Effect of deep learning image reconstruction with high-definition standard scan mode on image quality of coronary stents and arteries
Introduction
Coronary artery disease (CAD) is a significant health concern with a rising incidence and mortality. Percutaneous coronary intervention (PCI) is the major treatment for CAD, with coronary stent implantation being among the most commonly used approaches. However, in-stent restenosis is a major disadvantage of coronary stent implantation (1) and has an 11% to 46% incidence in bare metal stents (2). Thus, there is a pressing need for the early diagnosis of in-stent restenosis. Coronary computed tomography angiography (CCTA) is a reliable and noninvasive tool for detecting in-stent restenosis (3-5). However, the CCTA of coronary stents is restricted by a limited spatial resolution and the presence of metal artifacts (6). Artifacts can cause artificial thickening of the struts of the stent and narrowing of the lumen inside the stent, severely compromising the in-stent lumen assessment (7,8). Therefore, determining how to overcome the interference of metal artifacts to improve the visualization of the in-stent lumen, thus increasing the diagnostic accuracy of in-stent restenosis, is an urgent issue in CCTA.
The high-definition standard (HD-standard) scan mode can improve spatial resolution and is thus more suitable for evaluating coronary stents than is the conventional standard (STND) scan mode (9). Previous studies have demonstrated the superiority of HD-standard mode over STND mode for displaying stents (10-12). However, increased spatial resolution increases the image noise, thus limiting the more widespread use of the HD-standard scan mode. A tool that can reduce image noise is therefore needed to counteract this effect. Over the past few years, adaptive statistical iterative reconstruction (ASIR; GE HealthCare, Chicago, IL, USA) has been proven capable of reducing image noise and improve image quality in CCTA (13,14). However, ASIR algorithms, especially with high-strength iterative reconstruction (IR), can negatively impact the image textures features and spatial resolution (15,16).
Recently, some studies have reported that deep learning image reconstruction (DLIR; TrueFidelity, GE HealthCare) can achieve low image noise and high image quality without altering image texture (17,18,20). Despite this, the impact of DLIR in CT applications with high spatial resolution, such as CT imaging with HD-standard scan mode for stents—in which the balance between spatial resolution and image noise becomes especially important—has not been studied in depth. Accordingly, we aimed to subjectively and objectively evaluate the effect of HD-standard scan mode with DLIR algorithms on stent and coronary artery image quality in CCTA via a comparison to the STND scan mode with adaptive statistical iterative reconstruction-Veo (ASIR-V) algorithms. We hypothesized that the increased noise resulting from HD-standard mode could be offset by the noise reduction ability of DLIR to provide a higher spatial resolution and clarity for displaying stents without the concurrent noise increase in coronary and perivascular tissues. We present this article in accordance with the GRRAS reporting checklist (available at https://qims.amegroups.com/article/view/10.21037/qims-23-1064/rc).
Methods
Study population
The study was conducted in accordance with the Declaration of Helsinki (as revised in 2013) and was approved by the Ethics Committee of The Third Affiliated Hospital of Sun Yat-sen University. All patients or their family members signed informed consent before coronary CTA was performed, but the requirement for informed consent for use of the images was waived due to the retrospective nature of this study. We retrospectively analyzed images of adult patients with suspected CAD who underwent CCTA at the Third Affiliated Hospital of Sun Yat-sen University from November 2021 to July 2022. Patients were excluded according to the following exclusion criteria: pregnancy; allergic reaction to iodinated contrast medium; renal impairment (creatinine level of 120 mmol/L); and previous pacemaker placement, coronary artery bypass grafting, or valve replacement. A total of 121 consecutive patients were included in the study and divided into stent and coronary groups according to the presence or absence of coronary stenting: 47 patients who had coronary artery stent implantation were assigned to stent group (group A), and the remaining 74 patients were assigned to the normal coronary group (group B). All participants were enrolled regardless of CCTA image quality. Baseline demographics (e.g., age, sex, weight) were recorded for each participant. The baseline demographics were collected and recorded by a radiologist who was not involved in the subsequent image evaluation or analysis to ensure there was no bias in data collection. A summary of the study procedure is presented in Figure 1.
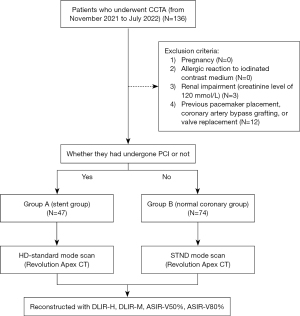
Coronary CT angiography acquisition and reconstruction
All patients were examined on a new 256-row, 16-cm wide-detector CT scanner (Revolution Apex CT, GE HealthCare). The HD-standard scan mode was used for patients in group A, while the STND scan mode was used for patients in group B. A prospective axial electrocardiogram-triggered protocol (automatic gate; GE HealthCare) was employed, with no additional beta blockers being administered to control heart rate. Patients with an irregular heart rate (heart rate variability over 10 beats/min) would have their whole heart scanned in one heartbeat. The scanning parameters were as follows: matrix size, 512×512; detector collimation, 128×0.625 mm; voxel size, 0.625 mm; slice thickness, 0.625 mm; gantry rotation time, 0.28 s; tube voltage, 100 kV; automatic adjustment of tube current (HD-standard mode: 400–600 mA; STND mode: 500–800 mA). The exposure windows were set as follows: for patients with a stable heart rate (heart rate variation ≤10 beats/min), the cardiac cycle was captured in both systole (35–60%) and diastole (70–80%); and for patients with an unstable heart rate (variation >10 beats/min), the exposure windows were set using the smart phase technique provided by the CT system. The best cardiac phase was automatically selected for image generation. The region of interest (ROI) within the descending aorta 1 cm below the tracheal bifurcation was scanned using the bolus injection tracking technique, and CT data acquisition was initiated 7.9 s after the CT attenuation reached the threshold of 60 Hounsfield units (HU). The scan length was 16 cm, from 1 cm below the rongeur to the diaphragm.
For both groups, the contrast agent (370 mgI/mL of iopromide; Ultravist, Bayer, Leverkusen, Germany) was intravenously injected through the antecubital vein with a double-syringe power injector at a flow rate of 4–5 mL/s for 50–60 mL of the contrast agent, which was followed by an injection of 30–40 mL of 0.9% saline solution.
After CCTA scans, a snapshot freeze technique (SSF2) was used to correct cardiac motion. All images were reconstructed with the ASIR-V algorithm (GE HealthCare) at a level of 50% (ASIR-V50%) and 80% (ASIR-V80%) and with DLIR (TrueFidelity, GE HealthCare) at the medium (DLIR-M) and high (DLIR-H) levels. ASIR-V50% was considered the state-of-the-art reconstruction algorithm and was used as the reference standard for comparison purposes. ASIR-V80% was included to further reduce image noise in HD-standard scan mode. DLIR also has several strength settings, including low, medium, and high. In our study, two of the strengths, medium and high, were included to identify the optimal selection of DLIR for HD-standard scan mode. For further image analysis, all data were transferred to the Advantage Workstation 4.7 (AW4.7, GE HealthCare) for the 3D post-processing reconstruction to generate maximum intensity projection (MIP), curved planar reformat (CPR), and volume rendering (VR) images. The image quality was evaluated both objectively and subjectively.
