Development and validation of a radiomics model based on T2-weighted imaging for predicting the efficacy of high intensity focused ultrasound ablation in uterine fibroids
Introduction
Uterine fibroid is a common benign tumor with more than 50% morbidities in reproductive-aged women, and some patients experience abnormal menstruation, anemia and other symptoms, seriously affecting the quality of life (1-3). High intensity focused ultrasound (HIFU) is a non-invasive therapy with few or without adverse events (4,5). HIFU ablation can induce the coagulated necrosis of uterine fibroids, which has been widely used in the treatment of uterine fibroids and some malignant tumors (6-8). The non-perfused volume ratio (NPVR) after HIFU procedure is an indicator of the efficacy of ablation in uterine fibroids, which is associated with the difference of T2-weighted imaging (T2WI) signal intensity. The intensity of T2WI signal is classified as hypointense, isointense, hyperintense and hybrid intense types (9,10). The T2WI signal intensity has been employed to predict the ablation efficacy of HIFU in treatment of uterine fibroids. However, the intensity classification could not fully reflect the characteristics of the fibroids (10,11).
Radiomics analysis is developing rapidly in recent years for medical images analysis (12,13). It can extract a large number of features from medical images to quantify phenotypic characteristics of tumors for diagnosis, clinical prognosis, treatment selection, or other decision support (12-16). Currently, it has been widely used in the differential diagnosis and analysis of benign and malignant tumors (17-21). A study has shown promising results for predicting the efficacy of HIFU ablation therapy in patients with adenomyosis using T2-weighted imaging-based radiomics model (19). Unfortunately, there remains lack of studies that using radiomics features of T2WI images to predict the efficacy of HIFU ablation for uterine fibroids due to the complexity of radiomics analysis (10,21). In order to investigate the predictive effectiveness of T2WI-based radiomics, we performed a retrospective study for the patients with uterine fibroids treated by HIFU ablation. The patients were divided into the two groups: higher ablation rate (NPVR ≥80%) and lower ablation rate (NPVR <80%) after HIFU ablation. This study aims to develop and validate a radiomics model based on the T2WI images to preoperatively predict efficacy of HIFU ablation of uterine fibroids. The clinicians can use this model to automatically predict HIFU treatment outcome so as to guide patient selection. We present this article in accordance with the TRIPOD reporting checklist (available at https://qims.amegroups.com/article/view/10.21037/qims-23-916/rc).
Methods
Patients
One hundred and seventy-eight patients with symptomatic uterine fibroids were consecutively enrolled from the imaging and interventional radiology clinic, and these patients were treated with ultrasound-guided HIFU (USgHIFU) in Shanghai Xuhui Central Hospital between February 2017 and December 2021. The inclusion criteria were as follows: premenopausal or perimenopausal women who (I) were above 25 years old; (II) diagnosed with clinical symptomatic uterine fibroids with diameters ≥3 cm; (III) received magnetic resonance imaging (MRI) examinations before and after HIFU therapy, and (IV) had no previous history of surgery or drug treatment. The exclusion criteria were as follows: women who (I) were pregnant or during menstrual period; (II) had contraindications of MRI exam or contrast agents containing gadolinium; (III) had a uterine malignant tumor; (IV) had pelvic inflammation disease or uncontrollable systemic disease; (V) could not lie in the prone position for 2–3 hours, and (VI) had history of high dose abdominal radiotherapy or severe connective tissue diseases. All data were collected retrospectively. The average age of all the patients was 40.28±6.58 years (range, 25 to 49 years), and a total of 200 fibroids from 178 patients were selected and included in the subsequent analysis (Figure 1). The non-perfusion volume ratio was acquired by the contrast-enhanced MRI (3D_VIBE_T1WI, three-dimension volumetric interpolated breath-hold examination T1-weighted image) sequence before and after the HIFU. The procedure time, sonication time, acoustic energy and average acoustic power of the HIFU ablation were collected also retrospectively and analyzed.
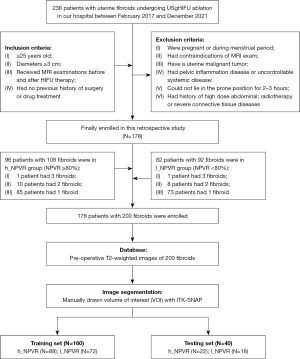
The study was conducted in accordance with the Declaration of Helsinki (as revised in 2013). The study was approved by the Ethics Committee of Shanghai Xuhui Central Hospital (No. 2019-094) and individual consent for this retrospective analysis was waived.
USgHIFU equipment and procedure
The USgHIFU procedure was performed using a JC-200 extracorporeal USgHIFU system (Chongqing Haifu Tech Co, Ltd., Chongqing, China), which was equipped with a diagnostic ultrasonic probe (frequency 5 MHz, Esaote, MyLab 70, Genoa, Italy). It had a single 20-cm diameter focused piezoelectric ceramic ultrasound transducer with a focal length of 14 cm and operating at a frequency of 1.0 MHz generated the ultrasound energy (the ultrasonic power range, 50 to 400 W). The transducer can be moved smoothly in six directions (X-axis: right and left; Y-axis: cranial and caudal; Z-axis: inferior and superior) by using computer control. The corresponding movement ranges were 120, 120, and 180 mm for three directions, respectively.
The patients underwent bowel preparation after 12 hours of fasting. Each patient was shaved from the anterior abdominal wall down to the public crest. A Foley catheter was inserted into the patient’s urinary bladder before the treatment. During the HIFU procedure, they took up a prone position on the treatment table. If the bowels were found to be laid between the transducer and the targeted fibroid, it could be pushed away from the acoustic pathway by filling the bladder with urine or using extracorporeally a degassed water balloon to avoid intestinal damage. All patients were administered an intravenous sedative and analgesic (0.8–1.0 µg/kg fentanyl; 0.02–0.03 mg/kg midazolam hydrochloride) to maintain conscious sedation. Their vital signs were monitored during the therapeutic procedure. Patients were requested to inform the operator if there was any pain or discomfort (1).
