A narrative review of deep learning in thyroid imaging: current progress and future prospects
Introduction
Thyroid diseases can be subclinical or present with structural (goiters, nodules, and cancer) or functional (hypothyroidism, hyperthyroidism) symptoms (1). Functional thyroid disorders include Graves disease and lymphocytic (Hashimoto) thyroiditis, which can lead to hyperthyroidism or hypothyroidism, respectively, with Graves disease accounting for 60–80% of hyperthyroidism and Hashimoto thyroiditis being the primary cause of hypothyroidism (2). Neoplastic thyroid conditions, such as cysts, adenomas, and multinodular goiter, can be further categorized into benign and malignant types. Thyroid nodules are present in about 5% population: most of these are benign and commonly include colloid nodules, cysts, nodular thyroiditis, or benign neoplasm, whereas about 5% are malignant (3). Thyroid cancer is the ninth most common disease in the world, with 586,000 cases reported in 2020 (4), while thyroid functional disorders affect more than 300 million people worldwide (5).
Several examinations are typically carried out to assess the thyroid, including ultrasound (US), computed tomography (CT), magnetic resonance imaging (MRI), and thyroid scintigraphy (6). Thyroid US is recommended to evaluate the size of a goiter or to classify the malignant risk of thyroid nodules and is often followed by fine-needle aspiration biopsy (FNAB) to support the diagnosis (7). Thyroid examination typically relies on gray-scale imaging and color Doppler flow imaging (CDFI), supplemented by more advanced technologies such as US elastography (USE) and contrast-enhanced US (CEUS) (8,9). CT and MRI thyroid scans are mainly used for surveillance and investigation of lesion metastasis, as they provide high-resolution imaging that can highlight the invasion of critical structures such as the neck blood vessels, trachea, and esophagus (10). Thyroid scintigraphy can reveal alterations in blood flow, function, and metabolism of organs or lesions (11). Imaging systems process these scanned images, allowing radiologists to quantify and measure them. The quantitative and qualitative features of images provide information for disease prediction and diagnosis, but there is often overlap in imaging features between different thyroid diseases (6). Moreover, medical imaging analysis is highly dependent on skill and experience, particularly under conditions of a heavy workload, and errors are therefore inevitable. Furthermore, there remains uncertainty in managing thyroid disease, such as whether subtle changes in thyroid function and structure actually indicate a disease requiring treatment, and this uncertainty can lead to misdiagnosis, overdiagnosis, and inappropriate treatment (12).
Deep learning (DL) is a subfield of artificial intelligence (AI) involving the computational architecture of interconnected nodes as inspired by biological neural networks (13). In recent years, DL has risen to prominence in medical imaging due to the increase of computational power and the availability of large-scale datasets (14). DL has been extensively used for image analysis in the radiology and digital pathology domains, including for the imaging of the brain, liver, thorax, and breast (15). DL is data-driven, and provided sufficient image pixels and corresponding medical image labels, it can automatically extract deep features from images that are indiscernible to human experts (16). Developments in DL have the potential to further improve diagnostic precision and workflows (12), and a review of the evidence, limitations, and future prospects related to DL in thyroid disease imaging is provided in this paper.
The aim of this review is to create a much-needed reference concerning the latest developments in the research of DL for thyroid imaging and its potential integration into clinical practice. This paper discusses key aspects ranging from model construction to actual clinical applications, highlighting new opportunities for building DL models based on practical needs. The majority of the articles in this review effectively discuss and elaborate upon the experimental design and construction of DL models. Nonetheless, a small portion of the articles reviewed are vague in their description of the clinical trial component, resulting in a lack of detailed information in assessment of study design according to the Population, Intervention, Control, Outcome, Study Design (PICOS) approach. We present this article in accordance with the Narrative Review reporting checklist (available at https://qims.amegroups.com/article/view/10.21037/qims-23-908/rc).
Methods
We searched PubMed, Web of Science, and Google Scholar for literature on DL applications in thyroid imaging published between April 2018 and April 2023. The following search terms were used: artificial intelligence or deep learning, thyroid diseases, and thyroid nodule or thyroid carcinoma. The inclusion criteria were as follows: (I) a discussion of models based on DL; (II) a focus on at least one thyroid imaging method; and (III) English-language articles. Meanwhile, reviews, letters, editorials, comments, case reports, and unpublished articles (unavailable full texts and preprints) were excluded (Table 1).
Table 1
Items | Specification |
---|---|
Date of search | Initial search time 30/04/2023, update on 25/09/2023 |
Databases and other sources searched | PubMed, Web of Science, and Google Scholar |
Search terms used | MeSH: artificial intelligence or deep learning, thyroid diseases, thyroid nodule or thyroid carcinoma |
Timeframe | April 2018 to September 2023 |
Inclusion and exclusion criteria | Inclusion criteria: (I) models based on deep learning; (II) a focus on at least 1 thyroid imaging method; (III) articles published in English |
Exclusion criteria: reviews, letters, editorials, comments, case reports, and unpublished articles (full text unavailable and preprints) | |
Selection process | Two reviewers (W.T.Y. and Y.C.) independently performed abstract and full-text screening as well as data collection; any discrepancies were resolved via consensus |
Overview of DL
The term deep learning describes a class of machine learning techniques based on artificial neural networks (ANNs) where deep represents the numerous hidden layers of neurons between the input and output layers (14). Various types of deep architecture can be obtained through the combination of different layer types including fully connected (FC), convolution, and pooling layers in a deep structure or by considering the directed, undirected, or recurrent connections between layers (17). The achievements of DL with convolutional neural networks (CNNs) for spatial data, recurrent neural networks (RNNs) for temporal data, and generative adversarial networks (GANs) for generated data in the medical imaging domains have heightened the expectations of and research into intelligent image analysis (Figure 1).
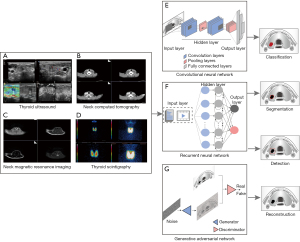
CNNs
A CNNs is a type of DL algorithm designed to process data that exhibit natural spatial invariance, particularly in those images whose meanings do not change under translation (14). CNNs include an input layer, an output layer, and hidden learning layers, which in most cases consist of convolutional and pooling sublayers. The convolutional and pooling layers act as feature extractors from the input image, while the FC layer acts as a classifier to output results (15,18).
After the superior performance of AlexNet was first demonstrated (19) on the ImageNet dataset in 2012, CNN-based image processing and computer vision tasks have come to the fore. Since AlexNet was proposed, researchers developed a variety of deeper, wider, and lighter CNN models. Subsequently, deeper network structures emerged, such as visual geometry group network (VGGNet) (20) and GoogLeNet (21), which have significantly improved the accuracy in classification tasks. The VGGNet increased image recognition accuracy by deepening the network depth to 19 layers. In the same year, GoogLeNet introduced the inception module, an innovative concept which involved several parallel convolution routes for extracting features at different spatial scales. Residual network (ResNet) (22) and dense convolutional network (DenseNet) (23) overcame the challenge of gradient disappearance and reduced the risk of overfitting by introducing the concept of skipping connections and by significantly increasing the depth of the network. The creation of inception network represented a breakthrough in developing CNN classifiers. With the inception network, an optimal sparse design in a local convolutional vision framework can be made reliable with the use of dense components that are readily accessible (24). In the segmentation task of CNN structures, the concept of fully convolutional networks was proposed to image semantic segmentation (25), and U-Net, with more multiscale features, was applied more frequently to medical image segmentation tasks (26). The approaches of object detection can be divided into two-stage approaches, such as region CNN (R-CNN) (27), and one-stage approaches, such as you only look once (YOLO) (28) and single-shot multibox detector (SSD) (29). We discuss the segmentation and detection tasks in detail in subsequent sections. Many new-generation networks have attracted the interest of researchers, including MobileNetV3, Inception-v4, and ShuffleNet series. Generally, the completion of medical imaging tasks currently involves all of the above architectures as the main backbone or the construction of new DL architectures.
RNNs
An RNN is a neural network that processes sequential information while maintaining a state vector within its hidden neurons (30). CNNs extract spatial features well, while RNNs are more suited to identifying temporal features (31). RNNs are useful tools in the processing of time-series data such as video, language, and speech. Each two-dimensional image frame in the video and the order of each frame (temporal features) are important. There are two popular types of RNN, long short-term memory (LSTM) and gated recurrent unit (GRU). The structure of LSTM allows it to contain information over a long duration, solving tasks that are challenging or impossible to do with traditional RNNs (32). GRU, as a simplified version of the LSTM, requires less memory, meaning that larger volumes can be fed into the network and larger networks can be designed for the same volume size (33). In image description generation, the encoder-decoder framework of RNN involves “translating” the semantic feature vector of the image into the text sequence (34). Furthermore, RNNs have the ability to gather time-series data over multiple time points, making them more sensitive to early pathological changes. Analysis of this longitudinal data can help monitor disease progression (15).
GANs
A GAN sets two networks against each other, with the generator network generating synthetic data from exemplars provided during training and the discriminator network evaluating the agreement between the generated and original data (35). The objective of a GAN is to reduce the degree of classification error of the second network, which results in the generation of images that more closely resemble the original (36-38). GANs have been used to perform traditional tasks such as classification, detection, and segmentation, in addition to synthesis, reconstruction, and image registration (39).
The strength of GANs lies in their ability to learn in an unsupervised or weakly supervised manner (40). Similar to other DL neural networks, the demonstrated applications of GANs have directly improved radiology workflow and patient management. Medical imaging has benefited from the generative aspect of GANs, which can help explore and discover the underlying structure of training data and learn to generate new images (41). This provides several applications, including augmentation of training data and image quality and resolution improvement (42). Generally speaking, GANs can be used for multimodal image fusion; for example, CNNs can obtain the required different imaging modal features, and information obtained from CNNs can be combined by GANs in some manner to make a clinical decision (35). Yang et al. proposed the dual-path semisupervised GAN for thyroid classification, integrating US B-mode images and the USE images (43); other proposals employed the fusion multimodal method based on GANs for thyroid nodule diagnosis (44,45).
