Assessing the role of GPT-4 in thyroid ultrasound diagnosis and treatment recommendations: enhancing interpretability with a chain of thought approach
Introduction
Thyroid nodules are the most common endocrine tumors, and in recent years, the incidence of thyroid cancer has been increasing (1). Ultrasound is often the preferred imaging modality for the thyroid due to its non-invasive nature, absence of radiation, and affordability (2). It is used for the staging of the disease, based on Thyroid Imaging-Reporting and Data System proposed by the American College of Radiology (ACR TI-RADS) (3). The accuracy of thyroid cancer diagnosis largely depends on the doctor’s experience, as physicians with varying expertise may conclude with inconsistent results with low repeatability (4).
Last year, open artificial intelligence (AI) introduced ChatGPT (5), a large language model with a notable capacity for text abstraction and comprehension. AI has shown capabilities that are close to or even exceed human performance in abstract and logical reasoning tasks. For instance, AI has achieved remarkable performance in tasks like DeepMind’s AlphaGo mastering the game of Go, Generative Pre-trained Transformer (GPT)-3’s natural language understanding, IBM Watson’s success on Jeopardy, and MuZero’s ability to learn board games without prior knowledge (6-9). AI has significant potential to improve diagnostic accuracy and efficiency while reducing human errors (10). The 2023 release of GPT-4 (11) represents the most powerful language model to date, sparking interest in applying AI to the medical field, particularly for automated medical report analysis (12). However, the limitations of AI models in specialized vertical domains, such as medical diagnosis, include a scarcity of training data in these specific fields, which can hinder the model’s ability to generalize and provide accurate predictions. Additionally, AI models may exhibit poor robustness, leading to increased vulnerability to adversarial examples or noise, which can significantly impact their performance and reliability in complex medical diagnostic tasks (13).
Our goal is to explore the real capabilities, limitations, and potential of GPT-4 in analyzing thyroid cancer ultrasound reports, providing diagnoses, and suggesting treatment plans.
The application of GPT-4 in medical diagnosis, particularly for evaluating thyroid cancer reports, is crucial as it can improve the accuracy, efficiency, and overall patient outcomes of diagnosis. This technology has the potential to save time, reduce costs, reduce human errors, and improve the accessibility of healthcare in remote areas. Furthermore, it encourages continuous learning and promotes collaboration among healthcare professionals, ultimately incorporating AI into clinical practice to enhance the level of patient care.
Methods
Data
In this study, we assessed GPT-4 performance using 109 diverse thyroid cancer cases from Beijing Friendship Hospital, Capital Medical University, between January 2022 and April 2023. These patients underwent thyroid ultrasound examinations. Their diagnoses were confirmed by fine needle aspiration (FNA) or surgical pathology, and the reports were written in Chinese according to the ACR TI-RADS (4) in a free-text style. Ultrasound examinations were conducted using a 3–12 linear probe (RS80A with Prestige, Samsung Medison, Co., Ltd., Seoul, South Korea) and a SL15–4 multi-frequency linear probe (SuperSonic Imagine, Aix-en-Provence, France). The cases included patients of different ages, genders, medical histories, and conditions, such as papillary (102 cases), follicular (3 cases), undifferentiated (2 cases), and medullary carcinoma (2 cases). The study was conducted in accordance with the Declaration of Helsinki (as revised in 2013). Ethical approval for this study was obtained from the Medical Ethics Committee of Beijing Friendship Hospital, Capital Medical University. This was not a study of human subjects. Therefore, informed consent was not required. Forty doctors, of which 13 junior (1–5 years of experience), 17 intermediate (6–10 years), and 10 senior (>10 years), participated in the study. They represented four hospitals—Beijing Friendship Hospital, Capital Medical University, Beijing Children’s Hospital affiliated with Capital Medical University, Binzhou Central Hospital in Shandong Province, and Department of Ultrasound, Peking University Third Hospital, Beijing—ensuring a comprehensive and objective assessment of the performance.
GPT-4 report generation and analysis and platform development
In this study, we hypothesize that an online platform based on GPT-4 can be built to provide evidence-based diagnosis and treatment recommendations based on thyroid ultrasound description reports. We input the ultrasound description reports into the GPT-4 model to generate structured diagnostic reports and treatment plan suggestions for patients.