Image quality assessment
Both subjective and objective evaluations of the images were performed independently by two radiologists with more than 10 years of experience, and the final results were reviewed by a third, more senior radiologist. In cases of disagreement, the decision of the senior radiologist was considered final. The “Hide image information” option was selected each time before the image was opened for evaluation or measurement to ensure the image evaluation was fully blind and objective.
Objective assessment
Quantitative image assessment was performed on a dedicated workstation (AW4.7). The “Compare” feature in the workstation was used to display four sets of reconstructed images with four algorithms on the same screen, with the ROI placed at the same locations precisely in the four sets of images. The ROIs were made as large as possible, with a round shape and excluded the stent wall and any plaque or thrombosis. All measurements were conducted on axial images.
For group A, objective image quality evaluation parameters included CT attenuation, noise, signal-to-noise ratio (SNR), contrast-to-noise ratio (CNR), artifact index (AI), inner stent diameter (ISD), and stent wall thickness. The two readers outlined the ROIs at 1 mm from the proximal end of stent (pre-stent) and the center of the stent (in-stent) to acquire the CT attenuation and noise (SD), and then calculated the AI, SNR and CNR according to the following formulae. Myocardial attenuation and noise were used as a reference. A window level/width of 450/1,250 was used for measuring the ISD and stent wall thickness at the center of the stent on the axial image on the picture archiving and communication system (PACS).
For Group B, the objective image quality evaluation parameters included CT attenuation, noise, SNR, and CNR. The CT attenuation (HU) and noise were measured in the aortic root (AO) and in the proximal portion of each of the four coronary arteries: the left main artery (LM), left anterior descending artery (LAD), left circumflex artery (LCX), and the right coronary artery (RCA).
The CT attenuation, noise, SNR, and CNR of the AO, LM, LAD, LCX, and RCA on the DLIR group images with HD-standard scan mode were measured and compared to those of the ASIR-V group images under STND scan mode to determine the noise reduction capability of DLIR.
The SNR, CNR, and AI were calculated using the following formulae, respectively (Figure 2):
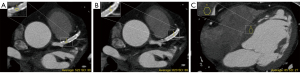
SD in the formulae refers to the image noise value.
Subjective assessment
For group A, the two aforementioned radiologists independently scored the reconstructed images obtained from the four different reconstruction algorithms (ASIR50%, ASIR80%, DLIR-M, and DLIR-H). The image scores of each reconstruction algorithm were given according to axial source images, MIP, CPR, and VR images for the following four parameters: image noise, stent artifacts, stent appearance, and overall image quality. Additionally, a diagnostic confidence score was provided based on the presence or absence of significant stenosis (≥50% lumen reduction) on axial, coronal, and sagittal images for each algorithm image. Images were scored using a 5-point scale (5 = excellent, stent without artifacts, smooth and sharp stent edges, and low noise; 4 = good, stent with slight artifacts, negligible blurring of stent edges, and low noise; 3 = fair, stent with moderate artifacts, moderate blurring of stent edges but not affecting assessment, and slightly high noise; 2 = poor, stent with severe artifacts, strong blurring of stent edges, barely assessable, and high noise; 1 = unreadable, image too poor to be assessable). Diagnostic confidence was also rated with a 5-point scale (5 = very confident, 4 = quite confident, 3 = moderately confident, 2 = less confident, 1 = no confidence).
For group B, the evaluation method involved scoring of the AO, LM, LAD, LCX, and RCA in terms of noise, vessel sharpness, and overall image quality with the following 5-point scale: 5 (excellent) = almost no image noise and excellent vessel sharpness, 4 (very good) = good vessel sharpness with minimal image noise, 3 (good) = moderate noise with minimal vessel sharpness limitation, 2 (poor) = severe image noise with significantly limited vessel sharpness, and 1 (non-evaluable) = excessive image noise resulting in significantly impaired image quality and difficulty of evaluation (21).
Radiation dose
The volumetric CT dose index (CTDIvol) and dose–length product (DLP) were recorded for each patient. The effective radiation dose for the patient was calculated as the DLP multiplied by the conversion factor of 0.014 mSv/mGy*cm (22).
Statistical analysis
Statistical analysis was performed using SPSS 26.0 (IBM Corp.). Continuous variables are expressed as the mean ± standard deviation, whereas categorical data are expressed as the frequency and rate. The independent samples t-test was used to statistically analyze the baseline data that matched a normal distribution. Objective and subjective evaluation data were analyzed using the Kruskal-Wallis test, and post hoc pairwise comparisons were adjusted for multiple comparisons through Bonferroni correction. Cohen kappa and intraclass correlation (ICC) statistics were used to test the interobserver agreement in subjective and objective evaluation (0.81–1.00: excellent, 0.61–0.8: very good, 0.41–0.6: good, <0.41: poor). A P value <0.05 was considered to be statistically significant.
Results
Patient characteristics and radiation dose
A total of 121 consecutive patients (74 males and 47 females, mean age 65.4±11.3 years, mean BMI 25.3±8.1 kg/m2) were included, and 76 stents were implanted in 47 patients. The estimated radiation dose exposure was 2.92±0.8 mSv in group A, which was significantly less than the 3.42±0.8 mSv in group B (P=0.01). The patient demographics and radiation dose parameters are presented in Table 1.
Table 1
Characteristic | Group A (n=47) | Group B (n=74) | P |
---|---|---|---|
Age (years) | 67±10 | 64±12 | 0.234 |
Body mass index (kg/m2) | 23.38 [21.79–25.71] | 24.25 [22.17–27.13] | 0.224 |
Mean heart rate during acquisition (beats/min) | 75±27 | 77±24 | 0.413 |
CTDIvol (mGy) | 13.15±3.3 | 15.27±3.8 | 0.02 |
DLP (mGy*cm) | 208.30±54.1 | 244.65±60.2 | 0.01 |
ED (mSv) | 2.92±0.8 | 3.42±0.8 | 0.01 |
Reconstruction phase | 75 [45–75] | 75 [60–75] | 0.067 |
Data are presented as the mean ± standard deviation or median [range]. CTDIvol, volumetric CT dose index; DLP, dose-length product; ED, effective radiation dose.
