Myelin water quantification in multiple sclerosis using short repetition time adiabatic inversion recovery prepared-fast spin echo (STAIR-FSE) imaging
Introduction
The myelin sheath of axons facilitates the fast transmission of electrical impulses between neurons, which is essential for the proper function of the central nervous system (CNS) (1). Multiple sclerosis (MS) is a debilitating disease characterized by autoimmune demyelination in the brain and/or spinal cord. It results in significant disability in patients and high economic costs in healthcare systems (2,3). The study of myelin quality and quantity is a crucial factor in the diagnosis and understanding of MS. Conventional magnetic resonance imaging (MRI) techniques such as T1- and T2-weighted fast spin echo (FSE) imaging are capable of detecting lesions caused by demyelination, and these are a hallmark of MS, but they lack specificity and may also be produced by other disease processes such as inflammation, edema, and axonal damage (4-7). Because of this, more myelin-specific MRI techniques are an important adjunct to conventional sequences both in diagnosis and the assessment of treatment (8).
Myelin water imaging (MWI) is one of the most promising techniques for more specific assessment of changes in myelin (8). Myelin water (MW) is a water component that is tightly bound within the myelin bilayers and has much shorter T1 and T2 values than the intracellular and extracellular water components found elsewhere in the brain. Several state-of-the-art MWI techniques have been developed to measure myelin water fraction (MWF) (defined as the ratio of the MW signals to total water signals), including multi-component T2 and T2* analysis (4,6,9-12), as well as multicomponent-driven equilibrium single pulse observation of T1 and T2 (mcDESPOT) (13). Studies have demonstrated a strong correlation between MRI-measured MWF and histology-measured myelin content (14,15). Moreover, lower MWFs have been found in both MS lesions and normal-appearing white matter (NAWM) in patients with MS when compared to MWFs in normal white matter (NWM) in healthy controls (16-18). While the existing suite of MWI techniques show promise in myelin assessment in MS, several studies have reported that multicompartment modeling techniques are relatively sensitive to MRI system imperfections (e.g., B1 and B0 inhomogeneities, as well as eddy currents), and this has led to sub-optimal performance (10,19-21). Furthermore, the ill-conditioned fitting process used in modeling is susceptible to noise and artifacts, further degrading the results obtained with MWF mapping (22-24). These lead to increased efforts to improve the performance of these multicompartment modeling techniques (19,20,22,25-28).
Oh et al. have proposed a selective MWI technique utilizing the short T1 of MW i.e., direct visualization of the short transverse relaxation time component (ViSTa) (29). The ViSTa technique utilizes a double inversion recovery (DIR) preparation to suppress signals from long T2 intra/extracellular water compartments which also have long T1 values covering a wide range. While the technique is less sensitive to B0 and B1 inhomogeneities because of the use of adiabatic full passage (AFP) pulses in the DIR preparation, ViSTa’s clinical translation is hampered by its long acquisition time (~3 min per slice).
More recently, a new selective technique, the short repetition time (TR) adiabatic inversion recovery (STAIR) prepared Cones (STAIR-Cones) sequence has been developed to improve the efficiency of MWI (30). This STAIR-Cones technique uses a short TR to effectively suppress long T1 water signals and allow selective imaging of short T1 MW. In the first study with this technique, the measured apparent myelin water fraction (aMWF) of lesions and NAWM in MS patients was significantly lower than the aMWF measured in NWM in the control group, demonstrating the feasibility of using the STAIR-Cones technique to detect the effects of demyelination (30). The technique was limited by the fact that the three-dimensional (3D) Cones acquisition sequence is not clinically available and has only been installed for research purposes on GE MRI scanners (31,32).
To address this issue, we developed a new short repetition time adiabatic inversion recovery prepared-fast spin echo (STAIR-FSE) sequence for translational MWI that can be easily implemented in different clinical settings. The FSE sequence is routinely used in clinical practice and is widely available on vendors’ MR systems. A proton density-weighted FSE (PD-FSE) sequence was also included in the examination to allow total water imaging; when combined with the STAIR-FSE sequence, the PD-FSE sequence facilitated aMWF quantification. In this study, seven MS patients and eight healthy volunteers were scanned with the STAIR-FSE technique. The STAIR-FSE-measured aMWF of MS lesions and NAWM in MS patients were compared with the aMWF in NWM in healthy volunteers.
