Accuracy of solid portion size measured on multiplanar volume rendering images for assessing invasiveness in lung adenocarcinoma manifesting as subsolid nodules
Introduction
With the widespread application of chest computed tomography (CT) in screening lung cancer, pulmonary subsolid nodules (SSNs) have been detected more frequently. Accurately diagnosing these nodules and selecting reasonable treatment methods has become increasingly important. (1). In 2011, the International Association for the Study of Lung Cancer introduced new concepts of adenocarcinoma in situ (AIS) and minimally invasive adenocarcinoma (MIA), which would have near 100% disease-specific survival after complete resection (2,3). Therefore, preinvasive lesions or MIA are usually considered for close follow-up or limited resection, whereas lobectomy is still considered the standard surgical treatment for invasive adenocarcinoma (IA) (3,4).
In the recent past, radiation therapy against lung cancer has developed substantially and has been validated as an effective treatment option, which could improve local control and overall survival (5). Local thermal ablation, a precise new minimally invasive technique, has been increasingly applied to treat early-stage lung cancer due to attributes such as fewer complications, minimal invasion, good tolerability, higher repeatability, and quicker recovery (6-8). Therefore, accurate preoperative diagnosis and clinical staging are crucial to the choice of treatment. Currently, the main preoperative diagnostic methods for pulmonary nodules are transthoracic needle aspiration and transbronchial forceps biopsy. However, these examinations are invasive and increase the risk of pneumothorax and hemoptysis, with incidence rates of 21% and 8%, respectively (9). Furthermore, due to small nodule volume or limited sampling, it is difficult to accurately determine the invasive degree of the whole lesion solely based on lung puncture biopsy. Therefore, the results are uncertain, making the diagnosis of pre-invasive lesions or MIA challenging.
Many studies have reported that solid components of SSNs on CT were associated with invasive components (10,11), and that their measurement is vital for categorization according to the Lung-Imaging Reporting and Data System (Lung-RADS) classification and assessing cancer risk (12). Additionally, although the Fleischner Society guidelines [2017] recommend measuring the solid components on the lung window (13), there are some controversies about measuring the solid components on the lung or mediastinal windows (14,15), and there is substantial measurement variability of solid components on the lung window (16). Meanwhile, a non-negligible amount of pure ground-glass nodules (pGGNs) are still diagnosed as MIAs or IAs, which indicates that invasive components may have various CT morphology, ranging from ground-glass to solid (14,17). Therefore, measurement of the size of the solid components on the lung window or mediastinal window is inconsistent with pathological measurement of the invasive components.
Multiplanar volume rendering (MPVR) is a 3-dimensional (3D) image post-processing technology which can customize the CT value transparency color curve of different tissues to assign different brightness and colors. If these parameters of MPVR can be adjusted to the optimal threshold, making the measurements consistent with the pathological results, then we can accurately identify its pathological subtypes and subsequently triage patients appropriately for different clinical treatments.
Therefore, the purpose of this study was to determine the optimal MPVR threshold for measuring solid components of SSNs through phantom research, and then investigate the correlation between the solid component measured on MPVR of this threshold and pathological invasive component through the clinal study. We present this article in accordance with the STARD reporting checklist (available at https://qims.amegroups.com/article/view/10.21037/qims-23-942/rc).
Methods
This study was conducted in accordance with the Declaration of Helsinki (as revised in 2013). The study was approved by the Ethics Board of the First Affiliated Hospital of Chongqing Medical University (No. 2019-062) and the requirement for individual consent for this retrospective analysis was waived. The patients included in our study have yet to be analyzed in previous studies (18,19).
Phantom research
An anthropomorphic chest phantom (Multipurpose Chest Phantom N1 Lungman; Kyoto Kagaku, Kyoto, Japan) simulating an adult male (height: 45 cm, chest circumference: 94 cm, weight: 18 kg) was used in this study (Figure 1). Polyurethane was used to simulate soft tissue and vessel-equivalent structures, and epoxy resin and calcium carbonate were used to simulate bone-equivalent structures. A total of 14 spherical synthetic nodules were manufactured by Kyoto Kagaku (Japan) with the following properties: pGGNs with 1.5 cm diameter ranging in density from −750 to −50 Hounsfield units (HU) in 100 HU increments (group 1) and part-solid nodules (PSNs) with 1 or 2 solid components (group 2). The diameter and density of GGNs (group 1 and group 2) are shown in Tables 1,2.