Medical imaging tasks
Computer vision tasks for the thyroid field are common applications in the field of radiology and include classification (43%), segmentation (29%), detection (19%), and reconstruction (9%) (18,45). Building an appropriate model for a task or a multitask learning model can improve the overall performance.
Classification
Classification is a fundamental part of medical image analysis and typically involves the processing of images or videos as input data, in which the model assigns weights and biases to different input features and then distinguishes them from each other (39). CNNs represent a breakthrough in this field. In the construction of classification model, selecting the activation function, loss function, and optimizer, along with tuning many other hyperparameters, greatly impacts model performance (46). Within a deep neural network, lies an activation function positioned between two layers. The last layer employs commonly used activation functions for classification, such as sigmoid for binary tasks and softmax for multiclassification tasks (47). Loss functions can be paired with CNNs to address both regression and classification issues, including mean absolute error, mean square error, cross entropy, and others (48). Optimizers are used during training to reduce the loss function and achieve optimal network parameters in a reasonable time; an example of this is gradient descent methods often being used to train CNN models (49). Several studies have used classical models such as ResNet, DenseNet, AlexNet, and VGGNet to complete tasks, from determining the presence or absence of disease to identifying malignancies (50). In addition to the previously mentioned CNN models, recent research has also proposed using the existing structures as the backbone coupled with different modifications for training. In one study, the DenseNet architecture was modified via addition of trainable weight parameters to each skip connection (51). In another study, the VGG16 model was used as the backbone for training, and the network was optimized using stochastic gradient descent and the cross-entropy function as a loss function (52). A model that was updated with the stochastic gradient descent algorithm was demonstrated to be capable of rapidly distinguishing between benign and malignant nodules, with only limited hardware usage (53). Some models may incorporate modules to improve performance; for example, the attention module can be used to shift attention to the most important regions of an image and ignore the irrelevant parts (54). One study employed ResNet50 as the backbone and incorporated the convolutional block attention module prior to the addition of a fire module (55). The use of RNNs in the diagnosis of thyroid disease, compared to the use of CNNs, is limited to a small number of studies. To optimally leverage both convolution and sequence processing, several frameworks combining CNNs and RNNs are being used for medical imaging. For instance, in one study, the VGG16 was used to complete classification tasks, while the LSTM network was used to map visual information to generate concise text description from thyroid US images (56). In other research, the conceptual framework of RNNs for processing sequential data was used for the analysis of CEUS and US video data as a time series (57).
Standard biostatistical measures such as accuracy, sensitivity, and specificity can be used for simple categorization, such as determining the presence of a given disease (39):
True positive (TP) and true negative (TN) indicate the correctly classified positive and negative samples, respectively, while false positive (FP) and false negative (FN) indicate the incorrectly classified negative and positive samples, respectively. A receiver operating characteristic (ROC) curve can be used to plot the TP rate (sensitivity) against the FP rate (1 − specificity) to thus determine the tradeoff between sensitivity and specificity and aid in adjusting the decision threshold. In the case of multiclassification tasks (e.g., for multiple types of lesions), statistical data for each classification can be reported (39).
Detection
Detection tasks consist of the localization and identification of regions of interest or lesions in the full image space (58). Accurate detection of the thyroid target region is an essential step for diagnosis, patient monitoring, and surgical intervention planning.
Typical detection architectures fall into two groups according to the number of stages within the detector. A common type is the two-stage detector, such as faster R-CNN and mask R-CNN (27). The first stage submits areas of interest for consideration, and the second stage predicts bounding box objects and classifications. In general, the process involves a network that proposes the potential location of objects and another that enhances the proposed areas, commonly referred to as a region proposal network (RPN) or a detection network (59). Applications of this architecture type include a multitask mask R-CNN model for automated localization of the bounding box of thyroid nodules in each frame of the US sweep (60) and a cascade R-CNN model based on US videos for automatic detection and segmentation of the thyroid gland and its surrounding tissues (61).
Another type of architecture is the one-stage model, such as YOLO and SSD (28,29). Compared to the two-stage models, it is quicker and easier to run these models. These networks avoid the region proposal stage and directly detect objects by evaluating the probability of their appearance at each point in the image (62). An example of this architecture includes a multiscale detection model based on YOLO for detecting and tracking thyroid nodules and surrounding tissues in thyroid US videos (63). Moreover, an SSD network was able to demonstrate, for the first time, that the pyramidal shape of the feature hierarchy of a CNN could be leveraged to predict objects at different scales, with for instance, the multiple predictive characteristic layers of the SSD network being able to detect thyroid nodules of different scales (62). Compared with SSD, faster R-CNN generally has a meaningful advantage in providing the detection and diagnosis of smaller nodules and in using the layer concatenation strategy to extract more detailed features of low-resolution US images (64). However, while one-stage models are faster than two-stage models, it is challenging for them to achieve higher accuracy without an RPN.
Commonly used evaluation metrics for target detection tasks include the intersection over union (IoU), average precision (AP), mean AP (mAP), and recall (39). Typically, the IoU of a predicted bounding box and a ground truth bounding box is used to measure the precision of the target detection model for object localization. A higher IoU indicates that the model’s predicted bounding box has a higher degree of overlap with the actual ground truth bounding box (53). The precision-recall (PR) curve of the model is plotted with recall as the horizontal coordinate and precision as the vertical coordinate. The area under the PR curve represents the AP value of the model (53). The IoU, the target detection precision, detection recall, and F1 score of the model can be calculated as follows:
A represents the labelled bounding box of the actual object position in the sample, while B represents the predicted bounding box of the object by the target detection model; FP and FN indicate the incorrectly classified negative and positive samples, respectively; and TP indicates the correctly classified positive samples.
Segmentation
Segmentation tasks require the identification of the corresponding target, the accurate depiction of its boundaries, and a determination of whether a given pixel belongs to the background or a target classification (50). Recently, medical image segmentation has been significantly improved by DL methods such as encoder-decoder neural networks, fully convolutional neural networks (FCNs), GANs, and U-net and its variants (64).
However, the gray value of pixels at the edge of thyroid nodules tends to be very similar to the surrounding pixels, and thus the important low-level features used to represent thyroid boundaries may be lost. Bi et al. developed two innovative self-attention pooling techniques to enhance boundary features and generate optimal boundary locations (65), and Yu et al. added an edge-based attention mechanism to strengthen the nodule edge segmentation effect (66).
Individual variability and perspective are significant sources of variation in the nodule size and distribution. This fact increases the importance of the ability of a network to detect target areas that are multiscale in nature. A frequency-domain enhancement network, based on the U-net, has been introduced with a cascaded cross-scale attention module that integrates various features of different receptive fields to overcome the insensitivity of the network to changes in target scale (67). A U-Net was constructed as the backbone, with an adaptive multiscale feature fusion module that fuses features and channel information at different scales (65).
A 2D network structure can often overlook contextual issues related to differences in the US diagnostic process between the thyroid and other organs or tissues. Transformer is a model architecture that eschews recurrence and instead relies entirely on an attention mechanism to draw global dependencies between input and output (55). A encoder-decoder framework and context-attention module based on transformer was proposed to add global associative information to the model (68). The development of alternatives to 3D CNNs includes the combination of 2D CNNs with neural networks specializing in sequence data, allowing for the processing of sequential 2D images of a 3D volume (39). To explore interframe contextual features for thyroid segmentation, a 3D transformer U-net was proposed, which involved integrating a designed 3D self-attending transformer module into the bottom layer of 3D U-net to refine contextual features (69). The primary challenge of dynamic CEUS-based segmentation is modelling the dynamic enhancement evolution patterns associated with differences in blood supply status. To effectively represent real-time enhancement characteristics and aggregate them in a global view, Wan et al. introduced the perfusion excitation gate and cross-attention temporal aggregation module for the automatic segmentation of lesions using dynamic CEUS imaging (70). Other authors proposed a trans-CEUS model for CEUS analysis for spatial temporal separation aggregation and global spatial-temporal fusion methods, with the aim of extracting the enhanced perfusion from the dynamic CEUS picture (71).
Segmentation performance experiments are typically evaluated using the Dice similarity coefficient (DSC) and the Jaccard index (JI) (68). Specifically, the DSC is the ratio of a twofold product of the intersection of the predicted value and the ground truth value to the sum of the predicted value and mask area. Meanwhile, the JI is the percentage of the intersection area between the predicted and true values of image segmentation in the predicted value and the sum of the true value. Both DSC and the JI range from 0 to 1, and the higher these coefficients are, the closer the predicted and true values of image segmentation (69). The definitions can be mathematically expressed as follows:
where Y and G represent the predicted segmentation result and the ground truth, respectively.
Reconstruction
Reconstruction tasks either involve learning how to translate raw sensory input directly into output images or completing a postprocessing step to reduce image noise and remove artifacts (72). A more recent trend for further advancing biomedical image reconstruction has been to exploit DL to improve resolution accuracy and speed up reconstruction results. Within the realm of CT and MRI, a variety of topics have been examined, including artifact reduction, denoising, and sparse-view and low-dose reconstruction (73). Within the field of US, DL has been mainly applied to rebuild quality images. Hyun et al. presented a framework for speckle reduction in US B-mode imaging using ANNs (74). A custom 3D fully CNN (3DCNN) was proposed to reduce diffuse reverberation noise in the channel signals (75). The 3DCNN showed improvements in image quality metrics such as generalized contrast-to-noise ratio and lag-one coherence (75). Shear-wave elastography (SWE) permits local estimation of tissue elasticity, an important imaging marker in biomedicine (8). In one study, a deep convolutional neural networks (DCNN) model served as an end-to-end nonlinear mapping function, transforming 2D B-mode US images to 2D SWE images (76). With DL technologies, longitudinal sound speed has become an alternative approach to diagnosis based on tissue elasticity. A type of fully CNN, which only uses convolutional layers and various nonlinear activations to recover sound speed maps from plane wave US channel data, has been proposed (77).
