Quantification of enlarged deep medullary vein volumes in Sturge-Weber syndrome
Introduction
Sturge-Weber syndrome (SWS) is a rare neurocutaneous disorder originating from the vascular tissue in the brain and skin and most commonly associated with a somatic activating pathogenic variant in the GNAQ gene (1). SWS brain involvement is unilateral in the majority of cases and includes venous abnormalities such as leptomeningeal venous malformations (LVMs) and enlarged deep medullary and subependymal veins (2). These latter are thought to develop as a result of the LVM-induced superficial venous flow insufficiency to provide collateral venous flow toward the deep venous system (3-6). Unless such collaterals provide adequate blood flow compensation, chronic venous congestion in affected cortex and subcortical white matter underlying the LVM can lead to brain atrophy and calcification (7,8). Typical early clinical manifestations of SWS include focal seizures, stroke-like episodes, visual field impairment and, in a subset of patients, progressive cognitive deficits (9,10). Neuro-cognitive outcome can be optimized with aggressive seizure treatment including epilepsy surgery in selected patients (11,12).
While conventional post-contrast brain magnetic resonance imaging (MRI) provides an excellent means to detect LVMs in most patients with SWS, it may miss them in young patients during the early disease course (13,14). MRI with susceptibility-weighted imaging (SWI) has been increasingly used for radiological diagnosis of SWS, partly due to its superior ability to detect small cerebral veins, including enlarged deep medullary veins (EDMVs) even without or before the LVM can be visualized (2,13,15). Recent studies have also demonstrated the post-natal evolution and expansion of these veins during the early SWS disease course, as detected by SWI (5,16), leading to EDMVs in the vast majority of patients with unilateral SWS (6). Curiously, extensive EDMVs appeared to be more frequent in right hemispheric SWS and more commonly present at a young age in those with right SWS as compared to left hemispheric brain involvement. Also, a larger number of EDMVs, assessed by visual scoring, was associated with better seizure and cognitive outcomes in a subset of SWS patients (6). These data indicated that EDMVs may serve as an imaging marker of adaptive, venous vascular remodeling in SWS. Therefore, objective detection and quantification of EDMVs could provide a reproducible and clinically relevant MRI marker in SWS.
Recent studies demonstrated that venous volume quantification from SWI is feasible and reproducible in healthy volunteers and in patients with cerebral venous disorders (17,18). However, accurate detection and quantification of EDMVs remains challenging in SWS, partly because of the complex pathology of SWS brain involvement that includes both white matter and cortical atrophy as well as a variable degree of calcifications causing susceptibility changes in affected brain regions. In the present study, we report an EDMV volume quantification approach based on the use of a neuroimaging protocol that included among other sequences both SWI and volumetric T1-weighted images, which are now widely used in the clinical setting for SWS brain imaging. The goals of this study included: (I) to assess the feasibility of EDMV volumetry in patients with unilateral SWS; (II) to evaluate the correlation of visual EDMV scores (based on the number of EDMVs) with objective EDMV volume in SWS-affected hemispheres; and (III) to evaluate the relationship between EDMV volume and quantified brain parenchymal abnormalities (including both cortical surface and hemispheric white matter volumes) in SWS.
Methods
Study subjects
All data were collected prospectively in a clinical research study between 2018 and 2022 in Wayne State University, Detroit, Michigan, USA. The protocol was approved by the Wayne State University Human Investigation Committee, and a written informed consent form was signed by the study subjects or their parents. This study conformed to the provisions of the Declaration of Helsinki (as revised in 2013). Inclusion criteria for the SWS group were the following: (I) unilateral SWS detected by clinical MRI, defined as the presence of unilateral LVMs on post-contrast MRI, with or without a facial port-wine birthmark; (II) age 3 years and above; (III) good quality SWI and volumetric T1-weighted images available; and (IV) presence of EDMVs in the affected hemisphere, detected by visual assessment of SWI images. Exclusion criteria were: (I) bilateral SWS brain involvement on MRI and (II) history of epilepsy surgery; such patients were not included for this prospective study. The control group was recruited from healthy siblings of SWS patients. Inclusion criteria for this group: (I) age 3 years and above; (II) no history of any neurological or psychiatric disease; (III) normal neurological status upon physical examination; and (IV) no brain parenchymal abnormalities on MRI. For SWS patients with epilepsy, the MRI was done at least 24 hours after the last clinical seizure to minimize the potential confounding effect of seizure activity on vascular dilatation/engorgement or blood oxygenation/deoxygenation levels potentially affecting the SWI signal in the veins.
MRI acquisition
All MRI studies were performed on a Siemens MAGNETOM Verio 3T scanner (Siemens Medical Solutions, Erlangen, Germany). MRI acquisition included a native volumetric axial T1-weighted 3-dimensional magnetization prepared rapid gradient echo (with a voxel size of 0.9 mm × 0.9 mm × 0.9 mm; acquisition time: 4 min 35 s), followed by an SWI (acquisition time: 5 min) with the following image parameters: field of view 224 mm × 168 mm × 128 mm; base resolution 448; voxel size 0.5 mm × 0.5 mm × 2.0 mm; TR 30 ms/30 ms, TE 5.1 ms/18 ms; bandwidth 160–410 Hz/pixel, 2× accelerated GRAPPA parallel imaging with 24 reference lines, and 6/8 partial Fourier along phase encoding. An axial fluid attenuated inversion recovery and T2-weighted turbo spin-echo image was also obtained but not specifically used for the current study; in patients with SWS (but not in healthy siblings), a post-gadolinium T1-weighted image was also acquired. The youngest child (age 3 years) with SWS underwent moderate sedation to make sure that no significant motion artifacts occurred; all images of all subjects were reviewed after acquisition to ensure good image quality.
