Radiomics derived from T2-FLAIR: the value of 2- and 3-classification tasks for different lesions in multiple sclerosis
Introduction
Multiple sclerosis (MS) is a chronic autoimmune disease characterized by focal lesions mainly involving white matter (WM) (1). MS patients exhibit remarkable heterogeneity of lesion types and these lesions have been proven to correspond to different histopathological types. Neuropathological studies have shown that MS lesions can be classified into four categories: acute active lesions, chronic active/smoldering lesions, chronic inactive, and remyelinated lesions (2,3). Acute active lesions, also called contrast enhancement lesions (CELs), show enhancement on T1-weighted (T1w) post-contrast magnetic resonance imaging (MRI), representing the breakdown of blood-brain-barrier (BBB) and acute inflammation (4). Chronic active/smoldering lesions, also called iron rim lesions (IRLs), display no contrast enhancement. The IRLs are characterized by an iron-laden rim of activated macrophages/microglia, indicating ongoing innate inflammation and tissue damage, and can be visualized as the paramagnetic rim on quantitative susceptibility mapping (QSM) (5). The chronic active lesions are associated with brain atrophy, aggressive tissue damage, disease progression, and disability and have been suggested as a potential therapeutic target (6-9). The chronic inactive and remyelinated lesions have the same characteristic of neither contrast enhancement nor paramagnetic rim, which can be classified into one category, called non-iron rim lesions (NIRLs). Thus, rapid and accurate identification of CELs, IRLs, and NIRLs is of great importance for predicting disease progression, judging prognosis, and developing or monitoring treatment.
Currently, conventional identification of CELs and IRLs is invasive and time-consuming. The former requires injecting the contrast agent, while the latter depends on the susceptibility-weighted sequence. Radiomics is an emerging technology and can extract massive quantitative imaging features such as intensity, shape, and texture from medical images. These extracted features can be converted to mineable high-dimensional data and thus offering deep information regarding the lesion’s microenvironment, which cannot be observed by clinicians with naked eyes (10). Radiomics is widely used in the diagnosis, differential diagnosis, prognosis, and prediction of cognitive impairment and disability progression of MS (11-14). However, to our knowledge, there is limited research using machine learning to establish lesion classification models in MS.
In this study, we investigated 2- and 3-class classification radiomics tasks based on T2-FLAIR images. The 2-class classification radiomics task was only performed between IRLs and NIRLs, as most MS patients were in chronic stage. CELs and IRLs are the focus of clinical practice and research, as well as being the important imaging biomarkers. Thus, we aimed to construct an effective and noninvasive model for discriminating between IRLs and NIRLs or among CELs, IRLs, and NIRLs. We present this article in accordance with the TRIPOD reporting checklist (available at https://qims.amegroups.com/article/view/10.21037/qims-23-1287/rc).
Methods
Subjects
Forty-five patients with relapsing-remitting MS (RRMS) were consecutively enrolled in this retrospective study from April 10, 2019 to December 24, 2022 in the First Affiliated Hospital of Chongqing Medical University. The inclusion criteria were as follow: (I) a confirmed diagnosis of RRMS according to the 2017 revised McDonald diagnostic criteria (15). (II) Absence of other neurodegenerative diseases and contraindications for MRI scans. (III) Free of corticosteroid treatment for at least three months before MRI examination. The study was conducted in accordance with the Declaration of Helsinki (as revised in 2013). This study was approved by the Institutional Review Board of the First Affiliated Hospital of Chongqing Medical University, and all patients provided written informed consent.