Objective assessment of image quality
For group A, there was no significant difference in pre-stent or in-stent lumen CT attenuation among the four image sets (P=0.56). Regarding noise, DLIR-H exhibited superior performance compared to ASIR-V80% and ASIR-V50% (P=0.01) in terms of pre-stent and in-stent SNR and CNR, but there was no statistical difference between DLIR-M and DLIR-H (P=0.315). As for the AI, DLIR-H, DLIR-M, ASIR-V50%, and ASIR-V80%, yielded the lowest to highest values, respectively; there was no statistical difference between DLIR-M and DLIR-H (P=0.80) or between ASIR-V50% and ASIR-V80% (P=0.91), but there was a significant difference between DLIR and ASIR-V (P<0.001). Of further note, pre-stent DLIR-H showed a 36% reduction in image noise, a 51% increase in SNR, and a 71% increase in CNR compared with ASIR-V50%. Similarly, in-stent DLIR-H showed a 42% reduction in image noise, a 57% increase in SNR, and a 60% increase in CNR compared with ASIR-V50%. As for the AI, DLIR-H demonstrated a 36% reduction compared with ASIR-V50%. Regarding ISD, DLIR-H, DLIR-M, ASIR-V80%, and ASIR-V50% yielded the lowest to highest values, respectively, whereas for stent wall thickness, they yielded the highest to lowest values, respectively; DLIR-M and DLIR-H did not differ significantly (P=0.75) but DLIR and ASIR-V did (P=0.04) (Figure 3).
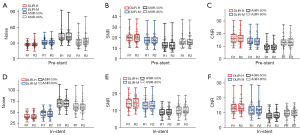
For group B, there was no significant difference in CT attenuation for any coronary lumens among the four image sets (P=0.70). In terms of SNR and CNR, DLIR-H performed significantly better than did DLIR-M, ASIR-V80%, and ASIR-V50% for the AO, LM, LAD, LCX, and RCA (P=0.01). Compared to ASIR-V50%, DLIR-H provided a 48% reduction in image noise, a 90% increase in SNR, and a 76% increase in CNR for the AO; a 41% reduction in image noise, a 72% increase in SNR, and a 61% increase in CNR for the LM; a 33% reduction in image noise, a 49% increase in SNR, and a 55% increase in CNR for LAD; a 34% reduction in image noise, a 53% increase in SNR, and a 56% increase in CNR for the LCX; and a 31% reduction in image noise, a 51% increase in SNR, and a 27% increase in CNR for the RCA.
The objective image quality assessment of group A and group B is presented in Table 2 and Table 3. The interobserver correlation of the measurement was very good to excellent (r=0.70–0.99; P<0.001).
Table 2
Parameter | DLIR-H | DLIR-M | ASIR-V50% | ASIR-V80% |
---|---|---|---|---|
Pre-stent | ||||
Attenuation (HU) | ||||
Reader 1 | 563.0±124.0 | 563.7±122.4 | 562.7±123.3 | 557.9±123.8 |
Reader 2 | 562.6±123.7 | 563.7±124.4 | 561.9±129.1 | 562.9±129.4 |
ICC | 0.99 | 0.99 | 0.95 | 0.98 |
Noise (HU) | ||||
Reader 1 | 27.9±4.8 | 31.9±6.3 | 43.3±11.3 | 33.7±10.3 |
Reader 2 | 27.9±5.1 | 31.9±5.8 | 43.9±11.2 | 35.0±10.2 |
ICC | 0.85 | 0.80 | 0.92 | 0.85 |
SNR | ||||
Reader 1 | 20.7±5.6 | 18.2±5.1 | 13.7±4.5 | 17.6±5.8 |
Reader 2 | 20.6±5.4 | 18.1±4.8 | 13.4±4.4 | 16.9±4.9 |
ICC | 0.94 | 0.90 | 0.95 | 0.83 |
CNR | ||||
Reader 1 | 16.8±4.9 | 14.5±4.5 | 9.8±3.3 | 13.8±4.7 |
Reader 2 | 16.7±4.7 | 14.4±4.3 | 9.7±3.3 | 13.5±4.3 |
ICC | 0.98 | 0.98 | 0.97 | 0.93 |
In-stent | ||||
CT attenuation (HU) | ||||
Reader 1 | 579.1±119.1 | 595.4±125.7 | 626.3±142.7 | 611.3±134.5 |
Reader 2 | 587.4±115.2 | 605.0±120.6 | 633.5±136.6 | 615.3±128.1 |
ICC | 0.97 | 0.97 | 0.95 | 0.94 |
Noise (HU) | ||||
Reader 1 | 41.6±7.1 | 47.4±8.1 | 71.2±11.6 | 63.7±14.3 |
Reader 2 | 41.3±7.2 | 47.2±8.5 | 70.7±11.1 | 63.2±13.6 |
ICC | 0.82 | 0.84 | 0.71 | 0.76 |
SNR | ||||
Reader 1 | 14.6±3.5 | 13.3±3.3 | 9.3±2.5 | 10.4±3.0 |
Reader 2 | 15.0±3.5 | 13.6±3.5 | 9.4±2.3 | 10.6±2.9 |
ICC | 0.90 | 0.90 | 0.82 | 0.86 |
CNR | ||||
Reader 1 | 13.6±3.8 | 12.2±3.7 | 8.5±2.7 | 10.0±2.9 |
Reader 2 | 13.9±3.8 | 12.5±3.8 | 8.6±2.6 | 10.1±2.8 |
ICC | 0.92 | 0.93 | 0.93 | 0.88 |
AI | ||||
Reader 1 | 32.4±8.9 | 35.8±10.6 | 50.3±16.2 | 55.0±17.6 |
Reader 2 | 31.2±10.1 | 34.9±11.7 | 49.7±16.4 | 55.0±16.5 |
ICC | 0.82 | 0.82 | 0.70 | 0.79 |
In-stent lumen diameter (mm) | ||||
Reader 1 | 2.18±0.61 | 2.13±0.58 | 2.01±0.56 | 2.05±0.56 |
Reader 2 | 2.19±0.61 | 2.13±0.58 | 2.01±0.56 | 2.05±0.56 |
ICC | 0.99 | 0.99 | 0.99 | 0.99 |
Stent wall thickness (mm) | ||||
Reader 1 | 1.27±0.25 | 1.31±0.24 | 1.41±0.23 | 1.38±0.23 |
Reader 2 | 1.27±0.25 | 1.32±0.24 | 1.42±0.23 | 1.38±0.22 |
ICC | 0.99 | 0.99 | 0.99 | 0.99 |
Data are presented as the mean ± standard deviation. CT, computed tomography; ICC, intraclass correlation; SNR, signal-to-noise ratio; CNR, contrast-to-noise ratio; AI, artifact index; HU, Hounsfield units; mm, millimeter; DLIR, deep learning image reconstruction; DLIR-H, DLIR at a high level; DLIR-M, DLIR at a medium level; ASIR-V, adaptive statistical iterative reconstruction-Veo; ASIR-V50%, ASIR-V at 50% strength; ASIR-V80%, ASIR-V at 80% strength.
