Application of deep learning image reconstruction-high algorithm in one-stop coronary and carotid-cerebrovascular CT angiography with low radiation and contrast doses
Introduction
Atherosclerosis has been widely considered as one of the leading causes of mortality worldwide, involving multiple sites including coronary, cerebrovascular, and peripheral arteries. Evidence has been collected to show that coronary and cerebrovascular atherosclerosis often happen simultaneously, due to their similar underlying pathophysiology and shared cardiovascular risk factors (1-5). Co-existed coronary and cerebrovascular atherosclerosis have been proved to increase the risk of major vascular events compared to either coronary or cerebrovascular atherosclerosis alone. Stroke patients with asymptomatic coronary artery disease (CAD) have greater risks of future major vascular events than those without (6,7). Carotid atherosclerosis can be an independent predicting factor for major adverse cardiac events in CAD patients(8). Therefore, choosing appropriate image modality to identify patients with co-existing coronary and cerebral-carotid atherosclerosis possesses great clinical significance.
Computed tomography angiography (CTA) is the recommended tool for screening, diagnosing, and evaluating atherosclerosis diseases. Besides its non-invasive and time-saving properties, CTA is capable of precisely describing the anatomies of arteries and exhibits high sensitivity in the screening for CAD (9-11). Nevertheless, conventional CTA scan protocol was designed for separately imaging coronary or carotid-cerebrovascular arteries, resulting in an attendant increase in radiation and contrast agent doses. Repeated examination for different sites will also increase the treatment expense. Recently, one-stop coronary and carotid-cerebrovascular CTA (C&CC-CTA) protocol using iterative reconstruction (IR) algorithm has been introduced and provides a potential clinically feasible examination for patients suspected with co-existing atherosclerosis disease (12-17). However, previously published one-stop C&CC-CTA protocols still presented with slightly high image noise, and the radiation and contrast dose are expected to be further reduced.
The introduction of deep learning image reconstruction (DLIR) algorithm, incorporating a convolutional neural network, has brought new hope for decreasing image noise, and thus optimizing image quality with a more balanced spatial resolution for CTA (18,19). DLIR provides three selectable strength levels (low, medium and high), and DLIR-high (DLIR-H) has been proved to gain the highest ability for reducing image noise while maintaining spatial resolution of images reasonably well (20-22). In our recent study, compared with an adaptive statistical iterative reconstruction-V (ASIR-V) based protocol, the combination of DLIR-H algorithm and low tube voltage for coronary CTA demonstrated superiority in achieving higher image quality as well as significantly lower radiation and contrast dose (23). Furthermore, our preliminary study revealed that under the same scanning condition, DLIR-H presented significantly better image quality than ASIR-V at a strength level of 50% (ASIR-V50%) for one-stop C&CC-CTA (Table S1). To our knowledge, the effect of DLIR-H on one-stop C&CC-CTA has not been investigated elsewhere.
The aim of this study was to validate the feasibility of one-stop C&CC-CTA using DLIR-H algorithm with 256-slice CT and explore its capacity in reducing radiation and contrast agent dose. We present this article in accordance with the STROBE reporting checklist (available at https://qims.amegroups.com/article/view/10.21037/qims-23-864/rc).
Methods
Study population
This prospective cohort study was conducted in accordance with the Declaration of Helsinki (as revised in 2013). The study was approved by the Ethics Committee of West China Hospital. All participants provided written informed consents.
From February 2018 to January 2019, adult patients, with body mass index (BMI) within 18–25 kg/m2, who were referred to both C&CC-CTA in West China Hospital were initially recruited for this study. Exclusion criteria were then applied including patients who (I) had a history of cardiac, neck or cephalic surgery, (II) suffered superior vena cava syndrome, or (III) had contraindications to contrast-enhanced CT examinations (e.g., renal insufficiency, allergic to contrast medium). The enrolled patients were randomly divided into the DLIR-H group and the ASIR-V50% group (Figure 1). Demographic data (age, gender), height, weight, heart rate, vascular risk factors and reasons for one-stop CTA were collected once the patient was included in the study.
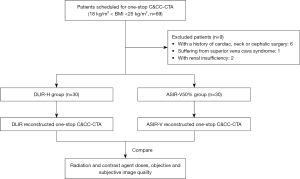
Image acquisition and reconstruction
All participants underwent CTA scanning with a 256-row CT scanner (Revolution CT, GE HealthCare, Milwaukee, USA) with their arms raised at the sides of the head. Patients in both groups underwent the non-contrast CT first. After the contrast injection, all patients underwent a coronary CTA with prospective electrocardiogram gating, followed by carotid-cerebrovascular scanning after a delay of 1.5 s. Following published articles, the coronary CTA scanning used 80 kVp and smart mA for noise index (NI) of 36 for the DLIR-H group, and 100 kVp and smart mA for NI of 25 for the ASIR-V50% group (23-26). In both groups, the coronary CTA was acquired within one heartbeat triggered using the automatic gating technique, with the best systolic and diastolic phases recommended by the device and snapshot freeze cardiac motion correction technique activated: for patients with a heart rate (HR) <66 bpm, images were collected at the 70–80% of the R-R cardiac interval. For patients with a HR between 66 and 85 bpm, images were collected at both 40–55% and 70–80% of the R-R interval. As for those with a HR >85 bpm, images were collected at the 40–60% of the R-R interval. The carotid-cerebrovascular image acquisition parameters were set to be 80 kVp and 500 mA for the DLIR-H group, and 100 kVp and 450 mA for the ASIR-V50% group.