MRI data acquisition
All MRI exams were performed on the same 3T scanner (MAGNETOM Skyra, Siemens, Erlangen, Germany, 32-channel head coil). MRI sequences were acquired as follows: (I) sagittal 3-dimensional (3D) T1w magnetization-prepared rapid gradient echo (MPRAGE) before and after gadolinium [repetition time (TR) =2,300 ms, echo-time (TE) =2.26 ms, inversion time (TI) =900 ms, 192 slices, field of view (FOV) =256 mm, voxel size =1.0 mm × 1.0 mm × 1.0 mm], (II) sagittal 3D fluid-attenuated inversion recovery (FLAIR) (TR =5,000 ms, TE =388 ms, TI =1,800 ms, 192 slice, FOV =256 mm, voxel size =0.5 mm × 0.5 mm × 1 mm), (III) 3D-GRE imaging for QSM (TE1/TE2/TE3/TE4 =7.5 ms/15 ms/22.5 ms/30 ms, TR =36 ms, 64 slices, FOV =220 mm, voxel size = 0.5 mm × 0.5 mm × 2.0 mm), (IV) the 3D MPRAGE performed 10 minutes after gadolinium injection (0.1 mmol/kg).
Lesion segmentation and QSM processing
FLAIR and QSM images were registered to pre-contrast T1wMPRAGE images using statistical parametric mapping (SPM12; http://www.fil.ion.ucl.ac.uk/spm; MATLAB toolbox). WM lesions were manually outlined slice by slice on registered FLAIR images by one experienced radiologist (with 6 years’ experience) using open-source image analysis software (ITK-SNAP, version 3.8). Then the regions of interest (ROIs) were examined and corrected by one senior neuroradiologist (with 30 years’ experience). Finally, the classification of WM lesions performed by two experienced raters jointly and in case of disagreement, the senior radiologist would make the decision. The classification steps were: (I) identification of CELs on post-contrast T1wMPRAGE images, (II) distinction of IRLs and NIRLs based on QSM images for non-enhancement lesions. IRLs were defined as hyperintense ring-like signals or dots at the lesion edge, encircling it fully or partially, visible on at least two contiguous slices (16). To avoid partial-volume effects, only supratentorial lesions with a volume more than 64 voxels on T1 images were included further analysis. Furthermore, confluent lesions were excluded. The example of lesion segmentation is detailed in Figure S1.
QSM was reconstructed by sepia (SEPIA version 0.8.1.1) on the MATLAB platform (2018a, Mathworks), as previously described (17).
Radiomics feature extraction and selection
In our study, we utilized the pyradiomics package (18) of Python to extract high-dimensional features in the binary classification. Firstly, two experience radiologists were assigned to independently extract imaging radiomics features from the delineated ROIs, and the intraclass correlation coefficient (ICC) was calculated among the features. Only features with ICC ≥0.80 were retained. Next, Mann-Whitney U test was applied to identify features with significant differences (P<0.05). Lastly, least absolute shrinkage and selection operator (LASSO), reliefF, and mutual information (MI) methods were used to screen the features which exhibited significant differences after ICC and U test.
For the 3-class classification task, we employed the same methodology as mentioned above for feature extraction of CELs, IRLs, and NIRLs. The features selected after ICC test were subjected to analysis of variance (ANOVA). Finally, we utilized LASSO, reliefF, and MI methods respectively to identify the most significant features for model construction.
Radiomics classifier model construction
In this study, a random split of data in an 8:2 ratio was employed to create training and testing datasets. The training dataset was utilized to train random forest (RF), support vector machine (SVM), and eXtreme gradient boosting (XGBoost) classifiers respectively for constructing the imaging radiomics classifier model. To determine the optimal model combination, grid search and cross-validation methods were employed. The GridSearchCV class provided by Scikit-learn was utilized to iterate through different parameter combinations. Five-fold cross-validation performed on the training dataset to select the parameter combination that yielded the best model performance. The testing dataset was used to evaluate the performance of the model. Receiver operating characteristic (ROC) curves were used to assess the diagnostic performance of the imaging radiomics model.