Table 3
Parameter | DLIR-H | DLIR-M | ASIR-50% | ASIR-80% |
---|---|---|---|---|
Attenuation (HU) | ||||
AO | ||||
Reader 1 | 592.26±112.0 | 592.07±112.3 | 592.20±112.5 | 592.18±112.4 |
Reader 2 | 593.12±110.6 | 592.73±110.7 | 592.54±111.6 | 592.26±111.6 |
ICC | 0.99 | 0.99 | 0.99 | 0.99 |
LM | ||||
Reader 1 | 570.41±114.1 | 569.97±115.6 | 567.19±118.0 | 567.51±118.5 |
Reader 2 | 579.20±116.0 | 579.04±115.5 | 570.01±132.2 | 576.23±118.4 |
ICC | 0.98 | 0.98 | 0.86 | 0.98 |
LAD | ||||
Reader 1 | 549.45±133.7 | 549.04±133.8 | 546.38±134.9 | 546.32±133.3 |
Reader 2 | 557.77±120.3 | 559.50±120.8 | 557.39±122.1 | 556.92±122.7 |
ICC | 0.96 | 0.96 | 0.96 | 0.96 |
LCX | ||||
Reader 1 | 556.62±119.8 | 557.39±119.0 | 552.31±120.4 | 551.24±120.1 |
Reader 2 | 565.80±121.7 | 565.68±122.1 | 563.23±122.7 | 561.01±122.3 |
ICC | 0.96 | 0.94 | 0.92 | 0.93 |
RCA | ||||
Reader 1 | 568.43±107.2 | 563.45±104.5 | 562.88±104.2 | 563.11±104.7 |
Reader 2 | 569.89±110.1 | 565.03±110.2 | 561.63±109.5 | 560.85±108.7 |
ICC | 0.96 | 0.95 | 0.95 | 0.95 |
Noise (HU) | ||||
AO | ||||
Reader 1 | 21.31±4.3 | 28.27±5.9 | 41.01±9.3 | 34.30±6.9 |
Reader 2 | 21.12±3.4 | 27.54±4.8 | 40.93±8.8 | 33.88±7.0 |
ICC | 0.75 | 0.78 | 0.81 | 0.72 |
LM | ||||
R1 | 19.51±4.3 | 22.65±4.3 | 32.42±6.7 | 25.54±5.8 |
R2 | 20.49±4.8 | 23.01±4.4 | 32.76±6.8 | 26.11±5.7 |
ICC | 0.77 | 0.96 | 0.98 | 0.96 |
LAD | ||||
Reader 1 | 19.96±4.1 | 22.45±5.1 | 29.73±6.2 | 25.07±5.0 |
Reader 2 | 20.24±4.5 | 22.86±5.1 | 30.35±6.4 | 25.26±5.3 |
ICC | 0.94 | 0.94 | 0.96 | 0.96 |
LCX | ||||
Reader 1 | 19.18±4.2 | 21.69±5.3 | 29.12±6.7 | 23.38±5.9 |
Reader 2 | 19.74±4.3 | 22.86±7.3 | 29.88±7.6 | 23.82±7.3 |
ICC | 0.84 | 0.66 | 0.66 | 0.67 |
RCA | ||||
Reader 1 | 18.64±6.2 | 20.89±6.6 | 27.12±7.5 | 22.99±6.9 |
Reader 2 | 18.72±5.3 | 21.92±5.7 | 28.56±6.9 | 23.39±6.4 |
ICC | 0.67 | 0.73 | 0.77 | 0.80 |
SNR | ||||
AO | ||||
Reader 1 | 28.90±6.8 | 21.77±5.6 | 15.05±3.9 | 17.84±4.4 |
Reader 2 | 28.74±6.5 | 22.11±5.2 | 15.01±3.7 | 18.03±4.1 |
ICC | 0.82 | 0.84 | 0.83 | 0.72 |
LM | ||||
Reader 1 | 31.02±10.6 | 26.12±7.3 | 18.18±5.0 | 23.05±6.0 |
Reader 2 | 29.91±9.8 | 26.03±6.9 | 18.03±5.2 | 22.78±5.5 |
ICC | 0.89 | 0.99 | 0.91 | 0.97 |
LAD | ||||
Reader 1 | 28.34±8.3 | 25.55±8.4 | 19.02±6.0 | 22.33±6.3 |
Reader 2 | 28.58±8.3 | 25.52±8.1 | 18.99±5.4 | 22.70±6.2 |
ICC | 0.97 | 0.96 | 0.97 | 0.98 |
LCX | ||||
Reader 1 | 30.51±9.6 | 27.30±8.9 | 19.97±6.2 | 24.96±8.0 |
Reader 2 | 30.00±9.0 | 26.45±8.6 | 20.00±6.6 | 25.22±8.4 |
ICC | 0.84 | 0.79 | 0.77 | 0.77 |
RCA | ||||
Reader 1 | 33.71±12.6 | 29.65±11.0 | 22.39±7.8 | 26.36±8.4 |
Reader 2 | 32.29±9.6 | 27.25±8.3 | 20.67±5.9 | 25.23±7.0 |
ICC | 0.73 | 0.70 | 0.77 | 0.76 |
CNR | ||||
AO | ||||
Reader 1 | 23.90±6.5 | 18.35±5.1 | 13.61±3.8 | 16.89±4.4 |
Reader 2 | 23.91±6.4 | 18.53±5.0 | 13.57±3.7 | 17.00±4.3 |
ICC | 0.97 | 0.96 | 0.93 | 0.91 |
LM | ||||
Reader 1 | 23.73±7.5 | 19.44±6.1 | 14.70±4.7 | 19.27±5.6 |
Reader 2 | 23.71±7.2 | 19.64±5.9 | 14.68±4.8 | 19.35±5.4 |
ICC | 0.96 | 0.99 | 0.91 | 0.98 |
LAD | ||||
Reader 1 | 22.44±8.0 | 18.82±7.4 | 14.50±5.1 | 18.47±6.3 |
Reader 2 | 22.75±7.5 | 19.08±6.9 | 14.72±4.6 | 18.87±5.9 |
ICC | 0.98 | 0.98 | 0.98 | 0.98 |
LCX | ||||
Reader 1 | 23.52±7.8 | 19.47±6.3 | 15.12±5.0 | 19.67±6.4 |
Reader 2 | 23.60±7.5 | 19.37±6.3 | 15.25±5.1 | 19.86±6.5 |
ICC | 0.94 | 0.91 | 0.86 | 0.87 |
RCA | ||||
Reader 1 | 20.55±5.0 | 17.32±4.2 | 16.12±5.4 | 20.54±6.4 |
Reader 2 | 20.54±4.8 | 17.03±4.0 | 15.49±4.7 | 20.05±5.7 |
ICC | 0.90 | 0.89 | 0.94 | 0.91 |
Data are presented as mean ± standard deviation. ICC, intraclass correlation; AO, aortic root; LM, left main artery; LAD, left anterior descending artery; LCX, left circumflex artery; RCA, right coronary artery; SNR, signal-to-noise ratio; CNR, contrast-to-noise ratio; HU, Hounsfield units; DLIR, deep learning image reconstruction; DLIR-H, DLIR at a high level; DLIR-M, DLIR at a medium level; ASIR-V, adaptive statistical iterative reconstruction-Veo; ASIR-V50%, ASIR-V at 50% strength; ASIR-V80%, ASIR-V at 80% strength; R1, reader 1; R2, reader 2.