The non-ionized contrast agent (Iopamidol, concentration 370 mgI/mL) was intravenously injected through the antecubital vein using a double-syringe power injector. A reduced weight-dependent contrast dose rate of 24 mgI/kg/s was set for the DLIR-H group, while a conventional weight-dependent contrast dose rate of 32 mgI/kg/s was set for the ASIR-V50% group. The injection was finished in 10 s for each participant. Following contrast injection, 30 mL of saline was injected with a same injection rate for both groups. Bolus-tracking technique was applicated to control the contrast agent injection timing, with a monitoring region of interest (ROI) placed in the root of ascending aorta for triggering at the threshold of 200 HU. The coronary CTA started with a delay time of 3.1 s after triggering.
All images were reconstructed with a standard kernel at 0.625 mm section thickness. DLIR-H algorithm and ASIR-V50% algorithm were used for image reconstruction for the DLIR-H group and ASIR-V50% group, respectively. The axial images were transferred to an advanced workstation (AW4.7, GE Healthcare) for the 3D post-processing reconstruction to generate maximum intensity projection, curved planar reformat, and volume rendering images for the subjective evaluation of image quality.
Image quality analysis
The coronary arteries were divided into 18 segments according to the American Heart Association standard for analysis (27). Segments with coronary artery diameter ≥1.5 mm were evaluated. The carotid and cerebral arteries were divided into 19 segments according to previous studies (28). Segments with carotid and cerebral artery diameter ≥2 mm were evaluated. Image quality was analyzed by two independent radiologists (both with medical degree with 6 and 15 years of imaging experience). A 5-point grading system was used to grade the vessel enhancement, image noise and artifact: (I) score 1 for poor vessel enhancement, inadequate delineation between the vessel and its surrounding tissue, severe image noise and artifact; (II) score 2 for suboptimal vessel enhancement and blurring of vessel margin, substantial image noise and obvious artifact; (III) score 3 for acceptable vessel enhancement, moderate blurring of vessel margin, and moderate image noise and artifact; (IV) score 4 for good vessel enhancement, slightly blurring of vessel margin and mild image noise and artifact; (V) score 5 for excellent vessel enhancement, clear vessel margin, very low image noise and negligible artifact.
For the objective analysis of coronary artery images, three ROIs were placed at the origin of right coronary artery (RCA), left anterior descending artery (LAD) and left circumflex artery (LCX) to measure the mean CT value (CTvessel) as the attenuation of vessels and standard deviation (SDvessel). Another ROI was placed on the fat of adjacent chest wall to measure the CT attenuation value (CTfat) and standard deviation (SDfat) of fat. For carotid-cerebrovascular images, CTvessel and SDvessel were measured by placing three ROIs at the median thyroid level for common carotid artery (CCA), internal carotid artery (ICA) and middle cerebral artery (MCA). Then background ROIs were drawn at the lateral muscles at the corresponding level to acquire attenuation (CTmuscle) and standard deviation (SDmuscle) of muscles. Signal-to-noise ratio (SNR) for vessels was calculated as CTvessel/SDvessel, and contrast-to-noise ratio (CNR) was defined as (CTvessel − CTmuscle) / SDmuscle.
Radiation dose and contrast agent
Volume CT dose index (CTDIvol) and dose length product (DLP) for each patient were recorded. Effective dose (ED) was calculated according to the following formula: ED = DLP × k. The k values vary along with the regions of the scanned body (for coronary imaging, k=0.014 mSv·mGy−1·cm−1; for carotid-cerebrovascular imaging, k=0.0040 mSv·mGy−1·cm−1) (29,30). Contrast agent volume and flow rate were also recorded by the operators.
Statistical analysis
All statistical analyses were performed with the R program (v. 4.1.0, R Foundation for Statistical Computing; https://www.r-project.org/). The sample size was defined by referring to a previous study performed at our institution, with similar research design, methodology and hypotheses (23). Nevertheless, a dedicated power analysis to determine the sample size was not performed. Continuous data were expressed as mean ± SD. Data distribution normality was tested using the Shapiro-Wilk test. The independent two-tailed t-test and Mann-Whitney U-test were employed to compare quantitative variables. Categorical data were expressed as frequency and the corresponding percentage. Chi-squared test was employed to make comparison of categorical data. Kappa statistics were used to compare interobserver agreement (kappa value over 0.7 was defined as excellent agreement, 0.4–0.7 as good agreement, and less than 0.4 as bad agreement) (23). A P value below 0.05 was considered to indicate statistical significance.