The therapeutic strategy was that the target regions of focused ultrasound were deployed on a deeper layer of the fibroid initially. Then, the focal ablation gradually moved from its sub-deep layer toward superficial layers. The initial treatment was at 100 W, and the highest power was 400 W. The duration of each sonication was 1–3 seconds, followed by a 2- or 3-second cooling period for HIFU treatment. The acoustic power and therapeutic energy were determined based on the patients’ feedback or/and the grayscale changes on the sonographic images. After a targeted focus ablation was completed, the focus was moved to the next neighboring target based on the treatment plan. When the grayscale change (echo enhancement) covered most of the fibroid, the treatment was considered completed. Treatment would be stopped or the focal spot would be changed if the patient felt severe or intolerant pain or complained of nerve numbness or pain radiating to the lower leg (1). One of the authors (Y.X.), who has had more than 15 years’ HIFU ablation experience, performed all patients’ procedures. All patients had only one treatment session.
Magnetic resonance (MR) equipment and examination
A Siemens 3.0 T MRI scanner (Verio Tim, Siemens Medical Systems, Erlangen, Germany) was used for pre- and post-HIFU therapy image evaluation. Patients were in supine position on an MRI bed and 4-channel abdominal coils were performed. All patients underwent MRI using a standardized protocol including T1WI, T2WI, diffusion-weighted imaging (DWI), pre- and post-contrast 3D-T1WI images at the axial, coronal, and sagittal planes. The scan sequences and parameters were as follows: sagittal T1WI: repetition time (TR) =720 ms, echo time (TE) =12 ms, field of view (FOV) =260 mm × 260 mm, slice thickness =4 mm, slice gap =1.2 mm, slice =25, number of excitation (NEX) =1, bandwidth =260 Hz/Px, flip angle =140°, acquisition time =79 s. Transverse and sagittal T2WI: TR =5,000 ms, TE =85 ms, FOV =260 mm × 260 mm, slice thickness =4 mm, slice gap =1.5 mm, slice =23, NEX =1, bandwidth =200 Hz/Px, flip angle =150°, acquisition time =88 s. Transverse DWI: TR =5,300 ms, TE =93 ms, FOV =260 mm × 260 mm, slice thickness =4 mm, slice gap =1.2, slice =23, b1 =0 s/mm2 and b2 =1,000 s/mm2, NEX =4, bandwidth =1,158 Hz/Px, acquisition time =101 s; transverse, sagittal and coronal 3D_VIBE_T1WI: TR =4.4 ms, TE =1.5 ms, FOV =240 mm × 280 mm, slice thickness =4mm, slice =40, NEX =1, bandwidth =400 Hz/Px, flip angle =9°, acquisition time =18 s. The type, number, location, diameter, and T2WI signal intensity of all fibroids were determined by MR images.
Evaluation of HIFU treatment efficacy and measurement of signal
The axial, sagittal, and coronal 3D_VIBE_T1WI MRI were taken within 24 hours following HIFU ablation, and the images were analyzed to assess the non-perfused volume (NPV). The MRI scanning protocol and parameters were the same as those used for the pre-treatment MRI. The NPV and fibroid volumes were determined from the 3D_VIBE_T1WI images by manually contouring the total non-perfused area and tumor area on each imaging slice. The NPV ratio (NPVR), defined as the NPV divided by the fibroid volume, was then calculated. We defined the higher ablation rate group was NPVR ≥80% [higher NPVR (h_NPVR)], and the lower ablation group was NPVR <80% [lower NPVR (l_NPVR)] (Figure 2) (21,22).
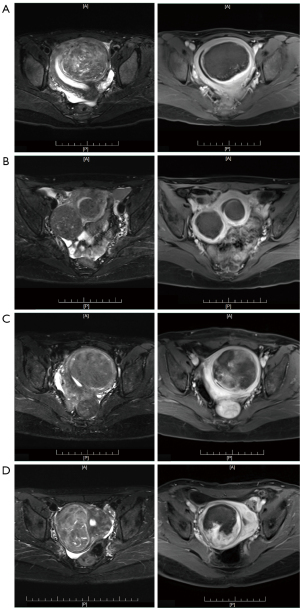
Pre-HIFU treatment T2WI images was used to measure the maximum diameter, T2WI signal intensity value, and to classify the fibroid type and location. The largest layer on the axial T2WI images was selected to measure the maximum diameter. Three consecutive layers of images were selected from the centre of fibroids, and the region of interest (ROI) was drawn along the edge of the fibroids and the rectus abdominis muscle of the same layer. Each measurement was carried out three times, and the average intensity value was taken as the final. T2WI signal intensity ratio = T2WI signal intensity value of fibroids/T2WI signal intensity value of rectus abdominis.
ROI segmentation and radiomics analysis
The fibroids (ROIs) in pre-treatment T2-weighted images and in post-treatment contrasted T1-weighted images were delineated by two radiologists together with more than 10 years’ experience. The inconsistent annotation was determined by the third senior radiologist. The delineation of ROIs in pre- and post-treatment images were separate and were done in different time. All of them did not know the results of NPVR, especially. The annotation tool is ITK-SNAP (v3.6.0, http://www.itksnap.org/pmwiki/pmwiki.php), which is an open-source software. The ROIs were recognized and outlined manually by the polygon model tool in ITK-SNAP based on the fibroids’ boundaries shown in the T2-weighted images slice by slice, and a 3D ROI was obtained for each subject, as shown in Figure 3. After manual annotation, the original images and the ROIs are resampled to the same resolution. Then, the radiomics analysis was performed based on fibroid regions by a clinical research platform (23), uAI Research Portal (uRP) (Shanghai United Imaging Intelligence, Shanghai, China) (Version:20220715). The main processing procedure includes feature extraction, feature selection and model construction to distinguish the ablation efficacy.