Furthermore, we compared the quality of the DLIR-H and DLIR-M images for group A to that of the ASIR-V50% and ASIR-V80% images for group B according to the parameters of noise, SNR, and CNR. The results showed that DLIR-H had the lowest noise and highest SNR and CNR, followed by DLIR-M, ASIR-V50%, and ASIRV-80%, with significant differences observed between groups (P=0.04) (Figure 4).
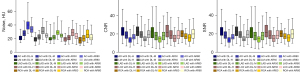
Subjective assessment of image quality
For group A, DLIR-H achieved the highest score in terms of noise in the four image sets, followed by DLIR-M, ASIR-V80%, and ASIR-V50%; DLIR-H and DLIR-M did not differ significantly (P>0.99), but DLIR-H and ASIR-V did (P<0.001). In terms of stent appearance, stent artifacts, and overall image quality, DLIR-H demonstrated the highest score compared to the other methods (Figure 5), with significantly statistical differences observed between DLIR-H and ASIR-V (P=0.007). Concerning diagnostic confidence, DLIR-H, DLIR-M, ASIR-V80%, and ASIRV-50% produced the highest to lowest scores, respectively; DLIR-H and DLIR-M did not differ significantly (P=0.088), but DLIR-H and ASIR-V did (P<0.001).
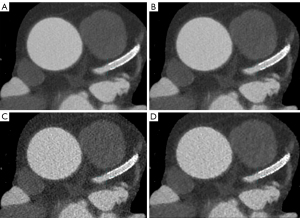
For group B, DLIR-H, DLIR-M, ASIR-V80%, and ASIRV-50% had the highest to lowest scores, respectively, in terms of in terms of noise, vessel sharpness, and overall image quality; there were significant differences between all methods (P=0.004) except for between DLIR-H and DLIR-M (P>0.99).
Tables 4,5 show the subjective image quality measurements of group A and group B, respectively. The interobserver correlation of the measurement was very good to excellent (r=0.66–0.97; P<0.001).
Table 4
Quality score | Reader | DLIR-H (N=76) | DLIR-M (N=76) | ASIR-V50% (N=76) | ASIR-V80% (N=76) |
---|---|---|---|---|---|
Noise | |||||
2 | Reader 1 | 0 | 0 | 3 | 1 |
Reader 2 | 0 | 0 | 3 | 2 | |
3 | Reader 1 | 1 | 3 | 36 | 28 |
Reader 2 | 1 | 3 | 36 | 30 | |
4 | Reader 1 | 17 | 26 | 35 | 43 |
Reader 2 | 19 | 24 | 35 | 41 | |
5 | Reader 1 | 58 | 47 | 2 | 4 |
Reader 2 | 56 | 49 | 2 | 4 | |
Kappa value | 0.93 | 0.95 | 0.95 | 0.95 | |
Stent appearance | |||||
2 | Reader 1 | 0 | 0 | 2 | 1 |
Reader 2 | 0 | 0 | 2 | 1 | |
3 | Reader 1 | 1 | 2 | 33 | 21 |
Reader 2 | 1 | 2 | 34 | 23 | |
4 | Reader 1 | 18 | 38 | 40 | 52 |
Reader 2 | 19 | 39 | 39 | 47 | |
5 | Reader 1 | 57 | 36 | 1 | 2 |
Reader 2 | 56 | 35 | 1 | 5 | |
Kappa value | 0.82 | 0.92 | 0.93 | 0.76 | |
Stent artifacts | |||||
2 | Reader 1 | 0 | 0 | 2 | 1 |
Reader 2 | 0 | 0 | 2 | 1 | |
3 | Reader 1 | 2 | 4 | 33 | 22 |
Reader 2 | 2 | 4 | 35 | 24 | |
4 | Reader 1 | 20 | 41 | 39 | 52 |
Reader 2 | 23 | 40 | 37 | 47 | |
5 | Reader 1 | 54 | 31 | 2 | 1 |
Reader 2 | 51 | 32 | 2 | 4 | |
Kappa value | 0.80 | 0.98 | 0.90 | 0.76 | |
Overall image quality | |||||
2 | Reader 1 | 0 | 0 | 2 | 1 |
Reader 2 | 0 | 0 | 2 | 1 | |
3 | Reader 1 | 2 | 3 | 33 | 22 |
Reader 2 | 2 | 3 | 34 | 25 | |
4 | Reader 1 | 18 | 40 | 40 | 52 |
Reader 2 | 19 | 44 | 38 | 46 | |
5 | Reader 1 | 56 | 33 | 1 | 1 |
Reader 2 | 55 | 29 | 2 | 4 | |
Kappa value | 0.84 | 0.90 | 0.90 | 0.73 | |
Diagnostic confidence | |||||
2 | Reader 1 | 0 | 0 | 8 | 4 |
Reader 2 | 0 | 1 | 8 | 3 | |
3 | Reader 1 | 4 | 7 | 35 | 25 |
Reader 2 | 4 | 6 | 35 | 27 | |
4 | Reader 1 | 12 | 39 | 27 | 36 |
Reader 2 | 14 | 40 | 27 | 34 | |
5 | Reader 1 | 60 | 30 | 6 | 11 |
Reader 2 | 58 | 29 | 6 | 12 | |
Kappa value | 0.86 | 0.82 | 0.92 | 0.86 |
DLIR, deep learning image reconstruction; DLIR-H, DLIR at a high level; DLIR-M, DLIR at a medium level; ASIR-V, adaptive statistical iterative reconstruction-Veo; ASIR-V50%, ASIR-V at 50% strength; ASIR-V80%, ASIR-V at 80% strength.