Results
Patient characteristics
During the study period, 69 patients were eligible for inclusion. Nine patients were excluded for the following reasons: with a history of cardiac, neck or cephalic surgery (n=6), suffering from superior vena cava syndrome (n=1), with renal insufficiency (n=2). The remaining 60 patients were randomly divided into the DLIR-H group (n=30, male/female, 23/7) and the ASIR-V50% group (n=30, male/female, 25/5). A flowchart briefly describing the patient selection is shown in Figure 1.
Characteristics of the participants are summarized in Table 1. There were 15 cases of stroke, 6 cases of transient ischemic attack (TIA), 7 cases of CAD and 2 cases of abdominal aortic aneurysm (AAA) in the DLIR-H group, while 11 cases of stroke, 7 cases of TIA, 9 cases of CAD and 3 cases of AAA in the ASIR-V50% group (all P>0.05). No statistically significant difference was observed in sex, age, BMI, HR, and vascular risk factors between the two groups.
Table 1
Characteristic | DLIR-H group (n=30) | ASIR-V50% group (n=30) | t/χ2 | P |
---|---|---|---|---|
Age, years | 56.77±13.31 | 54.77±12.41 | 0.60 | 0.55 |
Gender, female/male | 7/23 | 5/25 | 0.42 | 0.52 |
Basic clinical and CT data | ||||
BMI, kg/m2 | 21.96±1.99 | 22.51±2.01 | −1.06 | 0.29 |
Heart rate, bpm | 72.87±13.46 | 73.97±12.43 | −0.33 | 0.74 |
Arrythmia | 4 (13.33) | 3 (10.00) | 0.16 | 0.69 |
Vascular risk factor | ||||
Hypertension | 19 (63.33) | 17 (56.67) | 0.28 | 0.60 |
Dyslipidemia | 13 (43.44) | 11 (36.67) | 0.28 | 0.60 |
Type II diabetes | 9 (30.00) | 7 (23.33) | 0.34 | 0.56 |
Family history of CAD | 13 (43.44) | 15 (50.00) | 0.27 | 0.61 |
Smoking | 12 (40.00) | 15 (50.00) | 0.61 | 0.44 |
Reasons referred to one-stop CTA | ||||
Stroke | 15 (50.00) | 11 (36.67) | 1.09 | 0.30 |
TIA | 6 (20.00) | 7 (23.33) | 0.10 | 0.75 |
CAD | 7 (23.33) | 9 (30.00) | 0.34 | 0.56 |
AAA | 2 (6.67) | 3 (10.00) | 0.22 | 0.64 |
Parametric continuous data are expressed as means ± standard deviation with t value. Category data are expressed as numbers with percentages in parentheses, with χ2. DLIR-H, deep learning image reconstruction-high; ASIR-V50%, 50% adaptive statistical iterative reconstruction-V; BMI, body mass index; bpm, beat per minute; CAD, coronary artery disease; CTA, computed tomography angiography; TIA, transient ischemic attack; AAA, abdominal aortic aneurysm.
Radiation dose and contrast agent
The overall CTDIvol and DLP values for the DLIR-H group were statistically lower than that for the ASIR-V50% group (5.55±0.39 vs. 10.29±1.75 mGy in CTDIvol, P<0.001 and 158.26±10.26 vs. 293.97±34.14 mGy·cm in DLP, P<0.001). The ED was 1.00±0.09 mSv for the DLIR-H Group, and 48% lower than the 1.91±0.42 mSv for the ASIR-V50% Group (P<0.001). Furthermore, when considering the radiation dose of separate coronary or cerebrovascular scanning, the DLP and ED of DLIR-H group were all significantly lower than those of ASIR-V50% group (C-CTA: 36.27±6.05 vs. 73.25±29.21 mGy·cm in DLP, P<0.001 and 0.51±0.08 vs. 1.03±0.41 mSv in ED, P<0.001; CC-CTA: 121.99±8.03 vs. 220.72±15.21 mGy·cm in DLP, P<0.001 and 0.49±0.03 vs. 0.88±0.06 mSv in ED, P<0.001). The contrast agent volume was 35.80±4.85 mL for the DLIR-H group, 30% lower than the 51.13±6.91 mL for the ASIR-V50% group (P<0.001). The contrast flow rate of the DLIR-H group was also significantly lower than that of the ASIR-V50% group (3.58±0.49 vs. 5.09±0.65 mL/s, P<0.001). The CTDIvol, DLP, and ED values for C-CTA and CC-CTA are listed separately in Table 2.