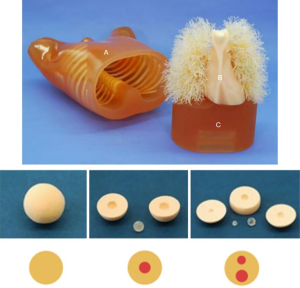
Table 1
pGGN | Diameter (cm) | Density (HU) |
---|---|---|
1 | 1.5 | −50 |
2 | – | −150 |
3 | – | −250 |
4 | – | −350 |
5 | – | −450 |
6 | – | −550 |
7 | – | −650 |
8 | – | −750 |
pGGN, pure ground-glass nodule.
Table 2
PSN | External ground-glass opacity | Internal solid component | |||
---|---|---|---|---|---|
Diameter (cm) | Density (HU) | Diameter (cm) | Density (HU) | ||
1 | 2 | −650 | 0.3 | 0 | |
2 | 0.5 | 0 | |||
3 | 0.7 | 0 | |||
4 | 0.9 | 0 | |||
5 | 2 | −650 | 0.3/0.5 | 0 | |
6 | 0.5/0.7 | 0 |
PSN, part-solid nodule; HU, Hounsfield unit.
Patients
From October 2020 to October 2021, consecutive patients with a solitary SSN who had undergone surgical resection for lung adenocarcinoma at the First Affiliated Hospital of Chongqing Medical University were retrospectively collected in this study. The inclusion criteria were as follows: (I) patients who had undergone CT examination within 2 weeks before the operation; (II) patients without previous chemotherapy or radiation therapy; (III) patients with thin-section CT images (section thickness of ≤1 mm); and (IV) patients with pulmonary nodules pathologically confirmed as lung adenocarcinoma. A total of 193 patients with 193 nodules were initially selected.
After reviewing CT and pathological images, cases with CT images with artifacts (n=3), CT images scanned at outside hospitals (n=17), pathological sections resected along the short diameter of pulmonary nodules (n=11), tumors with uncertain margins (n=6), interwoven invasive components and inflammatory cells within nodules (n=9), and indeterminate pathological results (n=5) were excluded. Finally, 142 patients consisting of 103 women and 39 men were enrolled in this study. The flow chart is shown in Figure 2.
Imaging protocols/image acquisition
A 128-multidetector CT scanner (Somatom Definition Flash; Siemens Healthcare, Erlangen, Germany) was used for the chest phantom containing simulated nodules and patients with SSNs. These simulated nodules were randomly placed in the simulated lung parenchyma (the upper, middle, and lower lungs, centrally and peripherally) and scanned 3 times. All patients were scanned from the lung apex to base, during breath-holding at the end of inspiration. The acquisition protocols were as follows: tube voltage, 100–120 kVp; tube current, 50–150 mA; beam pitch, 1.0; detector collimation, 0.6 mm; rotation time, 0.5 seconds; and a caudocranial scanning direction. Images were reconstructed into 0.625 or 1 mm slice thickness, and a lung algorithm was used for the lung window images.
Imaging analysis
The threshold of MPVR was determined by adjusting the CT value transparent color curve and other parameters to make measurements of MPVR consistent with the actual value of the simulated nodules. The MPVR with the optimal threshold was used to evaluate the simulated nodules and patients’ tumors. Then, 2 radiologists (Z.G.C. and F.J.L.) with more than 10 years of experience of chest CT interpretation jointly analyzed CT images of phantom and patients on a PACS workstation (Carestream Vue PACS; Carestream Health, Rochester, NY, USA) and were blinded to the clinical data and pathological results. Any disagreements between the 2 radiologists during the evaluation were resolved by consensus. CT images were analyzed on MPVR images and CT images with a lung window (window level, −600 HU; width, 1,600 HU) using Picture Archiving and Communication System (PACS; Carestream, USA). The patients’ CT images closest to the date of the surgical resection were used for imaging analysis. The largest radiological size of the tumor on MPVR images, and solid component on MPVR (RSSm) and lung window (RSSl) was measured 3 times, and the average measurements were used as the final results.
Pathologic diagnosis
According to the 2021 lung adenocarcinoma classification described by the World Health Organization Classification of Tumors Editorial Board (20), all pathologic diagnoses were jointly determined by the 2 experienced pulmonary pathologists, who were blinded to the clinical data and radiological results. In order to precisely measure the invasive component, samples were fixed in formalin after the cut sections of each specimen were placed in cassettes. Pathologists first observed the lesion under low magnification and selected the specimen section with the largest lesion and invasiveness as final measured section. Pathological tumor size and pathological invasive component size (PIS) were then measured on the final frozen section or the permanent paraffin section by performing stereoscopic microscopy. Tumors with uncertain margins were excluded. If multiple micro-invasive areas were found in a tumor, the largest invasive area was selected, and its size was recorded. Bland scars and areas of stromal collapse or other non-fibroblastic expansions of alveolar septa were recorded but not counted as invasive foci.