Table 5
Quality score | DLIR-H (N=74) | DLIR-M (N=74) | ASIR-V50% (N=74) | ASIR-V80% (N=74) | |||||||
---|---|---|---|---|---|---|---|---|---|---|---|
Reader 1 | Reader 2 | Reader 1 | Reader 2 | Reader 1 | Reader 2 | Reader 1 | Reader 2 | ||||
AO | |||||||||||
Noise | |||||||||||
3 | 0 | 0 | 0 | 0 | 7 | 5 | 0 | 0 | |||
4 | 6 | 7 | 14 | 12 | 54 | 56 | 36 | 35 | |||
5 | 68 | 67 | 60 | 62 | 13 | 13 | 38 | 39 | |||
Kappa (value) | 0.92 | 0.81 | 0.80 | 0.86 | |||||||
Vessel sharpness | |||||||||||
3 | 0 | 0 | 0 | 0 | 8 | 7 | 0 | 0 | |||
4 | 6 | 7 | 16 | 15 | 56 | 57 | 37 | 36 | |||
5 | 68 | 67 | 58 | 59 | 10 | 10 | 37 | 38 | |||
Kappa (value) | 0.93 | 0.86 | 0.91 | 0.88 | |||||||
Overall image quality | |||||||||||
3 | 0 | 0 | 0 | 0 | 7 | 6 | 0 | 0 | |||
4 | 6 | 7 | 14 | 12 | 55 | 56 | 36 | 35 | |||
5 | 68 | 67 | 60 | 62 | 12 | 12 | 38 | 39 | |||
Kappa (value) | 0.88 | 0.86 | 0.91 | 0.88 | |||||||
LM | |||||||||||
Noise | |||||||||||
3 | 0 | 0 | 1 | 1 | 14 | 7 | 3 | 1 | |||
4 | 1 | 2 | 12 | 6 | 47 | 57 | 44 | 45 | |||
5 | 73 | 72 | 61 | 67 | 13 | 10 | 27 | 28 | |||
Kappa (value) | 0.66 | 0.66 | 0.61 | 0.87 | |||||||
Vessel sharpness | |||||||||||
3 | 0 | 0 | 1 | 1 | 11 | 11 | 1 | 3 | |||
4 | 5 | 4 | 10 | 8 | 49 | 50 | 41 | 32 | |||
5 | 69 | 70 | 63 | 65 | 14 | 13 | 32 | 39 | |||
Kappa (value) | 0.88 | 0.89 | 0.97 | 0.92 | |||||||
Overall image quality | |||||||||||
3 | 0 | 0 | 1 | 1 | 15 | 11 | 1 | 1 | |||
4 | 2 | 3 | 10 | 8 | 45 | 50 | 39 | 38 | |||
5 | 72 | 71 | 63 | 65 | 14 | 13 | 34 | 35 | |||
Kappa (value) | 0.79 | 0.89 | 0.97 | 0.97 | |||||||
LAD | |||||||||||
Noise | |||||||||||
3 | 0 | 0 | 0 | 0 | 8 | 6 | 0 | 0 | |||
4 | 1 | 2 | 12 | 11 | 59 | 61 | 34 | 32 | |||
5 | 73 | 72 | 62 | 63 | 7 | 7 | 40 | 42 | |||
Kappa (value) | 0.66 | 0.95 | 0.96 | 0.89 | |||||||
Vessel sharpness | |||||||||||
3 | 0 | 0 | 0 | 0 | 7 | 6 | 0 | 0 | |||
4 | 2 | 1 | 12 | 12 | 61 | 61 | 34 | 32 | |||
5 | 72 | 73 | 62 | 62 | 6 | 7 | 40 | 42 | |||
Kappa (value) | 0.80 | 0.90 | 0.91 | 0.89 | |||||||
Overall image quality | |||||||||||
3 | 0 | 0 | 0 | 0 | 7 | 6 | 0 | 0 | |||
4 | 2 | 2 | 12 | 12 | 60 | 61 | 34 | 33 | |||
5 | 72 | 72 | 62 | 62 | 7 | 7 | 40 | 41 | |||
Kappa (value) | 0.81 | 0.85 | 0.96 | 0.92 | |||||||
LCX | |||||||||||
Noise | |||||||||||
3 | 0 | 0 | 0 | 0 | 23 | 21 | 1 | 0 | |||
4 | 3 | 4 | 17 | 14 | 48 | 49 | 48 | 45 | |||
5 | 71 | 70 | 57 | 60 | 5 | 4 | 25 | 29 | |||
Kappa (value) | 0.85 | 0.88 | 0.92 | 0.86 | |||||||
Vessel sharpness | |||||||||||
3 | 0 | 0 | 0 | 0 | 24 | 19 | 2 | 2 | |||
4 | 8 | 9 | 30 | 27 | 46 | 50 | 46 | 41 | |||
5 | 66 | 65 | 44 | 47 | 4 | 5 | 26 | 31 | |||
Kappa (value) | 0.93 | 0.89 | 0.86 | 0.87 | |||||||
Overall image quality | |||||||||||
3 | 0 | 0 | 0 | 0 | 25 | 18 | 2 | 1 | |||
4 | 2 | 3 | 27 | 21 | 45 | 49 | 48 | 43 | |||
5 | 72 | 71 | 47 | 53 | 4 | 7 | 24 | 30 | |||
Kappa (value) | 0.79 | 0.82 | 0.74 | 0.81 | |||||||
RCA | |||||||||||
Noise | |||||||||||
3 | 0 | 0 | 0 | 0 | 8 | 9 | 0 | 0 | |||
4 | 2 | 3 | 12 | 11 | 56 | 54 | 29 | 26 | |||
5 | 72 | 71 | 62 | 63 | 10 | 11 | 45 | 48 | |||
Kappa (value) | 0.79 | 0.96 | 0.94 | 0.83 | |||||||
Vessel sharpness | |||||||||||
3 | 0 | 0 | 0 | 0 | 10 | 10 | 0 | 0 | |||
4 | 3 | 3 | 14 | 13 | 55 | 54 | 30 | 28 | |||
5 | 71 | 71 | 60 | 61 | 9 | 10 | 44 | 46 | |||
Kappa (value) | 0.71 | 0.90 | 0.86 | 0.81 | |||||||
Overall image quality | |||||||||||
3 | 0 | 0 | 0 | 0 | 10 | 10 | 0 | 0 | |||
4 | 2 | 3 | 13 | 11 | 54 | 53 | 30 | 26 | |||
5 | 72 | 71 | 61 | 63 | 10 | 11 | 44 | 48 | |||
Kappa (value) | 0.79 | 0.93 | 0.86 | 0.81 |
AO, aortic root; LM, left main artery; LAD, left anterior descending artery; LCX, left circumflex artery; RCA, right coronary artery; R1, reader 1; R2, reader 2; DLIR, deep learning image reconstruction; DLIR-H, DLIR at a high level; DLIR-M, DLIR at a medium level; ASIR-V, adaptive statistical iterative reconstruction-Veo; ASIR-V50%, ASIR-V at 50% strength; ASIR-V80%, ASIR-V at 80% strength.
Discussion
In this study, we investigated the feasibility of using DLIR (TrueFidelity, GE HealthCare) algorithms to improve the image quality of the stents and coronary images acquired on HD-standard scan modes. The main findings of our study were as follows: (I) the DLIR algorithm could reduce the noise of normal structures, such as the coronary artery and myocardium, and improve the overall image quality under the HD-standard scan mode to generate better images than those of the ASIR-V under the STND scan mode; (II) the DLIR algorithm could maintain the high spatial resolution obtained via the HD-standard scan mode and reduce the metal artifacts of the stent to improve the clarity and visibility of the in-stent lumen; (III) the radiation dose of the HD-standard scan mode was further reduced compared to the STND scan mode.
