussion
In this study, we developed and validated a radiomics signature derived from MR images for preoperatively predicting MVI in HCC patients, which showed good discrimination both in the training and validation cohorts. Encouragingly, the diagnostic performance of the radiomics signature did not vary with tumor size or AFP level, suggesting that it is a robust predictor of MVI. The radiomics nomogram that incorporates fusion radiomics signature and clinicoradiological characteristics achieved satisfactory preoperative prediction of MVI, which may aid in clinical decision-making.
Recently few studies about the utility of radiomics in MVI prediction of HCC have been reported. Bakr et al. (8) explored noninvasive biomarkers of MVI in HCC using quantitative and semantic image features extracted from triphasic contrast-enhanced CT images, showing that the radiomics signature better-identified MVI (AUC=0.76), compared to the semantic feature signature. However, the case number was 28 only, and the data lacked further validation. Zheng et al. (19) created two multivariate models for the prediction of MVI using quantitative image analysis, where the tumor was stratified by size, one model for patients with ≤5 cm tumors predicted MVI with an AUC of 0.80, and the other model for patients with >5 cm tumors with an AUC of 0.88. The study similarly lacked further validation, and quantitative image analysis was only performed on a tumor-liver interface; however, the internal region of a tumor is also very informative and may reflect the tumor’s underlying biological properties. Thus, our study used bi-regional radiomics parameters to predict the MVI of HCC. Peng et al. (21) reported that a nomogram that included portal venous and arterial phase CT-based radiomics features, radiologic features, and clinical factors could potentially distinguish MVI+ and MVI− in HBV-related HCC, with an AUC of 0.846 in the training cohort and 0.844 in the validation cohort, which was in accord with the results of our study. However, unlike our study, their study used only the largest cross-sectional area rather than the entire tumor, which might have caused information loss (34), and only extract portal venous and arterial phase CT data. Because our study fully extracted and utilized the space and timing characteristics contained in the triphasic DCE-MR images, the results of our study were more repeatable. Xu et al. (22) developed a computational approach integrating large-scale clinical and imaging modalities, especially radiomic features from contrast-enhanced CT, to predict MVI and clinical outcomes in HCCs, but the study did not include an equilibrium phase that would have been useful for the prediction of MVI (8). Ma et al. (23) were first to use 3D contrast-enhanced CT images to build a radiomics signature for predicting the MVI status of HCC, with an AUC of 0.727 in the training dataset. Our radiomics signature exhibited better performance; in addition to bi-regional radiomic features, the additional information carried by MR imaging might be one of the reasons for this improved performance. In addition to the above studies based on CT images, recently, Feng et al. (24) reported radiomics analysis of MR images for MVI prediction; however, the above studies were both based on liver-specific agent-enhanced MR images, and the costs of liver-specific agents are too high to be suitable for most HCC patients.
Our results revealed that the fusion radiomics signature derived from triphasic DCE MR images achieved optimal performance. This finding was not unexpected given that MVI can change the tumor perfusion by minute portal venous invasion (35,36), leading to detectable differences in contrast-enhancement between HCCs with and without MVI. However, the AUC of the radiomics signature derived from DW images for prediction of MVI in the present study was not very high (up to 0.732). The possible explanations may be due to the lower imaging resolution of DW images, which might be insufficient to reveal radiomics feature differences. Some predictive features came from the peritumoral region in our study. Zheng et al. (19) showed that texture analysis in CT enhancement at the liver-tumor interface could predict MVI status in HCCs. The peritumoral region is a very informative region that may reflect underlying tumor biological properties, including MVI, extracellular matrix remodeling, and associated inflammatory response (15,36,37). During construction of the optimal radiomics signature, nearly all of the predictive features were wavelet features. This is in accord with previous studies that incorporated wavelet features in radiomics signature construction (38-42). The three-dimensional wavelet transformation decomposed image data into different frequency components, which may be useful for further exploration of the spatial tumor heterogeneity on multiple scales (39).
The fusion radiomics signature still performed well in the subgroups defined by tumor size and AFP level, which has very important clinical applicability. The fusion radiomics signature could contribute to the improved stratification of HCC patients into subgroups for individualized treatment. For example, the Milan criteria for LT is clearly imperfect, as described above. In this regard, the use of the radiomics signature for MVI prediction could broaden the criteria and further optimize the selection of LT patients. Moreover, we found that the radiomics signature had more discriminating power in tumors ≤5 cm, compared to those that were >5 cm, and it also showed a strong discriminating power in tumors with normal serum AFP levels, suggesting that the biomarker could be used as an early detector for the aggressive behavior of HCC.