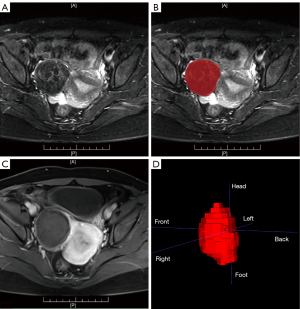
From each ROI, radiomics features was extracted based on the original and filtered T2-weighted images. The original features consist of 104 features, including 18 first-order features, 14 shape features, 21 gray-level co-occurrence matrix (GLCM) features, 14 gray-level dependence matrix (GLDM) features, 16 gray-level run-length matrix (GLRLM) features, 16 gray-level size-zone matrix (GLSZM) features and 5 neighboring gray-tone difference matrix (NGTDM) features. We applied 25 filters on original images, including log, mean, normalize, binomialblurimage, boxmean, boxsigmaimage, curvatureflow, laplaciansharpening, discretegaussian, recursivegaussian, shotnose, specklenoise, additive, Gaussian noise and wavelet. For wavelet decomposition, high- and low-pass filters were applied stepwise to the x, y, and z coordinates. As a result, a total of eight decompositions were obtained from one ROI, namely LLL, LLH, LHL, LHH, HLL, HLH, HHL, and HHH (L and H represent low- and high-pass filters respectively). Combining original and filtering features, 2,264 radiomics features were finally extracted from each ROI (Figure 4).
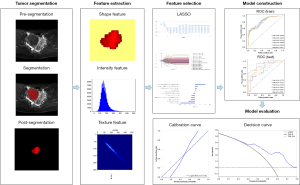
For feature selection, each feature was given the same priority and a pre-processing was applied with a Z-score normalization before using the least absolute shrinkage and selection operator (LASSO). LASSO is a type of linear regression method utilized for feature selection and regularization. It involves adding a penalty term to the most function of the linear regression model, which helps prevent overfitting by shrinking the coefficients towards zero. The LASSO parameter α was set to 0.05 to prevent the overfitting problem. The logistic regression classifier was applied to build the prediction model (Figure 5). The five-fold cross validation method was applied to effectively evaluate the prediction model.
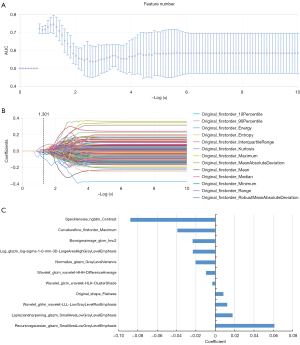
Data and statistical analysis
SPSS software (SPSS 18.0; SPSS Inc., Chicago, IL, USA) was used for statistical analysis. The Kolmogorov-Smirnov (K-S) statistical test was used to test for normality, data were described as the mean ± standard deviation (SD) for normally distributed data or median with a quartile range for non-normally distributed data. The Student’s t-test, Mann-Whitney test and Chi-square test were employed for data analysis. The receiver operating characteristic (ROC) curve, area under the curve (AUC), specificity, sensitivity and accuracy were used to evaluate the performance of the model. The closer the AUC was to 1, the better the identification ability. A P value less than 0.05 was considered statistically significant.
Results
General clinic data
Ninety-six patients (aged 40.16±6.71 years) with 108 fibroids were in h_NPVR group, among them one patient had three fibroids, ten patients had two fibroids, and the remaining patients had one fibroid. The mean maximum diameter was 6.07±1.76 cm (range, 3.48 to 11.62 cm). The subserosal, intramural and submucosal fibroids were 3, 60 and 45, respectively. Eighty-two patients (aged 40.43±6.46 years) with 92 fibroids were in l_NPVR group, among them one patient had three fibroids, eight patients had two fibroids, and the remaining patients had one fibroid. The mean maximum diameter was 6.44±2.06 cm (range, 3.82 to 12.01 cm). The subserosal, intramural and submucosal fibroids were 2, 70 and 20, respectively. There was no statistically significant difference for the patient age, fibroids maximum diameter, location and T2 signal intensity typing between the two groups (P=0.785, P=0.168, P=0.231, P=0.209), yet with statistically significant difference for the fibroids T2WI signal value and signal ratio as shown in Table 1 (P<0.001, P<0.001).
Table 1
Characteristics/parameters | h_NPVR (n=96) (NPVR ≥80%) | l_NPVR (n=82) (NPVR <80%) | t/χ2/Z value | P value |
---|---|---|---|---|
Age (years) | 40.16±6.71 (range, 25 to 49) |
40.43±6.46 (range, 25 to 48) |
t=−2.71 | 0.785 |
Maximum diameter (cm) | 6.07±1.76 (range, 3.48 to 11.62) |
6.44±2.06 (range, 3.82 to 12.01) |
t=−1.382 | 0.168 |
Fibroid type (cases) | 108 | 92 | χ2=9.365 | 0.009* |
Subserosal | 3 | 2 | – | – |
Intramural | 60 | 70 | – | – |
Submucosal | 45 | 20 | – | – |
Fibroid location (cases) | 108 | 92 | χ2=5.597 | 0.231 |
Anterior | 45 | 39 | – | – |
Right side | 25 | 14 | – | – |
Left side | 21 | 20 | – | – |
Bottom | 6 | 2 | – | – |
Posterior | 11 | 17 | – | – |
T2WI signal value | 206.75 (114.85) (range, 108.57 to 492.31) |
328.58 (216.39) (range, 136.92 to 902.46) |
Z=−6.268 | <0.001 |
T2WI signal ratio | 2.1 (1.11) (range, 0.93 to 8.39) |
3.27 (2.17) (range, 1.21 to 7.02) |
Z=−5.870 | <0.001 |
T2WI signal intensity (cases) | 108 | 92 | χ2=4.541 | 0.209 |
Hypointense | 69 | 47 | – | – |
Isointense | 26 | 30 | – | – |
Hybrid intense | 12 | 15 | – | – |
Hyperintense | 1 | 0 | – | – |
Procedure time (min) | 123.83±43.16 | 151.28±43.57 | t=−3.34 | 0.001* |
Sonication time (s) | 2,428 (1,201) | 3,467 (1,558) | Z=−3.888 | <0.001* |
Acoustic energy (kJ) | 564.570 (592.080) | 829.905 (671.962) | Z=−2.853 | 0.004* |
Average acoustic power (W) | 265.62±68.49 | 262.86±67.24 | t=0.21 | 0.831 |
Mean ± standard deviation for normally distributed data; median (quartile range) for non-normally distributed data. The largest layer on the axial T2WI images was selected to measure the maximum diameter. Three consecutive layers of images were selected from the centre of fibroids, and the region of interest was drawn along the edge of the fibroids and the rectus abdominis muscle of the same layer. Each measurement was carried out three times, and the average value was taken as the final value. T2WI signal ratio = T2WI signal value of fibroids/T2WI signal value of rectus abdominis. Procedure time = from the first sonication time to the last sonication, including the free time of sonication unworking. Sonication time = from the first sonication time to the last sonication, excluding the time of sonication unworking. *, P<0.05. h_NPVR, higher NPVR; l_NPVR, lower NPVR. USgHIFU, ultrasound-guided high-intensity focused ultrasound; NPVR, non-perfused volume ratio; T2WI, T2-weighted imaging.