MRI image analysis
SWI images were processed offline using SPIN (Signal Processing in NMR; SpinTech MRI, Bingham Farms, MI, USA). SWI minimal intensity projection (MIP) images were generated, and the extent of EDMVs were determined visually by one of the investigators (who was blind to the subjects’ identity) in five major deep venous regions of the affected hemisphere in the frontal, central, parietal, temporal, and occipital regions of the SWS patients, as described recently (6). In this visual review process, each SWI MIP image was displayed on a screen, and EDMVs were identified in each of the regions by comparing the SWI-visualized medullary veins between homotopic regions in the affected and unaffected hemispheres. EDMV extent in the affected hemisphere was scored based on consensus as follows—score 0: no EDMVs; score 1: few [1–2] EDMVs in the region; score 2: several [3–5] EDMVs in the region; score 3: extensive [≥6] EDMVs in the region; thus, when combining the values from the five regions, the total hemispheric EDMV score ranged between 0 and 15. Test-retest reliability of this scoring was robust (intraclass correlation: 0.96) in a recent study (6).
Objective deep vein segmentation and volumetry
A series of voxel-based image analytic steps were performed to objectively segment EDMVs in selected white matter regions of individual subjects (see block diagram on Figure 1). Magnitude and phase images from each echo were combined by standard SWI reconstruction and averaged to generate a native SWI image of individual subjects (15). The native SWI image was then resampled at 1 mm × 1 mm × 1 mm resolution, denoised with a non-local means algorithm (19) and corrected to remove the intensity variation with shrink factor 4 and 50×50×50 iterations (20). Finally, the modified SWI data was linearly scaled to match intensity histogram peaks across all subjects and then used as a target image to co-register the native T1-weighted image using an affine transformation, D(x) with Advanced Normalization Tools (ANTs, http://stnava.github.io/ANTs/).
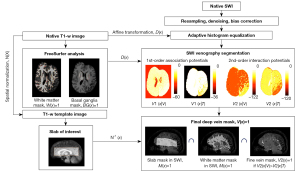
FreeSurfer analysis (21) was used for the native T1-weighted image (registered to native SWI) to create two binary masks of interest, (I) white matter mask: , if a voxel x locates at white matter and otherwise, , and (II) basal ganglia mask: , if a voxel x is located at basal ganglia including thalamus, putamen and caudate, and otherwise, . These masks are necessary for the following SWI venous segmentation to include the hypointense vessels only inside white matter and exclude similar hypointense voxels inside the basal ganglia.
Our SWI venous segmentation employed the following hybrid approach to create the deep vein mask, , if x belongs to the class of white matter deep vein and otherwise. Briefly, our approach consisted of two separate steps. Step 1 considers three first-order potentials of SWI intensity distribution, appearance, shape, and location to quantify the likelihood of vessel presence at the voxel x. Step 2 includes the second-order interaction potential to further improve the quantification of Step 1 by considering the likelihood values of neighboring voxels.
Step 1: evaluation of the first-order association potentials, and that integrate three association potentials, appearance (φ), shape (ψ), and location (ϕ) to quantify the likelihood of vessel (V) and tissue (T) with the given subsets of observation: at the voxel of x, respectively. Specifically, we define the association parameters as follows:
where, and model the potentials of vessel and tissue that exist at the voxel location x and were estimated using finite mixture model (FMM) with expectation-maximization (EM) algorithm (22). Based on the intensity histogram measured from the normal whole brain on SWI (23), the present study used the FMM-EM algorithm to classify the whole brain of individual subjects into four subclasses of distinct intensity levels, i.e., from the lowest to the highest intensity levels, as follows: lowest intensity regions (subclass 1, that may include high iron content/calcifications), 2nd lowest intensity regions (subclass 2, that may include deep veins/deep nuclei), 3rd lowest intensity regions (subclass 3, that may include cerebrospinal fluid/ventricles), and 4th lowest intensity regions (subclass 4 that may include gray/white matter). Under this configuration of the FMM-EM algorithm, is modeled as one normal (or Gaussian) probabilistic distribution (i.e., subclass 2 for V) and is modeled as a mixture of three normal (or Gaussian) probabilistic distributions (i.e., a mixture of three subclasses, 1, 3, and 4). All FMM parameters (i.e., proportion, mean, variance of individual distribution) were optimized using the EM algorithm. It should be noted that our FMM model works well to segment four subclasses in both healthy controls and SWS patients, since all FMM parameters were optimized based on four distinct ranges of absolute intensity values.
The function models the potential of tubular vessels at the voxel of x that Frangi’s maximal “vesselness” response filter quantifies at the level of vesselness scale range: σ (24); vesselness indicates the likelihood of an image region containing blood vessels or other image ridges and is measured by the eigenvectors of the Hessian matrix to quantify the tubular structure of the image object (25). In the present study, the range of σ was fixed from 0.5 to 2.5 at the step size of 0.25 to quantify . The shape-based was modeled to complement the intensity-based (24) so that a hypointense voxel with no tubular shape (e.g., due to calcification, which is common in SWS) becomes penalized to have less likelihood to be detected as the vessel class. is to guide the vein segmentation by incorporating two probabilistic masks: and (i.e., , where ρ defines the probability (or percentage) of vein voxel assumed in the . and are binary masks for white matter and basal ganglia, respectively).