In this study, we also evaluated the clinicoradiological factors as surrogate biomarkers of MVI in HCC. Serum AFP levels and arterial peritumoral enhancement, which were included in our combined radiomics model, were also independent risk factors for MVI. These results agreed with those from prior studies (8,11,13,14). Surprisingly, in this study, tumor size was not an independent risk factor for MVI, even though it was a significant predictor in the univariate analysis. Large tumor size has long been considered to increase the risk of MVI (43), but it has not always been proven to be an independent predictor (44). One possible reason was the selection bias: in this study, nearly 34% of HCCs exhibited MVI positivity, having a mean tumor size of 4.6 cm.
The combined model incorporating the significant clinicoradiological characteristics with the radiomics signature performed better than the radiomics signature alone did in the preoperative prediction of MVI for different individuals with HCCs. Perhaps a combination of more factors such as genomic datasets might obtain better efficiency of MVI prediction in HCCs. However, using multiple factors to predict MVI might also increase the burden on patients due to additional tests and exorbitant costs. Our developed combined model based on routinely available radiologic images and clinical laboratory data was easy to implement in routine clinical practice. Furthermore, of all the available prediction tools, a nomogram can provide a personalized, risk score-based, and highly accurate estimation along with ease of use (31). Our nomogram, based on the combined radiomics model, performed well, and demonstrated clinical utility for individualized therapeutic decision making.
This study has several limitations. First, this is a retrospective single-center study and requires further prospective multicentre validation with larger cohorts. Second, we did not examine tumor histopathology to correlate radiomics features with the exact locations of microscopic tumor thrombi, but promising results in this study may guide future studies on radiopathological correlations. Third, a follow-up of postoperative patients was not performed in this study. Next, we will follow up these patients and explore the potential of the combined nomogram in a survival prediction.
In conclusion, the fusion radiomics signature derived from triphasic DCE MR images can effectively predict MVI in HCC patients. The diagnostic performance of the fusion radiomics signature did not vary with tumor size or AFP level, suggesting that it is a robust predictor of MVI. The combined radiomics nomogram that incorporates clinicoradiological characteristics and the fusion radiomics signature achieved satisfactory preoperative prediction of MVI and thus will be able to assist clinicians in selecting optimal treatment strategies to improve clinical outcomes.
Supplementary
Supplementary I Stability and reproducibility test for radiomics features
We performed the stability and reproducibility test for image features. We randomly chose 60 patients from the overall patient dataset. A radiologist (J. Z.) repeated the tumor segmentation process for these 60 patients in DCE images. Then, the quantitative image features were extracted again. We defined the feature set used in the signature construction process as feature-set 1, the feature set extracted to test the stability and reproducibility as the feature-set 2. We used the Mann-Whitney U test to assess the difference for each image feature between the feature-set 1 and feature-set 2. Then, the correlation coefficient for each image feature was calculated using the Spearman's rank correlation test between the feature set-1 and feature set-2.
A total of 2,561 features (the overall number: 2,932) showed no statistical significant difference between the feature-set 1 and feature-set 2. By using the correlation test, 2,463 image features showed statistical correlation between feature-set 1 and feature-set 2. The correlation coefficients and P values for features used in the radiomics signature are shown in Table S1.
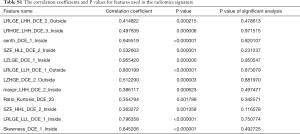
Full table
Acknowledgments
Funding: This research was funded by the Major Research Program of the National Natural Science Foundation of China-CT image registration and clinical application (91630311), and the Major Project of the Joint Foundation for Zhejiang Natural Science Foundation and Zhejiang Mathematical Medicine Foundation (LSD19H180003).
Footnote
Conflicts of Interest: The authors have no conflicts of interest to declare.
Ethical Statement: This study was approved by our Institutional Review Board, and the requirement for informed consent was waived.