The average procedure time was 123.83±43.16 and 151.28±43.57 minutes, median sonication time was 2,428 (1,201) and 3,467 (1,558) s, median acoustic energy was 564.570 (592.080) and 829.905 (671.962) kJ in the h_NPVR group and l_NPVR group, respectively (P=0.001, P=0.000, P=0.004). The average acoustic power was 265.62±68.49 and 262.86±67.24 W in the h_NPVR group and l_NPVR group (P=0.831) (Table 1).
Radiomics feature extraction, feature election and model construction
After feature selection, a total of 11 most significant features were selected by LASSO, including four GLSZM features, three GLCM features, one NGTDM feature, one GLRLM feature, one first-order feature and one shape feature. The selected feature name and the correlation coefficients are shown in Figure 5. Based on the 11 selected features, the logistic regression classifier was built for each fold and the performance for each fold was shown in Table 2. To summarize the overall performance, the mean AUC was 0.817 [95% confidence interval (CI): 0.755–0.882], for training set and 0.805 (95% CI: 0.670–0.941) for testing set, which showed a strong classification ability. The Delong test showed that there was no significant difference in the area under the ROC curve between the training set and testing set (as shown in Table 2 and Figure 6).
Table 2
Five-fold cross validation | Training | Testing | |||||||||||
---|---|---|---|---|---|---|---|---|---|---|---|---|---|
AUC (95% CI) | Sensitivity | Specificity | Accuracy | Precision | F1 score | AUC (95% CI) | Sensitivity | Specificity | Accuracy | Precision | F1 score | ||
1 | 0.824 (0.760–0.887) |
0.716 | 0.708 | 0.712 | 0.750 | 0.733 | 0.775 (0.632–0.919) |
0.682 | 0.579 | 0.634 | 0.652 | 0.667 | |
2 | 0.836 (0.775–0.896) |
0.727 | 0.712 | 0.720 | 0.753 | 0.74 | 0.727 (0.569–0.885) |
0.636 | 0.556 | 0.600 | 0.636 | 0.636 | |
3 | 0.819 (0.755–0.882) |
0.705 | 0.671 | 0.689 | 0.721 | 0.713 | 0.790 (0.650–0.930) |
0.636 | 0.667 | 0.650 | 0.700 | 0.667 | |
4 | 0.808 (0.744–0.873) |
0.716 | 0.658 | 0.689 | 0.716 | 0.716 | 0.861 (0.731–0.991) |
0.727 | 0.778 | 0.750 | 0.800 | 0.762 | |
5 | 0.804 (0.738–0.870) |
0.693 | 0.658 | 0.677 | 0.709 | 0.701 | 0.874 (0.767–0.98) |
0.773 | 0.778 | 0.775 | 0.810 | 0.791 | |
Mean | 0.817 (0.755–0.882) |
0.711 | 0.681 | 0.697 | 0.730 | 0.721 | 0.805 (0.670–0.941) |
0.691 | 0.671 | 0.682 | 0.720 | 0.704 | |
SD | 0.013 | 0.013 | 0.027 | 0.018 | 0.020 | 0.016 | 0.061 | 0.059 | 0.106 | 0.076 | 0.082 | 0.068 |
AUC, area under the curve; CI, confidence space; SD, standard deviation.
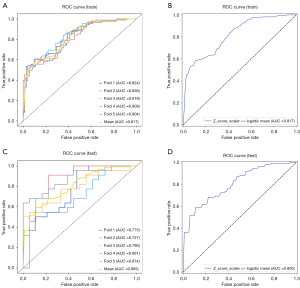
Verification of the prediction model
The calibration curves of the prediction model exhibited a high degree of consistency between the predicted outcomes and actual observations in both the training and test cohorts, as depicted in Figure 7A,7B. The decision curve analysis plots demonstrated that the model provided significant clinical net benefit in both the training and test cohorts, as shown in Figure 7C,7D.
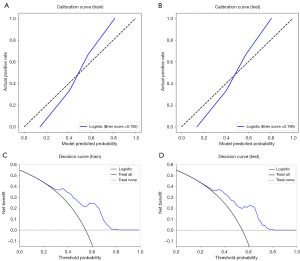
Discussion
The evaluation of NPVR by T1WI contrast-enhanced imaging has become an important indicator of the success of HIFU ablation of uterine fibroids, and the higher the NPVR, better the clinical efficacy. Sixty percent, 70%, 75%, 80%, or 90% of the NPVR have been set as the criteria for technical success in different studies (24-26). Park et al. (27) reported that an immediate NPVR of at least 80% was safe in USgHIFU ablation of uterine fibroids, which could achieve a greater tumor volume shrinkage compared with lower NPVR. While Mindjuk et al. (28) found that the NPVR results of more than 80% were correlated with higher clinical success rates, Bitton et al. (29) considered >80% of the NPVR as the image biomarker for the largest improvement in symptoms after HIFU treatment. Therefore, the NPVR 80% was chosen as the criterion for separating l_NPVR from h_NPVR in our study (30).