Previous studies have shown that the HD-standard scan mode provides improved image quality and measurement accuracy for coronary stents compared to the conventional STND scan mode (10-12). The increased spatial resolution of the HD-standard scan mode is achieved by the gemstone detector that utilizes a garnet structure and, coupled with the dynamic focal spot control tube, is the foundation for obtaining high definition images (9). We thus chose to image the coronary stent in the HD-standard scan mode. However, an increased spatial resolution is always associated with an increase in image noise; therefore, we needed to find a noise reduction technology to offset this effect. Filtered back projection (FBP) and IR algorithms were two major algorithms used for CT image reconstruction. FBP is based on a high-pass filter designed to create images in a stable and efficient method, and used to be the most commonly used technique. IR algorithms were developed to reduce the image noise that often occurs in low radiation dose FBP images and have gradually overtaken FBP as the dominant reconstruction technique. Most IR algorithms are operated at moderate strength to balance image noise reduction with maintaining image spatial resolution and image appearance. To further reduce noise or radiation dose, high strength IR algorithms need to be used. However, the use of high-strength IR algorithms sometimes produces a “plastic looking” and over-smoothing, especially at the borders of tissues and blood vessels, thus limiting the use of current IR algorithms (15,16). The DLIR algorithm is based on a deep neural network (DNN) and is trained by comparing reconstructed images from the low-dose raw dataset to ground truth images from high-quality FBP images, optimizing the similarities and differences between the two sets of images in terms of noise, noise texture, low-contrast resolution, and high-contrast spatial resolution to obtain output images that precisely match the ground truth images (19). Furthermore, unlike conventional IR algorithms that contain only a few ten tunable parameters, DLIR contains more than one million adjustable parameters to optimise image generation. As a result, DLIR is more capable of reducing image noise and beam-hardening artefacts, and balancing spatial resolution and image appearance. It has been demonstrated that CCTA reconstructed by different vendor techniques based on deep learning can achieve higher image quality than can traditional image reconstruction (FBP and IR) (23-25), but no previous studies have evaluated the effect of using specific DLIR algorithms on HD-standard scanned CCTA images. One recent study evaluated coronary artery images acquired with the HD-standard mode and the DLIR algorithm (26), but the sample size of the study was insufficiently large (only four stents were evaluated), and STND scan mode was not used for comparison. In contrast, our study evaluated 76 stents with a greater array of evaluation parameters and used the STND scan mode of comparison; in other words, our study further complemented and confirmed these findings. Other research has demonstrated the ability of DLIR to reduce image noise in the myocardium, blood vessels, and pericoronary tissue (17,18,27), and our study is in line with this in in terms of improved image quality, with lower image noise, and a higher SNR and CNR. Furthermore, our data showed that the DLIR algorithms significantly reduced the AI of the stent, decreased noise, and improved pre-stent and in-stent SNR and CNR as compared to the ASIR-V algorithms. Due to the inherent elasticity of the stent retraction, the measured values of ISD were all less than the known true stent diameter (TSD) in four sets of the reconstructed images, but the measurements from DLIR-H images were closest to the TSD, which indicated that the blurring of the stent was the lowest under DLIR, with higher sharpness and fewer blooming artifacts. Accurate visualization of the stent can improve the accuracy of in-stent patency diagnosis. In our study, both readers found that the DLIR-H group showed sharper stent edges and clearer in-stent lumens, giving them more confidence in diagnosing stent restenosis. In fact, out of the stent group, only eight patients underwent digital subtraction angiography (DSA) approximately 1 week after CCTA. Our two readers also compared the diagnostic results of the reconstructed images from the four algorithms with the corresponding DSA results and found that the diagnostic results of the DLIR images were in high agreement with the DSA results. One of these cases involved an in-stent thrombosis in which low-attenuation filling defects were detected in the coronary stent, which is considered to be a direct sign of in-stent restenosis (28); DLIR-H provided the clearest image of the phenomenon among all the modalities.
In addition to the increased noise associated with the higher spatial resolution of the HD-standard scan mode, the tube current in HD-standard scan mode is capped at 600 mA compared to 800 mA in STND scan mode, and the image noise is inversely proportional to the square root of the tube current (29). Therefore, the original images in HD-standard scan mode were noisier and grainier than were the original images in STND mode in our study; however, with the addition of the DLIR algorithm, the HD-standard scan mode images had a lower noise and a higher SNR and CNR than did the images generated under the STND mode with the ASIR algorithm (Figure 6). Furthermore, the radiation dose of patients scanned in HD-standard mode (2.92±0.8 mSv) was significantly lower than that of patients scanned in STND mode (3.42±0.8 mSv; P=0.01). As radiation exposure is associated with cancer risk, it is advisable to perform the examination in HD-standard scan mode to reduce radiation dose exposure.
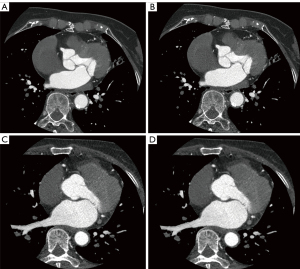
This study has a few limitations that should be mentioned. First, we did not have a sufficiently robust set of invasive coronary angiography results to serve as the gold standard in determining the diagnostic accuracy of stent restenosis. Second, this study focused on stent imaging, but it is unclear whether the HD-standard scan mode with DLIR can play a similar role for severely calcified plaques. Finally, this study was conducted in a single institution and more than one stent per patient was assessed, thus potentially introducing bias in our study. Nevertheless, efforts were made in the design of the study to minimize confounding factors and eliminate observer bias as much as possible. Future studies should be conducted in multiple centers to confirm the unique advantages of HD-standard scan mode with DLIR and potentially broaden its use in clinical work.
Conclusions
The HD-standard scan mode with DLIR-H significantly decreased stent metal artifacts and image noise, improved the overall image quality, and allowed for a reduced radiation dose compared to the STND scan mode with ASIR-V. In future clinical work, the HD-standard scan mode with DLIR algorithm may be used to improve CCTA image quality and reduce patients’ radiation dose.
Acknowledgments
Funding: This work was supported by
Footnote
Reporting Checklist: The authors have completed the GRRAS reporting checklist. Available at https://qims.amegroups.com/article/view/10.21037/qims-23-1064/rc
Conflicts of Interest: All authors have completed the ICMJE uniform disclosure form (available at https://qims.amegroups.com/article/view/10.21037/qims-23-1064/coif). The authors have no conflicts of interest to declare.
Ethical Statement: The authors are accountable for all aspects of the work in ensuring that questions related to the accuracy or integrity of any part of the work are appropriately investigated and resolved. This study was conducted in accordance with the Declaration of Helsinki (as revised in 2013) and was approved by the Ethics Committee of The Third Affiliated Hospital of Sun Yat-sen University. All patients or their family members signed informed consent before coronary CTA was performed. The requirement for informed consent for using the images was waived due to the retrospective nature of this study.
Open Access Statement: This is an Open Access article distributed in accordance with the Creative Commons Attribution-NonCommercial-NoDerivs 4.0 International License (CC BY-NC-ND 4.0), which permits the non-commercial replication and distribution of the article with the strict proviso that no changes or edits are made and the original work is properly cited (including links to both the formal publication through the relevant DOI and the license). See: https://creativecommons.org/licenses/by-nc-nd/4.0/.