Methods
STAIR-FSE sequence
Figure 1 shows the STAIR-FSE sequence. Following an AFP pulse and a period for recovery of longitudinal magnetization, a conventional FSE sequence is employed for rapid data acquisition. The FSE component of the sequence begins with a 90° radiofrequency (RF) excitation pulse, and this is succeeded by a series of refocusing pulses with identical flip angles (FAs) of 125°. The inversion time (TI) is the interval between the center of the AFP pulse and the center of the excitation pulse. The saturation time (ST) is defined as the time between the center of the excitation pulse and the center of the last refocusing pulse in each echo train. During this period, the longitudinal magnetization is close to zero due to the 90° excitation and repeated refocusing pulses. Consequently, we define an effective repetition time (TReff), which excludes the ST (i.e., ), to simplify the STAIR-FSE signal model. By utilizing a short TReff ranging from 180 to 300 ms together with an appropriate TI and short echo time (TE) in the STAIR sequence, the signals originating from long T2 water components which exhibit a wide range of T1 values from 600 to 2,000 ms can be effectively suppressed (30).

The signal equation for the STAIR-FSE sequence is:
The longitudinal magnetizations of MW and long T2 water components in the equilibrium state are denoted as . In STAIR-FSE imaging, the signal intensities of MW and long T2 water components are represented by . The inversion efficiency (Q) signifies the impact of the AFP pulse on the z-magnetization and ranges from −1 (full inversion) to 1 (no disturbance). For long T2 water components, Q is assumed to be −1. However, for MW, which has a short T2* of ~10 ms, Q is set at −0.75 when a relatively long AFP pulse (in the case of this study, 8.64 ms) is employed for signal inversion, as determined through Bloch simulation (19,33-35). During the signal inversion phase, specifically the AFP pulse period, transverse signals follow the T2* decay. Thus, T2* of MW should be used in the Bloch simulation to calculate the inversion efficacy Q.
The optimal TI of the STAIR-FSE sequence is determined by minimizing the signals originating from long T2 components with a wide range of T1 values (600–2,000 ms) (30).
To facilitate quantification of aMWF, a PD-FSE sequence is additionally performed to provide total water imaging. The signal equation of the PD-FSE sequence can be expressed as follows:
represents the equilibrium longitudinal magnetization of total water within the brain. In this equation, T2 is employed due to the susceptibility-refocusing properties inherent to the FSE acquisition.
aMWF is defined as the ratio of MW to total water, and is expressed as follows:
Given the known signal intensities of MW (i.e., and total water (i.e., SPD), the calculation of the aMWF is a straightforward division of Eq. [1] by Eq. [2]. The T1 and T2 values of MW are set to 220 and 20 ms, respectively (18,36-38). The T2 of total water is set to 80 ms (39).
In vivo study
This study was conducted in accordance with the Declaration of Helsinki (as revised in 2013) and received approval from the institutional review board (IRB) of University of California, San Diego, with the registration number of 172121. Written informed consents were obtained from all individual participants. A total of seven patients with MS (mean age: 53.7±8.7 years, two males, five females) and eight healthy volunteers (mean age: 38±14 years, four males, four females) were recruited and underwent MRI scans. The inclusion criteria for MS patients required a documented MS diagnosis, age above 18 years, and exclusion of concurrent malignancy or other serious conditions such as stroke. Healthy volunteer inclusion criteria were being in good health and over 18 years of age. Subjects with any contraindications for MRI were excluded from participating in the study.
All participants underwent scanning on a 3 Tesla (T) clinical MRI scanner (MR750, GE Healthcare Technologies, Milwaukee, WI, USA) using a 12-channel head coil for signal reception. The imaging protocol included the acquisition of images in the axial plane using a conventional T2-fluid attenuated inversion recovery (T2-FLAIR) sequence for clinical validation purposes and the STAIR-FSE and PD-FSE sequences for whole brain MWI and total water imaging, respectively.
The T2-FLAIR sequence was scanned with the following parameters: field of view (FOV) =25.6×25.6×16.3 cm3, matrix =256×256×136, TR/TI/TE =7,000/2,028/130 ms, acceleration factor =4, bandwidth =62.5 kHz, and scan time =5.5 min.
The key parameters of the STAIR-FSE and PD-FSE sequences were as follows: (I) STAIR-FSE: FOV =22×22 cm2, matrix =140×140, TReff/TI/TE =250/117/6.8 ms, FA =90°, echo-train-length (ETL) =8, slice thickness =5 mm, number of slices =14, number of excitations (NEX) =7, bandwidth =62.5 kHz, and scan time =8.5 min; (II) PD-FSE: FOV =22×22 cm2, matrix =140×140, TR/TE =8,000/6.8 ms, slice thickness =5 mm, number of slices =14, NEX =1, and scan time =1.4 min.
To evaluate scan repeatability, two healthy subjects were scanned three times, with the scanner conditions reset before each scan.