A series of MRI parameters, such as T2 signal intensity, DWI apparent diffusion coefficient (ADC) value, Ktrans (31), etc., have been employed for predicting the efficacy of HIFU ablation (30,32). Previous studies showed that T2 signal intensity of uterine fibroids was negatively correlated with the NPVR of focused ultrasound ablation (9,30,33). Different from International Federation of Gynecology and Obstetrics (FIGO) classification system (33), which emphasizes the relationship between the fibroids and the adjacent mucosa, muscular and serosal layer, the Funaki classification (9) pays more attention to the MRI T2 signal intensity of fibroids. Funaki et al. (9) classified the T2 signals of uterine fibroids into three types: hypointensity, iso-intensity and hyperintensity. And they reported the hypointense signal type could achieve the best ablation effect; the isointense signal type had relatively satisfactory ablation efficacy and potential recurrence; whereas hyperintense signal type had the worst ablation effect. However, in clinical practices the high NPVR could be achieved in some of T2 hyperintense fibroids, whereas some of T2 hypo- or iso-intense fibroids did not have good ablation effect after focused ultrasound therapy (34). The fibroids with T2 hypointense or isointense signal are mainly composed of collagen fibrous tissue with insufficient blood supply, which would result in more deposition of acoustic energy (7,33). The fibroids with T2WI hybrid intense signal usually indicate complex pathological changes, such as cystic, necrosis and calcification (33,35). The content of water, representing T2 hyperintense signal, in the degenerated uterine fibroids makes it easy for focused ultrasound energy to penetrate the focused region (36,37). Although the blood flow of fibroid has heat-sink effect on thermal ablation in radiofrequency therapy, the perfusion has only a small impact on the thermal dose volume during HIFU procedure in comparison with ultrasound absorption because of a shorter time of sonication (38). Our previous data showed that the T2 signal intensity of fibroids with a poor blood supply were lower than those of the fibroids with a rich blood supply (30). The T2 signal was related to the variation of cell density, collagen fiber content, blood supply types and water content of the tumors (31,39), however its intensity could not accurately reflect the complex texture of uterine fibroids. A single qualitative classification based on T2 signal intensity could not accurately evaluate the heterogeneous conditions, although it is still considered as primary imaging biomarker to predict the efficacy of HIFU ablation in treatment of uterine fibroids (29). The key issue should be addressed to precisely select the patients suitable to HIFU ablation of the uterine fibroids before treatment for clinically curative response.
The radiomics studies has shown its advantages on the differential diagnosis of uterine fibroids (40-42). Kurban et al. (41) collected 75 patients with 212 uterine fibroids who were successfully treated by uterine artery embolization (UAE) and developed a radiomics model of treatment response to UAE. A five-variable predictive ROC model was developed to evaluate the diagnostic accuracy of the signal intensity ratio and heterogeneity ratio on T2-weighted MRI, contrast enhancement ratio on T1-weighted MRI, location, and baseline fibroid volume. The five-variable ROC model showed high diagnostic accuracy with an AUC of 0.85, sensitivity of 82%, and specificity of 71%. Lakhman et al. (42) reported that T2WI based radiomics was feasible in distinguishing leiomyosarcoma (LMS) and atypical uterine fibroids. Thus, the radiomics analysis of T2 signal could quantify and figure out the overlooked but potentially valuable texture features of fibroids through a large number of imaging information. Radiomics models are superior to conventional clinical models for the tumor characteristic analysis (21), which would probably be helpful for HIFU ablation efficacy prediction, since there was no statistically significant difference in T2WI signal intensity typing between the h_NPVR group and the l_NPVR group in our study (P=0.209) (Table 1).
Our study selected a total of 11 valuable radiomics features based on T2WI, including four grayscale region matrix (GLSZM) features, three grayscale co-occurrence matrix (GLCM) features, one neighborhood grayscale difference matrix (NGTDM) feature, and one grayscale run matrix (GLRLM) feature, with only one first-order feature and one shape feature (Figure 5). Wei et al. (20) extracted 20 sets of 745 quantitative features from preprocessed T2WI images and evaluated the correlation between NPVR and the radiomics features of uterine fibroids using a multiple linear regression model. The final regression model R2 for NPVR was found to be 0.422, and the independent and valuable parameter was the size zone non-uniformity (SZN) parameter in the grayscale region matrix (GLSZM). The larger the SZN parameter value, the greater the heterogeneity of the image. In our study, both the glszm_smallerarealowgraylevelemphasis and ngtdm_contrast features showed the best significance at the time of modeling in the LASSO feature selection results. The small area low gray level emphasis (SALGLE) obtained from the grayscale size region matrix (GLSZM), measures the proportion in the image of the joint distribution of smaller size zones with lower gray-level values. The GLSZM quantifies the clusters of homogeneous intensity regions within the tumor (43). The ngtdm_contrast is one of the features of the neighborhood gray tone difference matrix (NGTDM) group. NGTDM, as a higher-order statistical parameter, detects the signal intensity and spatial correlation between adjacent voxels and describes the dynamic range of intensity at the local level, which is better for quantifying the heterogeneity within the ROI (44). In our research, both the GLSZM and NGTDM features were significantly correlated with NPVR of fibroids. These results indicated that higher-order feature parameters had higher overall effectiveness in predicting HIFU efficacy than first-order features and shape features.