The GPT series, developed by OpenAI, represents a cutting-edge pre-training language model capable of handling highly complex Natural Language Processing tasks, such as article generation, code generation, machine translation, and questions and answers (Q&A) (14). GPT-4, released in March 2023, is the latest upgrade of the architecture, widely acknowledged as the most advanced AI-generated content (AIGC) model available (7). The research workflow is shown in Figure 1. During our report analysis process, we input ultrasound medical descriptions onto ChatGPT and prompted it to generate diagnosis and treatment recommendations. We subsequently collected the output reports for evaluation. Figure 2 exhibits the examples of collecting questions and answers. The detailed prompt provided was: “According to the following thyroid ultrasound description, please offer a comprehensive diagnosis (TI-RADS classification) and corresponding treatment recommendations”.
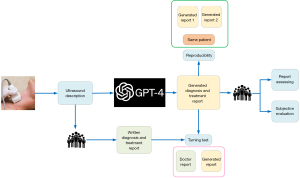
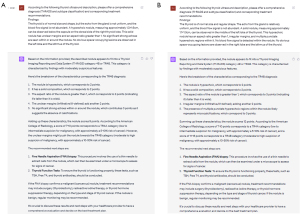
In parallel, to enhance the practicality of our research, we employed the Flask framework (15) to create an intelligent diagnostic platform named “ThyroAIGuide” (Figure 3). ThyroAIGuide is an intuitive online diagnostic tool designed for preliminary thyroid cancer diagnosis. Users are required to input pertinent clinical data and ultrasound image descriptions, after which the platform harnesses the power of the GPT-4 model to generate comprehensive diagnostic reports. These reports include TI-RADS grading, risk assessment, and recommendations on whether a biopsy is necessary. Access to this system is available via the following link: http://47.101.207.206:8000/thyro. Details for “ThyroAIGuide” can be found in Appendix 1.
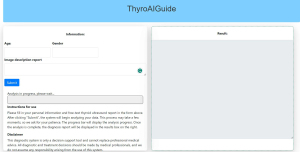
Assessing GPT-4’s performance in report analysis
To gain a deeper understanding of ChatGPT’s accuracy level in generating reports, we collected and analyzed the ratings of the generated reports. Evaluation criteria included diagnostic accuracy (TI-RADS Classification), report structure, use of professional terminology, and clarity of expression. Details of the score table are shown in the Appendix 2.
In addition, we explored the level of expertise that AI-generated reports can achieve by comparing their ratings with those of doctors with different levels of experience. We also conducted an in-depth investigation of erroneous reports.
Turing Test for generated report evaluation
To assess doctors’ ability to discern between human-written and AI-generated reports (16), we randomly included 50% of the AI-generated reports into the evaluation set. The report source was confidential and randomized. Doctors were asked to read the reports and rate the likelihood of human authorship (1= very low, 5= very high). While no specific instructions were provided, doctors typically assessed reports based on language quality, coherence, and medical context relevance. These factors influenced the assigned scores, with higher scores signifying a closer resemblance to human-authored reports.
We collected their judgments and calculated the proportion of correct determinations. If doctors’ accuracy exceeded random guessing (50%), it would imply GPT-4 passed the Turing Test, suggesting its generated reports are nearly indistinguishable from human-written ones.
Report reproducibility experiment restated
To assess the consistency of GPT-4 answers, we compared two responses generated by different temporary model instances to analyze their similarities. For each question, we examined the scores assigned to both responses. We then evaluated the statistical differences between the two sets of answers to determine whether there were significant discrepancies between the two experiments. This evaluation provides insight into the consistency of GPT-4 performance when addressing similar diagnosis.
Subjective evaluation by doctors
Subjective evaluation of clinical diagnostic reports by doctors is crucial for assessing AI-generated report effectiveness (17). We collected feedback from doctors, analyzed it, and classified it into positive (praises AI-generated report for accuracy, clarity, or usefulness), negative (highlights concerns, such as inaccuracies, ambiguities, or irrelevance), or neutral (neither praises nor criticizes; offers general observations or suggestions) categories. To better understand the results, we calculated the proportions of each feedback category, shedding light on the overall sentiment towards the AI-generated reports. To complement the analysis, we employed word cloud methods to visualize high-frequency keywords from the doctors’ feedback. This graphical representation allowed us to quickly identify common themes and areas of concern or praise in the AI-generated reports (18). This approach enabled comprehensive assessment of the clinical impact of AI-generated reports (AIGC reports). Examining doctors’ perspectives helps identify the AI system’s strengths and areas needing refinement to better serve medical professionals’ needs.