Table 2
Parameter | DLIR-H group (n=30) | ASIR-V50% group (n=30) | t value | P |
---|---|---|---|---|
Radiation dose | ||||
Coronary CTA | ||||
CTDIvol, mGy | 2.49±0.39 | 4.88±1.75 | −7.32 | <0.001 |
DLP, mGy·cm | 36.27±6.05 | 73.25±29.21 | −6.79 | <0.001 |
ED, mSv | 0.51±0.08 | 1.03±0.41 | −6.77 | <0.001 |
Carotid-cerebrovascular CTA | ||||
CTDIvol, mGy | 3.06 | 5.41 | NA | NA |
DLP, mGy·cm | 121.99±8.03 | 220.72±15.21 | −31.43 | <0.001 |
ED, mSv | 0.49±0.03 | 0.88±0.06 | −31.44 | <0.001 |
Overall | ||||
CTDIvol, mGy | 5.55±0.39 | 10.29±1.75 | −14.51 | <0.001 |
DLP, mGy·cm | 158.26±10.26 | 293.97±34.14 | −20.85 | <0.001 |
ED, mSv | 1.00±0.09 | 1.91±0.42 | −11.61 | <0.001 |
Contrast agent dose | ||||
Contrast doses, mL | 35.80±4.85 | 51.13±6.91 | −9.94 | <0.001 |
Contrast flow rate, mL/s | 3.58±0.49 | 5.09±0.65 | −10.18 | <0.001 |
Parametric continuous data are expressed as means ± standard deviation with t value. DLIR-H, deep learning image reconstruction-high; ASIR-V50%, 50% adaptive statistical iterative reconstruction-V; CTA, computed tomography angiography; CTDIvol, volume CT dose index; DLP, dose length product; ED, effective dose; NA, not applicable.
Image quality
Subjective analysis
Coronary artery
The distribution of the subjective quality scores is listed in Table 3. All scores for both groups exceeded 3, meeting the diagnostic requirement. The mean score was slightly higher in the DLIR-H group compared with that in the ASIR-V50% group (4.38±0.67 vs. 4.17±0.81); however, the difference was not statistically significant (P=0.337). The kappa analysis indicated a good consistency in image quality evaluation between the two readers with kappa value of 0.656.
Table 3
Quality score | DLIR-H group (n=30) | ASIR-V50% group (n=30) | |||
---|---|---|---|---|---|
Reader 1 | Reader2 | Reader 1 | Reader 2 | ||
Coronary CTA | |||||
1 | 0 | 0 | 0 | 0 | |
2 | 0 | 0 | 0 | 0 | |
3 | 3 | 4 | 8 | 9 | |
4 | 12 | 11 | 8 | 8 | |
5 | 15 | 15 | 14 | 13 | |
Carotid-cerebrovascular CTA | |||||
1 | 0 | 0 | 0 | 0 | |
2 | 0 | 0 | 0 | 0 | |
3 | 9 | 9 | 8 | 9 | |
4 | 6 | 7 | 11 | 10 | |
5 | 15 | 14 | 11 | 11 |
DLIR-H, deep learning image reconstruction-high; ASIR-V50%, 50% adaptive statistical iterative reconstruction-V; CTA, computed tomography angiography.
Carotid and cerebral artery
The distribution of subjective quality scores for carotid and cerebral arteries is also listed in Table 3. Diagnostic requirement was validated in both groups as all scores exceeded 3. There was no statistically significant difference in mean score between the DLIR-H group and ASIR-V50% group (4.18±0.87 vs. 4.08±0.79, P=0.604). The kappa analysis indicated a good consistency in image quality evaluation between the two readers with kappa value of 0.796. Figure 2 reveals an example of the good image quality using the DLIR-H scan protocol for normal patients. Figure 3 exhibits the capacity of the DLIR-H scan protocol in detecting mixed and calcified plaques of coronary and cerebral arteries.
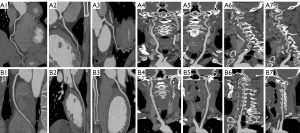
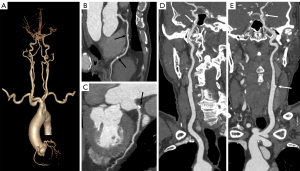
Objective analysis
Coronary artery
At all three branches assessed, there was no statistical difference in CT attenuation value between the DLIR-H and ASIR-V50% group (all P>0.05). Nevertheless, image noise in the DLIR-H group was significantly reduced compared with that of ASIR-V50% group (P<0.001), resulted in statistically higher CNR for all vessels in the DLIR-H group compared to the ASIR-V50% group (details in Table 4). Besides, in the DLIR-H group, SNR of RCA and LCX were significantly higher, compared with the ASIR-V50% group (P=0.001, and P=0.032). And SNR of LAD was slightly higher in the DLIR-H group, while the difference was not statistically significant (P=0.301) (Table 4 and Figure 4).