In our study, there was no statistically significant difference in the maximum diameter, location and T2 signal intensity typing of fibroids between the h_NPVR group and l_NPVR group (P>0.05), and the acoustic power were very close between the two groups (265.62±68.49 vs. 262.86±67.24 W) (P>0.05). The procedure time, sonication time and acoustic energy in the h_NPVR group were lower than that of the l_NPVR group, respectively (P<0.05). These results are consistent with many previous reports (9,11,20,45). The results of our study showed that the eleven radiomics characteristic of T2 signal of fibroids are effective indicators for predicting the HIFU efficacy (Figure 5), and the radiomics model constructed based on this had the good application value in both of the training set (AUC =0.817, sensitivity of 71.1%, and specificity of 68.1%) and the testing set (AUC =0.805, sensitivity of 69.1%, and specificity of 67.1%) (Figure 6 and Table 2). The Delong test showed that there was no significant difference in the area under the ROC curve between the training set and testing set (Figure 6 and Table 2). Wang (21) developed a machine learning model for prediction of malignancy in T2 hyperintense mesenchymal uterine tumors based on T2WI features and clinical information, and the results showed that comparing with the T2WI-based radiomics model (AUC =0.76) and the clinical model (AUC =0.79), the combined model significantly improved the AUC value to 0.9. Zheng (37) enrolled 205 patients with uterine fibroids treated by HIFU, and extract radiomics features from T2-weighted images and ADC, and combined the selected radiomics features and clinical parameters to established the clinical-radiological model, radiomics model, and radiomics-clinical model. The results showed that the multi-parametric MRI-based radiomics model outperformed T2WI-based radiomics model, which achieved an average AUC of 0.769, and showed satisfactory prediction performance for NPVR classification. The radiomics-clinical model demonstrated best prediction performance for HIFU treatment outcome, with an average AUC of 0.802 and an accuracy of 0.762 in the testing cohort. These studies had showed that the radiomics was of great value for identifying the malignant tumor and assessing HIFU ablation efficacy of the fibroids. Without considering the influence of clinical and other radiological parameters on the results, our study demonstrated that T2WI-based radiomics model had the positive outcome in predicting the HIFU ablation efficacy of uterine fibroids.
The limitations of our study are: (I) a relatively small sample size from one medical center, and there might be bias in patient selection; (II) only T2WI images was leveraged, thus the feature extraction was not comprehensively enough; (III) some laboratory parameters such as luteinizing hormone, follicle stimulating hormone and serum estradiol were not evaluated; (IV) lack of validating application of different MRI scanning parameters from the other vendors in this radiomics model. Therefore, it is expected that the multi-center clinical trial with large samples will be performed in the future, and a more comprehensive and perfect prediction model can be established based on multi-parameter MRI and laboratory parameters. In conclusion, our preliminary study demonstrated that radiomics model based on T2WI, using the high-order textural features instead of the first-order and shape features, had a good effectiveness in predicting the efficacy of HIFU ablation in the treatment of uterine fibroids with higher coefficients, which would be helpful for physicians to develop appropriate strategy of HIFU ablation.
Conclusions
Our study showed that the radiomics model based on T2WI is feasible and effective for predicting the efficacy of HIFU ablation in treatment of uterine fibroids. The preoperative radiomics evaluation can potentially be used to screen the uterine fibroids with high ablation rate for improving HIFU postoperative efficacy.
Acknowledgments
We thank Dr. Wu Shun Felix Wong from School of Women’s and Children’s Health, The University of New South Wales, NSW, Australia, for language editing of the manuscript.
Funding: This work was supported by
Footnote
Reporting Checklist: The authors have completed the TRIPOD reporting checklist. Available at https://qims.amegroups.com/article/view/10.21037/qims-23-916/rc
Conflicts of Interest: All authors have completed the ICMJE uniform disclosure form (available at https://qims.amegroups.com/article/view/10.21037/qims-23-916/coif). X.P., L.C. and X.C. are full-time employees of Shanghai United Imaging Intelligence Co., Ltd. The other authors have no conflicts of interest to declare.
Ethical Statement: The authors are accountable for all aspects of the work in ensuring that questions related to the accuracy or integrity of any part of the work are appropriately investigated and resolved. The study was conducted in accordance with the Declaration of Helsinki (as revised in 2013). The study was approved by the Ethics Committee of Shanghai Xuhui Central Hospital (No. 2019-094) and individual consent for this retrospective analysis was waived.
Open Access Statement: This is an Open Access article distributed in accordance with the Creative Commons Attribution-NonCommercial-NoDerivs 4.0 International License (CC BY-NC-ND 4.0), which permits the non-commercial replication and distribution of the article with the strict proviso that no changes or edits are made and the original work is properly cited (including links to both the formal publication through the relevant DOI and the license). See: https://creativecommons.org/licenses/by-nc-nd/4.0/.
References