Statistical analyses
All statistical analyses were performed with Python software (version 3.8.0). Continuous variables were expressed as mean value ± standard deviation or median with interquartile range as appropriate. Mann-Whitney U test and ANOVA were used to identify differential features among different lesion-groups. Scikit-learn module (version 1.2.0) in Python was utilized for feature selection and machine learning model construction. Additionally, the area under the curve (AUC), accuracy, sensitivity, specificity, and precision were also calculated to better evaluate the performance of models. For the 3-class classification, we took the Micro-average method for a comprehensive evaluation. P<0.05 was considered statistically significant.
Results
Demographic and radiomics features extraction
The study’s workflow is presented in Figure 1. A total of 45 patients with 875 WM lesions were included in this final analysis (92 CELs, 367 IRLs, and 416 NIRLs, respectively). There were 85 confluent lesions and 176 lesions of less than 64 voxels excluded. Figure 2 shows examples of three types of MS lesions. Demographic information is listed in Table 1.
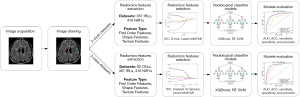
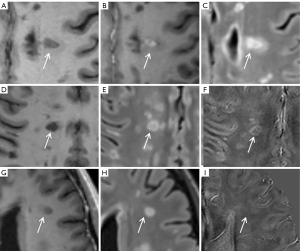
Table 1
Demographic | Test set (n=35) | Training set (n=10) | P |
---|---|---|---|
Age (years) | 27.3±7.1 | 28.9±9.5 | 0.565a |
Sex (male/female) | 5/30 | 1/9 | 0.725b |
Disease duration (years) | 2 (1.5) | 3 (2.25) | 0.097c |
Disease-modifying therapy | 10 | 4 | 0.491b |
EDSS scores | 0 (1) | 1 (1.125) | 0.059c |
P<0.05 considered statistically significant. Data were shown as mean ± standard deviation or median (interquartile range) or n. a, P obtained using independent sample t-tests; b, P obtained using the Chi-square test; c, P obtained using the Mann-Whitney U tests. EDSS, Expanded Disability Status Scale.
Radiomics feature selection
For the 2-class task, 783 ROIs were utilized (367 IRLs and 416 NIRLs) and 1,111 radiomics features were extracted for each ROI. After ICC test, only 854 features remained, which were further screened using Mann-Whitney U test, resulting in 140 features. For these 140 features, we applied three different methods (LASSO, reliefF, and MI) to select the important radiomics features. After the above steps, totally 7 (LASSO), 8 (reliefF), and 10 (MI) features were finally selected. These selected features are detailed in Table S1.
For the 3-class task, all the 875 ROIs, comprising 92 CELs, 367 IRLs, and 416 NIRLs, were utilized. Same as 2-class task, 930 features were remained after ICC test. Subsequently, ANOVA performed, resulting in 167 features. Finally, LASSO, reliefF, and MI were performed to screen the important features. After the above steps, we ultimately selected 7 (LASSO), 5 (reliefF), and 10 (MI) features for model construction and they are listed in Table S2. The feature coefficients are also shown in Figure 3.
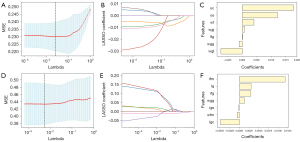
Comparison of radiological classifier models
The data in this study were segregated into training and testing sets through a random allocation process based on an 8:2 ratio. Both 2- and 3-class task had nine different combinations of three classifiers and three features selection algorithms. Table 2 present the representative performance metrics for nine models in 2-class task in the testing dataset. LASSO feature selection combined with RF model showed the best performance with the AUC of 0.893 (95% CI: 0.838–0.942), accuracy of 0.813, sensitivity of 0.833, specificity of 0.781, and precision of 0.851, followed by LASSO with XGBoost (AUC =0.884).