Data analysis
Elastix registration software is utilized for co-registration between STAIR-FSE and PD-FSE images (40). Regions of interest (ROIs) were manually drawn on the MS lesions using images captured by the STAIR-FSE technique in MS patients, as well as on eight non-lesion white matter regions in both the healthy volunteers and the MS patients. The lesions were selected for analysis when they met the classical definition for MS lesion appearance: hyperintense in T2, oval shape or patchy, high predilection for periventricular white matter, and perpendicular to the ependymal surface (i.e., Dawson’s fingers). Any other non-MS lesions were avoided while drawing ROIs. The MS lesions with sizes ranging from 20 to 300 mm2 were included for aMWF measurement. The non-lesion regions consisted of the left and right centrum semiovale, subcortical white matter, periventricular regions, splenium, and genu of the corpus callosum. The sizes of the ROIs for NWM and NAWM areas were ~300 mm2. The signal to noise (SNR) was calculated according to the following formula: SNR = (NAWM signal)/noise standard deviation (SD), and the contrast to noise ratio (CNR) for MS lesions was calculated according to the following formula: CNR = (lesion signal − NAWM signal)/Noise SD. Drawing of ROIs and calculation of aMWF were performed using MATLAB 2022a software (MathWorks Inc., Natick, MA, USA).
Statistical analysis
Two radiologists, with 3 and 10 years’ experience respectively, drew ROIs independently and the inter-class coefficient (ICC) was calculated to assess the reliability of the aMWF quantification. ICC was also calculated between three scans for each subject to evaluate repeatability of the STAIR-FSE technique. To evaluate the normal distribution of aMWF measures and compare the three distinct groups (MS lesions, NAWM, and NWM), the Kolmogorov-Smirnov test was employed followed by a one-way analysis of variance (ANOVA) test. Moreover, post hoc comparisons between each pair of groups (i.e., NAWM vs. MS lesions, NWM vs. MS lesions, and NWM vs. NAWM) were performed using the Games-Howell test considering the small sample size of this study. Two types of data analysis strategies were employed for the ANOVA test and paired comparisons: (I) single measurement for each subject (i.e., the mean aMWF value of eight different ROIs); (II) multiple measurements for each subject (i.e., different ROIs considered as different measurements). The statistical differences of age and body mass index (BMI) between healthy volunteers and MS patients were tested by independent t-test.
Results
Table 1 summarizes the basic demographic information of the participants including age, sex, race, BMI, and treatments. Both age and BMI show significant difference between healthy volunteers and MS patients due to the relatively small size of the cohorts. Figure 2 displays representative whole brain STAIR-FSE images obtained from a 31-year-old healthy male volunteer. The STAIR-FSE images show significantly higher MW signal intensities in white matter regions compared to gray matter regions. In Figure 3, representative STAIR-FSE and PD-FSE images are presented, along with the corresponding aMWF maps, all acquired from the same 31-year-old male healthy volunteer. The aMWF maps further illustrate higher aMWF values in white matter regions compared to gray matter regions.
Table 1
Participants | Age (years) | Sex | Race | BMI (kg/m2) | Treatment |
---|---|---|---|---|---|
MS patients | |||||
1 | 48 | Female | White | 25.6 | Ocrelizumab |
2 | 52 | Male | White | 26.8 | Natalizumab |
3 | 57 | Female | White | 26.6 | Gabapentin |
4 | 57 | Male | White | 29.7 | N/A |
5 | 67 | Female | White | 24.1 | Glatiramer acetate |
6 | 37 | Female | Asian | 27.3 | Vumerity 231 |
7 | 58 | Female | White | 28.3 | Ocrelizumab |
Mean ± SD | 53.7±8.7 | – | – | 26.9±1.8 | – |
Healthy volunteers | |||||
1 | 30 | Male | White | 20.7 | N/A |
2 | 40 | Female | Latino | 27.5 | N/A |
3 | 70 | Female | Asian | 21 | N/A |
4 | 25 | Male | Asian | 24.9 | N/A |
5 | 30 | Female | Latino | 25.8 | N/A |
6 | 25 | Male | Asian | 26.3 | N/A |
7 | 52 | Female | Asian | 21.5 | N/A |
8 | 32 | Male | White | 22.7 | N/A |
Mean ± SD | 38±14 | – | – | 23.8±2.6 | – |
BMI, body mass index; MS, multiple sclerosis; N/A, not applicable; SD, standard deviation.
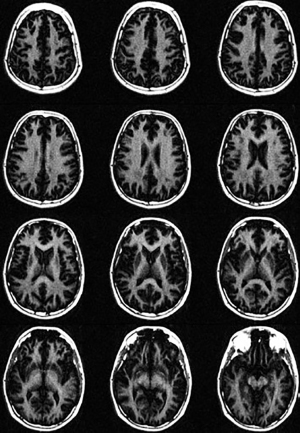
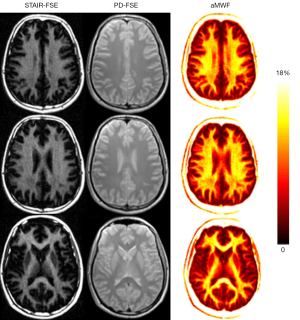
Figure 4 depicts the T2-FLAIR images serving as a reference, the STAIR-FSE and PD-FSE images alongside corresponding aMWF maps from three MS patients. The hyperintense lesions identified in the T2-FLAIR images exhibit an obvious signal loss in the STAIR-FSE images and a decrease in aMWF values within the lesions. The mean SNR for NAWM and mean CNR for MS lesions were 31±0.5 and −10.5±4.0, respectively. In addition, there was an obvious visual correlation between the sizes and shapes of lesions shown on the T2-FLAIR and STAIR-FSE images.