GPT Chain of Thought (CoT) visualization
The CoT method breaks down the decision-making process of the GPT model into several stages, each symbolizing a link in the thought chain (19). These chains were then gathered and depicted as a flowchart, offering a clear and insightful way to scrutinize the model’s decision-making patterns. To provide a more detailed walkthrough of the CoT, we took a patient as an example, with the GPT-4 illustrating its thought chain during the diagnostic process. Initially, the CoT tracked how GPT-4 interacted with the given input, following the AI’s process of interpreting the ultrasound description and extracting key information. The visual representation of the thought chain elucidates the decisions made by GPT-4, enhancing our understanding of its decision-making process. This transparency of the AI’s reasoning process also provides clinicians with valuable insight into the AI’s suggestions, fostering trust and better integration of AI advice in the clinical process.
Statistical analyses
The statistical analysis was carried out using the Mann-Whitney U test method (20), provided by the scipy package (21), with all code written in Python 3.8. A P value lower than 0.05 was deemed to be statistically significant.
Results
In this Results section, we summarize our findings on GPT-4’s performance in generating thyroid gland diagnosis reports. We assessed its performance through report scoring, comparison with doctors of varying experience levels, a Turing Test, and consistency analysis. The following sections present the outcomes of these evaluations.
GPT-4 report generation performance evaluation result
We evaluated GPT-4’s performance in creating Chinese medical reports by having doctors of native speaker analyzing them based on diagnostic accuracy, report organization, utilization of professional terminology, clarity of language, and overall assessment. The results are presented in Figure 4A. Based on a 5-point Likert scale, where higher scores indicate better performance, GPT-4 had a diagnostic accuracy mean score of 3.68 [95% confidence interval (CI): 3.52–3.85] and a report structure mean score of 4.28 (95% CI: 4.15–4.40). The AI’s mean score for using professional terminology was 4.28 (95% CI: 4.17–4.38), and for clarity of expression, it achieved a mean score of 4.26 (95% CI: 4.16–4.37). In the general evaluation, GPT-4’s mean score was 3.80 (95% CI: 3.67–3.94). The AI’s performance in report structure, professional terminology, and clarity of expression exceeded a score of 4. The radar chart in Figure 4B displays the average indicators for each group. The chart shows that GPT-4 performed particularly well in report structure, professional terminology, and clarity of expression, with scores exceeding 4. However, the diagnostic accuracy score was slightly lower, at 3.68, indicating room for improvement in this area. Overall, GPT-4’s general evaluation score was 3.80, suggesting that its performance was generally satisfactory. This visualization allows for a quick and comprehensive understanding of the AI’s strengths and areas requiring further development.
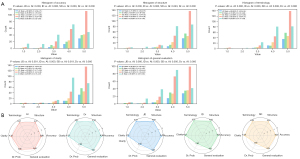
Turing Test results
Figure 5A presents the proportion of correct judgments made by doctors when assessing the origin of the reports. Doctors assigned scores over 3 to 37% of the reports, indicating a higher likelihood of the report being human-written. On the other hand, 71% of the AI-generated reports received scores over 3, with 60.8% of the scores falling in the highest category (class 5). Figure 5B supports this trend, showing the majority of human reports scored in class 1, while AI-generated reports were predominantly scored in class 5. These findings suggest that the AI-generated reports have a high probability of being perceived as human-written.
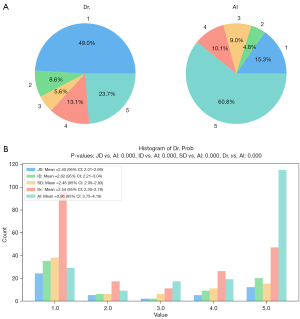
Report reproducibility experiment results
The box diagram in Figure 6 displays the distribution of features for assessing the consistency between two sets of answers generated by GPT-4. The distribution outlooks were observed for accuracy, structure, terminology, clarity, and general evaluation. The P values for each aspect were as follows—accuracy: 0.028, structure: 0.095, terminology: 0.110, clarity: 0.060, and general evaluation: 0.019. These P values revealed varying levels of consistency in the AI-generated reports across the accuracy and general evaluation aspects.