Statistical analysis
All experimental data were statistically analyzed using the software SPSS 21.0 (IBM Corp., Armonk, NY, USA). The diameter of simulated nodules was compared to the reference standard, and the relative error (RE) was calculated. RE was calculated as (/a − b/)/b*100%, where a and b were CT-derived diameters and reference standards, respectively. Measurement data were expressed as mean ± standard deviation (SD). The paired t-test was used to analyze the measurement difference between CT and pathology. Interclass correlation coefficients (ICCs) were used to assess inter-observer and inter-measurement agreement and interpreted as follows: 0–0.20, poor; 0.21–0.40, fair; 0.41–0.60, moderate; 0.61–0.80, good; and 0.81–1.00, excellent (21). A Bland-Altman plot was used to explore the differences between the radiological solid component size (RSS) and PIS. Receiver operating characteristic (ROC) curve analysis was performed to predict the invasive degree. The cut-off values were defined as those for maximal sensitivity and specificity. The positive predictive value and negative predictive value of MPVR in predicting invasiveness were analyzed. A 2-tailed P value <0.05 was considered statistically significant.
Results
Phantom research
The REs of observer 1 (RE1) and observer 2 (RE2) in measuring the diameters of simulated pulmonary nodules are listed in Table 3. The mean RE1 and RE2 of diameters of pGGN, PSN, and solid component were 1.86%±0.43%, 1.00%±0.57%, 1.14%±0.68%, and 1.44%±0.63%, 1.17%±0.47%, and 1.53%±1.42%, respectively. The mean RE of diameters of pGGN, PSN, and solid component were 1.56%±0.56%, 1.08%±0.51%, and 1.34%±1.10%, respectively. The inter-observer agreement of simulated pulmonary nodules’ diameters was excellent (ICC =1.000, P<0.001).
Table 3
Diameter (mm) | Density (HU) | RE1, % | RE2, % | |
---|---|---|---|---|
pGGN | ||||
1 | 15 | −50 | 2.44 | 2.00 |
2 | −150 | 2.44 | 1.78 | |
3 | −250 | 1.78 | 0.67 | |
4 | −350 | 1.33 | 0.44 | |
5 | −450 | 1.78 | 1.33 | |
6 | −550 | 1.78 | 1.33 | |
7 | −650 | 2.00 | 1.78 | |
8 | −750 | 1.33 | 2.22 | |
Mean | – | – | 1.86 | 1.44 |
SD | – | – | 0.43 | 0.63 |
PSN | ||||
1 | 20 | −50 | 1.50 | 1.00 |
2 | −150 | 0.67 | 1.17 | |
3 | −250 | 1.83 | 2.00 | |
4 | −350 | 1.00 | 1.33 | |
5 | −450 | 0.67 | 0.83 | |
6 | −550 | 0.33 | 0.67 | |
Mean | – | – | 1.00 | 1.17 |
SD | – | – | 0.57 | 0.47 |
Solid component | ||||
1 | 3 | 0 | 2.22 | 0.00 |
2 | 5 | 0 | 1.33 | 0.67 |
3 | 7 | 0 | 0.95 | 1.90 |
4 | 9 | 0 | 0.37 | 1.11 |
5 | 3 | 0 | 1.11 | 4.67 |
5 | 0.67 | 0.67 | ||
6 | 5 | 0 | 2.00 | 1.33 |
7 | 0.48 | 1.90 | ||
Mean | – | – | 1.14 | 1.53 |
SD | – | – | 0.68 | 1.42 |
RE1 and RE2 were calculated with diameter measurements by observer 1 and observer 2 on MPVR, respectively. RE, relative error; SD, standard deviation; pGGN, pure ground-glass nodule; PSN, part-solid nodules; HU, Hounsfield unit; MPVR, multiplanar volume rendering.