The location-based favors keeping hypointense vessels inside white matter but excludes non-vessel-related low-intensity voxels inside basal ganglia nuclei (which are rich in iron) and elsewhere. Otherwise, and may incorrectly classify these iron-rich nuclei as blood vessels. All three association potentials ranged from 0 to 1 (i.e., the higher the value the higher likelihood to be classified as veins). Thus, a set of voxels, x, having (or ) constitutes the vein class (or 0) that incorporates three different attributes of vessel: intensity, shape, and anatomical location.
Step 2: evaluation of the second-order interaction potentials, and that model contextual dependencies of class memberships between all pairs of neighboring voxels, , that are defined in and .
where and penalize dissimilarity classification () between the memberships of neighbor voxels (xi,xj) to regularize the heterogeneous memberships at local neighbors and detect finer vein memberships at the edges of vessel and tissue. That is, and are the improved versions of and that have less noisy memberships and more vessel memberships. Thus, a set of voxels, x having [or ] constitutes fine vein class, (or 0). All details of the above potentials [i.e., , , , ] are well-documented in the previous literature (23). This study implemented these potentials with the user variables that were proposed in that study.
Finally, the native T1-weighted image (registered to native SWI) was spatially normalized using the ANTs to the T1-weighted template image that was generated from T1-weighted images of the subjects in the control group using an unbiased group averaging technique (26). The resulting non-linear deformation field, was then inversely applied to place a slab of interest (i.e., covering the middle section of the hemispheres vertically including the entire extent of the ventricles and encompassing much of the hemispheric white matter) at the same anatomical location of individual subjects. This slab was defined in the template space designated to exclude superficial veins near the boundaries of gray and white matter vertices and in the basal brain. Thus, the intersection of three binary masks in native SWI space: (I) the transformed slab mask ; (II) white matter mask ; and (III) fine vein mask defined the final mask of deep veins: that can quantify the volumes of medullary deep veins at the same anatomical locations of individual subjects. The voxel numbers of the deep vein mask were separately counted in both hemispheres to measure deep vein volumes (i.e., count × 1 mm3) in both (left and right) cerebral hemispheres. The volume of the final vein mask: , was controlled at three different levels of sensitivity (low, middle, high) by assuming three different values of ρ (0.05, 0.1, 0.15) for the location-based (23). Due to intrinsic partial volume effects, a higher ρ yields higher sensitivity detecting more EDMV voxels at the cost of more noisy voxels.
Calculation of hemispheric cortical surface and white matter volume
FreeSurfer analysis was used to define the gray-white matter surface in each hemisphere of the volumetric T1-weighted image that was pre-registered to the SWI. The area assigned to each vertex of the gray-white matter surface was calculated as the average area of all triangles that surrounded the vertex. We calculated the total area of the hemispheric cortical surface by multiplying total number of triangles by the constant size of each triangle. In addition, white matter volume of individual hemispheres was quantified using the FreeSurfer’s segmentation process. The volume inside the white matter surface for an individual hemisphere excluded cerebellar white matter and brainstem.
Statistical analysis
The Shapiro-Wilk test showed normal distributions for the study variables. Therefore, statistical analyses were performed using parametric tests (such as Pearson’s correlation, unpaired and paired t-tests, where appropriate). SPSS 26.0 was used to perform the statistical analysis, and P<0.05 was considered to be significant.
Results
A total of 20 subjects were included in the study: 10 patients with unilateral SWS and 10 healthy control siblings (7 females, 3 males) (Table 1). The mean age of the two groups was similar (SWS group: 14.5±6.7 years, control group: 16.0±7.0 years, P=0.62).
Table 1
Subject # | SWS | Healthy siblings | ||||||
---|---|---|---|---|---|---|---|---|
Gender | Age (years) | SWS side | Lobes involved | EDMV score | Gender | Age (years) | ||
1 | Female | 3 | Right | FTPO | 12 | Male | 7 | |
2 | Male | 8 | Right | P | 2 | Female | 10 | |
3 | Male | 9 | Right | TP (F†) | 10 | Male | 10 | |
4 | Female | 13 | Right | FTPO | 15 | Female | 11 | |
5 | Male | 13 | Left | FP | 1 | Male | 13 | |
6 | Female | 13.5 | Right | FTPO | 10 | Female | 16 | |
7 | Male | 20 | Left | TPO (F†) | 3 | Female | 20 | |
8 | Female | 20 | Right | P | 1 | Female | 21 | |
9 | Female | 21 | Left | FTPO | 9 | Female | 24 | |
10 | Male | 24 | Left | PO | 8 | Female | 28 |
†, limited EDMV in frontal white matter. SWS, Sturge-Weber syndrome; EDMVs, enlarged deep medullary veins; F, frontal; T, temporal; P, parietal; O, occipital.
EDMV scores on visual assessment
The number of EDMVs varied widely in the affected hemisphere of the 10 SWS patients (Table 1), with a hemispheric EDMV score ranging between 1–15 (mean: 7±5). A small asymptomatic developmental venous malformation was detected in the right frontal lobe of one of the healthy siblings (a 16-year-old girl), likely an incidental finding. No brain parenchymal abnormalities were present. Due to this, EDMV volume values from the right hemisphere of this healthy control subject were not included in subsequent calculations.