Table 2
Model | AUC (95% CI) | Accuracy | Sensitivity | Specificity | Precision |
---|---|---|---|---|---|
LASSO-XGBoost | 0.884 (0.828–0.932) | 0.825 | 0.865 | 0.766 | 0.847 |
ReliefF-XGBoost | 0.694 (0.606–0.776) | 0.646 | 0.641 | 0.652 | 0.720 |
MI-XGBoost | 0.803 (0.732–0.870) | 0.741 | 0.750 | 0.727 | 0.793 |
LASSO-RF | 0.893 (0.838–0.942) | 0.813 | 0.833 | 0.781 | 0.851 |
ReliefF-RF | 0.726 (0.643–0.805) | 0.690 | 0.696 | 0.682 | 0.753 |
MI-RF | 0.798 (0.732–0.862) | 0.741 | 0.794 | 0.667 | 0.768 |
LASSO-SVM | 0.733 (0.650–0.809) | 0.719 | 0.781 | 0.609 | 0.750 |
ReliefF-SVM | 0.749 (0.667–0.824) | 0.741 | 0.783 | 0.652 | 0.758 |
MI-SVM | 0.730 (0.646–0.800) | 0.652 | 0.761 | 0.561 | 0.707 |
AUC, area under the curve; CI, confidence interval; LASSO, least absolute shrinkage and selection operator; MI, mutual information; XGBoost, eXtreme gradient boosting; RF, random forest, SVM, support vector machine.
In the 3-class task, the testing set exhibited a range of AUC from 0.725 to 0.920. Our investigation revealed that LASSO features selection with XGBoost classifier displayed the highest classification performance with the AUC of 0.920 (95% CI: 0.887–0.950), accuracy of 0.796, sensitivity of 0.839, specificity of 0.881, and precision of 0.846, followed by LASSO with RF (AUC =0.890). Results of other models in 3-class task are presented in Table 3. The ROC curves for 2- and 3-class task are shown in Figure 4. For a comprehensive assessment of the model, we conducted the calibration curves and display them in Figures S2-S4.
Table 3
Model | AUC (95% CI) | Accuracy | Sensitivity | Specificity | Precision |
---|---|---|---|---|---|
LASSO-XGBoost | 0.920 (0.887–0.950) | 0.796 | 0.839 | 0.881 | 0.846 |
ReliefF-XGBoost | 0.725 (0.655–0.793) | 0.631 | 0.572 | 0.786 | 0.617 |
MI-XGBoost | 0.883 (0.843–0.922) | 0.767 | 0.722 | 0.860 | 0.812 |
LASSO-RF | 0.890 (0.848–0.926) | 0.739 | 0.720 | 0.843 | 0.809 |
ReliefF-RF | 0.725 (0.652–0.800) | 0.648 | 0.582 | 0.791 | 0.657 |
MI-RF | 0.865 (0.819–0.906) | 0.699 | 0.616 | 0.820 | 0.754 |
LASSO-SVM | 0.811 (0.760–0.854) | 0.636 | 0.555 | 0.764 | 0.744 |
ReliefF-SVM | 0.743 (0.680–0.806) | 0.625 | 0.550 | 0.780 | 0.645 |
MI-SVM | 0.776 (0.708–0.833) | 0.619 | 0.540 | 0.769 | 0.668 |
AUC, area under the curve; CI, confidence interval; LASSO, least absolute shrinkage and selection operator; MI, mutual information; XGBoost, eXtreme gradient boosting; RF, random forest; SVM, support vector machine.
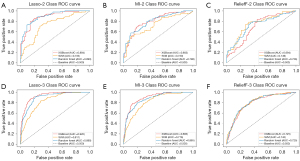
Discussion
In this study, we tried to explore the feasibility of applying the radiomics-based model to classify CELs, IRLs, and NIRLs in RRMS. Three selection methods (LASSO, reliefF, and MI) and three classifier models (XGBoost, RF, and SVM) were chosen and their performance was evaluated, as choosing the correct algorithm can improve the reliability and robustness of models. Our study provides a potential method for rapid and non-invasive identification of different MS lesions. Several studies reported that texture analysis based on conventional MRI or susceptibility-weighted imaging (SWI) showed promising results in distinguishing between acute and chronic lesions (11,19). However, to our knowledge, the 3-class radiomics classification model for MS lesions was less considered. In contrast to previous studies, we built a radiomics model for differential diagnosis of IRLs and NIRLs or between CELs, IRLs, and NIRLs, and achieved reliable performance.