Clinical research
Characteristics of patients
Demographic data from the study population are summarized in Table 4. A total of 142 patients (mean age, 54±11 years; age range, 23–79 years) were enrolled in this study. Of the 142 patients, 103 (72.5%) were women (mean age, 55±12 years; age range, 23–79 years), and 39 (27.5%) were men (mean age, 54±10 years; age range, 31–76 years). Among them, there were 26 (18.3%), 92 (64.8%), and 24 (16.9%) patients with AISs, MIAs, and IAs, respectively. Of the 142 SSNs, 70 (49.3%) were pGGNs (21 AISs, 45 MIAs, and 4 IAs), and 72 (50.7%) were PSNs (5 AISs, 47 MIAs, and 20 IAs).
Table 4
Variable | Values |
---|---|
Sex | |
Male | 39 (27.5) |
Female | 103 (72.5) |
Age (years) | 54±11 [23–79] |
Location | |
Right upper lobe | 54 (38.0) |
Right middle lobe | 7 (4.9) |
Right lower lobe | 21 (14.8) |
Left upper lobe | 42 (29.6) |
Left lower lobe | 18 (12.7) |
Density | |
pGGN | 70 (49.3) |
PSN | 72 (50.7) |
Pathologic subtype | |
AIS | 26 (18.3) |
MIA | 92 (64.8) |
IA | 24 (16.9) |
Data are presented as number (percentage) or mean ± standard deviation [range]. pGGN, pure ground-glass nodule; PSN, part-solid nodules; AIS, adenocarcinoma in situ; MIA, minimally invasive adenocarcinoma; IA, invasive adenocarcinoma.
CT and pathology measurement
Quantitative measurements of SSNs are summarized in Table 5. The mean (± SD) radiological tumor size measured on MPVR and pathological tumor size were 13.11±4.00 and 7.53±2.68 mm, respectively, and radiological tumor size was significantly larger than pathological tumor size (P<0.001). The mean (± SD) RSSm and PIS were 4.22±3.08 and 3.08±2.90 mm, respectively, and RSSm was significantly larger than PIS (P<0.001). Inter-observer agreement of RSSm was excellent (ICC =0.952, P<0.001), and the inter-measurement agreement of RSSm and PIS was excellent (ICC =0.829, P<0.001). The mean (± SD) RSSl measured by observers 1 and 2 were 2.50±2.89 and 2.72±3.02 mm, respectively. The mean RSSl of 2 observers was 2.61±2.95 mm, which was significantly smaller than the PIS (P<0.001). Inter-observer agreement of RSSl was moderate (ICC =0.761, P<0.001), and the inter-measurement agreement of RSSl and PIS was fair (ICC =0.562, P<0.001). The scatter plot between CT (RSSm, RSSl) and pathology measurements is shown in Figure S1.
Table 5
Variables | Measurements |
---|---|
Radiological tumor size (mm) | 13.11±4.00 (6.30–15.40) |
RSSm (mm) | 4.22±3.08 (0–14.00) |
RSSl (mm) | 2.61±2.95 (0–12.00) |
Pathological tumor size (mm) | 7.53±2.68 (5.60–14.00) |
PIS (mm) | 3.08±2.90 (0–15.30) |
Data are mean ± standard deviation (range). RSSm, radiological solid component size measured on MPVR; RSSl, radiological solid components size measured on lung window; PIS, pathological invasive component size; MPVR, multiplanar volume rendering.
Comparison of MPVR and pathology
When using MPVR to assess the invasive degree of SSNs, the classification of 114 (80.28%) nodules on MPVR was consistent with pathologic results (Figure 3A-3C). In comparison, there was a discrepancy in histologic classification between radiological and pathological diagnosis in 28 (19.72%) lung adenocarcinomas (Figure 3D). Pathologically, the solid components of 25 lung adenocarcinomas were confirmed with apparent fibroblastic proliferation, inflammatory cell infiltration, and hemorrhage. Among them were 1 AIS, 18 MIAs, and 6 IAs. Of the 28 nodules, 9 (6.34%) AISs were upgraded into MIAs on MPVR, and 19 (23.7%) MIAs were upgraded into IAs on MPVR (Figure 4).
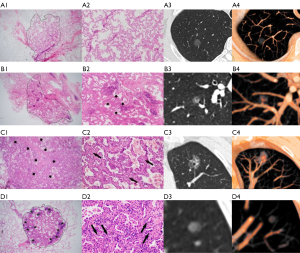
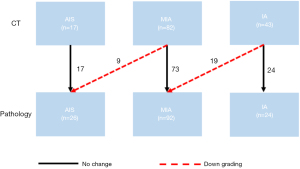
According to Spearman’s correlation analysis (Figure 5), there was a strong correlation between RIS and PIS (r=0.820, P<0.001). The Bland-Altman plot (Figure 5) showed that 5.6% (8/142) points were outside the 95% agreement limit, and the mean difference was 1.14 mm. ROC curve analysis of RSSm for predicting invasive degree showed that the optimal cutoff value between AIS and MIA was 1.85 mm, with an area under the curve (AUC) of 0.963 [95% confidence interval (CI): 0.914–0.993; sensitivity and specificity, 93.8% and 85.7%, P<0.001], and the optimal cutoff value between MIA and AIS was 6.45 mm, with an AUC of 0.956 (95% CI: 0.900–0.983; sensitivity and specificity, 95.5% and 88.2%, P<0.001). The PPV and NPV of MPVR in predicting SSN with invasiveness were 92.8% and 100%, respectively (Table S1).