References
- Gao Y, Lu B, Hou ZH, et al. Coronary In-Stent Restenosis: Assessment with Corrected Coronary Opacification Difference across Coronary Stents Measured with CT Angiography. Radiology 2015;275:403-12. [Crossref] [PubMed]
- Antoniucci D, Valenti R, Santoro GM, et al. Restenosis after coronary stenting in current clinical practice. Am Heart J 1998;135:510-8. [Crossref] [PubMed]
- Ohnesorge BM, Hofmann LK, Flohr TG, et al. CT for imaging coronary artery disease: defining the paradigm for its application. Int J Cardiovasc Imaging 2005;21:85-104. [Crossref] [PubMed]
- Abdulla J, Abildstrom SZ, Gotzsche O, et al. 64-multislice detector computed tomography coronary angiography as potential alternative to conventional coronary angiography: a systematic review and meta-analysis. Eur Heart J 2007;28:3042-50. [Crossref] [PubMed]
- Wykrzykowska JJ, Arbab-Zadeh A, Godoy G, et al. Assessment of in-stent restenosis using 64-MDCT: analysis of the CORE-64 Multicenter International Trial. AJR Am J Roentgenol 2010;194:85-92. [Crossref] [PubMed]
- Carrabba N, Schuijf JD, de Graaf FR, et al. Diagnostic accuracy of 64-slice computed tomography coronary angiography for the detection of in-stent restenosis: a meta-analysis. J Nucl Cardiol 2010;17:470-8. [Crossref] [PubMed]
- Mahnken AH. CT Imaging of Coronary Stents: Past, Present, and Future. ISRN Cardiol 2012;2012:139823. [Crossref] [PubMed]
- Seifarth H, Ozgün M, Raupach R, et al. 64- Versus 16-slice CT angiography for coronary artery stent assessment: in vitro experience. Invest Radiol 2006;41:22-7. [Crossref] [PubMed]
- Cui X, Li T, Li X, et al. High-definition computed tomography for coronary artery stents imaging: Initial evaluation of the optimal reconstruction algorithm. Eur J Radiol 2015;84:834-9. [Crossref] [PubMed]
- Yang WJ, Zhang H, Xiao H, et al. High-definition computed tomography for coronary artery stents imaging compared with standard-definition 64-row multidectector computed tomography: an initial in vivo study. J Comput Assist Tomogr 2012;36:295-300. [Crossref] [PubMed]
- Min JK, Swaminathan RV, Vass M, et al. High-definition multidetector computed tomography for evaluation of coronary artery stents: comparison to standard-definition 64-detector row computed tomography. J Cardiovasc Comput Tomogr 2009;3:246-51. [Crossref] [PubMed]
- Tanami Y, Jinzaki M, Yamada M, et al. Improvement of in-stent lumen measurement accuracy with new high-definition CT in a phantom model: comparison with conventional 64-detector row CT. Int J Cardiovasc Imaging 2012;28:337-42. [Crossref] [PubMed]
- Leipsic J, Labounty TM, Heilbron B, et al. Adaptive statistical iterative reconstruction: assessment of image noise and image quality in coronary CT angiography. AJR Am J Roentgenol 2010;195:649-54. [Crossref] [PubMed]
- Scheffel H, Stolzmann P, Schlett CL, et al. Coronary artery plaques: cardiac CT with model-based and adaptive-statistical iterative reconstruction technique. Eur J Radiol 2012;81:e363-9. [Crossref] [PubMed]
- Verdun FR, Racine D, Ott JG, et al. Image quality in CT: From physical measurements to model observers. Phys Med 2015;31:823-43. [Crossref] [PubMed]
- Samei E, Richard S. Assessment of the dose reduction potential of a model-based iterative reconstruction algorithm using a task-based performance metrology. Med Phys 2015;42:314-23. [Crossref] [PubMed]
- Benz DC, Benetos G, Rampidis G, et al. Validation of deep-learning image reconstruction for coronary computed tomography angiography: Impact on noise, image quality and diagnostic accuracy. J Cardiovasc Comput Tomogr 2020;14:444-51. [Crossref] [PubMed]
- Tatsugami F, Higaki T, Nakamura Y, et al. Deep learning-based image restoration algorithm for coronary CT angiography. Eur Radiol 2019;29:5322-9. [Crossref] [PubMed]
- Hsieh J, Liu E, Nett B, Tang J, Thibault J-B, Sahney S. A new era of image reconstruction: TrueFidelityTM. Available online: https://www.gehealthcare.com/-/jssmedia/040dd213fa89463287155151fdb01922.pdf
- Greffier J, Hamard A, Pereira F, et al. Image quality and dose reduction opportunity of deep learning image reconstruction algorithm for CT: a phantom study. Eur Radiol 2020;30:3951-9. [Crossref] [PubMed]
- Leipsic J, Nguyen G, Brown J, et al. A prospective evaluation of dose reduction and image quality in chest CT using adaptive statistical iterative reconstruction. AJR Am J Roentgenol 2010;195:1095-9. [Crossref] [PubMed]
- Deak PD, Smal Y, Kalender WA. Multisection CT protocols: sex- and age-specific conversion factors used to determine effective dose from dose-length product. Radiology 2010;257:158-66. [Crossref] [PubMed]
- Liu P, Wang M, Wang Y, et al. Impact of Deep Learning-based Optimization Algorithm on Image Quality of Low-dose Coronary CT Angiography with Noise Reduction: A Prospective Study. Acad Radiol 2020;27:1241-8. [Crossref] [PubMed]
- Hong JH, Park EA, Lee W, et al. Incremental Image Noise Reduction in Coronary CT Angiography Using a Deep Learning-Based Technique with Iterative Reconstruction. Korean J Radiol 2020;21:1165-77. [Crossref] [PubMed]
- Otgonbaatar C, Ryu JK, Shin J, et al. Improvement in Image Quality and Visibility of Coronary Arteries, Stents, and Valve Structures on CT Angiography by Deep Learning Reconstruction. Korean J Radiol 2022;23:1044-54. [Crossref] [PubMed]
- Wang Y, Wang G, Huang X, et al. Improving image quality and resolution of coronary arteries in coronary computed tomography angiography by using high-definition scans and deep learning image reconstruction. Quant Imaging Med Surg 2023;13:2933-40. [Crossref] [PubMed]
- Wang M, Fan J, Shi X, et al. A deep-learning reconstruction algorithm that improves the image quality of low-tube-voltage coronary CT angiography. Eur J Radiol 2022;146:110070. [Crossref] [PubMed]
- Hecht HS, Zaric M, Jelnin V, et al. Usefulness of 64-detector computed tomographic angiography for diagnosing in-stent restenosis in native coronary arteries. Am J Cardiol 2008;101:820-4. [Crossref] [PubMed]
- Birnbaum BA, Hindman N, Lee J, et al. Multi-detector row CT attenuation measurements: assessment of intra- and interscanner variability with an anthropomorphic body CT phantom. Radiology 2007;242:109-19. [Crossref] [PubMed]