As mentioned above, the breakdown of BBB represents the radiological marker of acute inflammatory and disease activity in MS. Due to the transient opening of BBB, the CELs usually exist for 3 weeks and converted to chronic MS lesions (20). Given that most MS patients are in the chronic phase, we only investigated the IRLs and NIRLs for the 2-class classification task. Among the nine models, LASSO with RF reached the best performance with an AUC of 0.893. Our results showed that the radiomics model could distinguish IRLs from NIRLs based on FLAIR images. IRLs have been previously described as inactive centers and characterized by a rim of activated microglia/macrophages (8,21). However, NIRLs contain two types of MS lesions (chronic inactive and remyelinated), and neither of them display inflammation cells and they are accompanied by different degrees of remyelination (22). Consequently, FLAIR image characteristics may modify during the evolution from IRLs to NIRLs, while these changes cannot be found by naked eyes. Our previous research has proven that the radiomics model based on FLAIR images can predict MS lesion evolution (23). Thus, our results may provide a new method of quickly and accurately identifying IRLs for clinical practice.
The LASSO with XGBoost model outperformed other models showing satisfactory performance for 3-class classification. For RRMS patients, relapsing is the most common clinical case (24), and assessing the activity of MS lesions is particularly important for judging prognosis and treatment efficacy. Therefore, a 3-class classification was developed and our results supported the potential application of the radiomics model based on FLAIR images to identify CELs, IRLs, and NIRLs respectively. The CELs have been accepted as a surrogate marker of BBB breakdown and many research has shown that acute inflammatory cell infiltration within CELs would lead to acute axonal transection and oligodendrocyte loss (25,26). Furthermore, different degrees of edema on FLAIR images can be observed in part of CELs, which is related to inflammatory mediators such as cytokines, chemokines, and other reactive oxygen species (26,27). However, IRLs have been proven to come with inflammatory cells that mainly locate at the paramagnetic rim. The reasons above may partially explain the feasibility of this experiment and the results of the 3-class classification model once again demonstrated that radiomics models based on FLAIR images can identify in depth information of different types of lesions, thereby achieving rapid and accurate differential diagnosis.
In the current study, we used three feature selection methods and three classifier models and combined them in pairs to explore the best model. Interestingly, both 2- and 3-class classification models revealed that LASSO is the most suitable features selection algorithm. In radiomics researches, high-dimensional feature vectors can lead to feature redundancy, which may diminish the diagnostic performance of predictive models. However, by adding the H penalty term of the linear model coefficients to the loss function, LASSO method encourages coefficients associated with weakly correlated features to become zero. This approach effectively compresses and reduces the dimensionality of MRI-based radiomics features, allowing further selection of valuable image-based features for model construction. Due to the small sample size of this study, common machine learning methods were employed rather than deep learning methods such as convolutional neural networks. For the 2- and 3-class task, both LASSO with XGBoost or RF demonstrated excellent performance, and the difference between RF and XGBoost was slight. However, the SVM showed unsatisfactory results, no matter what feature selection method was used. Choosing the correct and appropriate algorithm can improve the reliability and robustness of models. Thus, this study compared the performance of different algorithm combinations and evaluated the diagnostic efficacy among models.
To our knowledge, it is the first time to apply a 3-class classification model of radiomics in MS lesions. Previous studies performed 3-class classification tasks to discriminate among benign, borderline, and malignant ovarian tumors and achieved good performance (28). Identification of CELs without gadolinium-based contrast agents is significant in clinical practice (29,30), and our study provides a potential method to achieve this goal.