Hemispheric enlarged deep vein volumes
Figure 2 shows representative examples of segmented deep vein masks obtained from one SWS subject (a 13-year-old girl with extensive right hemispheric SWS brain involvement; #4 in Table 1), when applying the three sensitivity levels. Most of the EDMVs were detected in the right hemisphere with all three sensitivities, while the large calcified area in the frontal lobe was excluded. Still, the high sensitivity segmentation at ρ=0.15 detected EDMVs with higher noise levels (i.e., many tiny and broken branch segments appeared due to intrinsic partial volume effect) in the affected hemisphere and several (normal) deep vein segments in the unaffected hemisphere, whereas the noisy branch segments as well as background noise points were minimized with the segmentations using the middle sensitivity at ρ=0.1 and the low sensitivity at ρ=0.05, while picking up substantially the EDMVs in the affected hemisphere. Representative segmented enlarged deep veins, when using the middle sensitivity level, are shown in two left and two right SWS patients on Figures 3,4, respectively. Table 2 shows the individual segmented deep vein volumes picked up by each sensitivity setting in all SWS patients, along with the range of these values in the healthy control group. As expected, the measured deep vein volumes increased with the increasing ρ values of the location-based (providing higher sensitivity to detect thinner veins at the cost of picking up some noise, as more voxels were picked up). The mean quantified EDMV volumes were approximately 10–12-fold greater in the SWS-affected hemispheres than in the unaffected or control hemispheres at all three sensitivity settings (Table 3). The volumes measured in the brains of the control subjects vs. in the unaffected hemispheres of the SWS subjects were similar, did not differ significantly (Table 3).
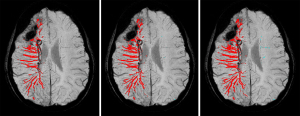
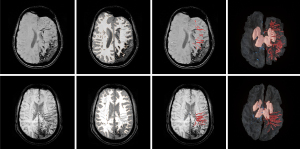
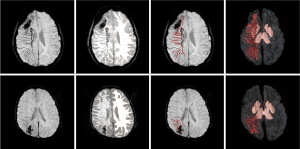
Table 2
Patient # | SWS side | Low sensitivity | Middle sensitivity | High sensitivity | EDMV score | |||||
---|---|---|---|---|---|---|---|---|---|---|
Left [0–175]a | Right [0–190]a | Left [0–308]a | Right [0–316]a | Left [0–459]a | Right [8–420]a | |||||
1 | Right | 63 | 1,338c | 74 | 2,322c | 78 | 3,147c | 12 | ||
2 | Right | 95 | 117b | 144 | 203b | 199 | 314b | 2 | ||
3 | Right | 22 | 1,118c | 48 | 1,827c | 60 | 2,360c | 10 | ||
4 | Right | 101 | 4,824c | 190 | 7,117c | 266 | 8,777c | 15 | ||
5 | Left | 188c | 200d | 276b | 301 | 331b | 380 | 1 | ||
6 | Right | 175 | 2,835c | 206 | 4,273c | 241 | 5,425c | 10 | ||
7 | Left | 242c | 211d | 403c | 451d | 619c | 713 | 3 | ||
8 | Right | 26 | 71b | 99 | 92b | 185 | 137b | 1 | ||
9 | Left | 2,257c | 107 | 2,994c | 135 | 3,853c | 167 | 9 | ||
10 | Left | 1,996c | 119 | 2,660c | 196 | 3,160c | 305 | 8 |
a, indicates the range of deep vein volumes measured in the 10 healthy controls in the left and right hemispheres (i.e., the normative range) with each level of sensitivity; b, indicates deep vein volumes measured within the normal ranges in the SWS-affected hemisphere which occurred only in patients with low [1–2] EDMV scores; c, indicates volumes outside (above) the normal range in SWS-affected hemispheres; d, indicates individual EDMV volumes outside (above) the normal range in SWS unaffected hemispheres (picked up with all sensitivity levels in patient #7). SWS, Sturge-Weber syndrome; EDMV, enlarged deep medullary vein.
Table 3
Hemisphere side | Low sensitivity | Middle sensitivity | High sensitivity | |||||
---|---|---|---|---|---|---|---|---|
Left | Right | Left | Right | Left | Right | |||
Normal hemispheres | ||||||||
SWS unaffected | 80±57 | 159±54 | 127±64 | 271±138 | 172±85 | 392±232 | ||
Healthy siblings | 75±61 | 88±68 | 241±102 | 140±10 | 210±156 | 195±145 | ||
P value | 0.86 | 0.1 | 0.76 | 0.1 | 0.59 | 0.09 | ||
SWS-affected hemisphere | 1,170±1,109 | 1,717±1,826 | 1,583±1,444 | 2,638±2,680 | 1,991±1,777 | 3,360±3,296 |
Mean (± standard deviation) left and right hemispheric EDMV volumes (at low, middle, and high levels of segmentation sensitivity; in mm3), measured in the unaffected (left and right, depending on the side of SWS brain involvement) hemispheres of the SWS patients vs. healthy siblings showed no significant differences. Mean hemispheric EDMV volumes measured in the SWS-affected hemispheres (bottom cells) were approximately 10–12-fold greater, in average. EDMV, enlarged deep medullary vein; SWS, Sturge-Weber syndrome.
Comparison of EDMV volumes and visual EDMV scores
In all seven patients with visual EDMV scores >2, the measured EDMV volumes in the SWS-affected hemisphere were outside the range of the healthy control group (Table 2). In contrast, in the three patients with only a few EDMVs (i.e., scores 1–2 on visual assessment), the measured deep vein volumes were within the normal range in two, when the low sensitivity level was used, and in all three subjects when the middle or high sensitivity levels were used. Deep vein volumes in the SWS-unaffected hemispheres were within the normal range in 8 or 9 patients and slightly above the range in 2 or 1, depending on the sensitivity level used (Table 2). Overall, the segmented EDMV values measured with the middle and high sensitivity level picked up the same hemispheres showing above the normal range, while the values obtained with the low sensitivity level showed above-range values in one additional case (#5) in both hemispheres. The measured EDMV volumes in the SWS-affected hemispheres showed a strong correlation with the EDMV scores (r=0.88, P=0.001 for the middle sensitivity; very similar for the other two sensitivities, data not shown).