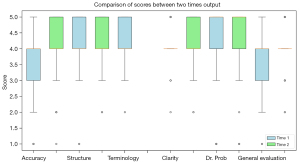
Subjective feedback
The analysis of collected feedback (Figure 7) showed the proportion of negative comments (65%) to be higher than positive (14%) and neutral (20%) evaluations. The most common positive feedback keywords were “detailed” and “clear”, while the prevalent negative feedback terms included “cumbersome”, “misdiagnose”, and “unclear”. The term “professional” frequently appeared in neutral evaluations. Some doctors may have found the AI-generated reports detailed and clear, leading to positive feedback. However, others might have encountered cumbersome or unclear sections, causing them to provide negative feedback. Additionally, some doctors might have focused on the professional terminology used, contributing to the neutral evaluations.
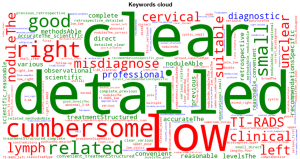
Visualization CoT result
The CoT method was applied in three steps to assess thyroid nodule malignancy risk and propose treatment recommendations (Figure 8). In Step 1, the model extracted and scored data about the thyroid nodules using the TI-RADS standard. In Step 2, it combined demographic data and lymph node information with the TI-RADS score to calculate an overall cancer risk score. In Step 3, the model matched the patient’s risk level with treatment guidelines to propose a suitable treatment plan. Additional factors like medical history, nodule count and location, and symptoms were also considered for a comprehensive risk assessment. The CoT method proved effective in enhancing AI decision-making interpretability in this medical context.
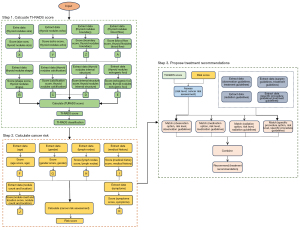
Discussion
In this study, we evaluated GPT-4’s performance in generating thyroid gland diagnosis reports, focusing on report scoring, quality comparison by doctors with different experience levels, Turing Test results, and consistency analysis. Our findings provide valuable insights into GPT-4’s current capabilities, as well as areas for potential improvement and practical applications in the medical field (22,23).
In fact, due to their complex architecture and opaque operations, large language models like GPT are often described as “black boxes” (24). This opacity becomes particularly challenging in the field of medical decision-making, where the interpretability of a model is crucial for gaining the trust of clinicians and effectively integrating AI suggestions into diagnostic and treatment plans. To address this issue, we adopted the CoT method (19), a technique aiming at enhancing the transparency and interpretability of AI model reasoning processes. We uniquely applied the CoT method to deconstruct the decision-making chain of the GPT model in diagnosing thyroid cancer cases. This allows us to track, elucidate, and understand the decision-making process and reasoning logic of the GPT model step by step, thereby revealing the “black box” of AI and systematically dissecting the reasoning path of AI to enhance the interpretability of the model.
GPT-4 report generation performance
The assessment of GPT-4’s performance in generating medical reports reveals both strengths and areas for improvement spanning various aspects of report generation. GPT-4 demonstrated strong capabilities in report structuring, use of professional terminology, and clarity of expression, with scores exceeding 4 in each of these categories. However, GPT-4’s diagnostic accuracy was significantly lower than that of human doctors, as evidenced by the P values and a mean score of 3.68. While the AI showed some diagnostic capability with an 85% accuracy rate for generated answers with scores ≥3, it still falls short of the performance of human doctors in this crucial aspect of medical report generation (25). Conversely, GPT-4 excels in report structuring, using professional terminology, and ensuring clarity of expression: the AI can produce well-structured reports with appropriate professional language and clear communication, which are essential components of effective medical reporting (26).
Turing Test
GPT-4 excels at generating medical reports resembling human-written ones, with potential applications in diagnostics, telemedicine, and patient education. However, medical professionals should monitor AIGC for patient safety and care quality. GPT-4’s performance, though impressive, still reveals occasional discrepancies, such as overly detailed reports. Further development can refine the language model for more accurate and nuanced outputs, increasing the difficulty of distinguishing between human and AI-generated reports (27).