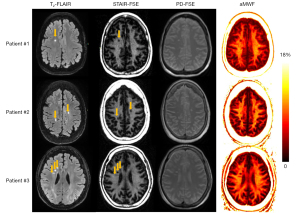
The ICC values showed excellent repeatability (ICC for the first subject =0.95, ICC for the second subject =0.98) between all three scans for each subject. Excellent reliability was found for the two independent measurements in all three groups (i.e., ICC =0.92 for NWM, ICC =0.93 for NAWM, and ICC =0.96 for MS lesions).
Table 2 provides a summary of mean and standard deviation (SD) values for the aMWF measurements for the two types of data analysis. For the first type of analysis, the aMWF values of MS lesions and NAWM in MS patients were found to be 5.1%±1.7% and 10.5%±1.7%, respectively. In healthy volunteers, the aMWF value of NWM was 11.3%±0.7%. For the second type of analysis, the aMWF values of MS lesions and NAWM in MS patients were found to be 5.3%±2.1% and 10.5%±1.7%, respectively. In healthy volunteers, the aMWF value of NWM was 11.3%±1.1%. ANOVA analysis revealed a significant difference between all measurements for the two types of data analysis.
Table 2
ROI | First type of analysis | Second type of analysis | |||||
---|---|---|---|---|---|---|---|
aMWF (%, mean ± SD) |
95% CI | P value | aMWF (%, mean ± SD) |
95% CI | P value | ||
MS lesion | 5.1±1.7 | 3.5–6.6 | <0.001 | 5.3±2.1 | 4.8–5.8 | <0.001 | |
NAWM | 10.5±1.7 | 8.9–12.0 | 10.5±1.7 | 10.0–10.9 | |||
NWM | 11.3±0.7 | 10.7–11.9 | 11.3±1.1 | 11.0–11.6 |
First type of analysis: single measurement (mean value for eight different white matter regions) for each subject; second type of analysis: multiple measurements (i.e., eight values) for each subject. aMWF, apparent myelin water fraction; MS, multiple sclerosis; NAWM, normal-appearing white matter; NWM, normal white matter; ANOVA, analysis of variance; ROI, region of interest; SD, standard deviation; CI, confidence interval.
Figure 5 shows comparisons of measured aMWF values for each of the pairs: NAWM vs. MS lesions, NWM vs. MS lesions, and NWM vs. NAWM. For the first type of data analysis (i.e., single measurement for each subject), significant differences in aMWF measures were found between MS and NAWM and between MS and NWM. Though NAWM had a lower aMWF value than NWM, no significant difference was found for the aMWF measurements between them (P=0.4). For the second type of data analysis (i.e., multiple measurements for each subject), significant differences were found for aMWF measures between MS and NAWM, between MS and NWM, and between NAWM and NWM.
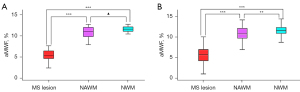
Discussion
In this study, we employed a combination of the STAIR technique and FSE data acquisition to detect MW changes in patients with MS. Using the STAIR-FSE technique on a 3T clinical scanner, whole brain MWI was performed on eight healthy volunteers and seven MS patients. The STAIR-FSE images revealed substantially lower signal intensities in MS lesions compared to NAWM in MS patients. The measured aMWF values in MS lesions (5.1%±1.7%) were significantly lower than those in both NWM of healthy volunteers (11.3%±0.7%) and NAWM of MS patients (10.5%±1.7%). These findings show the potential of the mapping of aMWF using the STAIR-FSE technique to detect and quantify the effects of demyelination in MS. Furthermore, the implementation of the FSE version of the STAIR method facilitates a seamless path for clinical translation of whole brain MWI.
The STAIR-FSE sequence utilized a short TR and TI to efficiently suppress long T2 water components with a range of long T1 values. The clinical FSE sequence enabled rapid data acquisition of the MW signals. More signals will be received with a higher refocusing FA in FSE imaging, whereas fewer signals will be acquired with a larger number of echo times (nTE) (longer effective TE). However, we do not expect dramatic changes in aMWF quantification with either a higher refocusing FA or a larger nTE since the same refocusing FAs and nTE are used in the STAIR-FSE and PD-FSE scans. The signal changes in STAIR-FSE and PD-FSE can be largely canceled in their division process for aMWF quantification.