Cortical surface area, white matter volume, and their correlation with enlarged deep vein volumes
In the control group, left and right hemispheric values of the cortical surface areas and white matter volumes did not differ (1,030±89 vs. 1,038±96 cm2, P=0.24; and 220±18 vs. 223±15 cm3, P=0.16, respectively) (Table 4). In the SWS group, both variables were lower in the affected hemispheres than in the unaffected hemispheres [mean surface area: 918±182 vs. 1,015±123 cm2, respectively, P=0.020; mean white matter volume: 187±47 vs. 218±44 cm3, P=0.032], consistent with atrophy in affected hemispheres (Table 5). Individually, lower values were measured in the SWS-affected hemisphere in all but one patient (#2, an 8-year-old with a small right parietal SWS involvement) (Table 5). In addition, white matter volume ratios of the affected/unaffected hemispheres showed an inverse correlation with duration of epilepsy (r=−0.68, P=0.03) and a trend with age (r=−0.59, P=0.07), indicating more robust white matter volume loss in older patients with longer epilepsy duration; cortical surface ratios (affected/unaffected hemisphere) did not correlate with age or epilepsy duration (P>0.10). Finally, cortical surface ratios in the SWS group showed an inverse correlation with EDMV volumes measured in the affected hemispheres using the middle threshold [r=−0.65, P=0.04 (Figure 5); similar significant correlations were present with data from the other two sensitivities; data not shown]. White matter volume ratios did not correlate with EDMV volumes (P>0.1 for all thresholds).
Table 4
Control group # | Cortical surface area (cm2) | White matter volume (cm3) | Age (years) | |||
---|---|---|---|---|---|---|
Left | Right | Left | Right | |||
1 | 943 | 986 | 188 | 203 | 7 | |
2 | 939 | 917 | 214 | 215 | 10 | |
3 | 1,137 | 1,163 | 219 | 226 | 10 | |
4 | 1,049 | 1,057 | 202 | 199 | 11 | |
5 | 1,157 | 1,153 | 240 | 241 | 13 | |
6 | 1,091 | 1,113 | 228 | 236 | 16 | |
7 | 1,047 | 1,053 | 227 | 225 | 20 | |
8 | 1,040 | 1,030 | 228 | 234 | 21 | |
9 | 1,020 | 1,043 | 248 | 241 | 24 | |
10 | 875 | 864 | 208 | 212 | 28 | |
Mean ± SD | 1,030±89 | 1,038±96 | 220±18 | 223±15 | – |
SD, standard deviation.
Table 5
SWS group # | Cortical surface area (cm2) | White matter volume (cm3) | Age (years) | |||
---|---|---|---|---|---|---|
Affected | Unaffected | Affected | Unaffected | |||
1 | 720 | 811 | 125 | 128 | 3 | |
2 | 1,043 | 946 | 208 | 184 | 8 | |
3 | 996 | 1,059 | 200 | 209 | 9 | |
4 | 939 | 1,105 | 190 | 212 | 13 | |
5 | 1,173 | 1,225 | 233 | 251 | 13 | |
6 | 654 | 875 | 127 | 187 | 13 | |
7 | 1,072 | 1,080 | 240 | 258 | 20 | |
8 | 971 | 1,027 | 224 | 246 | 20 | |
9 | 647 | 932 | 116 | 226 | 21 | |
10 | 961 | 1,093 | 207 | 276 | 24 | |
Mean ± SD | 918±182 | 1,015±123 | 187±47 | 218±44 | – |
SD, standard deviation; SWS, Sturge-Weber syndrome.
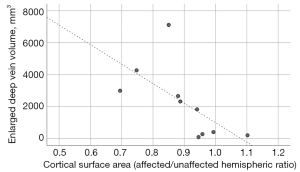
Discussion
In this study, we evaluated an objective approach to quantify EDMVs in patients with SWS. With the combination of SWI and volumetric T1-weighted sequences, we were able to detect EDMVs in the SWS-affected hemispheres: the actual EDMV volumes depended on the assumed level of segmentation sensitivity, but visual inspection of the images demonstrated adequate pick-up of the bulk of EDMVs with all three levels of segmentation sensitivity. Small, mostly isolated fragments of likely normal deep veins were also picked up in both healthy controls and unaffected hemispheres of the SWS patients; still, as expected, SWS-affected hemispheres showed much higher deep vein volumes, as compared to non-affected hemispheres. The applied method was able to confine the volume detection to deep veins, while excluding calcified brain lesions that are common in SWS and show similar signal loss. Thus, EDMV volumes, along with the other quantitative image variables used in this study (white matter volume and cortical surface area, characterizing the level of atrophy) may provide useful imaging biomarkers for future clinical trials for which such biomarkers are needed to monitor the effects of therapeutic interventions objectively (27). Unlike previous studies in SWS patients, the present study also included data from healthy siblings of SWS patients. This allowed us to demonstrate that EDMV volumes, hemispheric white matter volumes, and gray matter surface areas were similar in the unaffected hemispheres of SWS patients and healthy controls. These data indicated that hemispheres not affected by the LVM in SWS patients are largely intact structurally, which is important for allowing effective contralateral functional reorganization in those with extensive unilateral SWS brain involvement (28).