Table 4
Parameter | DLIR-H group (n=30) | ASIR-V50% group (n=30) | P value |
---|---|---|---|
Attenuation, HU | |||
RCA | 464.47±77.48 | 438.77±72.39 | 0.190 |
LAD | 455.87±68.67 | 444.27±71.49 | 0.524 |
LCX | 439.53±80.29 | 419.37±75.19 | 0.320 |
SDvessel, HU | |||
RCA | 25.40±3.57* | 28.90±3.48 | <0.001 |
LAD | 28.64±4.15 | 30.18±5.55 | 0.228 |
LCX | 29.29±5.24* | 31.97±4.85 | 0.044 |
SNR | |||
RCA | 18.66±4.31* | 15.37±2.96 | 0.001 |
LAD | 16.26±3.44 | 15.26±3.98 | 0.301 |
LCX | 15.69±4.95* | 13.37±2.91 | 0.032 |
CNR | |||
RCA | 31.51±4.93* | 25.21±8.40 | <0.001 |
LAD | 30.49±5.57* | 25.43±8.08 | 0.007 |
LCX | 29.36±6.31* | 24.29±6.72 | 0.004 |
Noise, HU | 18.93±1.43* | 22.86±3.75 | <0.001 |
Parametric continuous data are expressed as mean ± standard deviation. *, significant difference from AIR-V50% group. DLIR-H, deep learning image reconstruction-high; ASIR-V50%, 50% adaptive statistical iterative reconstruction-V; RCA, right coronary artery; LAD, left anterior descending artery; LCX, left circumflex artery; SDvessel, standard deviation of CT value measured at vessel; SNR, signal-to-noise ratio; CNR, contrast-to-noise ratio.
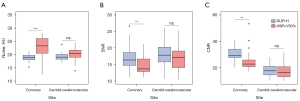
Carotid and cerebral vascular
The CT attenuation value, SNR and CNR of all arteries comparable within the two groups (all P>0.05). The DLIR-H group possessed significantly lower noise for the CCA level than the ASIR-V50% group (20.49±2.88 vs. 23.06±3.90 HU, P=0.005), while for the ICA level, MCA level, and the average of the three levels, no statistical differences were reached between the two groups (all P>0.05) (Table 5 and Figure 4).
Table 5
Parameter | DLIR-H group (n=30) | ASIR-V50% group (n=30) | P value |
---|---|---|---|
Attenuation, HU | |||
CCA | 418.20±83.98 | 415.92±105.24 | 0.926 |
ICA | 418.48±89.22 | 420.25±106.16 | 0.945 |
MCA | 371.25±91.11 | 386.38±104.80 | 0.553 |
SDvessel, HU | |||
CCA | 23.38±4.58 | 24.02±3.50 | 0.545 |
ICA | 20.93±2.76* | 23.93±4.28 | 0.002 |
MCA | 24.88±4.37 | 25.98±4.86 | 0.362 |
SNR | |||
CCA | 18.71±5.75 | 17.55±4.95 | 0.403 |
ICA | 20.30±4.89 | 18.01±5.09 | 0.082 |
MCA | 15.29±4.44 | 15.28±4.72 | 0.995 |
CNR | |||
CCA | 17.31±4.79 | 15.82±5.34 | 0.260 |
ICA | 19.53±5.59 | 19.58±6.31 | 0.973 |
MCA | 16.06±5.27 | 17.12±6.45 | 0.489 |
Noise, HU | |||
CCA level | 20.49±2.88* | 23.06±3.90 | 0.005 |
ICA level | 17.96±1.35 | 18.56±3.40 | 0.380 |
MCA level | 19.30±3.04 | 19.07±3.09 | 0.769 |
Average | 19.25±1.42 | 20.23±2.40 | 0.061 |
Parametric continuous data are expressed as mean ± standard deviation. *, significant difference from AIR-V50% group. CTA, computed tomography angiography; DLIR-H, deep learning image reconstruction-high; ASIR-V50%, 50% adaptive statistical iterative reconstruction-V; CCA, common carotid artery; ICA, internal carotid artery; MCA, middle cerebral artery; SDvessel, standard deviation of CT value measured at vessel; SNR, signal-to-noise ratio; CNR, contrast-to-noise ratio.
Discussion
Our low-contrast and radiation dose one-stop C&CC-CTA protocol with DLIR-H achieved at least non-inferior image quality but significantly reduced contrast and radiation doses compared to the IR-based routine-dose one-stop protocol. Image quality improvement was demonstrated predominantly in coronary arteries, where the vessel enhancement was significantly improved, and image noise was reduced by 17.2% to 18.93 HU. As for the carotid and cerebrovascular section, our protocol exhibited comparable image quality compared with the routine-dose ASIR-V50% group. The DLIR-H algorithm again showed stable ability in reducing image noise and retaining image quality at low radiation and contrast doses. Clinical application of DLIR-H algorithm might achieve contrast and radiation “double” saving CTA protocol. Therefore, further studies on validating its impact on clinical diagnosis work-up are warranted.
DLIR has been applied to a wide range of CTA protocols like chest, abdominal and vascular diseases, exhibiting 13.6–37.6% reduction in image noise (19,31-33). Our study utilized DLIR-H algorithm to reconstruct one-stop CTA images for the first time and demonstrated its superiority again in preserving image quality under low radiation and contrast dose settings. By contrast, previously proposed one-stop CTA protocols were mainly based on IR algorithm and reported evidently higher image noise of 24.2–29.0 HU at the aorta root (15-17). We believe the higher noise in these studies was because under low radiation settings, IR would result in substantial image noise and affect the display of subtle imaging features (34,35). Considering all one-stop CTA protocols designed for simplifying the scanning process, with the double-low protocol we presented in our study, stroke patients would benefit from our protocol for more precise coronary atherosclerosis assessment. CAD patients could also receive screening for carotid-cerebrovascular stenosis at the same time as the coronary CTA examination. However, no guideline has clarified the indication for one-stop C&CC-CTA. Therefore, further studies are in need to determine the target population for this test.