References
- Forner A, Llovet JM, Bruix J. Hepatocellular carcinoma. Lancet (London, England) 2012;379:1245-55. [Crossref] [PubMed]
- Fujiwara N, Friedman SL, Goossens N, Hoshida Y. Risk factors and prevention of hepatocellular carcinoma in the era of precision medicine. J Hepatol 2018;68:526-49. [Crossref] [PubMed]
- Llovet JM, Schwartz M, Mazzaferro V. Resection and liver transplantation for hepatocellular carcinoma. Semin Liver Dis 2005;25:181-200. [Crossref] [PubMed]
- De Angelis R, Sant M, Coleman MP, Francisci S, Baili P, Pierannunzio D, Trama A, Visser O, Brenner H, Ardanaz E, Bielska-Lasota M, Engholm G, Nennecke A, Siesling S, Berrino F, Capocaccia R. EUROCARE-5 Working Group. Cancer survival in Europe 1999-2007 by country and age: results of EUROCARE--5-a population-based study. Lancet Oncol 2014;15:23-34. [Crossref] [PubMed]
- Roayaie S, Blume IN, Thung SN, Guido M, Fiel MI, Hiotis S, Labow DM, Llovet JM, Schwartz ME. A system of classifying microvascular invasion to predict outcome after resection in patients with hepatocellular carcinoma. Gastroenterology 2009;137:850-5. [Crossref] [PubMed]
- Shi M, Guo RP, Lin XJ, Zhang YQ, Chen MS, Zhang CQ, Lau WY, Li JQ. Partial hepatectomy with wide versus narrow resection margin for solitary hepatocellular carcinoma: a prospective randomized trial. Ann Surg 2007;245:36-43. [Crossref] [PubMed]
- Omata M, Cheng AL, Kokudo N, Kudo M, Lee JM, Jia J, Tateishi R, Han KH, Chawla YK, Shiina S, Jafri W, Payawal DA, Ohki T, Ogasawara S, Chen PJ, Lesmana CRA, Lesmana LA, Gani RA, Obi S, Dokmeci AK, Sarin SK. Asia-Pacific clinical practice guidelines on the management of hepatocellular carcinoma: a 2017 update. Hepatol Int 2017;11:317-370. [Crossref] [PubMed]
- Bakr S, Echegaray S, Shah R, Kamaya A, Louie J, Napel S, Kothary N, Gevaert O. Noninvasive radiomics signature based on quantitative analysis of computed tomography images as a surrogate for microvascular invasion in hepatocellular carcinoma: a pilot study. J Med Imaging (Bellingham) 2017;4:041303. [Crossref] [PubMed]
- Mazzaferro V, Regalia E, Doci R, Andreola S, Pulvirenti A, Bozzetti F, Montalto F, Ammatuna M, Morabito A, Gennari L. Liver transplantation for the treatment of small hepatocellular carcinomas in patients with cirrhosis. N Engl J Med 1996;334:693-9. [Crossref] [PubMed]
- Grasso A, Stigliano R, Morisco F, Martines H, Quaglia A, Dhillon AP, Patch D, Davidson BR, Rolles K, Burroughs AK. Liver transplantation and recurrent hepatocellular carcinoma: predictive value of nodule size in a retrospective and explant study. Transplantation 2006;81:1532-41. [Crossref] [PubMed]
- Duvoux C, Roudot-Thoraval F, Decaens T, Pessione F, Badran H, Piardi T, Francoz C, Compagnon P, Vanlemmens C, Dumortier J, Dharancy S, Gugenheim J, Bernard PH, Adam R, Radenne S, Muscari F, Conti F, Hardwigsen J, Pageaux GP, Chazouillères O, Salame E, Hilleret MN, Lebray P, Abergel A, Debette-Gratien M, Kluger MD, Mallat A, Azoulay D, Cherqui D. Liver Transplantation French Study Group. Liver transplantation for hepatocellular carcinoma: a model including α-fetoprotein improves the performance of Milan criteria. Gastroenterology 2012;143:986-94.e3. [Crossref] [PubMed]
- He J, Shi J, Fu X, Mao L, Zhou T, Qiu Y, Zhu B. The Clinicopathologic and Prognostic Significance of Gross Classification on Solitary Hepatocellular Carcinoma After Hepatectomy. Medicine (Baltimore) 2015;94:e1331. [Crossref] [PubMed]
- An C, Kim DW, Park YN, Chung YE, Rhee H, Kim MJ. Single hepatocellular carcinoma: preoperative MR imaging to predict early recurrence after curative resection. Radiology 2015;276:433-43. [Crossref] [PubMed]