Limitations
This study has some limitations. First, the number of RRMS included is limited and our model lacks validation from external data. Future studies will increase the sample size of CELs and add the multicenter data. Second, we chose the method of manually outlining MS lesions and one of the aims of this study was to apply the radiomics-based model to quickly classify different types of lesions. Manual lesion segmentation is time-consuming, but current lesion segmentation software mostly identifies and creates a mask for all WM lesions (31). If we want to analyze the individual lesion, we need to manually segment the lesion mask into individual ROIs. Therefore, we chose manual segmentation from the beginning for the advantage of high accuracy for ROIs.
Conclusions
On balance, the current study demonstrated that the radiomics model based on FLAIR images might be useful to provide complementary differential diagnosis information and new insight to effectively distinguish among CELs, IRLs, and NIRLs in MS. The excellent performance of radiomics models could contribute to minimizing the workload, better monitoring the disease, and reducing the use of contrast agents.
Acknowledgments
Funding: This study was supported by
Footnote
Reporting Checklist: The authors have completed the TRIPOD reporting checklist. Available at https://qims.amegroups.com/article/view/10.21037/qims-23-1287/rc
Conflicts of Interest: All authors have completed the ICMJE uniform disclosure form (available at https://qims.amegroups.com/article/view/10.21037/qims-23-1287/coif). The authors have no conflicts of interest to declare.
Ethical Statement: The authors are accountable for all aspects of the work in ensuring that questions related to the accuracy or integrity of any part of the work are appropriately investigated and resolved. The study was conducted in accordance with the Declaration of Helsinki (as revised in 2013). This study was approved by the Institutional Review Board of the First Affiliated Hospital of Chongqing Medical University, and all patients provided written informed consent.
Open Access Statement: This is an Open Access article distributed in accordance with the Creative Commons Attribution-NonCommercial-NoDerivs 4.0 International License (CC BY-NC-ND 4.0), which permits the non-commercial replication and distribution of the article with the strict proviso that no changes or edits are made and the original work is properly cited (including links to both the formal publication through the relevant DOI and the license). See: https://creativecommons.org/licenses/by-nc-nd/4.0/.
References
- Reich DS, Lucchinetti CF, Calabresi PA. Multiple Sclerosis. N Engl J Med 2018;378:169-80. [Crossref] [PubMed]
- Frischer JM, Weigand SD, Guo Y, Kale N, Parisi JE, Pirko I, Mandrekar J, Bramow S, Metz I, Brück W, Lassmann H, Lucchinetti CF. Clinical and pathological insights into the dynamic nature of the white matter multiple sclerosis plaque. Ann Neurol 2015;78:710-21. [Crossref] [PubMed]
- Filippi M, Rocca MA, Barkhof F, Brück W, Chen JT, Comi G, DeLuca G, De Stefano N, Erickson BJ, Evangelou N, Fazekas F, Geurts JJ, Lucchinetti C, Miller DH, Pelletier D, Popescu BF, Lassmann H. Attendees of the Correlation between Pathological and MRI findings in MS workshop. Association between pathological and MRI findings in multiple sclerosis. Lancet Neurol 2012;11:349-60. [Crossref] [PubMed]