The other advantage of our protocol was that we drastically reduced the total effective radiation dose to about 1.00 mSv. As the scanning area of one-stop CTA involves radiation-sensitive organs like thyroid gland and crystalline lens, reducing the radiation dose as much as possible is of essence to avoid the radiation-associated adverse events. With the application of DLIR algorithm, we performed CTA using the 80 kVp tube voltage, equal to the minimum tube voltage reported in the previous one-stop CTA studies reported by Zhao et al. (14). However, in their study, a further reduction of ED to 0.32±0.11 mSv was reported. They utilized the high-pitch scanning of dual-source CT (DSCT), with rapid gantry rotation and table-moving speed, to decrease radiation dose while preserving high temporal resolution. However, coronary artery visualization by DSCT might be impaired with motion artifact when heart rate exceeds a certain level (36), as in the study by Zhao et al., only patients with an HR below 70 bpm were included. In our study, the mean HR was 72.87±13.46 bpm and 4 arrythmia patients were enrolled in the DLIR-H group, indicating our protocol can be applied to more patients and may be more suitable in clinical application.
Another advantage of our protocol was the significantly improved coronary artery image quality, both in objective and subjective evaluation. Although validation test in diagnostic accuracy was not performed in our study, with evidence from Benz, D. C.’s study indicating a non-inferior/superior diagnostic accuracy in coronary diseases with CTA images using DLIR (37), we believe the diagnostic accuracy for coronary artery using our C&CC-CTA protocol should not be inferior to that of using conventional protocol. Nevertheless, for carotid and cerebrovascular vessels, we have to admit that the image quality was not significantly improved using DLIR-H under the lower-dose conditions. Similarly, all the former one-stop C&CC-CTA protocols reported comparable or even worse image quality at the same site (12,14,15). We speculate that it might be attributed to the order of scanning, as the carotid and cerebrovascular arteries were always scanned behind coronary arteries in the one-stop scanning process. Till now, only a few studies have been done to explore the application CTA images of DLIR in carotid and cerebrovascular vessel under lower radiation and contrast dose settings. Also, these studies could not reach a further decrease in image noise compared with the standard-dose protocol with ASIR-V algorithm (20,38). Even if a mean subjective score of 4.18 in our study proved that our carotid and cerebrovascular images were able to meet the diagnostic criteria, further studies to validate its impact on diagnostic accuracy are warranted to confirm its efficacy.
Limitations
Even the results of this study were promising, several limitations should be noted. First, as a single center study, all individuals enrolled were routine patients referred to a simultaneously coronary and carotid artery CTA. The scale was limited, and multicenter studies are in need to validate this result. Second, our study evaluated the feasibility of our CTA protocol with vessel enhancement and image noise. Even though image quality which is closely related to diagnosis was rigorously tested in our study, at this stage, our results should not be expanded to diagnostic accuracy. Third, our study was limited to a cohort of BMI <25 kg/m2, restricting its applicability in obese patients. Finally, the scanning parameters of our protocol were selected based on previous research and our preliminary experiment results, and may not be most suitable for DLIR-H. Further evaluation is needed to assess the optimal settings for DLIR-H one-stop C&CC CTA.
Conclusions
In conclusion, we evaluated the image quality of a one-stop C&CC-CTA under reduced radiation dose and contrast dose using DLIR-H. With this convolutional network-based reconstruction algorithm, we managed to generate superior image quality for the coronary arteries and similar image quality for the carotid and cerebral arteries while achieving 48% and 30% reduction in radiation and contrast dose, respectively compared with the routine-dose protocol with ASIR-V50%. We also eliminated the restriction for heart rate in recent-proposed one-stop CTA protocols.
Acknowledgments
Funding: This work was a project supported by
Footnote
Reporting Checklist: The authors have completed the STROBE reporting checklist. Available at https://qims.amegroups.com/article/view/10.21037/qims-23-864/rc
Conflicts of Interest: All authors have completed the ICMJE uniform disclosure form (available at https://qims.amegroups.com/article/view/10.21037/qims-23-864/coif). The authors have no conflicts of interest to declare.
Ethical Statement: The authors are accountable for all aspects of the work in ensuring that questions related to the accuracy or integrity of any part of the work are appropriately investigated and resolved. The study was conducted in accordance with the Declaration of Helsinki (as revised in 2013). The study was approved by the Ethics Committee of West China Hospital. All participants provided written informed consents.