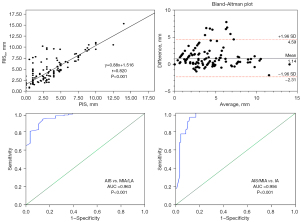
Discussion
Many studies have revealed that the solid component of SSN corresponded well with pathological invasive component (16,17), and its prognostic implication has been investigated (13,21). The clinical T categorization of the SSNs is based on tumor size and the measurement of solid component on CT (22). However, the use of the lung window or mediastinal window to measure the solid component remains controversial and has low inter-observer agreement (10,23,24). Consequently, finding a new technology to assess the invasive degree of SSNs accurately is urgent. This study explored a new PACS-based CT post-processing technique, MPVR, and found that MPVR could predict SSNs’ pathological classification with high accuracy (80.28%) and good inter-observer agreement (ICC =0.952), which could facilitate the early and accurate diagnosis of SSNs and the determination of treatment methods (21).
Initially, the Fleischner Society recommended using the mediastinal window, but many studies reported that the mediastinal window would underestimate the solid component (10,25). Thus, the revised guideline published in 2017 recommended using the lung window with a high-spatial-frequency algorithm (13), which stayed in line with the eighth tumor-node-metastasis (TNM) staging system. However, some studies demonstrated the low inter-observer agreement for measuring solid component using lung window (26-28). Kim et al. (28) reported that the range of measurement variability of the solid component was ±3.7 mm (±61.0%) on the lung window, demonstrating the substantial inter-observer variability in determining the size of the solid component. In this study, the accuracy of diagnosing SSNs on MPVR is higher than that in previous studies (13,28), and the inter-observer agreement of MPVR is better than that of the lung window, which are critical for follow-up assessment, management, and prognosis prediction of patients (Figure 6).
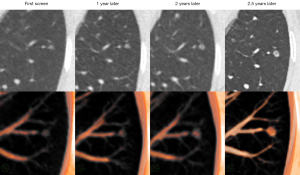
In this study, the radiological size of tumor and invasive component were significantly larger than the pathological measurements, as has been reported in many researches (29). The explanations for the differences between radiological and pathological measurements may be multi-factorial. First, the discrepancies between CT and pathology mainly contributed to the different degrees of lung aeration and expansion in fresh and fixed tissue (29). Radiological measurements are usually performed with the lung fully expanded when patients take deep breaths, whereas pathological measurements are performed with the lung collapsed after the deflation and blood drainage of specimens (30). Second, the pathological subtype of SSNs is also an influential factor, the lepidic component of adenocarcinomas is believed to be responsible for tumor contractibility during routine pathology processes (31). Third, the plane of pathological excision is often not accurately aligned with the maximum plane of the tumor on CT, which makes it difficult for the pathological results to be consistent with the MPVR measurements. Finally, the invasion of lung adenocarcinoma pathologically only refers to any histologic subtype other than a lepidic pattern, whereas radiological invasive components include not only invasive components but may also include alveolar collapse, fibroblast proliferation, inflammation, and hemorrhage (18).
In this study, a new PACS-based CT post-processing technique, MPVR, was explored to measure the solid component more conveniently and accurately. In order to display the lung structure more realistically and intuitively, we set the pulmonary vessels as red and the lung background as black. According to phantom study and clinical practice, we determined the consistency between solid component size on thin-section CT evaluated by MPVR and PIS to predict invasive degree of SSNs. On MPVR images, the component within SSN with the CT value of greater than −350 HU was defined as solid component and displayed as red part consistent with the color of pulmonary vessels, which was close to the results of previous studies (32-35). Cohen et al. (34) reported that software measurements of solid component at a threshold of -350 HU showed the highest agreement without significant difference with invasive component sizes on pathology with a mean difference of −0.12 mm (P>0.05). Meanwhile, the contrast between the red solid components and the white ground-glass opacities (GGO) improves inter-observer consistency.