Report reproducibility
The report reproducibility experiment results shed light on GPT-4’s strengths and weaknesses in generating medical reports. GPT-4 demonstrates consistent performance in producing reports with coherent structure, appropriate professional terminology, and clear expression. However, the AI encounters challenges in maintaining diagnostic accuracy and overall evaluation consistency, as evidenced by statistically significant P values below 0.05 for both aspects. These findings emphasize the need to refine GPT-4’s capabilities, focusing on enhancing the reliability and precision of its generated reports for better utility in medical practice (28).
Subjective feedback
Feedback analysis highlights areas for improvement to better meet medical professionals’ needs and expectations. Although GPT-4 excels in generating detailed and clear reports, the prevalence of negative comments suggests challenges remain. Terms like “cumbersome”, “benign”, and “unclear” imply reports may be overly complex, hard to interpret, or lack clarity. Addressing these concerns is vital for enhancing report quality and utility. The term “professional” in neutral evaluations indicates GPT-4’s acceptable professionalism. Refining performance in areas identified by negative feedback will strengthen GPT-4’s role in the medical field, promoting efficient and accurate diagnosis processes (29).
GPT-4 CoT
As demonstrated in this study, the CoT method offers a systematic and transparent approach to understanding the decision-making process of AI models. This method is particularly valuable in a clinical setting where interpretability and trust are crucial for integrating AI into healthcare. From a clinical perspective, the CoT method allows for a comprehensive assessment of thyroid cancer risk. By considering a wide range of factors, including nodule characteristics, patient demographics, lymph node information, as well as other factors such as medical history, nodule count and location, and symptoms, the model can provide a more comprehensive and personalized risk assessment. This could lead to more accurate diagnoses and more targeted treatment plans, thereby improving patient outcomes. Moreover, the CoT method highlights the potential of AI in enhancing the diagnostic process. By automating the extraction and scoring of data and the calculation of risk scores, the model can save clinicians’ time and reduce the possibility of human error. This could be particularly beneficial in high-volume or resource-limited settings where efficiency is paramount. In terms of insights, this study showcases the potential of AI, particularly large language models like GPT-4, in the field of medical diagnosis. However, it also emphasizes the importance of interpretability in these models. As AI continues to advance and become increasingly integrated into healthcare, methods like CoT are crucial for ensuring these models are transparent, trustworthy, and clinically useful (30).
Clinical relevance
Addressing the rising prevalence of thyroid nodules and the need for accurate ultrasound-based diagnoses is crucial, especially given the variability in diagnostic accuracy and repeatability due to doctors’ differing experience levels. This study investigates GPT-4’s potential in the medical field, with a focus on thyroid cancer ultrasound report analysis, to enhance diagnostic outcomes by supporting human expertise. We examine GPT-4’s capabilities in improving diagnostic accuracy, efficiency, and minimizing human error in the diagnostic process. A clear use case for GPT-4 in this setting is assisting radiologists in reviewing and interpreting ultrasound description, providing a second opinion to support decision-making. This AI-assisted approach could foster collaboration among healthcare teams, facilitate continuous learning, and improve healthcare quality and accessibility in remote or underserved areas. Through this investigation, we aim to contribute to the development of a reliable, accessible, and efficient diagnostic tool for clinical practice, ultimately benefiting healthcare professionals and patients by promoting better patient care and outcomes (31). Furthermore, the integration of GPT-4 and the CoT method into the clinical process presents valuable opportunities for improving the diagnostic process. By providing a second opinion in the interpretation of ultrasound descriptions, GPT-4 can aid in identifying critical details that may be overlooked and in preventing diagnostic errors. Meanwhile, the CoT method provides a comprehensive insight into GPT-4’s decision-making process. Understanding how the AI arrived at its conclusions not only enables clinicians to verify the AI’s recommendations but also to incorporate AI insights into their diagnosis more confidently. This transparency ultimately leads to better-informed medical decisions, fostering trust in AI-assisted diagnostic processes. Additionally, understanding the AI’s thought process could provide a basis for continuous learning and improvement, facilitating the development of more refined diagnostic strategies (32).