Multicompartment modeling methods, such as multicomponent T2 and T2* analysis as well as mcDESPOT, require complicated post-processing to solve ill-conditioned problems associated with the determination of MWI, which may reduce the reliability of the MWF quantification (5,19,22-24,30). In comparison, the STAIR technique is free from either complicated post-processing or sensitivity to B0 and B1 inhomogeneities associated with ViSTa is (29,30). The STAIR technique is also more time-efficient than ViSTa because it uses a much shorter TR. Furthermore, the proposed STAIR-FSE sequence has greater potential for clinical translation than the STAIR-Cones sequence because FSE is available on all MRI scanners. In comparison, the Cones sequence is a research sequence that is only available on GE scanners, making it limited for clinical use (32).
Previous studies have reported a wide spectrum of MWFs in MS lesions, NAWM, and NWM, ranging from 4% to 16%. Specifically for NWM, multicomponent T2 analysis techniques have reported MWFs ranging from 9% to 15.6% (4,6,9,11,41-43), while multicomponent T2* analysis techniques have reported MWFs ranging from 6.9% to 14.4% (21,44), and multicomponent T1 modeling techniques have yielded MWFs ranging from 5% to 15% (36). The initial STAIR-Cones study reported an aMWF of 9.2% (30). In the present study, the measured aMWF was 11.2%, which is consistent with previous research findings. Since the T2 is longer than the T2* of MW, it is not surprising that the STAIR-FSE-measured aMWF was slightly higher than the STAIR-Cones-measured aMWF.
The STAIR technique has been integrated with Cones and ultrashort echo time (UTE) for specific MW and myelin imaging, respectively, to take advantage of the method’s long T1 tissue suppression capability (30,45). The efficacy of STAIR’s short T1 tissue suppression is contingent upon the duration of the chosen TR. In the context of non-aqueous myelin imaging with STAIR-UTE, the minimal TR [governed by specific absorption rate (SAR) limitations] is employed (45). In comparison, for MWI using STAIR-Cones and STAIR-FSE, the TR must not be excessively short to prevent undue MW signal suppression by the STAIR method (30). The optimal TR for STAIR in MWI is established by maximizing the CNR efficiency of MW (i.e., 180–300 ms) (30). The 3D STAIR-Cones method facilitates volumetric MWI and exhibits a lower SAR compared to the two-dimensional (2D) STAIR-FSE method. Nevertheless, the ubiquity of the FSE sequence on MRI scanners makes STAIR-FSE more conducive to clinical adoption.
High-field and low-field MRIs are increasingly popular in both the clinic and research environments. At high field strengths, such as 7T, the STAIR-FSE technique could benefit from the improved SNR. The image resolution and scan efficiency could be further improved. Considering the longer tissue T1 relaxation times at higher field MRI, the TR could be increased in STAIR-FSE for selective MWI to mitigate the SAR issue. At low field strengths, such as 0.3T, the major challenge for STAIR-FSE imaging is the reduced image SNR. Recent advancements in denoising using deep learning may improve the STAIR-FSE image quality. In addition, the TR could be decreased in STAIR-FSE MWI due to the shorter tissue T1 relaxation times at lower field MRI. SAR will not be an issue for STAIR-FSE imaging at low-field MRI due to the much-reduced B1 power.
Our study is subject to several limitations. Firstly, the 10-minute scan time utilized in this study is still relatively long for practical clinical applications. To enhance scan efficiency in the future, the incorporation of a deep learning denoising technique with reduced NEX could prove beneficial (46,47). Secondly, the study comprised a sample size of seven MS patients, limiting the power of the statistical analysis. For instance, no significant difference was found between NAWM and NWM measurements in the first type of analysis, while a significant difference was found in the second type of analysis. This difference is primarily attributed to the disparity in sample size. To enhance the generalizability of our findings, we intend to recruit a larger number of MS patients in future studies. Moreover, a study of the correlation between aMWF measurements and patient disability levels [evaluated by Expanded Disability Status Scale (EDSS) scores] would be interesting. Furthermore, a region-specific analysis was not performed in the study due to the limited sample size. Such an analysis will be conducted in the future large cohort study. Thirdly, it is important to note that the specific SAR associated with the STAIR-FSE sequence employed in this study is relatively high. This issue can be addressed by decreasing the FA for the refocusing RF pulses in FSE, thereby substantially reducing the SAR of the sequence. Fourthly, a future comparison study to investigate the MWF value range and imaging reliability between different MWI techniques including multicomponent T2 analysis, multicomponent T2* analysis, ViSTa, STAIR-Cones, and STAIR-FSE would be interesting. Fifthly, in this study, the TI, Q, and T1 and T2 values of MW and long T2 water components were determined empirically. It would be more convincing if these parameters could be measured in the current study as opposed to using reported values in the literature. Once more solid methods are developed to measure these parameters, we will apply them to a future STAIR-FSE MWI study. Sixthly, the imaging resolution of the 2D STAIR-FSE is much lower than the 3D T2-FLAIR in the study. As a result, small lesions may not be detectable in the STAIR-FSE images due to the partial volume effect. To facilitate accurate quantification, the aMWF measurement was performed only for the lesions with sizes larger than 20 mm2. Efforts were made to match anatomical regions between STAIR-FSE and T2-FLAIR during ROI drawing. In future studies, the imaging resolution of the STAIR-FSE technique should be increased and the image registration between STAIR-FSE and T2-FLAIR will be performed for data analysis.