In addition, there are other techniques that increase the detection of solid component in SSNs, such as artificial intelligence-based techniques and CT post-processing technology (36-38). Singh et al. (36) reported that artificial intelligence-based vessel suppression improved the identification of solid components within PSN, thereby improving the detection of PSN. However, they did not assess its accuracy of measuring the size of solid component. Li et al. (37) demonstrated that the GGO component/solid component proportion measured using 3D computer-quantified CT number analysis was related to the lepidic growth pattern percentage, which is important for the prediction of tumor invasiveness in cT1N0M0 lung adenocarcinoma. Kamiya et al. (38) compared the maximal size of solid component on axial image and multiplanar reconstructed images with measurement acquired by using 3D volumetry software and showed that volumetry of solid component was more correlated with postoperative recurrence. However, these studies did not provide the pathological size of invasive component and compare it with the measurement using these new techniques.
The current study has several limitations. Firstly, this is a retrospective single-center study, which might have issues of inadvertent selection bias in the patient population. Further prospective studies, including patients from multiple centers, must confirm the present results. Secondly, there are no uniform international standards for pathology, including whether an inflation method is applied, the width of the tissue segments the pathologist cuts and the measurement of tumor and solid component size (39,40), which would influence the consistency and accuracy of pathological results. Thirdly, the maximum dimensions of the tumor and solid component on MPVR cannot always be accurately compared with those on pathology because the lung specimens after resection tend to collapse, especially for GGN. However, preventing alveolar collapse after resection would have been difficult.
Conclusions
In conclusion, using MPVR to measure solid component size can predict the invasive degree of lung adenocarcinoma manifesting as SSNs with high accuracy and good inter-observer agreement, and the consistency of measurement between MPVR and pathology is higher than that between lung window and pathology. Although the MPVR-measured size of solid component is larger than the pathological size of invasive component, we would not underestimate the malignant degree of SSNs to avoid delaying the treatment of patients.
Acknowledgments
Funding: This work was supported by
Footnote
Reporting Checklist: The authors have completed the STARD reporting checklist. Available at https://qims.amegroups.com/article/view/10.21037/qims-23-942/rc
Conflicts of Interest: All authors have completed the ICMJE uniform disclosure form (available at https://qims.amegroups.com/article/view/10.21037/qims-23-942/coif). The authors have no conflicts of interest to declare.
Ethical Statement: The authors are accountable for all aspects of the work in ensuring that questions related to the accuracy or integrity of any part of the work are appropriately investigated and resolved. The study was conducted in accordance with the Declaration of Helsinki (as revised in 2013). The study was approved by the Ethics Board of the First Affiliated Hospital of Chongqing Medical University (No. 2019-062) and the requirement for individual consent for this retrospective analysis was waived.
Open Access Statement: This is an Open Access article distributed in accordance with the Creative Commons Attribution-NonCommercial-NoDerivs 4.0 International License (CC BY-NC-ND 4.0), which permits the non-commercial replication and distribution of the article with the strict proviso that no changes or edits are made and the original work is properly cited (including links to both the formal publication through the relevant DOI and the license). See: https://creativecommons.org/licenses/by-nc-nd/4.0/.