- Wang Y, Xu Y, Wong F, Wang Y, Cheng Y, Yang L. Preliminary study on ultrasound-guided high-intensity focused ultrasound ablation for treatment of broad ligament uterine fibroids. Int J Hyperthermia 2021;38:18-23. [Crossref] [PubMed]
- Lee JS, Hong GY, Lee KH, Song JH, Kim TE. Safety and Efficacy of Ultrasound-Guided High-Intensity Focused Ultrasound Treatment for Uterine Fibroids and Adenomyosis. Ultrasound Med Biol 2019;45:3214-21. [Crossref] [PubMed]
- Stewart EA. Clinical practice. Uterine fibroids. N Engl J Med 2015;372:1646-55. [Crossref] [PubMed]
- Liu L, Wang T, Lei B. High-intensity focused ultrasound (HIFU) ablation versus surgical interventions for the treatment of symptomatic uterine fibroids: a meta-analysis. Eur Radiol 2022;32:1195-204. [Crossref] [PubMed]
- Bachu VS, Kedda J, Suk I, Green JJ, Tyler B. High-Intensity Focused Ultrasound: A Review of Mechanisms and Clinical Applications. Ann Biomed Eng 2021;49:1975-91. [Crossref] [PubMed]
- Yang T, Chen Q, Kuang L, Fu Z, Wang Y, Chen Y, Yang L, Xu Y. Effectiveness and safety of ultrasound-guided high-intensity focused ultrasound ablation for the treatment of colorectal cancer liver metastases. Int J Hyperthermia 2022;39:829-34. [Crossref] [PubMed]
- Xu Y, Fu Z, Yang L, Huang Z, Chen WZ, Wang Z. Feasibility, Safety, and Efficacy of Accurate Uterine Fibroid Ablation Using Magnetic Resonance Imaging-Guided High-Intensity Focused Ultrasound With Shot Sonication. J Ultrasound Med 2015;34:2293-303. [Crossref] [PubMed]
- Wang Y, Geng J, Bao H, Dong J, Shi J, Xi Q. Comparative Effectiveness and Safety of High-Intensity Focused Ultrasound for Uterine Fibroids: A Systematic Review and Meta-Analysis. Front Oncol 2021;11:600800. [Crossref] [PubMed]
- Funaki K, Fukunishi H, Funaki T, Sawada K, Kaji Y, Maruo T. Magnetic resonance-guided focused ultrasound surgery for uterine fibroids: relationship between the therapeutic effects and signal intensity of preexisting T2-weighted magnetic resonance images. Am J Obstet Gynecol 2007;196:184.e1-6. [Crossref] [PubMed]
- Sainio T, Saunavaara J, Komar G, Mattila S, Otonkoski S, Joronen K, Perheentupa A, Blanco Sequeiros R. Feasibility of apparent diffusion coefficient in predicting the technical outcome of MR-guided high-intensity focused ultrasound treatment of uterine fibroids - a comparison with the Funaki classification. Int J Hyperthermia 2021;38:85-94. [Crossref] [PubMed]
- Fan HJ, Zhang C, Lei HT, Cun JP, Zhao W, Huang JQ, Zhai Y. Ultrasound-guided high-intensity focused ultrasound in the treatment of uterine fibroids. Medicine (Baltimore) 2019;98:e14566. [Crossref] [PubMed]
- Lambin P, Rios-Velazquez E, Leijenaar R, Carvalho S, van Stiphout RG, Granton P, Zegers CM, Gillies R, Boellard R, Dekker A, Aerts HJ. Radiomics: extracting more information from medical images using advanced feature analysis. Eur J Cancer 2012;48:441-6. [Crossref] [PubMed]
- Gillies RJ, Kinahan PE, Hricak H. Radiomics: Images Are More than Pictures, They Are Data. Radiology 2016;278:563-77. [Crossref] [PubMed]
- Suomi V, Komar G, Sainio T, Joronen K, Perheentupa A, Blanco Sequeiros R. Comprehensive feature selection for classifying the treatment outcome of high-intensity ultrasound therapy in uterine fibroids. Sci Rep 2019;9:10907. [Crossref] [PubMed]
- Qin S, Jiang Y, Wang F, Tang L, Huang X. Development and validation of a combined model based on dual-sequence MRI radiomics for predicting the efficacy of high-intensity focused ultrasound ablation for hysteromyoma. Int J Hyperthermia 2023;40:2149862. [Crossref] [PubMed]
- Kumar V, Gu Y, Basu S, Berglund A, Eschrich SA, Schabath MB, Forster K, Aerts HJ, Dekker A, Fenstermacher D, Goldgof DB, Hall LO, Lambin P, Balagurunathan Y, Gatenby RA, Gillies RJ. Radiomics: the process and the challenges. Magn Reson Imaging 2012;30:1234-48. [Crossref] [PubMed]
- Zhou Y, Zhang J, Chen J, Yang C, Gong C, Li C, Li F. Prediction using T2-weighted magnetic resonance imaging-based radiomics of residual uterine myoma regrowth after high-intensity focused ultrasound ablation. Ultrasound Obstet Gynecol 2022;60:681-92. [Crossref] [PubMed]
- An C, Kim DW, Park YN, Chung YE, Rhee H, Kim MJ. Single Hepatocellular Carcinoma: Preoperative MR Imaging to Predict Early Recurrence after Curative Resection. Radiology 2015;276:433-43. [Crossref] [PubMed]
- Li Z, Zhang J, Song Y, Yin X, Chen A, Tang N, Prince MR, Yang G, Wang H. Utilization of radiomics to predict long-term outcome of magnetic resonance-guided focused ultrasound ablation therapy in adenomyosis. Eur Radiol 2021;31:392-402. [Crossref] [PubMed]
- Wei C, Li N, Shi B, Wang C, Wu Y, Lin T, Chen Y, Ge Y, Yu Y, Dong J. The predictive value of conventional MRI combined with radiomics in the immediate ablation rate of HIFU treatment for uterine fibroids. Int J Hyperthermia 2022;39:475-84. [Crossref] [PubMed]
- Wang T, Gong J, Li Q, Chu C, Shen W, Peng W, Gu Y, Li W. A combined radiomics and clinical variables model for prediction of malignancy in T2 hyperintense uterine mesenchymal tumors on MRI. Eur Radiol 2021;31:6125-35. [Crossref] [PubMed]
- Gong X, Zhang X, Liu D, Yang C, Zhang R, Xiao Z, Chen W, Chen J. Evaluation of physician experience in achieving non-perfused volume ratio of high-intensity focused ultrasound ablation for uterine fibroids: a multicentre study. J Int Med Res 2022;50:3000605221102087. [Crossref] [PubMed]
- Wu J, Xia Y, Wang X, Wei Y, Liu A, Innanje A, Zheng M, Chen L, Shi J, Wang L, Zhan Y, Zhou XS, Xue Z, Shi F, Shen D. uRP: An integrated research platform for one-stop analysis of medical images. Front Radiol 2023;3:1153784. [Crossref] [PubMed]
- Chen J, Li Y, Wang Z, McCulloch P, Hu L, Chen W, Liu G, Li J, Lang JCommittee of the Clinical Trial of HIFU versus Surgical Treatment for Fibroids. Evaluation of high-intensity focused ultrasound ablation for uterine fibroids: an IDEAL prospective exploration study. BJOG 2018;125:354-64. [Crossref] [PubMed]