Conclusions
The combination of the STAIR technique with a clinical FSE sequence allows the detection of the consequences of myelin loss in MS. This sequence has great potential for the translation of the technique to clinical applications.
Acknowledgments
Funding: This work was supported by
Footnote
Conflicts of Interest: All authors have completed the ICMJE uniform disclosure form (available at https://qims.amegroups.com/article/view/10.21037/qims-23-1021/coif). J.D. serves as an unpaid editorial board member of Quantitative Imaging in Medicine and Surgery. G.M.B. is a clinical consultant to Magnetica, Brisbane, Australia. The other authors have no conflicts of interest to declare.
Ethical Statement: The authors are accountable for all aspects of the work in ensuring that questions related to the accuracy or integrity of any part of the work are appropriately investigated and resolved. The study was conducted in accordance with the Declaration of Helsinki (as revised in 2013). The study was approved by institutional review board (IRB) of University of California, San Diego, with the registration number of 172121, and written informed consents were obtained from all individual participants.
Open Access Statement: This is an Open Access article distributed in accordance with the Creative Commons Attribution-NonCommercial-NoDerivs 4.0 International License (CC BY-NC-ND 4.0), which permits the non-commercial replication and distribution of the article with the strict proviso that no changes or edits are made and the original work is properly cited (including links to both the formal publication through the relevant DOI and the license). See: https://creativecommons.org/licenses/by-nc-nd/4.0/.
References
- Lee Y, Morrison BM, Li Y, Lengacher S, Farah MH, Hoffman PN, Liu Y, Tsingalia A, Jin L, Zhang PW, Pellerin L, Magistretti PJ, Rothstein JD. Oligodendroglia metabolically support axons and contribute to neurodegeneration. Nature 2012;487:443-8. [Crossref] [PubMed]
- Morell P, Greenfield S, Norton WT, Wisniewski H. Isolation and characterization of myelin protein from adult quaking mice and its similarity to myelin protein of young normal mice. Adv Exp Med Biol 1972;32:251-61. [Crossref] [PubMed]
- Bebo B, Cintina I, LaRocca N, Ritter L, Talente B, Hartung D, Ngorsuraches S, Wallin M, Yang G. The Economic Burden of Multiple Sclerosis in the United States: Estimate of Direct and Indirect Costs. Neurology 2022;98:e1810-7. [Crossref] [PubMed]
- MacKay A, Whittall K, Adler J, Li D, Paty D, Graeb D. In vivo visualization of myelin water in brain by magnetic resonance. Magn Reson Med 1994;31:673-7. [Crossref] [PubMed]
- Lee LE, Ljungberg E, Shin D, Figley CR, Vavasour IM, Rauscher A, Cohen-Adad J, Li DKB, Traboulsee AL, MacKay AL, Lee J, Kolind SH. Inter-Vendor Reproducibility of Myelin Water Imaging Using a 3D Gradient and Spin Echo Sequence. Front Neurosci 2018;12:854. [Crossref] [PubMed]
- Du YP, Chu R, Hwang D, Brown MS, Kleinschmidt-DeMasters BK, Singel D, Simon JH. Fast multislice mapping of the myelin water fraction using multicompartment analysis of T2* decay at 3T: a preliminary postmortem study. Magn Reson Med 2007;58:865-70. [Crossref] [PubMed]
- Kitzler HH, Su J, Zeineh M, Harper-Little C, Leung A, Kremenchutzky M, Deoni SC, Rutt BK. Deficient MWF mapping in multiple sclerosis using 3D whole-brain multi-component relaxation MRI. Neuroimage 2012;59:2670-7. [Crossref] [PubMed]
- Mahajan KR, Ontaneda D. The Role of Advanced Magnetic Resonance Imaging Techniques in Multiple Sclerosis Clinical Trials. Neurotherapeutics 2017;14:905-23. [Crossref] [PubMed]
- Prasloski T, Rauscher A, MacKay AL, Hodgson M, Vavasour IM, Laule C, Mädler B. Rapid whole cerebrum myelin water imaging using a 3D GRASE sequence. Neuroimage 2012;63:533-9. [Crossref] [PubMed]