Open Access Statement: This is an Open Access article distributed in accordance with the Creative Commons Attribution-NonCommercial-NoDerivs 4.0 International License (CC BY-NC-ND 4.0), which permits the non-commercial replication and distribution of the article with the strict proviso that no changes or edits are made and the original work is properly cited (including links to both the formal publication through the relevant DOI and the license). See: https://creativecommons.org/licenses/by-nc-nd/4.0/.
References
- Pan Y, Jing J, Cai X, et al. Prevalence and Vascular Distribution of Multiterritorial Atherosclerosis Among Community-Dwelling Adults in Southeast China. JAMA Netw Open 2022;5:e2218307. [Crossref] [PubMed]
- Sigala F, Oikonomou E, Antonopoulos AS, et al. Coronary versus carotid artery plaques. Similarities and differences regarding biomarkers morphology and progno-sis. Curr Opin Pharmacol 2018;39:9-18. [Crossref] [PubMed]
- Molnár S, Kerényi L, Ritter MA, et al. Correlations between the atherosclerotic changes of femoral, carotid and coronary arteries: a post mortem study. J Neurol Sci 2009;287:241-5. [Crossref] [PubMed]
- Odink AE, van der Lugt A, Hofman A, et al. Association between calcification in the coronary arteries, aortic arch and carotid arteries: the Rotterdam study. Atheroscle-rosis 2007;193:408-13. [Crossref] [PubMed]
- Li AH, Chu YT, Yang LH, et al. More coronary artery stenosis, more cerebral artery stenosis? A simultaneous angiographic study discloses their strong correlation. Heart Vessels 2007;22:297-302. [Crossref] [PubMed]
- Yoo J, Song D, Baek JH, et al. Poor long-term outcomes in stroke patients with asymptomatic coronary artery disease in heart CT. Atherosclerosis 2017;265:7-13. [Crossref] [PubMed]
- Roh JW, Kwon BJ, Ihm SH, et al. Predictors of Significant Coronary Artery Disease in Patients with Cerebral Artery Atherosclerosis. Cerebrovasc Dis 2019;48:226-35. [Crossref] [PubMed]
- Steinvil A, Sadeh B, Bornstein NM, et al. Impact of carotid atherosclerosis on the risk of adverse cardiac events in patients with and without coronary disease. Stroke 2014;45:2311-7. [Crossref] [PubMed]
- Serruys PW, Hara H, Garg S, et al. Coronary Computed Tomographic Angiography for Complete Assessment of Coronary Artery Disease: JACC State-of-the-Art Re-view. J Am Coll Cardiol 2021;78:713-36. [Crossref] [PubMed]
- Cho I, Shim J, Chang HJ, et al. Prognostic value of multidetector coronary computed tomography angiography in relation to exercise electrocardiogram in patients with suspected coronary artery disease. J Am Coll Cardiol 2012;60:2205-15. [Crossref] [PubMed]
- Chao SP, Law WY, Kuo CJ, et al. The diagnostic accuracy of 256-row computed tomographic angiography compared with invasive coronary angiography in patients with suspected coronary artery disease. Eur Heart J 2010;31:1916-23. [Crossref] [PubMed]
- Liu J, Wang C, Li Q, et al. Free-Breathing, Non-Gated Heart-To-Brain CTA in Acute Ischemic Stroke: A Feasibility Study on Dual-Source CT. Front Neurol 2022;13:616964. [Crossref] [PubMed]
- Wang C, Wang Q, Li JL, et al. Clinical application of flash spiral mode of high-pitch dual source CT in carotid, cardiac and cerebral vessels combined one-stop angi-ography. Eur Rev Med Pharmacol Sci 2021;25:2852-7. [Crossref] [PubMed]
- Zhao L, Bao J, Guo Y, et al. Ultra-low dose one-step CT angiography for coronary, carotid and cerebral arteries using 128-slice dual-source CT: A feasibility study. Exp Ther Med 2019;17:4167-75. [Crossref] [PubMed]
- Zhang JL, Liu BL, Zhao YM, et al. Combining Coronary with Carotid and Cerebro-vascular Angiography Using Prospective ECG Gating and Iterative Reconstruction with 256-slice CT. Echocardiography 2015;32:1291-8. [Crossref] [PubMed]
- Sun K, Li K, Han R, et al. Evaluation of high-pitch dual-source CT angiography for evaluation of coronary and carotid-cerebrovascular arteries. Eur J Radiol 2015;84:398-406. [Crossref] [PubMed]
- Wang Z, Chen Y, Wang Y, et al. Feasibility of low-dose contrast medium high pitch CT angiography for the combined evaluation of coronary, head and neck arteries. PLoS One 2014;9:e90268. [Crossref] [PubMed]
- Xu C, Xu M, Yan J, et al. The impact of deep learning reconstruction on image qual-ity and coronary CT angiography-derived fractional flow reserve values. Eur Radiol 2022;32:7918-26. [Crossref] [PubMed]
- Tatsugami F, Higaki T, Nakamura Y, et al. Deep learning-based image restoration algorithm for coronary CT angiography. Eur Radiol 2019;29:5322-9. [Crossref] [PubMed]