- Cheung VYT. High-intensity focused ultrasound therapy. Best Pract Res Clin Obstet Gynaecol 2018;46:74-83. [Crossref] [PubMed]
- Froeling V, Meckelburg K, Schreiter NF, Scheurig-Muenkler C, Kamp J, Maurer MH, Beck A, Hamm B, Kroencke TJ. Outcome of uterine artery embolization versus MR-guided high-intensity focused ultrasound treatment for uterine fibroids: long-term results. Eur J Radiol 2013;82:2265-9. [Crossref] [PubMed]
- Park MJ, Kim YS, Rhim H, Lim HK. Safety and therapeutic efficacy of complete or near-complete ablation of symptomatic uterine fibroid tumors by MR imaging-guided high-intensity focused US therapy. J Vasc Interv Radiol 2014;25:231-9. [Crossref] [PubMed]
- Mindjuk I, Trumm CG, Herzog P, Stahl R, Matzko M. MRI predictors of clinical success in MR-guided focused ultrasound (MRgFUS) treatments of uterine fibroids: results from a single centre. Eur Radiol 2015;25:1317-28. [Crossref] [PubMed]
- Bitton RR, Fast A, Vu KN, Lum DA, Chen B, Hesley GK, Raman SS, Matsumoto AH, Price TM, Tempany C, Dhawan N, Dolen E, Kohi M, Fennessey FM, Ghanouni P. What predicts durable symptom relief of uterine fibroids treated with MRI-guided focused ultrasound? A multicenter trial in 8 academic centers. Eur Radiol 2023;33:7360-70. [Crossref] [PubMed]
- Liao L, Xu YH, Bai J, Zhan P, Zhou J, Li MX, Zhang Y. MRI parameters for predicting the effect of ultrasound-guided high-intensity focused ultrasound in the ablation of uterine fibroids. Clin Radiol 2023;78:61-9. [Crossref] [PubMed]
- Li C, Jin C, Liang T, Li X, Wang R, Zhang Y, Yang J. Magnetic resonance-guided high-intensity focused ultrasound of uterine fibroids: whole-tumor quantitative perfusion for prediction of immediate ablation response. Acta Radiol 2020;61:1125-33. [Crossref] [PubMed]
- Kim YS, Lim HK, Park MJ, Rhim H, Jung SH, Sohn I, Kim TJ, Keserci B. Screening Magnetic Resonance Imaging-Based Prediction Model for Assessing Immediate Therapeutic Response to Magnetic Resonance Imaging-Guided High-Intensity Focused Ultrasound Ablation of Uterine Fibroids. Invest Radiol 2016;51:15-24. [Crossref] [PubMed]
- Zhao WP, Zhang J, Han ZY, Yao JP, Zhou X, Liang P. A clinical investigation treating different types of fibroids identified by MRI-T2WI imaging with ultrasound guided high intensity focused ultrasound. Sci Rep 2017;7:10812. [Crossref] [PubMed]
- Zhao WP, Chen JY, Zhang L, Li Q, Qin J, Peng S, Li KQ, Wang ZB, Chen WZ. Feasibility of ultrasound-guided high intensity focused ultrasound ablating uterine fibroids with hyperintense on T2-weighted MR imaging. Eur J Radiol 2013;82:e43-9. [Crossref] [PubMed]
- Munro MG, Critchley HO, Broder MS, Fraser ISFIGO Working Group on Menstrual Disorders. FIGO classification system (PALM-COEIN) for causes of abnormal uterine bleeding in nongravid women of reproductive age. Int J Gynaecol Obstet 2011;113:3-13. [Crossref] [PubMed]
- Jiang L, Yu JW, Yang MJ, Zhong Q, Chen JY. Ultrasound-guided HIFU for uterine fibroids of hyperintense on T2-weighted MR imaging with or without GnRH-analogue-pretreated: A propensity score matched cohort study. Front Surg 2022;9:975839. [Crossref] [PubMed]
- Zheng Y, Chen L, Liu M, Wu J, Yu R, Lv F. Nonenhanced MRI-based radiomics model for preoperative prediction of nonperfused volume ratio for high-intensity focused ultrasound ablation of uterine leiomyomas. Int J Hyperthermia 2021;38:1349-58. [Crossref] [PubMed]
- Hyvärinen M, Huang Y, David E, Hynynen K. Comparison of computer simulations and clinical treatment results of magnetic resonance-guided focused ultrasound surgery (MRgFUS) of uterine fibroids. Med Phys 2022;49:2101-19. [Crossref] [PubMed]
- Oguchi O, Mori A, Kobayashi Y, Horiuchi A, Nikaido T, Fujii S. Prediction of histopathologic features and proliferative activity of uterine leiomyoma by magnetic resonance imaging prior to GnRH analogue therapy: correlation between T2-weighted images and effect of GnRH analogue. J Obstet Gynaecol (Tokyo 1995) 1995;21:107-17.
- Malek M, Tabibian E, Rahimi Dehgolan M, Rahmani M, Akhavan S, Sheikh Hasani S, Nili F, Hashemi H. A Diagnostic Algorithm using Multi-parametric MRI to Differentiate Benign from Malignant Myometrial Tumors: Machine-Learning Method. Sci Rep 2020;10:7404. [Crossref] [PubMed]
- Kurban LAS, Metwally H, Abdullah M, Kerban A, Oulhaj A, Alkoteesh JA. Uterine Artery Embolization of Uterine Leiomyomas: Predictive MRI Features of Volumetric Response. AJR Am J Roentgenol 2021;216:967-74. [Crossref] [PubMed]
- Lakhman Y, Veeraraghavan H, Chaim J, Feier D, Goldman DA, Moskowitz CS, Nougaret S, Sosa RE, Vargas HA, Soslow RA, Abu-Rustum NR, Hricak H, Sala E. Differentiation of Uterine Leiomyosarcoma from Atypical Leiomyoma: Diagnostic Accuracy of Qualitative MR Imaging Features and Feasibility of Texture Analysis. Eur Radiol 2017;27:2903-15. [Crossref] [PubMed]
- Jajodia A, Gupta A, Prosch H, Mayerhoefer M, Mitra S, Pasricha S, Mehta A, Puri S, Chaturvedi A. Combination of Radiomics and Machine Learning with Diffusion-Weighted MR Imaging for Clinical Outcome Prognostication in Cervical Cancer. Tomography 2021;7:344-57. [Crossref] [PubMed]
- Gourtsoyianni S, Doumou G, Prezzi D, Taylor B, Stirling JJ, Taylor NJ, Siddique M, Cook GJR, Glynne-Jones R, Goh V. Primary Rectal Cancer: Repeatability of Global and Local-Regional MR Imaging Texture Features. Radiology 2017;284:552-61. [Crossref] [PubMed]
- Peng S, Zhang L, Hu L, Chen J, Ju J, Wang X, Zhang R, Wang Z, Chen W. Factors influencing the dosimetry for high-intensity focused ultrasound ablation of uterine fibroids: a retrospective study. Medicine (Baltimore) 2015;94:e650. [Crossref] [PubMed]