- Hwang D, Kim DH, Du YP. In vivo multi-slice mapping of myelin water content using T2* decay. Neuroimage 2010;52:198-204. [Crossref] [PubMed]
- Oh J, Han ET, Pelletier D, Nelson SJ. Measurement of in vivo multi-component T2 relaxation times for brain tissue using multi-slice T2 prep at 1.5 and 3 T. Magn Reson Imaging 2006;24:33-43. [Crossref] [PubMed]
- Does MD, Gore JC. Rapid acquisition transverse relaxometric imaging. J Magn Reson 2000;147:116-20. [Crossref] [PubMed]
- Deoni SC, Rutt BK, Peters TM. Rapid combined T1 and T2 mapping using gradient recalled acquisition in the steady state. Magn Reson Med 2003;49:515-26. [Crossref] [PubMed]
- Laule C, Kozlowski P, Leung E, Li DK, Mackay AL, Moore GR. Myelin water imaging of multiple sclerosis at 7 T: correlations with histopathology. Neuroimage 2008;40:1575-80. [Crossref] [PubMed]
- Laule C, Leung E, Lis DK, Traboulsee AL, Paty DW, MacKay AL, Moore GR. Myelin water imaging in multiple sclerosis: quantitative correlations with histopathology. Mult Scler 2006;12:747-53. [Crossref] [PubMed]
- Birkl C, Doucette J, Fan M, Hernández-Torres E, Rauscher A. Myelin water imaging depends on white matter fiber orientation in the human brain. Magn Reson Med 2021;85:2221-31. [Crossref] [PubMed]
- O’Muircheartaigh J, Vavasour I, Ljungberg E, Li DKB, Rauscher A, Levesque V, Garren H, Clayton D, Tam R, Traboulsee A, Kolind S. Quantitative neuroimaging measures of myelin in the healthy brain and in multiple sclerosis. Hum Brain Mapp 2019;40:2104-16. [Crossref] [PubMed]
- Deoni SC, Rutt BK, Arun T, Pierpaoli C, Jones DK. Gleaning multicomponent T1 and T2 information from steady-state imaging data. Magn Reson Med 2008;60:1372-87. [Crossref] [PubMed]
- Prasloski T, Mädler B, Xiang QS, MacKay A, Jones C. Applications of stimulated echo correction to multicomponent T2 analysis. Magn Reson Med 2012;67:1803-14. [Crossref] [PubMed]
- Lee D, Lee J, Lee J, Nam Y. Single-scan z-shim method for reducing susceptibility artifacts in gradient echo myelin water imaging. Magn Reson Med 2018;80:1101-9. [Crossref] [PubMed]
- Shin HG, Oh SH, Fukunaga M, Nam Y, Lee D, Jung W, Jo M, Ji S, Choi JY, Lee J. Advances in gradient echo myelin water imaging at 3T and 7T. Neuroimage 2019;188:835-44. [Crossref] [PubMed]
- Nam Y, Lee J, Hwang D, Kim DH. Improved estimation of myelin water fraction using complex model fitting. Neuroimage 2015;116:214-21. [Crossref] [PubMed]
- Lankford CL, Does MD. On the inherent precision of mcDESPOT. Magn Reson Med 2013;69:127-36. [Crossref] [PubMed]
- Zhang J, Kolind SH, Laule C, MacKay AL. Comparison of myelin water fraction from multiecho T2 decay curve and steady-state methods. Magn Reson Med 2015;73:223-32. [Crossref] [PubMed]
- Nagtegaal M, Koken P, Amthor T, de Bresser J, Mädler B, Vos F, Doneva M. Myelin water imaging from multi-echo T(2) MR relaxometry data using a joint sparsity constraint. Neuroimage 2020;219:117014. [Crossref] [PubMed]
- Liu H, Xiang QS, Tam R, Dvorak AV, MacKay AL, Kolind SH, Traboulsee A, Vavasour IM, Li DKB, Kramer JK, Laule C. Myelin water imaging data analysis in less than one minute. Neuroimage 2020;210:116551. [Crossref] [PubMed]
- Lee J, Lee D, Choi JY, Shin D, Shin HG, Lee J. Artificial neural network for myelin water imaging. Magn Reson Med 2020;83:1875-83. [Crossref] [PubMed]
- Omer N, Galun M, Stern N, Blumenfeld-Katzir T, Ben-Eliezer N. Data-driven algorithm for myelin water imaging: Probing subvoxel compartmentation based on identification of spatially global tissue features. Magn Reson Med 2022;87:2521-35. [Crossref] [PubMed]
- Oh SH, Bilello M, Schindler M, Markowitz CE, Detre JA, Lee J. Direct visualization of short transverse relaxation time component (ViSTa). Neuroimage 2013;83:485-92. [Crossref] [PubMed]