The measured EDMV volumes correlated well with the visually assessed EDMV scores; the latter relied on the number (rather than volume) of enlarged deep veins (6). EDMV volumes in the SWS-affected hemispheres were outside the normal range in all cases with more than a few EDMVs (i.e., scores greater than 1–2). In the three patients with EDMV scores of 1 or 2, the hemispheric EDMV volumes fell within the control range, as the overall volume of the few enlarged veins was small. This indicates a limitation of the sensitivity of hemispheric deep vein volumetry, but regional (lobar) volumetry may overcome this in the future. A limited number of EDMVs is usually associated with small (often unilobar) SWS brain involvement as seen in our three patients with low EDMV scores. Most of these patients are doing well neurologically, while in those with extensive multilobar involvement the extent of deep venous remodeling may be important to salvage venous blood flow and avoid severe parenchymal damage (5,6). In such cases, EDMV volumes and their longitudinal changes can be assessed by non-contrast SWI and T1-weighted images and provide an objective imaging marker of the deep venous compensatory changes during the disease course.
Our data also indicate a variable decrease in hemispheric white matter volume and cortical surface area in the SWS-affected hemispheres, consistent with atrophy. White matter volume ratios (affected/unaffected side; which was used to diminish age effects) were lower in those with longer duration of epilepsy. This is consistent with a previous study indicating progressive hemispheric atrophy as suggested by more severe volumetric abnormalities in older SWS patients (7). In that study, lower white matter volume (but not gray matter volume) was an independent predictor of poorer cognitive functions. While the current cohort was too small to evaluate neuro-cognitive correlates of volumetric values, or perform multivariate correlations, the data reinforce that white matter volume loss is likely progressive in SWS, as the hemispheric differences are more robust in older patients with longer epilepsy history. Interestingly, EDMV volumes correlated better with cortical surface area ratios than with hemispheric white matter volume ratios (where the correlation was not significant). This is consistent with the notion that extensive LVMs are associated with more extensive atrophy of the underlying cortex, which can facilitate development of more robust and extensive enlarged deep vein collaterals.
Potential mechanisms of such deep venous remodeling have been discussed in our recent study (6). One plausible mechanism is the early increase of venous pressure around the LVM-affected brain regions as a result of impaired superficial venous drainage, which can then facilitate the expansion of medullary and other deep vein collaterals to relieve this pressure by channeling the venous blood toward the deep vein system (29). The brain vasculature can undergo large-scale rearrangements not only in SWS but in various other pathologies, including stroke (30), traumatic brain injury (31), and epilepsy (32). Adaptive venous remodeling induced by cerebral venous occlusion or insufficiency (present in but not confined to SWS) can diminish parenchymal damage by developing adequate collaterals during the early disease course (3). Our current study demonstrates the ability of SWI (combined with volumetric T1-weighted images) to quantify the extent of such remodeling in the developing human brain, even in the presence of other pathology such atrophy and calcifications. The applied approach may also be tested in other pathologic conditions associated with abnormalities of deep medullary veins.
Our study limitations include the small number of patients: SWS is a rare disorder and collection of prospective imaging data in a single center takes a long time; we included only recent patients who met all inclusion criteria and had a uniform imaging acquisition protocol using the same scanner. In addition, for the first time, we collected similar imaging data from healthy siblings, which is a new aspect for an SWS brain imaging study. It should be noted that all study subjects except the youngest SWS patient had their MRI scans acquired without sedation in order to rule out the effect of sedative on SWI venous vascular contrast (33). The SWI of the sedated patient had good vascular contrast, similar to scans obtained without sedation, therefore, we included this patient in the study. Thus, no confounding issue related to the sedative should be considered in the statistical findings of the present study. However, the main disadvantage of the multi-echo SWI acquisition is that longer TEs accentuate T2*-weighted contrast increasing signal attenuation in subcortical structures (e.g., basal ganglia) with higher iron content. Even though this limitation was mitigated by adding their location priors, the iron in subcortical structures may not be completely differentiated from subcortical veins, especially near the outer boundary region of the subcortical structures, where intrinsic partial volume effect may add more false positive classifications to the resulting vessel class. Thus, the presented segmentation method may require additional masking (e.g., dilated subcortical mask) to correct such false positives for the subsequent analysis. Recent work using Strategically Acquired Gradient Echo (STAGE) imaging has automated the segmentation of the deep gray matter, which, along with the simultaneous collection of T1 and SWI data, avoids the need for co-registration (34,35). Future studies could improve the classification accuracy of the presented segmentation procedure by including larger cohorts and longitudinal STAGE data with quantitative susceptibility mapping data and improved signal-to-noise ratio to evaluate the progressive nature of the detected vascular and parenchymal abnormalities and compare them to clinical variables. Finally, a future study is warranted for the replication of our segmentation accuracy in larger, independent cohorts via a multi-center study.
Conclusions
The applied approach using the combination of SWI and volumetric T1-weighted images can provide volumes of EDMVs while avoiding calcifications in the affected cerebral hemisphere along with white matter volumes and cortical surface areas in patients with SWS. Acquisition of native SWI and volumetric T1-weighted images is clinically highly feasible, even in longitudinal studies, and the obtained quantitative variables have the potential to serve as prognostic and predictive imaging biomarkers in clinical trials in patients with SWS.
Acknowledgments
We are grateful to the Sturge-Weber Foundation for participant referrals.
Funding: This study was supported by
Footnote
Conflicts of Interest: All authors have completed the ICMJE uniform disclosure form (available at https://qims.amegroups.com/article/view/10.21037/qims-23-1271/coif). C.J. reports that this study was supported by NIH grants (Nos. NS041922 and NS065705). The other authors have no conflicts of interest to declare.