- Jiang C, Jin D, Liu Z, et al. Deep learning image reconstruction algorithm for carot-id dual-energy computed tomography angiography: evaluation of image quality and diagnostic performance. Insights Imaging 2022;13:182. [Crossref] [PubMed]
- Koo SA, Jung Y, Um KA, et al. Clinical Feasibility of Deep Learning-Based Image Reconstruction on Coronary Computed Tomography Angiography. J Clin Med 2023;12:3501. [Crossref] [PubMed]
- Zhu L, Ha R, Machida H, et al. Image quality of coronary CT angiography at ultra low tube voltage reconstructed with a deep-learning image reconstruction algorithm in patients of different weight. Quant Imaging Med Surg 2023;13:3891-901. [Crossref] [PubMed]
- Li W, Diao K, Wen Y, et al. High-strength deep learning image reconstruction in coronary CT angiography at 70-kVp tube voltage significantly improves image quality and reduces both radiation and contrast doses. Eur Radiol 2022;32:2912-20. [Crossref] [PubMed]
- Li W, Lu H, Wen Y, et al. Reducing both radiation and contrast doses for overweight patients in coronary CT angiography with 80-kVp and deep learning image recon-struction. Eur J Radiol 2023;161:110736. [Crossref] [PubMed]
- Chen Y, Liu Z, Li M, et al. Reducing both radiation and contrast doses in coronary CT angiography in lean patients on a 16-cm wide-detector CT using 70 kVp and ASiR-V algorithm, in comparison with the conventional 100-kVp protocol. Eur Ra-diol 2019;29:3036-43. [Crossref] [PubMed]
- Lenfant M, Comby PO, Guillen K, et al. Deep Learning-Based Reconstruction vs. Iterative Reconstruction for Quality of Low-Dose Head-and-Neck CT Angiography with Different Tube-Voltage Protocols in Emergency-Department Patients. Diagnos-tics (Basel) 2022;12:1287. [Crossref] [PubMed]
- Austen WG, Edwards JE, Frye RL, et al. A reporting system on patients evaluated for coronary artery disease. Report of the Ad Hoc Committee for Grading of Coro-nary Artery Disease, Council on Cardiovascular Surgery, American Heart Associa-tion. Circulation 1975;51:5-40. [Crossref] [PubMed]
- Wen D, Zhao H, Duan W, et al. Combined CT angiography of the aorta and crani-ocervical artery: a new imaging protocol for assessment of acute type A aortic dis-section. J Thorac Dis 2017;9:4733-42. [Crossref] [PubMed]
- Halliburton SS, Abbara S, Chen MY, et al. SCCT guidelines on radiation dose and dose-optimization strategies in cardiovascular CT. J Cardiovasc Comput Tomogr 2011;5:198-224. [Crossref] [PubMed]
- Deak PD, Smal Y, Kalender WA. Multisection CT protocols: sex- and age-specific conversion factors used to determine effective dose from dose-length product. Radi-ology 2010;257:158-66. [Crossref] [PubMed]
- Zhang X, Zhang G, Xu L, et al. Application of deep learning reconstruction of ul-tra-low-dose abdominal CT in the diagnosis of renal calculi. Insights Imaging 2022;13:163. [Crossref] [PubMed]
- Jiang B, Li N, Shi X, et al. Deep Learning Reconstruction Shows Better Lung Nod-ule Detection for Ultra-Low-Dose Chest CT. Radiology 2022;303:202-12. [Crossref] [PubMed]
- Wang X, Zheng F, Xiao R, et al. Comparison of image quality and lesion diagnosis in abdominopelvic unenhanced CT between reduced-dose CT using deep learning post-processing and standard-dose CT using iterative reconstruction: A prospective study. Eur J Radiol 2021;139:109735. [Crossref] [PubMed]
- Brady SL, Trout AT, Somasundaram E, et al. Improving Image Quality and Reducing Radiation Dose for Pediatric CT by Using Deep Learning Reconstruction. Radiology 2021;298:180-8. [Crossref] [PubMed]
- Mileto A, Guimaraes LS, McCollough CH, et al. State of the Art in Abdominal CT: The Limits of Iterative Reconstruction Algorithms. Radiology 2019;293:491-503. [Crossref] [PubMed]
- Gordic S, Husarik DB, Desbiolles L, et al. High-pitch coronary CT angiography with third generation dual-source CT: limits of heart rate. Int J Cardiovasc Imaging 2014;30:1173-9. [Crossref] [PubMed]
- Benz DC, Ersözlü S, Mojon FLA, et al. Radiation dose reduction with deep-learning image reconstruction for coronary computed tomography angiography. Eur Radiol 2022;32:2620-8. [Crossref] [PubMed]
- Huang X, Zhao W, Wang G, et al. Improving image quality with deep learning image reconstruction in double-low-dose head CT angiography compared with standard dose and adaptive statistical iterative reconstruction. Br J Radiol 2023;96:20220625. [Crossref] [PubMed]