Acknowledging the difficulty of modeling the whole US image acquisition pipeline, several studies have attempted to incorporate additional AI components, with DL techniques being used for freehand reconstruction of 3D US from 2D probes with image-based tracking. Prevost et al. described a network architecture based on CNN that is able to learn the complete 3D motion of the US probe between two successive frames (78). Wein et al. proposed the DL-based trajectory estimation of individual clips followed by an image-based 3D model optimization of the overlapping transverse and sagittal image data (79).
Data
During the data collection phase, potential data sources and variables of interest are identified. The data are then analyzed and annotated with varying degrees of human involvement. A standard strategy for improving and predicting the applicability of a model is to randomly divide a dataset into three distinct subsets: training, validation, and testing (80). The process of training models involves identifying weight values that lead to a model that appropriately fits the training dataset. The validation set assesses the performance of the model and retunes it if the expected training performance is not met. The test dataset, which the model has not encountered during training, is used to determine the model’s ability to generalize to new data (80). Finally, the real-world performance of a model can be evaluated by separate datasets that are completely external from the original data collection (81).
Advances in architecture have allowed for improved performance. However, it is still rare to achieve a perfect training dataset, especially in medical imaging. The process of data labeling is costly and time-consuming, which limits the accessibility of large medical image datasets (82). Data limitations associated with medical image datasets, specifically the scarcity and weakness of annotations, have proven to be challenging obstacles (83). We examined a variety of solutions, from those relying on human experts (45) to those devising fully automated solutions using unlabeled images and self-monitored pre-training techniques (84). The most common approach to scarcity labelling includes training a CNN on a task with significant data, known as transfer learning (85). The reusability of pretrained models on generic image datasets, such as ImageNet, has enabled many researchers to improve the performance of their image recognition models by fine-tuning the architecture (19). This fine-tuning typically involves adapting the final layers of the pretrained network to the requirements of a relatively small and specific dataset (50). Transfer learning can mitigate data requirements for model convergence and has become routinely used in medical imaging research (40). Some studies have utilized the domain knowledge of clinicians to create networks that resemble their trained and diagnostic patterns or focus on their particular features and areas of interest (86,87). Meanwhile, in ensemble learning, a set of features with a variety of transformations is first extracted, and then multiple learning algorithms are used to produce weak predictive results; the subsequently produced informative knowledge is then fused to achieve knowledge discovery and better predictive performance based on an adaptive voting mechanism (88). These integrated models not only improve diagnostic ability with limited data but also enhance the explainability of a given feature. Models are trained using several methods, including supervised learning, semisupervised learning, and self-supervised learning. In supervised learning, input data must be accurately labeled, often necessitating manual annotation from medical experts (89). The unsupervised and weakly supervised approaches can solve the problem of having a large number of unlabeled images in medical imaging tasks. Self-supervised learning involves pretraining the network with unlabeled images (84). Semisupervised learning includes two approaches: the first is pseudolabeling, where a DL model labels unlabeled images and incorporates them as new examples while training (38,90), while the second approach involves using both labeled and unlabeled images together to train a model without relying on pseudolabeling (66,91).
The digitization and sharing of medical records have increased concerns about data leakage, thus propelling privacy to the forefront. One effective solution is the removal of sensitive biometric or personal information from medical records that are irrelevant to healthcare (92). Data can be encrypted before being sent to the cloud, allowing clinicians or AI algorithms to review the reconstructed data (92). Another effective means to addressing privacy concerns is to generate realistic synthetic data, which can provide acceptable data quality and be used to improve model performance (93). Synthetically generated data can be shared publicly without privacy concerns and can facilitate numerous opportunities for collaborative research, including building predictive models and finding patterns. As synthetic data generation is inherently a generative process, GAN models have attracted considerable attention in this field (94).
Clinical applications of DL in thyroid imaging
This section outlines the clinical applications of DL according to various thyroid imaging modalities (Table 2, Figure 2).
Table 2
Reference | Imaging method | Clinical task | Dataset | Validation method | Model architectures | Performance |
---|---|---|---|---|---|---|
Zhao (55) | US | Management of thyroid nodule/carcinoma | Training set: 1,210 nodules; test set: 520 nodules | Internal validation | Detection: SSD network | AP 82.1%, ACC 94.8%, SEN 98.8%, SPE 87.5% |
Classification: ResNet50 | ||||||
Ma (95) | US | Management of thyroid nodule/carcinoma | 7,163 images from videos | Internal validation | SPR-Mask R-CNN: faster-R-CNN, ResNet, RPN | mAP 61.1%, AP50 82.8%, AP75 68.5% |
Luo (61) | US video | Management of thyroid nodule/carcinoma | 59 patients | Internal validation | Cascade R-CNN: ResNet, feature pyramid network, RPN | AP 60.8%, AP50 85.9%, AP75 68.0% |
Yang (45) | US (B-mode and elastography) | Management of thyroid nodule/carcinoma | 3,090 images | 5-fold cross-validation | Pre-trained VGG13, U-net to segment, a dual-path semi-supervised conditional generative adversarial network | ACC 90.0%, SEN 87.5%, SPE 92.2%, AUC 91.1% |
Wang (86) | US | Management of thyroid nodule/carcinoma | Training: 2,794 nodules; testing: 198 nodules | Internal validation | ResNet50 and XGBoost | ACC 76.8%, SEN 69.2%, SPE 81.7%, AUC 80.0% |
Peng (96) | US | Management of thyroid nodule/carcinoma | Training set: 18,049 images; test set: 4,305 images | External validation | ResNet, ResNeXt, and DenseNet | ACC 89.1%, SEN 94.9%, SPE 81.2%, AUC 94.4% |
Xu (31) | CEUS | Management of thyroid nodule/carcinoma | 84 patients | Internal validation | LSTM | Rise time ratio AUC: 85.6%; time to peak ratio AUC: 79.4%; mean transit time ratio AUC 76.1% |
Gong (97) | US | Management of thyroid nodule/carcinoma | Training set: 2,879 nodules; test set: 614 nodules | 5-fold cross-validation | A thyroid region prior-guided feature enhancement network | Jaccard 68.4%; Dice 81.3% |
Zhao (98) | US | Management of thyroid nodule/carcinoma | 21,597 images | 5-fold cross-validation | A local and global feature disentangled network | ACC 89.6%, SEN 92.4%, AUC 95.3% |
Sun (99) | US | Management of thyroid nodule/carcinoma | Training set: 2,520 images; validation set: 280 images; test set: 986 images | 10-fold cross-validation | TNSNet: a dual-path network containing a region path and a shape path; DeepLabV3+ | ACC 95.8%, SEN 87.7%, SPE 97.4%, Dice 85.3% |
Hou (100) | US | Management of thyroid nodule/carcinoma | Training set: 2,364 nodules; test set: 568 nodules | 10-fold cross-validation | Pre-trained DenseNet | ACC 85.2%, SEN 88.1%, SPE 84.6%, AUC 0.924 |
Chen (101) | US | Management of thyroid nodule/carcinoma | Training set: 1,345 nodules; test set: 243 nodules | 5-fold cross-validation | InceptionResNetV2 and a fully connected classifier | SEN 83%, SPE 87%, AUC 0.91 |
Ni (57) | US video | Management of thyroid nodule/carcinoma | Training and validation set: 713 videos; internal test set: 153 videos; external test set: 152 videos | Internal validation (7:3) and external validation | DenseNet121, ResNet50, InceptionV3, and LSTM | Internal test set: ACC 91.3%, SEN 94.5%, SPE 0.829%, AUC 0.929 |
External test set: ACC 91.2%, SEN 93.9%, SPE 81.8%, AUC 0.896 | ||||||
Qi (102) | US | Risk evaluation of thyroid cancer metastasis | Training and validation set: 4,441 nodules; internal test set: 222 nodules; external test set: 143 nodules | Internal validation and external validation | Mask-R-CNN network, ResNet50, RPN, bounding box regression, and the ROIAlign layer | Internal test set: ACC 87%, SEN 80%, SPE 92%, AUC 0.91 |
External test set: ACC 85%, SEN 92%, SPE 81%, AUC 0.88 | ||||||
Wang (103) | CT | Risk evaluation of thyroid cancer metastasis | Training set: 423 nodules; internal test set: 182 nodules; external test set: 66 nodules | 5-fold cross-validation, external validation | Pretrained DenseNet, convolutional block attention module |
Internal test set: ACC 78%, SEN 71%, SPE 84%, AUC 0.84 |
External test set: ACC 73%, SEN 62%, SPE 92%, AUC 0.81 | ||||||
Lee (104) | CT | Risk evaluation of thyroid cancer metastasis | Training set: 787 images; internal validation set: 104 images; test set: 104 images; external validation set: 3,838 images | Internal validation (8:1:1) and external validation | VGG16, VGG19, Xception, InceptionV3, InceptionResNetV2, DenseNet121, DenseNet169, and ResNet | ACC 75.7%, SEN 82.8%, SPE 75.3%, AUC 0.846 |
Wu (105) | US, clinic records | Risk evaluation of thyroid cancer metastasis | Training set: 1,031 patients; validation set II: 50 patients; validation set II: 50 patients |
External validation | InceptionResnetV2, multilayer perceptron, and LSTM | Validation set I: ACC 90.0%, SEN 92.0%, SPE 88.0%, AUC 0.970 |
Validation set II: ACC 86.0%, SEN 96.0%, SPE 76.0%, AUC 0.976 | ||||||
Yu (106) | US | Risk evaluation of thyroid cancer metastasis | Main set: 1,013 cases; test set 1: 368 cases; test set 2: 513 cases | Internal cross-validation (8:2) and external validation | A transfer learning radiomics model | Main test set: ACC 0.84, SEN 0.94, SPE 0.77, AUC 0.93 |
Testing set 1: ACC 0.86, SEN 0.83, SPE 0.89, AUC 0.93 | ||||||
Testing set 2: ACC 0.84, SEN 0.95, SPE 0.75, AUC 0.93 | ||||||