- Lassmann H. The pathologic substrate of magnetic resonance alterations in multiple sclerosis. Neuroimaging Clin N Am 2008;18:563-76. ix. [Crossref] [PubMed]
- Bagnato F, Hametner S, Yao B, van Gelderen P, Merkle H, Cantor FK, Lassmann H, Duyn JH. Tracking iron in multiple sclerosis: a combined imaging and histopathological study at 7 Tesla. Brain 2011;134:3602-15. [Crossref] [PubMed]
- Absinta M, Sati P, Masuzzo F, Nair G, Sethi V, Kolb H, Ohayon J, Wu T, Cortese ICM, Reich DS. Association of Chronic Active Multiple Sclerosis Lesions With Disability In Vivo. JAMA Neurol 2019;76:1474-83. [Crossref] [PubMed]
- Dal-Bianco A, Grabner G, Kronnerwetter C, Weber M, Kornek B, Kasprian G, Berger T, Leutmezer F, Rommer PS, Trattnig S, Lassmann H, Hametner S. Long-term evolution of multiple sclerosis iron rim lesions in 7 T MRI. Brain 2021;144:833-47. [Crossref] [PubMed]
- Dal-Bianco A, Grabner G, Kronnerwetter C, Weber M, Höftberger R, Berger T, Auff E, Leutmezer F, Trattnig S, Lassmann H, Bagnato F, Hametner S. Slow expansion of multiple sclerosis iron rim lesions: pathology and 7 T magnetic resonance imaging. Acta Neuropathol 2017;133:25-42. [Crossref] [PubMed]
- Weber CE, Krämer J, Wittayer M, Gregori J, Randoll S, Weiler F, Heldmann S, Roßmanith C, Platten M, Gass A, Eisele P. Association of iron rim lesions with brain and cervical cord volume in relapsing multiple sclerosis. Eur Radiol 2022;32:2012-22. [Crossref] [PubMed]
- Gillies RJ, Kinahan PE, Hricak H. Radiomics: Images Are More than Pictures, They Are Data. Radiology 2016;278:563-77. [Crossref] [PubMed]
- Caruana G, Pessini LM, Cannella R, Salvaggio G, de Barros A, Salerno A, Auger C, Rovira À. Texture analysis in susceptibility-weighted imaging may be useful to differentiate acute from chronic multiple sclerosis lesions. Eur Radiol 2020;30:6348-56. [Crossref] [PubMed]
- Zurita M, Montalba C, Labbé T, Cruz JP, Dalboni da Rocha J, Tejos C, Ciampi E, Cárcamo C, Sitaram R, Uribe S. Characterization of relapsing-remitting multiple sclerosis patients using support vector machine classifications of functional and diffusion MRI data. Neuroimage Clin 2018;20:724-30. [Crossref] [PubMed]
- Marzi C, d'Ambrosio A, Diciotti S, Bisecco A, Altieri M, Filippi M, Rocca MA, Storelli L, Pantano P, Tommasin S, Cortese R, De Stefano N, Tedeschi G, Gallo A. INNI Network. Prediction of the information processing speed performance in multiple sclerosis using a machine learning approach in a large multicenter magnetic resonance imaging data set. Hum Brain Mapp 2023;44:186-202. [Crossref] [PubMed]
- Batista S, Zivadinov R, Hoogs M, Bergsland N, Heininen-Brown M, Dwyer MG, Weinstock-Guttman B, Benedict RH. Basal ganglia, thalamus and neocortical atrophy predicting slowed cognitive processing in multiple sclerosis. J Neurol 2012;259:139-46. [Crossref] [PubMed]
- Thompson AJ, Banwell BL, Barkhof F, Carroll WM, Coetzee T, Comi G, et al. Diagnosis of multiple sclerosis: 2017 revisions of the McDonald criteria. Lancet Neurol 2018;17:162-73. [Crossref] [PubMed]
- Clarke MA, Pareto D, Pessini-Ferreira L, Arrambide G, Alberich M, Crescenzo F, Cappelle S, Tintoré M, Sastre-Garriga J, Auger C, Montalban X, Evangelou N, Rovira À. Value of 3T Susceptibility-Weighted Imaging in the Diagnosis of Multiple Sclerosis. AJNR Am J Neuroradiol 2020;41:1001-8. [Crossref] [PubMed]