- Ma YJ, Jang H, Lombardi AF, Corey-Bloom J, Bydder GM. Myelin water imaging using a short-TR adiabatic inversion-recovery (STAIR) sequence. Magn Reson Med 2022;88:1156-69. [Crossref] [PubMed]
- Ma YJ, Chang EY, Carl M, Du J. Quantitative magnetization transfer ultrashort echo time imaging using a time-efficient 3D multispoke Cones sequence. Magn Reson Med 2018;79:692-700. [Crossref] [PubMed]
- Carl M, Bydder GM, Du J. UTE imaging with simultaneous water and fat signal suppression using a time-efficient multispoke inversion recovery pulse sequence. Magn Reson Med 2016;76:577-82. [Crossref] [PubMed]
- Larson PE, Conolly SM, Pauly JM, Nishimura DG. Using adiabatic inversion pulses for long-T2 suppression in ultrashort echo time (UTE) imaging. Magn Reson Med 2007;58:952-61. [Crossref] [PubMed]
- Horch RA, Gochberg DF, Nyman JS, Does MD. Clinically compatible MRI strategies for discriminating bound and pore water in cortical bone. Magn Reson Med 2012;68:1774-84. [Crossref] [PubMed]
- Sussman MS, Pauly JM, Wright GA. Design of practical T2-selective RF excitation (TELEX) pulses. Magn Reson Med 1998;40:890-9. [Crossref] [PubMed]
- Labadie C, Lee JH, Rooney WD, Jarchow S, Aubert-Frécon M, Springer CS Jr, Möller HE. Myelin water mapping by spatially regularized longitudinal relaxographic imaging at high magnetic fields. Magn Reson Med 2014;71:375-87. [Crossref] [PubMed]
- Helms G, Hagberg GE. In vivo quantification of the bound pool T1 in human white matter using the binary spin-bath model of progressive magnetization transfer saturation. Phys Med Biol 2009;54:N529-40.
- Rioux JA, Levesque IR, Rutt BK. Biexponential longitudinal relaxation in white matter: Characterization and impact on T1 mapping with IR-FSE and MP2RAGE. Magn Reson Med 2016;75:2265-77. [Crossref] [PubMed]
- Lee J, Hyun JW, Lee J, Choi EJ, Shin HG, Min K, Nam Y, Kim HJ, Oh SH. So You Want to Image Myelin Using MRI: An Overview and Practical Guide for Myelin Water Imaging. J Magn Reson Imaging 2021;53:360-73. [Crossref] [PubMed]
- Klein S, Staring M, Murphy K, Viergever MA, Pluim JP. Elastix: a toolbox for intensity-based medical image registration. IEEE Trans Med Imaging 2010;29:196-205. [Crossref] [PubMed]
- Nguyen TD, Deh K, Monohan E, Pandya S, Spincemaille P, Raj A, Wang Y, Gauthier SA. Feasibility and reproducibility of whole brain myelin water mapping in 4 minutes using fast acquisition with spiral trajectory and adiabatic T2prep (FAST-T2) at 3T. Magn Reson Med 2016;76:456-65. [Crossref] [PubMed]
- Kolind SH, Mädler B, Fischer S, Li DK, MacKay AL. Myelin water imaging: Implementation and development at 3.0T and comparison to 1.5T measurements. Magn Reson Med 2009;62:106-15. [Crossref] [PubMed]
- Whittall KP, MacKay AL, Graeb DA, Nugent RA, Li DK, Paty DW. In vivo measurement of T2 distributions and water contents in normal human brain. Magn Reson Med 1997;37:34-43. [Crossref] [PubMed]
- Alonso-Ortiz E, Levesque IR, Pike GB. MRI-based myelin water imaging: A technical review. Magn Reson Med 2015;73:70-81. [Crossref] [PubMed]
- Ma YJ, Jang H, Wei Z, Cai Z, Xue Y, Lee RR, Chang EY, Bydder GM, Corey-Bloom J, Du J. Myelin Imaging in Human Brain Using a Short Repetition Time Adiabatic Inversion Recovery Prepared Ultrashort Echo Time (STAIR-UTE) MRI Sequence in Multiple Sclerosis. Radiology 2020;297:392-404. [Crossref] [PubMed]
- Chen Z, Pawar K, Ekanayake M, Pain C, Zhong S, Egan GF. Deep Learning for Image Enhancement and Correction in Magnetic Resonance Imaging-State-of-the-Art and Challenges. J Digit Imaging 2023;36:204-30. [Crossref] [PubMed]
- Tamada D. Review: Noise and artifact reduction for MRI using deep learning. arXiv 2020. Doi:
10.48550/arXiv.2002.12889 .