Qiao (107) | Thyroid scintigraphy | Discrimination between thyroid function disorders | Control: 175 healthy patients; Graves disease: 834 patients; subacute thyroiditis: 421 patients | Internal validation (7:3) | AlexNet, VGGNet, and ResNet | ACC 85.56–92.78%, SPE 83.83–97.00% |
Zhao (108) | US | Discrimination between thyroid function disorders | Training set: 16,533 images with HT and 14,890 images without HT; validation set: 4,133 images with HT and 3,723 images without HT | Internal validation (8:2) | VGG19, ResNet, dense network, and efficient network | ACC 89.2%, SEN 89.0%, SPE 89.5%, AUC 0.94 |
Ma (51) | Thyroid scintigraphy | Discrimination between thyroid function disorders | Graves disease: 780 images; HT: 438 images; subacute thyroiditis: 810 images; control: 860 images | Internal validation | A modified DenseNet | ACC 99.08–100%, SEN 98.50–100%, SPE 99.50–100% |
Zhang (109) | US | Discrimination between thyroid function disorders | Training set: 106,513 images; internal test set 1: 48,803 images; internal testing set 2: 185 videos; external test set: 5,304 images | Internal cross-validation, external validation | ResNet | Internal test set 1: ACC 0.832, SEN 0.826, SPE 0.835 |
Internal test set 2: ACC 0.832, SEN 0.846, SPE 0.827 | ||||||
External test set: ACC 0.821 SEN 0.842 SPE 0.813. | ||||||
Zhao (110) | Thyroid scintigraphy | Discrimination between thyroid function disorders | Training and internal testing set 1: 2,581 images; external testing set: 613 images | Internal validation (8:2), 5-fold cross-validation, external validation | ResNet-34, AlexNet, ShuffleNetV2, and MobileNetV3 |
Graves disease: SPE 99.0%, PRE 96.9%, AUC 0.997 |
Control: SPE 97.2%, PRE 91.3%, AUC 0.991 | ||||||
Subacute thyroiditis: SPE 99.2%, PRE 97.6%, AUC 0.992 | ||||||
Tumor: SPE 97.1%, PRE 91.5%, AUC 0.980 |
US, ultrasound; SSD, single-shot multibox detector; ResNet, residual network; AP, average precision; ACC, accuracy; SEN, sensitivity; SPE, specificity; CNN, convolutional neural network; R-CNN, region-CNN; RPN, region proposal network; AUC, area under the curve; CEUS, contrast-enhanced ultrasound; DenseNet, dense convolutional network; LSTM, long short-term memory; CT, computed tomography; VGGNet, visual geometry group network; HT, Hashimoto thyroiditis; PRE, precision.
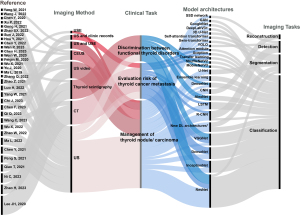
Management of thyroid nodules and carcinoma
Although the Thyroid Imaging Reporting and Data System (TI-RADS) has provided radiologists with a standardized risk stratification and management system to improve their performance in diagnosing thyroid nodules (10), the question remains as to whether more can be done to further optimize its use. Several studies have evaluated the diagnostic performance of DL models directly through radiologist assessments, most of which showed that they were comparable. The DL models integrated with TI-RADS performed more effectively and could prevent unnecessary invasive biopsies (96,111,112). In one study, a DL model for thyroid nodule classification was developed based on multitask DL using TI-RADS characteristics, while another DL model was trained only on benign and malignant diagnostic labels, and the TI-RADS-based model performed better (101). Among patients with Hashimoto thyroiditis, a thyroid parenchyma background repeatedly damaged by chronic inflammation makes it more difficult to distinguish benign nodules from malignant ones (113). In one study, a modified DenseNet model performed slightly more effectively than did the radiologists with different experiences in diagnosing thyroid nodules underlying Hashimoto thyroiditis (100). Dynamic AI based on CNN has been demonstrated to provide real-time synchronous dynamic analysis for diagnosing benign and malignant nodules against a background of Hashimoto thyroiditis (114). Over numerous studies, DL algorithms have achieved the same specificity and sensitivity as those of expert radiologists in thyroid nodule detection and classification tasks (64,96,115-117). Nonetheless, in a real-world setting, the final diagnosis should be made by radiologists. In one study, a DL-assisted strategy improved the pooled area under the curve (AUC) of the radiologists by 4.5% for US images and by 1.2% for US videos (96). Several studies have examined multimodality DL models combining CDFI, USE, or CEUS (45,111,118). The experimental results demonstrated that the accuracy of the proposed methods is better than that of other single-data source methods under the same conditions. A DL-based multimodal feature fusion network was proposed for the automatic diagnosis of thyroid disease based on three modalities: gray-scale US, SWE, and CDFI images (84). US image collection can capture and store data from a full sweep of the entire neck structure, including the entire thyroid lobe in a single volume and all surrounding tissue. However, previous US-based DL models have typically used only partial 2D US images. The recent DL frameworks provide a broader range of perceptions for acquiring tissue and global features of anatomical components, which is superior to local information on lesions (61,95,97,98). Moreover, the development of DL models in the thyroid field has become progressively more specific to subtle features. Multiview CNN models from multiple US images were developed to prevent slight deviations during thyroid scanning (119). For example, a dual-path network containing region and shape paths was shown to be capable of learning the texture and boundary features of nodules, respectively (99). Additionally, a triple-branch classification network was developed which consisted of a fundamental branch for extracting semantic characteristics from input patches, a context branch and a margin branch for extracting improved contextual and marginal features (64). Cordes et al. used a neural network to distinguish papillary thyroid carcinoma (PTC), follicular thyroid carcinoma, poorly differentiated thyroid carcinoma, and anaplastic thyroid carcinoma (120).
Risk evaluation of thyroid cancer metastasis
Patients with thyroid cancer have a high overall survival rate because most cases of PTC are typically indolent tumors with slow progression and low invasion (3). However, some individuals with PTC exhibit a poorer prognosis associated with early metastasis and recurrence following thyroidectomy (121). Therefore, research into the risk factors for highly invasive PTC is crucial. Several characteristics are associated with an unfavorable prognosis, including larger primary tumor size, extrathyroidal extension (ETE), lymph node metastasis (LNM), and distant metastasis (122). The feasibility of active surveillance versus surgical intervention, as well as the extent of surgery, must be assessed according to preoperative imaging, clinical information, and intraoperative conditions (10).
LNM, observed in 30–60% of patients with PTC, is an important indicator of PTC prognosis and is correlated with an increased risk of local recurrence. Improving the diagnostic level of cervical lymph nodes will help reduce unnecessary lymph node dissection (122). Therefore, accurate identification of cervical LNM occurrence bears considerable clinical importance. Wang et al. constructed the DeepLabv3+ networks to detect and quantify calcifications of thyroid nodules, which are considered one of the most important features in the US diagnosis of thyroid cancer, and to further investigate the value of calcifications in predicting the risk of LNM (123). Other studies have also shown that the combination of DL and radiomics provides higher accuracy and is more clinically valuable than either used alone. For example, a transfer learning radiomics model based on US images of thyroid lesions yielded an AUC value of 0.93 (106); meanwhile, DL algorithms that integrated health records and US multimodal images to predict LNM achieved an average AUC of 0.973 (105). Wu et al. proposed an end-to-end deep multimodal learning network, called the multimodal classification network, which used both clinical records and gray-scale US and CDFI images as input and corresponding metastatic status as output (105). In addition to DL models based on US images, those based on CT have been shown to be capable of predicting LNM preoperatively in patients with PTC (103,104). Patients with gross ETE are more likely to have LNM and distant metastasis, higher rates of tumor recurrence, and worse overall survival (124). However, as imaging examinations are unreliable in diagnosing ETE, one study developed a DL model to locate and evaluate thyroid cancer nodules in US images, assessing the presence of ETE before surgery (102).
Discrimination between thyroid function disorders
Most of the above-mentioned models have focused exclusively on the benign and malignant classification of individual thyroid nodules, while other common disorders, such as hyperthyroidism, hypothyroidism, and thyroiditis, have received insufficient attention (5). Functional thyroid diseases are often insidious, and their early symptoms can be nonspecific, leading to a delay in diagnosis (110). US imaging can indicate the presence of abnormal thyroid parenchyma but cannot assess changes in thyroid function. Thyroid scintigraphy is useful for evaluating abnormal thyroid function consistent with overt or subclinical hyperthyroidism (125). However, existing imaging modalities cannot identify the etiological factors that lead to hypothyroidism versus hyperthyroidism, such as Graves disease, Hashimoto thyroiditis, and subacute, postpartum, sporadic, and suppurative thyroiditis (6). Furthermore, the lack of defined standardized features leads to variability in the accurate recognition and consistent interpretation by radiologists. In contrast, using an automated learning procedure, the DL method offers significant advantages in overcoming heterogeneity issues. In one study, a DL-assisted strategy managed to identify the region of Hashimoto thyroiditis based on thyroid US images (108). Zhang et al. showed that a ResNet developed with thyroid US images could achieve high performance in the diagnosis of Hashimoto thyroiditis on static images and video streams (109). Other studies have used thyroid scintigraphy to construct DL models. DL-based models might also serve as tools in the diagnosis of Graves disease and subacute thyroiditis (107). A modified DenseNet architecture was tested for categorizing Graves disease, Hashimoto thyroiditis, and subacute thyroiditis (51). Moreover, a deep CNN-based model was reported to perform well in identifying Graves disease, subacute thyroiditis, and thyroid tumors (110).