References
- Huang P, Park S, Yan R, et al. Added Value of Computer-aided CT Image Features for Early Lung Cancer Diagnosis with Small Pulmonary Nodules: A Matched Case-Control Study. Radiology 2018;286:286-95. [Crossref] [PubMed]
- Travis WD, Brambilla E, Noguchi M, et al. International Association for the Study of Lung Cancer/American Thoracic Society/European Respiratory Society: international multidisciplinary classification of lung adenocarcinoma: executive summary. Proc Am Thorac Soc 2011;8:381-5. [Crossref] [PubMed]
- Qi L, Lu W, Yang L, et al. Qualitative and quantitative imaging features of pulmonary subsolid nodules: differentiating invasive adenocarcinoma from minimally invasive adenocarcinoma and preinvasive lesions. J Thorac Dis 2019;11:4835-46. [Crossref] [PubMed]
- Behera M, Owonikoko TK, Gal AA, et al. Lung Adenocarcinoma Staging Using the 2011 IASLC/ATS/ERS Classification: A Pooled Analysis of Adenocarcinoma In Situ and Minimally Invasive Adenocarcinoma. Clin Lung Cancer 2016;17:e57-64. [Crossref] [PubMed]
- Videtic GM. The Role of Radiotherapy in Small Cell Lung Cancer: a Revisit. Curr Oncol Rep 2015;17:37. [Crossref] [PubMed]
- Ye X, Fan W, Wang H, et al. Expert consensus workshop report: Guidelines for thermal ablation of primary and metastatic lung tumors (2018 edition). J Cancer Res Ther 2018;14:730-44. [Crossref] [PubMed]
- Vogl TJ, Naguib NN, Lehnert T, et al. Radiofrequency, microwave and laser ablation of pulmonary neoplasms: clinical studies and technical considerations--review article. Eur J Radiol 2011;77:346-57. [Crossref] [PubMed]
- Safi S, Rauch G. Sublobar Resection, Radiofrequency Ablation or Radiotherapy in Stage I Non-Small Cell Lung Cancer. Respiration 2015;89:550-7. [Crossref] [PubMed]
- Kiranantawat N, McDermott S, Petranovic M, et al. Determining malignancy in CT guided fine needle aspirate biopsy of subsolid lung nodules: Is core biopsy necessary? Eur J Radiol Open 2019;6:175-81. [Crossref] [PubMed]
- Cohen JG, Reymond E, Lederlin M, et al. Differentiating pre- and minimally invasive from invasive adenocarcinoma using CT-features in persistent pulmonary part-solid nodules in Caucasian patients. Eur J Radiol 2015;84:738-44. [Crossref] [PubMed]
- Lee KH, Goo JM, Park SJ, et al. Correlation between the size of the solid component on thin-section CT and the invasive component on pathology in small lung adenocarcinomas manifesting as ground-glass nodules. J Thorac Oncol 2014;9:74-82. [Crossref] [PubMed]
- Mendoza DP, Petranovic M, Som A, et al. Lung-RADS Category 3 and 4 Nodules on Lung Cancer Screening in Clinical Practice. AJR Am J Roentgenol 2022;219:55-65. [Crossref] [PubMed]
- Bankier AA, MacMahon H, Goo JM, et al. Recommendations for Measuring Pulmonary Nodules at CT: A Statement from the Fleischner Society. Radiology 2017;285:584-600. [Crossref] [PubMed]
- Yoo RE, Goo JM, Hwang EJ, et al. Retrospective assessment of interobserver agreement and accuracy in classifications and measurements in subsolid nodules with solid components less than 8mm: which window setting is better? Eur Radiol 2017;27:1369-76. [Crossref] [PubMed]
- Sakakura N, Inaba Y, Yatabe Y, et al. Estimation of the pathological invasive size of pulmonary adenocarcinoma using high-resolution computed tomography of the chest: A consideration based on lung and mediastinal window settings. Lung Cancer 2016;95:51-6. [Crossref] [PubMed]
- Hwang EJ, Park CM, Ryu Y, et al. Pulmonary adenocarcinomas appearing as part-solid ground-glass nodules: is measuring solid component size a better prognostic indicator? Eur Radiol 2015;25:558-67. [Crossref] [PubMed]
- Kakinuma R, Muramatsu Y, Kusumoto M, et al. Solitary Pure Ground-Glass Nodules 5 mm or Smaller: Frequency of Growth. Radiology 2015;276:873-82. [Crossref] [PubMed]
- Li WJ, Lv FJ, Tan YW, et al. Benign and malignant pulmonary part-solid nodules: differentiation via thin-section computed tomography. Quant Imaging Med Surg 2022;12:699-710. [Crossref] [PubMed]
- Chu ZG, Li WJ, Fu BJ, et al. CT Characteristics for Predicting Invasiveness in Pulmonary Pure Ground-Glass Nodules. AJR Am J Roentgenol 2020;215:351-8. [Crossref] [PubMed]
- WHO Classification of Tumours Editorial Board. WHO classification of tumours. Thoracic Tumours (5th edition). Lyon: IARC; 2021.
- Ahn H, Lee KW, Lee KH, et al. Effect of computed tomography window settings and reconstruction plane on 8th edition T-stage classification in patients with lung adenocarcinoma manifesting as a subsolid nodule. Eur J Radiol 2018;98:130-5.
- Travis WD, Asamura H, Bankier AA, et al. The IASLC Lung Cancer Staging Project: Proposals for Coding T Categories for Subsolid Nodules and Assessment of Tumor Size in Part-Solid Tumors in the Forthcoming Eighth Edition of the TNM Classification of Lung Cancer. J Thorac Oncol 2016;11:1204-23.