- Renzulli M, Brocchi S, Cucchetti A, Mazzotti F, Mosconi C, Sportoletti C, Brandi G, Pinna AD, Golfieri R. Can current preoperative imaging be used to detect microvascular invasion of hepatocellular carcinoma? Radiology 2016;279:432-42. [Crossref] [PubMed]
- Banerjee S, Wang DS, Kim HJ, Sirlin CB, Chan MG, Korn RL, Rutman AM, Siripongsakun S, Lu D, Imanbayev G, Kuo MD. A computed tomography radiogenomic biomarker predicts microvascular invasion and clinical outcomes in hepatocellular carcinoma. Hepatology 2015;62:792-800. [Crossref] [PubMed]
- Reginelli A, Vanzulli A, Sgrazzutti C, Caschera L, Serra N, Raucci A, Urraro F, Cappabianca S. Vascular microinvasion from hepatocellular carcinoma: CT findings and pathologic correlation for the best therapeutic strategies. Med Oncol 2017;34:93. [Crossref] [PubMed]
- Witjes CD, Willemssen FE, Verheij J, van der Veer SJ, Hansen BE, Verhoef C, de Man RA, Ijzermans JN. Histological differentiation grade and microvascular invasion of hepatocellular carcinoma predicted by dynamic contrast-enhanced MRI. J Magn Reson Imaging 2012;36:641-7. [Crossref] [PubMed]
- Kim KA, Kim MJ, Jeon HM, Kim KS, Choi JS, Ahn SH, Cha SJ, Chung YE. Prediction of microvascular invasion of hepatocellular carcinoma: usefulness of peritumoral hypointensity seen on gadoxetate disodium-enhanced hepatobiliary phase images. J Magn Reson Imaging 2012;35:629-34. [Crossref] [PubMed]
- Zheng J, Chakraborty J, Chapman WC, Gerst S, Gonen M, Pak LM, Jarnagin WR, DeMatteo RP, Do RKG, Simpson AL. Hepatopancreatobiliary Service in the Department of Surgery of the Memorial Sloan Kettering Cancer Center. Research Staff in the Department of Surgery at Washington University School of Medicine. Preoperative Prediction of Microvascular Invasion in Hepatocellular Carcinoma Using Quantitative Image Analysis. J Am Coll Surg 2017;225:778-788.e1. [Crossref] [PubMed]
- Lambin P, Leijenaar RTH, Deist TM, Peerlings J, de Jong EEC, van Timmeren J, Sanduleanu S, Larue RTHM, Even AJG, Jochems A, van Wijk Y, Woodruff H, van Soest J, Lustberg T, Roelofs E, van Elmpt W, Dekker A, Mottaghy FM, Wildberger JE, Walsh S. Radiomics: the bridge between medical imaging and personalized medicine. Nat Rev Clin Oncol 2017;14:749-62. [Crossref] [PubMed]
- Peng J, Zhang J, Zhang Q, Xu Y, Zhou J, Liu L. A radiomics nomogram for preoperative prediction of microvascular invasion risk in hepatitis B virus-related hepatocellular carcinoma. Diagn Interv Radiol 2018;24:121-7. [Crossref] [PubMed]
- Xu X, Zhang HL, Liu QP, Sun SW, Zhang J, Zhu FP, Yang G, Yan X, Zhang YD, Liu XS. Radiomic analysis of contrast-enhanced CT predicts microvascular invasion and outcome in hepatocellular carcinoma. J Hepatol 2019;70:1133-44. [Crossref] [PubMed]
- Ma X, Wei J, Gu D, Zhu Y, Feng B, Liang M, Wang S, Zhao X, Tian J. Preoperative radiomics nomogram for microvascular invasion prediction in hepatocellular carcinoma using contrast-enhanced CT. Eur Radiol 2019;29:3595-605. [Crossref] [PubMed]
- Feng ST, Jia Y, Liao B, Huang B, Zhou Q, Li X, Wei K, Chen L, Li B, Wang W, Chen S, He X, Wang H, Peng S, Chen ZB, Tang M, Chen Z, Hou Y, Peng Z, Kuang M. Preoperative prediction of microvascular invasion in hepatocellular cancer: a radiomics model using Gd-EOB-DTPA-enhanced MRI. Eur Radiol 2019;29:4648-59. [Crossref] [PubMed]
- Colen RR, Wang J, Singh SK, Gutman DA, Zinn PO. Glioblastoma: imaging genomic mapping reveals sexspecific oncogenic associations of cell death. Radiology 2015;275:215-27. [Crossref] [PubMed]
- Cong WM, Bu H, Chen J, Dong H, Zhu YY, Feng LH, Chen J. Guideline Committee. Practice guidelines for the pathological diagnosis of primary liver cancer: 2015 update. World J Gastroenterol 2016;22:9279-87. [Crossref] [PubMed]
- Wu J, Aguilera T, Shultz D, Gudur M, Rubin DL, Loo BW Jr, Diehn M, Li R. Early-Stage Non-Small Cell Lung Cancer: Quantitative Imaging Characteristics of (18)F Fluorodeoxyglucose PET/CT Allow Prediction of Distant Metastasis. Radiology 2016;281:270-8. [Crossref] [PubMed]