Limitations of DL in thyroid imaging
Critical issues still need to be addressed before wider clinical application of DL. One of these challenges is the “black box” problem (38), in that it is difficult to determine how a network arrives at its conclusion. For example, although we can explain the process of construction with mathematical algorithms, if there is an error in the hidden layer of the algorithms, there is no means of determining which layer it is, making it difficult to resolve this issue (126). Strategies have been proposed to reveal the complex internal operation and behavior of these models, including deep convolutional networks, gradient backpropagation, class activation maps, gradient-weighted class activation maps, and saliency maps for multiple CNN architectures (127). Despite these attempts, there is little insight into why these models achieve such good performances or how they may be improved. However, interpretable patterns enable radiologists to learn what features are found within a particular nodule and how important they are, thus serving as a potential tool to train junior radiologists (128).
Continuing to build and grow large-scale, well-annotated open datasets is just as crucial as developing new algorithms for strengthening the completion of clinical tasks (39). The limited performance of DL models trained from a single institution or vendor on external validation data sets raises concerns regarding the broad clinical utility of AI models (82). If subsequent studies use DL models developed from independent training and validation datasets containing data images from different devices and multicenter training cohorts may be able to provide good performance and clinical utility in real-world clinical settings. Moreover, for these AI technologies to take hold, adherence to consensus reporting standards and evaluation criteria for AI image interpretation are needed.
Future directions
Most DL models in this field only examine PTCs and not follicular, medullary, or lymphatic types. Therefore, there is a need to validate and expand these diagnostic models using various types of data. We anticipate that the issues of imbalanced and inadequate data will promote breakthroughs in DL research, particularly in the cases of rare neoplasms with limited availability of imaging data.
The use of DL algorithms in medical diagnosis based on images, particularly US, is still in the early stages of clinical trials. Due to the complexity of the anatomy of the thyroid region, the resolution of these images is typically insufficient for algorithms to identify representative features. The use of ANNs has provided a framework for reducing speckle in gray-scale US images. Despite the limited US scanning time in the clinical workflow, image optimization reduces costs while offering the prospect of multimodality and improved temporal and spatial resolution. Multimodal and multidimensional DL models may be a future direction of thyroid image analysis. In the thyroid field, DL models are built by combining multimodal data such as thyroid 2D or 3D imaging (from US, MRI, CT and radionuclide scans), clinical records, pathology findings, serum thyroid-stimulating hormone levels, thyroid antibody levels, and multiomics. These data can facilitate the refinement of disease management and improve the prognostic relevance of AI-generated biomarkers derived from standard radiographic images to support radiologists in disease diagnosis, imaging quality optimization, data visualization, and clinical assessment. DL models can enable the more accurate and meaningful modelling of healthy and disease states, thus supporting precision medicine.
Conclusions
From a review of studies in thyroid disease, it can be surmised that the main advances in this field involve standardizing the process of acquiring thyroid images and a more comprehensive assessment of thyroid disease images. This has consequently improved diagnostic accuracy, enabled further differential diagnosis, and reduced the number of time-consuming and energy-consuming routine tasks. DL methods are particularly suited for prediction based on existing data but lack precise predictions regarding distant future outcomes. Thus, a DL model can serve as a second opinion in the radiology process to minimize the influence of subjectivity. Although predictive algorithms cannot eliminate medical uncertainty, they have the potential to provide equal access to diagnostic tools in community hospitals and rural regions. With more ongoing research being conducted and more data being generated, we expect clinical settings to improve in the future.
Acknowledgments
Funding: This work was supported by
Footnote
Reporting Checklist: The authors have completed the Narrative Review reporting checklist. Available at https://qims.amegroups.com/article/view/10.21037/qims-23-908/rc
Conflicts of Interest: All authors have completed the ICMJE uniform disclosure form (available at https://qims.amegroups.com/article/view/10.21037/qims-23-908/coif). The authors have no conflicts of interest to declare.
Ethical Statement: The authors are accountable for all aspects of the work in ensuring that questions related to the accuracy or integrity of any part of the work are appropriately investigated and resolved.
Open Access Statement: This is an Open Access article distributed in accordance with the Creative Commons Attribution-NonCommercial-NoDerivs 4.0 International License (CC BY-NC-ND 4.0), which permits the non-commercial replication and distribution of the article with the strict proviso that no changes or edits are made and the original work is properly cited (including links to both the formal publication through the relevant DOI and the license). See: https://creativecommons.org/licenses/by-nc-nd/4.0/.
References
- Walsh JP. Managing thyroid disease in general practice. Med J Aust 2016;205:179-84. [Crossref] [PubMed]
- Taylor PN, Albrecht D, Scholz A, Gutierrez-Buey G, Lazarus JH, Dayan CM, Okosieme OE. Global epidemiology of hyperthyroidism and hypothyroidism. Nat Rev Endocrinol 2018;14:301-16. [Crossref] [PubMed]
- La Vecchia C, Malvezzi M, Bosetti C, Garavello W, Bertuccio P, Levi F, Negri E. Thyroid cancer mortality and incidence: a global overview. Int J Cancer 2015;136:2187-95. [Crossref] [PubMed]
- Sung H, Ferlay J, Siegel RL, Laversanne M, Soerjomataram I, Jemal A, Bray F. Global Cancer Statistics 2020: GLOBOCAN Estimates of Incidence and Mortality Worldwide for 36 Cancers in 185 Countries. CA Cancer J Clin 2021;71:209-49. [Crossref] [PubMed]
- Vanderpump MP. The epidemiology of thyroid disease. Br Med Bull 2011;99:39-51. [Crossref] [PubMed]
- Hoang JK, Sosa JA, Nguyen XV, Galvin PL, Oldan JD. Imaging thyroid disease: updates, imaging approach, and management pearls. Radiol Clin North Am 2015;53:145-61. [Crossref] [PubMed]
- Alexander LF, Patel NJ, Caserta MP, Robbin ML. Thyroid Ultrasound: Diffuse and Nodular Disease. Radiol Clin North Am 2020;58:1041-57. [Crossref] [PubMed]
- Shuzhen C. Comparison analysis between conventional ultrasonography and ultrasound elastography of thyroid nodules. Eur J Radiol 2012;81:1806-11. [Crossref] [PubMed]
- Trimboli P, Castellana M, Virili C, Havre RF, Bini F, Marinozzi F, D'Ambrosio F, Giorgino F, Giovanella L, Prosch H, Grani G, Radzina M, Cantisani V. Performance of contrast-enhanced ultrasound (CEUS) in assessing thyroid nodules: a systematic review and meta-analysis using histological standard of reference. Radiol Med 2020;125:406-15. [Crossref] [PubMed]
- Haugen BR, Alexander EK, Bible KC, Doherty GM, Mandel SJ, Nikiforov YE, Pacini F, Randolph GW, Sawka AM, Schlumberger M, Schuff KG, Sherman SI, Sosa JA, Steward DL, Tuttle RM, Wartofsky L. 2015 American Thyroid Association Management Guidelines for Adult Patients with Thyroid Nodules and Differentiated Thyroid Cancer: The American Thyroid Association Guidelines Task Force on Thyroid Nodules and Differentiated Thyroid Cancer. Thyroid 2016;26:1-133. [Crossref] [PubMed]
- Croker EE, McGrath SA, Rowe CW. Thyroid disease: Using diagnostic tools effectively. Aust J Gen Pract 2021;50:16-21. [Crossref] [PubMed]
- Li M, Dal Maso L, Vaccarella S. Global trends in thyroid cancer incidence and the impact of overdiagnosis. Lancet Diabetes Endocrinol 2020;8:468-70. [Crossref] [PubMed]
- Hosny A, Parmar C, Quackenbush J, Schwartz LH, Aerts HJWL. Artificial intelligence in radiology. Nat Rev Cancer 2018;18:500-10. [Crossref] [PubMed]
- LeCun Y, Bengio Y, Hinton G. Deep learning. Nature 2015;521:436-44. [Crossref] [PubMed]
- Litjens G, Kooi T, Bejnordi BE, Setio AAA, Ciompi F, Ghafoorian M, van der Laak JAWM, van Ginneken B, Sánchez CI. A survey on deep learning in medical image analysis. Med Image Anal 2017;42:60-88. [Crossref] [PubMed]
- Sollini M, Cozzi L, Chiti A, Kirienko M. Texture analysis and machine learning to characterize suspected thyroid nodules and differentiated thyroid cancer: Where do we stand? Eur J Radiol 2018;99:1-8. [Crossref] [PubMed]
- Moen E, Bannon D, Kudo T, Graf W, Covert M, Van Valen D. Deep learning for cellular image analysis. Nat Methods 2019;16:1233-46. [Crossref] [PubMed]
- Soffer S, Ben-Cohen A, Shimon O, Amitai MM, Greenspan H, Klang E. Convolutional Neural Networks for Radiologic Images: A Radiologist's Guide. Radiology 2019;290:590-606. [Crossref] [PubMed]
- Krizhevsky A, Sutskever I, Hinton GE. Imagenet classification with deep convolutional neural networks. Advances in Neural Information Processing Systems 25 (NIPS 2012); 2012.
-
.Simonyan K Zisserman A Very deep convolutional networks for large-scale image recognition. 2015 . arXiv:1409.1556. - Szegedy C, Liu W, Jia Y, Sermanet P, Reed S, Anguelov D, Erhan D, Vanhoucke V, Rabinovich A. Going deeper with convolutions. 2015 IEEE Conference on Computer Vision and Pattern Recognition (CVPR), Boston, MA, USA; 2015;1-9.