- Shi Z, Pan Y, Yan Z, Ding S, Hu H, Wei Y, Luo D, Xu Y, Zhu Q, Li Y. Microstructural alterations in different types of lesions and their perilesional white matter in relapsing-remitting multiple sclerosis based on diffusion kurtosis imaging. Mult Scler Relat Disord 2023;71:104572. [Crossref] [PubMed]
- van Griethuysen JJM, Fedorov A, Parmar C, Hosny A, Aucoin N, Narayan V, Beets-Tan RGH, Fillion-Robin JC, Pieper S, Aerts HJWL. Computational Radiomics System to Decode the Radiographic Phenotype. Cancer Res 2017;77:e104-7. [Crossref] [PubMed]
- Michoux N, Guillet A, Rommel D, Mazzamuto G, Sindic C, Duprez T. Texture Analysis of T2-Weighted MR Images to Assess Acute Inflammation in Brain MS Lesions. PLoS One 2015;10:e0145497. [Crossref] [PubMed]
- Maggi P, Macri SM, Gaitán MI, Leibovitch E, Wholer JE, Knight HL, Ellis M, Wu T, Silva AC, Massacesi L, Jacobson S, Westmoreland S, Reich DS. The formation of inflammatory demyelinated lesions in cerebral white matter. Ann Neurol 2014;76:594-608. [Crossref] [PubMed]
- Absinta M, Sati P, Schindler M, Leibovitch EC, Ohayon J, Wu T, Meani A, Filippi M, Jacobson S, Cortese IC, Reich DS. Persistent 7-tesla phase rim predicts poor outcome in new multiple sclerosis patient lesions. J Clin Invest 2016;126:2597-609. [Crossref] [PubMed]
- Rahmanzadeh R, Galbusera R, Lu PJ, Bahn E, Weigel M, Barakovic M, et al. A New Advanced MRI Biomarker for Remyelinated Lesions in Multiple Sclerosis. Ann Neurol 2022;92:486-502. [Crossref] [PubMed]
- Peng Y, Zheng Y, Tan Z, Liu J, Xiang Y, Liu H, Dai L, Xie Y, Wang J, Zeng C, Li Y. Prediction of unenhanced lesion evolution in multiple sclerosis using radiomics-based models: a machine learning approach. Mult Scler Relat Disord 2021;53:102989. [Crossref] [PubMed]
- Rovira A, León A. MR in the diagnosis and monitoring of multiple sclerosis: an overview. Eur J Radiol 2008;67:409-14. [Crossref] [PubMed]
- Prineas JW, Parratt JD. Oligodendrocytes and the early multiple sclerosis lesion. Ann Neurol 2012;72:18-31. [Crossref] [PubMed]
- Henderson AP, Barnett MH, Parratt JD, Prineas JW. Multiple sclerosis: distribution of inflammatory cells in newly forming lesions. Ann Neurol 2009;66:739-53. [Crossref] [PubMed]
- Barnett MH, Prineas JW. Relapsing and remitting multiple sclerosis: pathology of the newly forming lesion. Ann Neurol 2004;55:458-68. [Crossref] [PubMed]
- Song XL, Ren JL, Zhao D, Wang L, Ren H, Niu J. Radiomics derived from dynamic contrast-enhanced MRI pharmacokinetic protocol features: the value of precision diagnosis ovarian neoplasms. Eur Radiol 2021;31:368-78. [Crossref] [PubMed]
- Choi JW, Moon WJ. Gadolinium Deposition in the Brain: Current Updates. Korean J Radiol 2019;20:134-47. [Crossref] [PubMed]
- Zhang B, Liang L, Chen W, Liang C, Zhang S. An Updated Study to Determine Association between Gadolinium-Based Contrast Agents and Nephrogenic Systemic Fibrosis. PLoS One 2015;10:e0129720. [Crossref] [PubMed]
- Schmidt P, Gaser C, Arsic M, Buck D, Förschler A, Berthele A, Hoshi M, Ilg R, Schmid VJ, Zimmer C, Hemmer B, Mühlau M. An automated tool for detection of FLAIR-hyperintense white-matter lesions in Multiple Sclerosis. Neuroimage 2012;59:3774-83. [Crossref] [PubMed]