Ethical Statement: The authors are accountable for all aspects of the work in ensuring that questions related to the accuracy or integrity of any part of the work are appropriately investigated and resolved. The study was conducted in accordance with the Declaration of Helsinki (as revised in 2013). The study was approved by the Wayne State University Human Investigation Committee and a written informed consent form was signed by the study subjects or their parents.
Open Access Statement: This is an Open Access article distributed in accordance with the Creative Commons Attribution-NonCommercial-NoDerivs 4.0 International License (CC BY-NC-ND 4.0), which permits the non-commercial replication and distribution of the article with the strict proviso that no changes or edits are made and the original work is properly cited (including links to both the formal publication through the relevant DOI and the license). See: https://creativecommons.org/licenses/by-nc-nd/4.0/.
References
- Shirley MD, Tang H, Gallione CJ, Baugher JD, Frelin LP, Cohen B, North PE, Marchuk DA, Comi AM, Pevsner J. Sturge-Weber syndrome and port-wine stains caused by somatic mutation in GNAQ. N Engl J Med 2013;368:1971-9. [Crossref] [PubMed]
- Hu J, Yu Y, Juhasz C, Kou Z, Xuan Y, Latif Z, Kudo K, Chugani HT, Haacke EM. MR susceptibility weighted imaging (SWI) complements conventional contrast enhanced T1 weighted MRI in characterizing brain abnormalities of Sturge-Weber Syndrome. J Magn Reson Imaging 2008;28:300-7. [Crossref] [PubMed]
- Linscott LL, Leach JL, Jones BV, Abruzzo TA. Imaging patterns of venous-related brain injury in children. Pediatr Radiol 2017;47:1828-38. [Crossref] [PubMed]
- Hakim A, Aguiar de Sousa D. Brush sign in Sturge-Weber syndrome. Pediatr Radiol 2018;48:895-6. [Crossref] [PubMed]
- John F, Maqbool M, Jeong JW, Agarwal R, Behen ME, Juhász C. Deep cerebral vein expansion with metabolic and neurocognitive recovery in Sturge-Weber syndrome. Ann Clin Transl Neurol 2018;5:502-6. [Crossref] [PubMed]
- Juhász C, Luat AF, Behen ME, Gjolaj N, Jeong JW, Chugani HT, Kumar A. Deep Venous Remodeling in Unilateral Sturge-Weber Syndrome: Robust Hemispheric Differences and Clinical Correlates. Pediatr Neurol 2023;139:49-58. [Crossref] [PubMed]
- Juhasz C, Lai C, Behen ME, Muzik O, Helder EJ, Chugani DC, Chugani HT. White matter volume as a major predictor of cognitive function in Sturge-Weber syndrome. Arch Neurol 2007;64:1169-74. [Crossref] [PubMed]
- Pilli VK, Behen ME, Hu J, Xuan Y, Janisse J, Chugani HT, Juhász C. Clinical and metabolic correlates of cerebral calcifications in Sturge-Weber syndrome. Dev Med Child Neurol 2017;59:952-8. [Crossref] [PubMed]
- Comi AM. Presentation, diagnosis, pathophysiology, and treatment of the neurological features of Sturge-Weber syndrome. Neurologist 2011;17:179-84. [Crossref] [PubMed]
- Bosnyák E, Behen ME, Guy WC, Asano E, Chugani HT, Juhász C. Predictors of Cognitive Functions in Children With Sturge-Weber Syndrome: A Longitudinal Study. Pediatr Neurol 2016;61:38-45. [Crossref] [PubMed]
- Luat AF, Behen ME, Chugani HT, Juhász C. Cognitive and motor outcomes in children with unilateral Sturge-Weber syndrome: Effect of age at seizure onset and side of brain involvement. Epilepsy Behav 2018;80:202-7. [Crossref] [PubMed]
- Powell S, Fosi T, Sloneem J, Hawkins C, Richardson H, Aylett S. Neurological presentations and cognitive outcome in Sturge-Weber syndrome. Eur J Paediatr Neurol 2021;34:21-32. [Crossref] [PubMed]
- Mentzel HJ, Dieckmann A, Fitzek C, Brandl U, Reichenbach JR, Kaiser WA. Early diagnosis of cerebral involvement in Sturge-Weber syndrome using high-resolution BOLD MR venography. Pediatr Radiol 2005;35:85-90. [Crossref] [PubMed]
- Catsman-Berrevoets CE, Koudijs SM, Buijze MSJ, de Laat PCJ, Pasmans SGMA, Dremmen MHG. Early MRI diagnosis of Sturge Weber Syndrome type 1 in infants. Eur J Paediatr Neurol 2022;38:66-72. [Crossref] [PubMed]
- Haacke EM, Mittal S, Wu Z, Neelavalli J, Cheng YC. Susceptibility-weighted imaging: technical aspects and clinical applications, part 1. AJNR Am J Neuroradiol 2009;30:19-30. [Crossref] [PubMed]
- Pilli VK, Chugani HT, Juhász C. Enlargement of deep medullary veins during the early clinical course of Sturge-Weber syndrome. Neurology 2017;88:103-5. [Crossref] [PubMed]
- Egger K, Dempfle AK, Yang S, Schwarzwald R, Harloff A, Urbach H. Reliability of cerebral vein volume quantification based on susceptibility-weighted imaging. Neuroradiology 2016;58:937-42. [Crossref] [PubMed]