- He K, Zhang X, Ren S, Sun J. Deep residual learning for image recognition. 2016 IEEE Conference on Computer Vision and Pattern Recognition (CVPR), Las Vegas, NV, USA; 2016:770-8.
- Huang G, Liu Z, Van Der Maaten L, Weinberger KQ. Densely connected convolutional networks. 2017 IEEE Conference on Computer Vision and Pattern Recognition (CVPR) 2017:2261-9.
- Dhillon A, Verma GK. Convolutional neural network: a review of models, methodologies and applications to object detection. Prog Artif Intell 2019;9:85-112.
- Shelhamer E, Long J, Darrell T. Fully Convolutional Networks for Semantic Segmentation. IEEE Trans Pattern Anal Mach Intell 2017;39:640-51. [Crossref] [PubMed]
- Ronneberger O, Fischer P, Brox T. U-net: Convolutional networks for biomedical image segmentation. International Conference on Medical Image Computing and Computer-Assisted Intervention; 2015:234-41.
- He K, Gkioxari G, Dollár P, Girshick R. Mask R-CNN. 2017 IEEE International Conference on Computer Vision (ICCV), Venice, Italy; 2017:2980-8.
- Redmon J, Farhadi A. YOLO9000: better, faster, stronger. 2017 IEEE Conference on Computer Vision and Pattern Recognition (CVPR), Honolulu, HI, USA; 2017:6517-25.
- Liu W, Angue7lov D, Erhan D, Szegedy C, Reed S, Fu CY, Berg AC. SSD: Single Shot MultiBox Detector. Computer Vision–ECCV 2016: 14th European Conference, Amsterdam, The Netherlands; doi:
10.1007/978-3-319-46448-0_2 . - Williams RJ, Zipser D. A learning algorithm for continually running fully recurrent neural networks. Neural Comput 1989;1:270-80.
- Xu P, Du Z, Sun L, Zhang Y, Zhang J, Qiu Q. Diagnostic Value of Contrast-Enhanced Ultrasound Image Features under Deep Learning in Benign and Malignant Thyroid Lesions. Sci Program 2022; [Crossref]
- Lyu C, Chen B, Ren Y, Ji D. Long short-term memory RNN for biomedical named entity recognition. BMC Bioinformatics 2017;18:462. [Crossref] [PubMed]
- Majumdar A, Gupta M. Recurrent transform learning. Neural Netw 2019;118:271-9. [Crossref] [PubMed]
- Banerjee I, Ling Y, Chen MC, Hasan SA, Langlotz CP, Moradzadeh N, Chapman B, Amrhein T, Mong D, Rubin DL, Farri O, Lungren MP. Comparative effectiveness of convolutional neural network (CNN) and recurrent neural network (RNN) architectures for radiology text report classification. Artif Intell Med 2019;97:79-88. [Crossref] [PubMed]
- Yi X, Walia E, Babyn P. Generative adversarial network in medical imaging: A review. Med Image Anal 2019;58:101552. [Crossref] [PubMed]
- Bera K, Schalper KA, Rimm DL, Velcheti V, Madabhushi A. Artificial intelligence in digital pathology - new tools for diagnosis and precision oncology. Nat Rev Clin Oncol 2019;16:703-15. [Crossref] [PubMed]
- Thomasian NM, Kamel IR, Bai HX. Machine intelligence in non-invasive endocrine cancer diagnostics. Nat Rev Endocrinol 2022;18:81-95. [Crossref] [PubMed]
- Castiglioni I, Rundo L, Codari M, Di Leo G, Salvatore C, Interlenghi M, Gallivanone F, Cozzi A, D'Amico NC, Sardanelli F. AI applications to medical images: From machine learning to deep learning. Phys Med 2021;83:9-24. [Crossref] [PubMed]
- Cheng PM, Montagnon E, Yamashita R, Pan I, Cadrin-Chênevert A, Perdigón Romero F, Chartrand G, Kadoury S, Tang A. Deep Learning: An Update for Radiologists. Radiographics 2021;41:1427-45. [Crossref] [PubMed]
- Yu R, Tian Y, Gao J, Liu Z, Wei X, Jiang H, Huang Y, Li X. Feature discretization-based deep clustering for thyroid ultrasound image feature extraction. Comput Biol Med 2022;146:105600. [Crossref] [PubMed]
- Khojaste-Sarakhsi M, Haghighi SS, Ghomi SMTF, Marchiori E. Deep learning for Alzheimer's disease diagnosis: A survey. Artif Intell Med 2022;130:102332. [Crossref] [PubMed]
- Liang J, Chen J. Data augmentation of thyroid ultrasound images using generative adversarial network. 2021 IEEE International Ultrasonics Symposium (IUS), Xi'an, China; 2021:1-4.
- Yang W, Zhao J, Qiang Y, Yang X, Dong Y, Du Q, Shi G, Zia MB. DScGANS: Integrate Domain Knowledge in Training Dual-Path Semi-supervised Conditional Generative Adversarial Networks and S3VM for Ultrasonography Thyroid Nodules Classification. Medical Image Computing and Computer Assisted Intervention–MICCAI 2019: 22nd International Conference, Shenzhen, China, October 13–17, 2019, Proceedings, Part IV; 2019:558-66.
- Zhao J, Zhou X, Shi G, Xiao N, Song K, Zhao J, Hao R, Li K. Semantic consistency generative adversarial network for cross-modality domain adaptation in ultrasound thyroid nodule classification. Appl Intell (Dordr) 2022;52:10369-83. [Crossref] [PubMed]
- Yang W, Dong Y, Du Q, Qiang Y, Wu K, Zhao J, Yang X, Zia MB. Integrate domain knowledge in training multi-task cascade deep learning model for benign–malignant thyroid nodule classification on ultrasound images. Eng Appl Artif Intell 2021;98:104064.
- Li Z, Liu F, Yang W, Peng S, Zhou J. A Survey of Convolutional Neural Networks: Analysis, Applications, and Prospects. IEEE Trans Neural Netw Learn Syst 2022;33:6999-7019. [Crossref] [PubMed]
- Sharma S, Sharma S, Athaiya A. Activation functions in neural networks. International Journal of Engineering Applied Sciences and Technology 2017;6:310-6.
- Chen L, Qu H, Zhao J, Chen B, Principe JC. Efficient and robust deep learning with correntropy-induced loss function. Neural Comput Appl 2016;27:1019-31.
-
Ruder S. An overview of gradient descent optimization algorithms. arXiv: 1609.04747. - Chartrand G, Cheng PM, Vorontsov E, Drozdzal M, Turcotte S, Pal CJ, Kadoury S, Tang A. Deep Learning: A Primer for Radiologists. Radiographics 2017;37:2113-31. [Crossref] [PubMed]
- Ma L, Ma C, Liu Y, Wang X. Thyroid Diagnosis from SPECT Images Using Convolutional Neural Network with Optimization. Comput Intell Neurosci 2019;2019:6212759. [Crossref] [PubMed]
- Khan A, Sohail A, Zahoora U, Qureshi AS. A survey of the recent architectures of deep convolutional neural networks. Artif Intell Rev 2020;53:5455-516.
- Yu Z, Liu S, Liu P, Liu Y. Automatic detection and diagnosis of thyroid ultrasound images based on attention mechanism. Comput Biol Med 2023;155:106468. [Crossref] [PubMed]
- Vaswani A, Shazeer N, Parmar N, Uszkoreit J, Jones L, Gomez AN, Kaiser Ł, Polosukhin I. Attention is all you need. Advances in Neural Information Processing Systems 30 (NIPS 2017); 2017.
- Zhao Z, Yang C, Wang Q, Zhang H, Shi L, Zhang Z. A deep learning-based method for detecting and classifying the ultrasound images of suspicious thyroid nodules. Med Phys 2021;48:7959-70. [Crossref] [PubMed]
- Zhao Y, Tian M, Jin J, Wang Q, Song J, Shen Y. An automatically thyroid nodules feature extraction and description network for ultrasound images. 2021 IEEE International Ultrasonics Symposium (IUS), Xi'an, China; 2021:1-4.
- Ni C, Feng B, Yao J, Zhou X, Shen J, Ou D, Peng C, Xu D. Value of deep learning models based on ultrasonic dynamic videos for distinguishing thyroid nodules. Front Oncol 2022;12:1066508. [Crossref] [PubMed]
- Bharati P, Pramanik A. Deep learning techniques—R-CNN to mask R-CNN: a survey. In: Das A, Nayak J, Naik B, Pati S, Pelusi D, editors. Computational Intelligence in Pattern Recognition. Singapore: Springer; 2020:657-68.