- Prasanna P, Patel J, Partovi S, Madabhushi A, Tiwari P. Radiomic features from the peritumoral brain parenchyma on treatment-naïve multi-parametric MR imaging predict long versus short-term survival in glioblastoma multiforme: Preliminary findings. Eur Radiol 2017;27:4188-97. [Crossref] [PubMed]
- Duffy JP, Vardanian A, Benjamin E, Watson M, Farmer DG, Ghobrial RM, Lipshutz G, Yersiz H, Lu DS, Lassman C, Tong MJ, Hiatt JR, Busuttil RW. Liver transplantation criteria for hepatocellular carcinoma should be expanded: a 22-year experience with 467 patients at UCLA. Ann Surg 2007;246:502-9. [Crossref] [PubMed]
- Shim JH, Han S, Lee YJ, Lee SG, Kim KM, Lim YS, Chung YH, Lee YS, Lee HC. Half-life of serum alpha-fetoprotein: an early prognostic index of recurrence and survival after hepatic resection for hepatocellular carcinoma. Ann Surg 2013;257:708-17. [Crossref] [PubMed]
- Balachandran VP, Gonen M, Smith JJ, DeMatteo RP. Nomograms in oncology: more than meets the eye. Lancet Oncol 2015;16:e173-80. [Crossref] [PubMed]
- Kramer AA, Zimmerman JE. Assessing the calibration of mortality benchmarks in critical care: The Hosmer-Lemeshow test revisited. Crit Care Med 2007;35:2052-6. [Crossref] [PubMed]
- Vickers AJ, Elkin EB. Decision curve analysis: a novel method for evaluating prediction models. Med Decis Making 2006;26:565-74. [Crossref] [PubMed]
- Ng F, Kozarski R, Ganeshan B, Goh V. Assessment of tumor heterogeneity by CT texture analysis: can the largest crosssectional area be used as an alternative to whole tumor analysis? Eur J Radiol 2013;82:342-8. [Crossref] [PubMed]
- Simpson-Herren L, Noker PE, Wagoner SD. Variability of tumor response to chemotherapy. II. Contribution of tumor heterogeneity. Cancer Chemother Pharmacol 1988;22:131-6. [Crossref] [PubMed]
- Eccles SA, Welch DR. Metastasis: recent discoveries and novel treatment strategies. Lancet 2007;369:1742-57. [Crossref] [PubMed]
- Ünal E, İdilman İS, Akata D, Özmen MN, Karçaaltıncaba M. Microvascular invasion in hepatocellular carcinoma. Diagn Interv Radiol 2016;22:125-32. [Crossref] [PubMed]
- Liang W, Yang P, Huang R, Xu L, Wang J, Liu W, Zhang L, Wan D, Huang Q, Lu Y, Kuang Y, Niu T. A Combined Nomogram Model to Preoperatively Predict Histologic Grade in Pancreatic Neuroendocrine Tumors. Clin Cancer Res 2019;25:584-94. [Crossref] [PubMed]
- Wilson R, Devaraj A. Radiomics of pulmonary nodules and lung cancer. Transl Lung Cancer Res 2017;6:86-91. [Crossref] [PubMed]
- Huang Y, Liu Z, He L, Chen X, Pan D, Ma Z, Liang C, Tian J, Liang C. Radiomics Signature: A Potential Biomarker for the Prediction of Disease-Free Survival in Early-Stage (I or II) Non-Small Cell Lung Cancer. Radiology 2016;281:947-57. [Crossref] [PubMed]
- Wu S, Zheng J, Li Y, Yu H, Shi S, Xie W, Liu H, Su Y, Huang J, Lin T. A Radiomics Nomogram for the Preoperative Prediction of Lymph Node Metastasis in Bladder Cancer. Clin Cancer Res 2017;23:6904-11. [Crossref] [PubMed]
- Wu S, Zheng J, Li Y, Wu Z, Shi S, Huang M, Yu H, Dong W, Huang J, Lin T. Development and Validation of an MRI-Based Radiomics Signature for the Preoperative Prediction of Lymph Node Metastasis in Bladder Cancer. EBioMedicine 2018;34:76-84. [Crossref] [PubMed]
- Kaibori M, Ishizaki M, Matsui K, Kwon AH. Predictors of microvascular invasion before hepatectomy for hepatocellular carcinoma. J Surg Oncol 2010;102:462-8. [Crossref] [PubMed]
- Shirabe K, Kajiyama K, Abe T, Sakamoto S, Fukuya T, Akazawa K, Morita K, Maehara Y. Predictors of microscopic portal vein invasion by hepatocellular carcinoma: measurement of portal perfusion defect area ratio. J Gastroenterol Hepatol 2009;24:1431-6. [Crossref] [PubMed]