- Dempfle AK, Harloff A, Schuchardt F, Bäuerle J, Yang S, Urbach H, Egger K. Longitudinal Volume Quantification of Deep Medullary Veins in Patients with Cerebral Venous Sinus Thrombosis: Venous Volume Assessment in Cerebral Venous Sinus Thrombosis Using SWI. Clin Neuroradiol 2018;28:493-9. [Crossref] [PubMed]
- Coupe P, Yger P, Prima S, Hellier P, Kervrann C, Barillot C. An optimized blockwise nonlocal means denoising filter for 3-D magnetic resonance images. IEEE Trans Med Imaging 2008;27:425-41. [Crossref] [PubMed]
- Tustison NJ, Avants BB, Cook PA, Zheng Y, Egan A, Yushkevich PA, Gee JC. N4ITK: improved N3 bias correction. IEEE Trans Med Imaging 2010;29:1310-20. [Crossref] [PubMed]
- Fischl B. FreeSurfer. Neuroimage 2012;62:774-81. [Crossref] [PubMed]
- Wilson DL, Noble JA. An adaptive segmentation algorithm for time-of-flight MRA data. IEEE Trans Med Imaging 1999;18:938-45. [Crossref] [PubMed]
- Bériault S, Xiao Y, Collins DL, Pike GB. Automatic SWI Venography Segmentation Using Conditional Random Fields. IEEE Trans Med Imaging 2015;34:2478-91. [Crossref] [PubMed]
- Frangi AF, Niessen WJ, Vincken KL, Viergever MA. Multiscale vessel enhancement filtering. In: Wells WM, Colchester ACF, Delp SL, editors. Medical Image Computing and Computer-Assisted Intervention — MICCAI’98. LNCS, vol. 1496. Berlin: Springer, 1998, pp. 130-7.
- Avadiappan S, Payabvash S, Morrison MA, Jakary A, Hess CP, Lupo JM. A Fully Automated Method for Segmenting Arteries and Quantifying Vessel Radii on Magnetic Resonance Angiography Images of Varying Projection Thickness. Front Neurosci 2020;14:537. [Crossref] [PubMed]
- Tournier JD, Smith R, Raffelt D, Tabbara R, Dhollander T, Pietsch M, Christiaens D, Jeurissen B, Yeh CH, Connelly A. MRtrix3: A fast, flexible and open software framework for medical image processing and visualisation. Neuroimage 2019;202:116137. [Crossref] [PubMed]
- De la Torre AJ, Luat AF, Juhász C, Ho ML, Argersinger DP, Cavuoto KM, Enriquez-Algeciras M, Tikkanen S, North P, Burkhart CN, Chugani HT, Ball KL, Pinto AL, Loeb JA. A Multidisciplinary Consensus for Clinical Care and Research Needs for Sturge-Weber Syndrome. Pediatr Neurol 2018;84:11-20. [Crossref] [PubMed]
- Kim JA, Jeong JW, Behen ME, Pilli VK, Luat A, Chugani HT, Juhász C. Metabolic correlates of cognitive function in children with unilateral Sturge-Weber syndrome: Evidence for regional functional reorganization and crowding. Hum Brain Mapp 2018;39:1596-606. [Crossref] [PubMed]
- Parsa CF. Sturge-weber syndrome: a unified pathophysiologic mechanism. Curr Treat Options Neurol 2008;10:47-54. [Crossref] [PubMed]
- Liu J, Wang Y, Akamatsu Y, Lee CC, Stetler RA, Lawton MT, Yang GY. Vascular remodeling after ischemic stroke: mechanisms and therapeutic potentials. Prog Neurobiol 2014;115:138-56. [Crossref] [PubMed]
- Gama Sosa MA, De Gasperi R, Perez Garcia GS, Perez GM, Searcy C, Vargas D, Spencer A, Janssen PL, Tschiffely AE, McCarron RM, Ache B, Manoharan R, Janssen WG, Tappan SJ, Hanson RW, Gandy S, Hof PR, Ahlers ST, Elder GA. Low-level blast exposure disrupts gliovascular and neurovascular connections and induces a chronic vascular pathology in rat brain. Acta Neuropathol Commun 2019;7:6. [Crossref] [PubMed]
- Arango-Lievano M, Boussadia B, De Terdonck LDT, Gault C, Fontanaud P, Lafont C, Mollard P, Marchi N, Jeanneteau F. Topographic Reorganization of Cerebrovascular Mural Cells under Seizure Conditions. Cell Rep 2018;23:1045-59. [Crossref] [PubMed]
- Sedlacik J, Löbel U, Kocak M, Loeffler RB, Reichenbach JR, Broniscer A, Patay Z, Hillenbrand CM. Attenuation of cerebral venous contrast in susceptibility-weighted imaging of spontaneously breathing pediatric patients sedated with propofol. AJNR Am J Neuroradiol 2010;31:901-6. [Crossref] [PubMed]
- Chen Y, Liu S, Wang Y, Kang Y, Haacke EM. STrategically Acquired Gradient Echo (STAGE) imaging, part I: Creating enhanced T1 contrast and standardized susceptibility weighted imaging and quantitative susceptibility mapping. Magn Reson Imaging 2018;46:130-9. [Crossref] [PubMed]
- Haacke EM, Chen Y, Utriainen D, Wu B, Wang Y, Xia S, et al. STrategically Acquired Gradient Echo (STAGE) imaging, part III: Technical advances and clinical applications of a rapid multi-contrast multi-parametric brain imaging method. Magn Reson Imaging 2020;65:15-26. [Crossref] [PubMed]