- Ren S, He K, Girshick R, Sun J. Faster R-CNN: Towards Real-Time Object Detection with Region Proposal Networks. IEEE Trans Pattern Anal Mach Intell 2017;39:1137-49. [Crossref] [PubMed]
- Abdolali F, Kapur J, Jaremko JL, Noga M, Hareendranathan AR, Punithakumar K. Automated thyroid nodule detection from ultrasound imaging using deep convolutional neural networks. Comput Biol Med 2020;122:103871. [Crossref] [PubMed]
- Luo H, Ma L, Wu X, Tan G, Zhu H, Wu S, Li K, Yang Y, Li S. Deep learning-based ultrasonic dynamic video detection and segmentation of thyroid gland and its surrounding cervical soft tissues. Med Phys 2022;49:382-92. [Crossref] [PubMed]
- Xie X, Niu J, Liu X, Chen Z, Tang S, Yu S. A survey on incorporating domain knowledge into deep learning for medical image analysis. Med Image Anal 2021;69:101985. [Crossref] [PubMed]
- Wu X, Tan G, Zhu N, Chen Z, Yang Y, Wen H, Li K. CacheTrack-YOLO: Real-Time Detection and Tracking for Thyroid Nodules and Surrounding Tissues in Ultrasound Videos. IEEE J Biomed Health Inform 2021;25:3812-23. [Crossref] [PubMed]
- Liu T, Guo Q, Lian C, Ren X, Liang S, Yu J, Niu L, Sun W, Shen D. Automated detection and classification of thyroid nodules in ultrasound images using clinical-knowledge-guided convolutional neural networks. Med Image Anal 2019;58:101555. [Crossref] [PubMed]
- Bi H, Cai C, Sun J, Jiang Y, Lu G, Shu H, Ni X. BPAT-UNet: Boundary preserving assembled transformer UNet for ultrasound thyroid nodule segmentation. Comput Methods Programs Biomed 2023;238:107614. [Crossref] [PubMed]
- Yu M, Han M, Li X, Wei X, Jiang H, Chen H, Yu R. Adaptive soft erasure with edge self-attention for weakly supervised semantic segmentation: Thyroid ultrasound image case study. Comput Biol Med 2022;144:105347. [Crossref] [PubMed]
- Chen H, Yu MA, Chen C, Zhou K, Qi S, Chen Y, Xiao R. FDE-net: Frequency-domain enhancement network using dynamic-scale dilated convolution for thyroid nodule segmentation. Comput Biol Med 2023;153:106514. [Crossref] [PubMed]
- Tao Z, Dang H, Shi Y, Wang W, Wang X, Ren S. Local and Context-Attention Adaptive LCA-Net for Thyroid Nodule Segmentation in Ultrasound Images. Sensors (Basel) 2022.
- Chi J, Li Z, Sun Z, Yu X, Wang H. Hybrid transformer UNet for thyroid segmentation from ultrasound scans. Comput Biol Med 2023;153:106453. [Crossref] [PubMed]
- Wan P, Xue H, Liu C, Chen F, Kong W, Zhang D. Dynamic Perfusion Representation and Aggregation Network for Nodule Segmentation Using Contrast-Enhanced US. IEEE J Biomed Health Inform 2023;27:3431-42. [Crossref] [PubMed]
- Chen F, Han H, Wan P, Liao H, Liu C, Zhang D. Joint Segmentation and Differential Diagnosis of Thyroid Nodule in Contrast-Enhanced Ultrasound Images. IEEE Trans Biomed Eng 2023;70:2722-32. [Crossref] [PubMed]
- Ben Yedder H, Cardoen B, Hamarneh G. Deep learning for biomedical image reconstruction: a survey. Artif Intell Rev 2020;54:215-51.
- Wang G, Ye JC, De Man B. Deep learning for tomographic image reconstruction. Nat Mach Intell 2020;2:737-48.
- Hyun D, Brickson LL, Looby KT, Dahl JJ. Beamforming and Speckle Reduction Using Neural Networks. IEEE Trans Ultrason Ferroelectr Freq Control 2019;66:898-910. [Crossref] [PubMed]
- Brickson LL, Hyun D, Jakovljevic M, Dahl JJ. Reverberation Noise Suppression in Ultrasound Channel Signals Using a 3D Fully Convolutional Neural Network. IEEE Trans Med Imaging 2021;40:1184-95. [Crossref] [PubMed]
- Wildeboer RR, van Sloun RJG, Mannaerts CK, Moraes PH, Salomon G, Chammas MC, Wijkstra H, Mischi M. Synthetic Elastography Using B-Mode Ultrasound Through a Deep Fully Convolutional Neural Network. IEEE Trans Ultrason Ferroelectr Freq Control 2020;67:2640-8. [Crossref] [PubMed]
- Feigin M, Freedman D, Anthony BW. A Deep Learning Framework for Single-Sided Sound Speed Inversion in Medical Ultrasound. IEEE Trans Biomed Eng 2020;67:1142-51. [Crossref] [PubMed]
- Prevost R, Salehi M, Jagoda S, Kumar N, Sprung J, Ladikos A, Bauer R, Zettinig O, Wein W. 3D freehand ultrasound without external tracking using deep learning. Med Image Anal 2018;48:187-202. [Crossref] [PubMed]
- Wein W, Lupetti M, Zettinig O, Jagoda S, Salehi M, Markova V, Zonoobi D, Prevost R. Three-Dimensional Thyroid Assessment from Untracked 2D Ultrasound Clips. Medical Image Computing and Computer Assisted Intervention – MICCAI 2020: 23rd International Conference, Lima, Peru, October 4–8, 2020, Proceedings, Part III; 2020:514-23.
- Lambin P, Leijenaar RTH, Deist TM, Peerlings J, de Jong EEC, van Timmeren J, Sanduleanu S, Larue RTHM, Even AJG, Jochems A, van Wijk Y, Woodruff H, van Soest J, Lustberg T, Roelofs E, van Elmpt W, Dekker A, Mottaghy FM, Wildberger JE, Walsh S. Radiomics: the bridge between medical imaging and personalized medicine. Nat Rev Clin Oncol 2017;14:749-62. [Crossref] [PubMed]
- Yi J, Kang HK, Kwon JH, Kim KS, Park MH, Seong YK, Kim DW, Ahn B, Ha K, Lee J, Hah Z, Bang WC. Technology trends and applications of deep learning in ultrasonography: image quality enhancement, diagnostic support, and improving workflow efficiency. Ultrasonography 2021;40:7-22. [Crossref] [PubMed]
- Tajbakhsh N, Jeyaseelan L, Li Q, Chiang JN, Wu Z, Ding X. Embracing imperfect datasets: A review of deep learning solutions for medical image segmentation. Med Image Anal 2020;63:101693. [Crossref] [PubMed]
- Dai W, Cui Y, Wang P, Wu H, Zhang L, Bian Y, Li Y, Li Y, Hu H, Zhao J, Xu D, Kong D, Wang Y, Xu L. Classification regularized dimensionality reduction improves ultrasound thyroid nodule diagnostic accuracy and inter-observer consistency. Comput Biol Med 2023;154:106536. [Crossref] [PubMed]
- Xiang Z, Zhuo Q, Zhao C, Deng X, Zhu T, Wang T, Jiang W, Lei B. Self-supervised multi-modal fusion network for multi-modal thyroid ultrasound image diagnosis. Comput Biol Med 2022;150:106164. [Crossref] [PubMed]
- Al-Stouhi S, Reddy CK. Transfer Learning for Class Imbalance Problems with Inadequate Data. Knowl Inf Syst 2016;48:201-28. [Crossref] [PubMed]
- Wang J, Jiang J, Zhang D, Zhang YZ, Guo L, Jiang Y, Du S, Zhou Q. An integrated AI model to improve diagnostic accuracy of ultrasound and output known risk features in suspicious thyroid nodules. Eur Radiol 2022;32:2120-9. [Crossref] [PubMed]
- Chen Y, Li D, Zhang X, Jin J, Shen Y. Computer aided diagnosis of thyroid nodules based on the devised small-datasets multi-view ensemble learning. Med Image Anal 2021;67:101819. [Crossref] [PubMed]
- Dong X, Yu Z, Cao W, Shi Y, Ma Q. A survey on ensemble learning. Front Comput Sci 2020;14:241-58.
- Zhang J, Mazurowski MA, Allen BC, Wildman-Tobriner B. Multistep Automated Data Labelling Procedure (MADLaP) for thyroid nodules on ultrasound: An artificial intelligence approach for automating image annotation. Artif Intell Med 2023;141:102553. [Crossref] [PubMed]
- Yang X, Deng C, Liu T, Tao D. Heterogeneous Graph Attention Network for Unsupervised Multiple-Target Domain Adaptation. IEEE Trans Pattern Anal Mach Intell 2022;44:1992-2003. [Crossref] [PubMed]
- Wang J, Zhang R, Wei X, Li X, Yu M, Zhu J, Gao J, Liu Z, Yu R. An attention-based semi-supervised neural network for thyroid nodules segmentation. 2019 IEEE International Conference on Bioinformatics and Biomedicine (BIBM), San Diego, CA, USA; 2019:871-6.
- Yu KH, Beam AL, Kohane IS. Artificial intelligence in healthcare. Nat Biomed Eng 2018;2:719-31. [Crossref] [PubMed]
- Yang Y, Lyu J, Wang R, Wen Q, Zhao L, Chen W, et al. A digital mask to safeguard patient privacy. Nat Med 2022;28:1883-92. [Crossref] [PubMed]
- Torfi A, Fox EA, Reddy CK. Differentially private synthetic medical data generation using convolutional GANs. Information Sciences 2022;586:485-500.
- Ma L, Tan G, Luo H, Liao Q, Li S, Li K. A Novel Deep Learning Framework for Automatic Recognition of Thyroid Gland and Tissues of Neck in Ultrasound Image. IEEE Trans Circuits Syst Video Technol 2022;32:6113-24.
- Peng S, Liu Y, Lv W, Liu L, Zhou Q, Yang H, et al. Deep learning-based artificial intelligence model to assist thyroid nodule diagnosis and management: a multicentre diagnostic study. Lancet Digit Health 2021;3:e250-9. [Crossref] [PubMed]
- Gong H, Chen G, Wang R, Xie X, Mao M, Yu Y, Chen F, Li G. Multi-Task Learning For Thyroid Nodule Segmentation With Thyroid Region Prior. 2021 IEEE 18th International Symposium on Biomedical Imaging (ISBI), Nice, France; 2021:257-61.