- Naidich DP, Bankier AA, MacMahon H, et al. Recommendations for the management of subsolid pulmonary nodules detected at CT: a statement from the Fleischner Society. Radiology 2013;266:304-17. [Crossref] [PubMed]
- Lee SM, Goo JM, Lee KH, et al. CT findings of minimally invasive adenocarcinoma (MIA) of the lung and comparison of solid portion measurement methods at CT in 52 patients. Eur Radiol 2015;25:2318-25. [Crossref] [PubMed]
- Saji H, Matsubayashi J, Akata S, et al. Correlation between whole tumor size and solid component size on high-resolution computed tomography in the prediction of the degree of pathologic malignancy and the prognostic outcome in primary lung adenocarcinoma. Acta Radiol 2015;56:1187-95. [Crossref] [PubMed]
- Ridge CA, Yildirim A, Boiselle PM, et al. Differentiating between Subsolid and Solid Pulmonary Nodules at CT: Inter- and Intraobserver Agreement between Experienced Thoracic Radiologists. Radiology 2016;278:888-96. [Crossref] [PubMed]
- van Riel SJ, Sánchez CI, Bankier AA, et al. Observer Variability for Classification of Pulmonary Nodules on Low-Dose CT Images and Its Effect on Nodule Management. Radiology 2015;277:863-71. [Crossref] [PubMed]
- Kim H, Park CM, Song YS, et al. Measurement Variability of Persistent Pulmonary Subsolid Nodules on Same-Day Repeat CT: What Is the Threshold to Determine True Nodule Growth during Follow-Up? PLoS One 2016;11:e0148853. [Crossref] [PubMed]
- Lampen-Sachar K, Zhao B, Zheng J, et al. Correlation between tumor measurement on Computed Tomography and resected specimen size in lung adenocarcinomas. Lung Cancer 2012;75:332-5. [Crossref] [PubMed]
- Travis WD, Brambilla E, Noguchi M, et al. Diagnosis of lung adenocarcinoma in resected specimens: implications of the 2011 International Association for the Study of Lung Cancer/American Thoracic Society/European Respiratory Society classification. Arch Pathol Lab Med 2013;137:685-705. [Crossref] [PubMed]
- Park CH, Kim TH, Lee S, et al. Correlation between maximal tumor diameter of fresh pathology specimens and computed tomography images in lung adenocarcinoma. PLoS One 2019;14:e0211141. [Crossref] [PubMed]
- Lee JH, Kim TH, Lee S, et al. High versus low attenuation thresholds to determine the solid component of ground-glass opacity nodules. PLoS One 2018;13:e0205490. [Crossref] [PubMed]
- Scholten ET, Jacobs C, van Ginneken B, et al. Detection and quantification of the solid component in pulmonary subsolid nodules by semiautomatic segmentation. Eur Radiol 2015;25:488-96. [Crossref] [PubMed]
- Cohen JG, Goo JM, Yoo RE, et al. Software performance in segmenting ground-glass and solid components of subsolid nodules in pulmonary adenocarcinomas. Eur Radiol 2016;26:4465-74. [Crossref] [PubMed]
- Xu X, Chung JH, Jheon S, et al. The accuracy of frozen section diagnosis of pulmonary nodules: evaluation of inflation method during intraoperative pathology consultation with cryosection. J Thorac Oncol 2010;5:39-44. [Crossref] [PubMed]
- Singh R, Kalra MK, Homayounieh F, et al. Artificial intelligence-based vessel suppression for detection of sub-solid nodules in lung cancer screening computed tomography. Quant Imaging Med Surg 2021;11:1134-43. [Crossref] [PubMed]
- Li M, Wu N, Zhang L, et al. Solid component proportion is an important predictor of tumor invasiveness in clinical stage T(1)N(0)M(0) (cT(1)N(0)M(0)) lung adenocarcinoma. Cancer Imaging 2018;18:18. [Crossref] [PubMed]
- Kamiya S, Iwano S, Umakoshi H, et al. Computer-aided Volumetry of Part-Solid Lung Cancers by Using CT: Solid Component Size Predicts Prognosis. Radiology 2018;287:1030-40. [Crossref] [PubMed]
- Borczuk AC, Qian F, Kazeros A, et al. Invasive size is an independent predictor of survival in pulmonary adenocarcinoma. Am J Surg Pathol 2009;33:462-9. [Crossref] [PubMed]
- Myung JK, Choe G, Chung DH, et al. A simple inflation method for frozen section diagnosis of minute precancerous lesions of the lung. Lung Cancer 2008;59:198-202. [Crossref] [PubMed]