- Zhao SX, Chen Y, Yang KF, Luo Y, Ma BY, Li YJ. A Local and Global Feature Disentangled Network: Toward Classification of Benign-Malignant Thyroid Nodules From Ultrasound Image. IEEE Trans Med Imaging 2022;41:1497-509. [Crossref] [PubMed]
- Sun J, Li C, Lu Z, He M, Zhao T, Li X, Gao L, Xie K, Lin T, Sui J, Xi Q, Zhang F, Ni X. TNSNet: Thyroid nodule segmentation in ultrasound imaging using soft shape supervision. Comput Methods Programs Biomed 2022;215:106600. [Crossref] [PubMed]
- Hou Y, Chen C, Zhang L, Zhou W, Lu Q, Jia X, Zhang J, Guo C, Qin Y, Zhu L, Zuo M, Xiao J, Huang L, Zhan W. Using Deep Neural Network to Diagnose Thyroid Nodules on Ultrasound in Patients With Hashimoto's Thyroiditis. Front Oncol 2021;11:614172. [Crossref] [PubMed]
- Chen Y, Gao Z, He Y, Mai W, Li J, Zhou M, Li S, Yi W, Wu S, Bai T, Zhang N, Zeng W, Lu Y, Liu H. An Artificial Intelligence Model Based on ACR TI-RADS Characteristics for US Diagnosis of Thyroid Nodules. Radiology 2022;303:613-9. [Crossref] [PubMed]
- Qi Q, Huang X, Zhang Y, Cai S, Liu Z, Qiu T, Cui Z, Zhou A, Yuan X, Zhu W, Min X, Wu Y, Wang W, Zhang C, Xu P. Ultrasound image-based deep learning to assist in diagnosing gross extrathyroidal extension thyroid cancer: a retrospective multicenter study. EClinicalMedicine 2023;58:101905. [Crossref] [PubMed]
- Wang C, Yu P, Zhang H, Han X, Song Z, Zheng G, Wang G, Zheng H, Mao N, Song X. Artificial intelligence-based prediction of cervical lymph node metastasis in papillary thyroid cancer with CT. Eur Radiol 2023;33:6828-40. [Crossref] [PubMed]
- Lee JH, Ha EJ, Kim D, Jung YJ, Heo S, Jang YH, An SH, Lee K. Application of deep learning to the diagnosis of cervical lymph node metastasis from thyroid cancer with CT: external validation and clinical utility for resident training. Eur Radiol 2020;30:3066-72. [Crossref] [PubMed]
- Wu X, Li M, Cui XW, Xu G. Deep multimodal learning for lymph node metastasis prediction of primary thyroid cancer. Phys Med Biol 2022; [Crossref]
- Yu J, Deng Y, Liu T, Zhou J, Jia X, Xiao T, Zhou S, Li J, Guo Y, Wang Y, Zhou J, Chang C. Lymph node metastasis prediction of papillary thyroid carcinoma based on transfer learning radiomics. Nat Commun 2020;11:4807. [Crossref] [PubMed]
- Qiao T, Liu S, Cui Z, Yu X, Cai H, Zhang H, Sun M, Lv Z, Li D. Deep learning for intelligent diagnosis in thyroid scintigraphy. J Int Med Res 2021;49:300060520982842. [Crossref] [PubMed]
- Zhao W, Kang Q, Qian F, Li K, Zhu J, Ma B. Convolutional Neural Network-Based Computer-Assisted Diagnosis of Hashimoto's Thyroiditis on Ultrasound. J Clin Endocrinol Metab 2022;107:953-63. [Crossref] [PubMed]
- Zhang Q, Zhang S, Pan Y, Sun L, Li J, Qiao Y, et al. Deep learning to diagnose Hashimoto's thyroiditis from sonographic images. Nat Commun 2022;13:3759. [Crossref] [PubMed]
- Zhao H, Zheng C, Zhang H, Rao M, Li Y, Fang D, Huang J, Zhang W, Yuan G. Diagnosis of thyroid disease using deep convolutional neural network models applied to thyroid scintigraphy images: a multicenter study. Front Endocrinol (Lausanne) 2023;14:1224191. [Crossref] [PubMed]
- Zhao CK, Ren TT, Yin YF, Shi H, Wang HX, Zhou BY, Wang XR, Li X, Zhang YF, Liu C, Xu HX. A Comparative Analysis of Two Machine Learning-Based Diagnostic Patterns with Thyroid Imaging Reporting and Data System for Thyroid Nodules: Diagnostic Performance and Unnecessary Biopsy Rate. Thyroid 2021;31:470-81. [Crossref] [PubMed]
- Gild ML, Chan M, Gajera J, Lurie B, Gandomkar Z, Clifton-Bligh RJ. Risk stratification of indeterminate thyroid nodules using ultrasound and machine learning algorithms. Clin Endocrinol (Oxf) 2022;96:646-52. [Crossref] [PubMed]
- Xu S, Huang H, Qian J, Liu Y, Huang Y, Wang X, Liu S, Xu Z, Liu J. Prevalence of Hashimoto Thyroiditis in Adults With Papillary Thyroid Cancer and Its Association With Cancer Recurrence and Outcomes. JAMA Netw Open 2021;4:e2118526. [Crossref] [PubMed]
- Wang B, Wan Z, Zhang M, Gong F, Zhang L, Luo Y, Yao J, Li C, Tian W. Diagnostic value of a dynamic artificial intelligence ultrasonic intelligent auxiliary diagnosis system for benign and malignant thyroid nodules in patients with Hashimoto thyroiditis. Quant Imaging Med Surg 2023;13:3618-29. [Crossref] [PubMed]
- Buda M, Wildman-Tobriner B, Hoang JK, Thayer D, Tessler FN, Middleton WD, Mazurowski MA. Management of Thyroid Nodules Seen on US Images: Deep Learning May Match Performance of Radiologists. Radiology 2019;292:695-701. [Crossref] [PubMed]
- Li X, Zhang S, Zhang Q, Wei X, Pan Y, Zhao J, et al. Diagnosis of thyroid cancer using deep convolutional neural network models applied to sonographic images: a retrospective, multicohort, diagnostic study. Lancet Oncol 2019;20:193-201. [Crossref] [PubMed]
- Guo F, Chang W, Zhao J, Xu L, Zheng X, Guo J. Assessment of the statistical optimization strategies and clinical evaluation of an artificial intelligence-based automated diagnostic system for thyroid nodule screening. Quant Imaging Med Surg 2023;13:695-706. [Crossref] [PubMed]
- Qin P, Wu K, Hu Y, Zeng J, Chai X. Diagnosis of Benign and Malignant Thyroid Nodules Using Combined Conventional Ultrasound and Ultrasound Elasticity Imaging. IEEE J Biomed Health Inform 2020;24:1028-36. [Crossref] [PubMed]
- Wang L, Zhang L, Zhu M, Qi X, Yi Z. Automatic diagnosis for thyroid nodules in ultrasound images by deep neural networks. Med Image Anal 2020;61:101665. [Crossref] [PubMed]
- Cordes M, Götz TI, Lang EW, Coerper S, Kuwert T, Schmidkonz C. Advanced thyroid carcinomas: neural network analysis of ultrasonographic characteristics. Thyroid Res 2021;14:16. [Crossref] [PubMed]
- Craig SJ, Bysice AM, Nakoneshny SC, Pasieka JL, Chandarana SP. The Identification of Intraoperative Risk Factors Can Reduce, but Not Exclude, the Need for Completion Thyroidectomy in Low-Risk Papillary Thyroid Cancer Patients. Thyroid 2020;30:222-8. [Crossref] [PubMed]
- Mehanna H, Al-Maqbili T, Carter B, Martin E, Campain N, Watkinson J, McCabe C, Boelaert K, Franklyn JA. Differences in the recurrence and mortality outcomes rates of incidental and nonincidental papillary thyroid microcarcinoma: a systematic review and meta-analysis of 21 329 person-years of follow-up. J Clin Endocrinol Metab 2014;99:2834-43. [Crossref] [PubMed]
- Wang J, Dong C, Zhang YZ, Wang L, Yuan X, He M, Xu S, Zhou Q, Jiang J. A novel approach to quantify calcifications of thyroid nodules in US images based on deep learning: predicting the risk of cervical lymph node metastasis in papillary thyroid cancer patients. Eur Radiol 2023;33:9347-56. [Crossref] [PubMed]
- Lamartina L, Bidault S, Hadoux J, Guerlain J, Girard E, Breuskin I, Attard M, Suciu V, Baudin E, Al Ghuzlan A, Leboulleux S, Hartl D. Can preoperative ultrasound predict extrathyroidal extension of differentiated thyroid cancer? Eur J Endocrinol 2021;185:13-22. [Crossref] [PubMed]
- Giovanella L, Avram AM, Iakovou I, Kwak J, Lawson SA, Lulaj E, Luster M, Piccardo A, Schmidt M, Tulchinsky M, Verburg FA, Wolin E. EANM practice guideline/SNMMI procedure standard for RAIU and thyroid scintigraphy. Eur J Nucl Med Mol Imaging 2019;46:2514-25. [Crossref] [PubMed]
- Panayides AS, Amini A, Filipovic ND, Sharma A, Tsaftaris SA, Young A, Foran D, Do N, Golemati S, Kurc T, Huang K, Nikita KS, Veasey BP, Zervakis M, Saltz JH, Pattichis CS. AI in Medical Imaging Informatics: Current Challenges and Future Directions. IEEE J Biomed Health Inform 2020;24:1837-57. [Crossref] [PubMed]
- Akkus Z, Cai J, Boonrod A, Zeinoddini A, Weston AD, Philbrick KA, Erickson BJ. A Survey of Deep-Learning Applications in Ultrasound: Artificial Intelligence-Powered Ultrasound for Improving Clinical Workflow. J Am Coll Radiol 2019;16:1318-28. [Crossref] [PubMed]
- Liu Z, Wang S, Dong D, Wei J, Fang C, Zhou X, Sun K, Li L, Li B, Wang M, Tian J. The Applications of Radiomics in Precision Diagnosis and Treatment of Oncology: Opportunities and Challenges. Theranostics 2019;9:1303-22. [Crossref] [PubMed]