Online platform
The proposed online platform “ThyroAIGuide”, leveraging AI capabilities, holds substantial potential to transform healthcare delivery, especially in diagnostics. Its accessibility allows patients from remote or underserved areas to access preliminary diagnostic information anytime, anywhere, thereby identifying those requiring urgent care and guiding them towards appropriate resources. The platform’s efficiency lies in its automation of data extraction, scoring, and risk calculation, providing rapid diagnostic insights that save time for both patients and healthcare providers, and potentially hastening the initiation of suitable treatment. Furthermore, the AI-powered platform offers consistency in evaluations, reducing variability that can occur with different healthcare providers, which is particularly beneficial in complex or subjective areas of medicine like imaging study interpretation. Additionally, the platform serves as an educational tool, helping patients better understand their condition and risk factors, thereby empowering them to take a more active role in their healthcare and make informed treatment decisions.
Limitations and future directions
This study highlights GPT-4’s limitations in thyroid diagnosis and suggests future research on specialized models, advanced techniques, and medical partnerships to improve performance. Investigating the AI’s comprehension of medical terminology and context is crucial. One of the more tangible concerns raised is the clinical utility of AI-generated reports. While the system may reduce the time required to generate initial drafts of reports, the added time for proofreading, especially given the model’s current inaccuracies, often offsets this advantage. This dual-edged nature of AI assistance—where time saved in one aspect might be spent in another—needs to be carefully addressed in subsequent versions and applications of the model. Enhancing accuracy is not merely for the sake of reducing errors but also to genuinely save time and effort for medical professionals. Future work should also consider image analysis, time-cost efficiency, and specific diagnostic models to provide a comprehensive evaluation and develop more precise, efficient tools for medical professionals. Our study did not include the process of structuring or digitizing the free text data. Instead, we directly generated results without clear analytical processes, resembling black boxes, which may have led to lower clinical credibility. Moreover, as we transition into a more AI-assisted clinical landscape, it is paramount to ensure that these “black box” models gain the trust of the medical community. Transparency in how the AI reaches its conclusions or the potential integration of explainable AI mechanisms can be pivotal in future iterations. The ultimate goal is to align the efficiency gains from AI with the rigorous demands and standards of medical practice. In future studies, we aim to explore the potential application of GPT in structuring and organizing the data, addressing the limitations identified in our current research.
Conclusions
This study underscores GPT-4’s potential as an auxiliary tool in healthcare, particularly in generating thyroid gland diagnosis reports. Despite its proficiency in report structuring and clarity of expression, the need for further refinement is evident due to limitations in diagnostic accuracy. The proposed online platform “ThyroAIGuide” illustrates the potential of AI in enhancing diagnostic processes and improving healthcare accessibility. The CoT method, applied in this study, provides a systematic and transparent approach to understanding the decision-making process of AI models, thereby addressing the ‘black box’ nature of such large models. However, the journey towards fully integrating AI into healthcare is ongoing, requiring continuous research, development, and careful monitoring by medical professionals to ensure patient safety and quality of care.
Acknowledgments
Funding: This work was supported by
Footnote
Conflicts of Interest: All authors have completed the ICMJE uniform disclosure form (available at https://qims.amegroups.com/article/view/10.21037/qims-23-1180/coif). A.D. reports honoraria for consultancy from the following companies Varian, Janssen, Philips, BMS, Mirada Medical, Medical Data Works B.V. These conflicts of interest did not interfere with the submitted publication. The other authors have no conflicts of interest to declare.
Ethical Statement: The authors are accountable for all aspects of the work in ensuring that questions related to the accuracy or integrity of any part of the work are appropriately investigated and resolved. The study was conducted in accordance with the Declaration of Helsinki (as revised in 2013). Ethical approval for this study was obtained from the Medical Ethics Committee of Beijing Friendship Hospital, Capital Medical University. This was not a study of human subjects. Therefore, informed consent was not required.
Open Access Statement: This is an Open Access article distributed in accordance with the Creative Commons Attribution-NonCommercial-NoDerivs 4.0 International License (CC BY-NC-ND 4.0), which permits the non-commercial replication and distribution of the article with the strict proviso that no changes or edits are made and the original work is properly cited (including links to both the formal publication through the relevant DOI and the license). See: https://creativecommons.org/licenses/by-nc-nd/4.0/.
References
- Durante C, Grani G, Lamartina L, Filetti S, Mandel SJ, Cooper DS. The Diagnosis and Management of Thyroid Nodules: A Review. JAMA 2018;319:914-24. [Crossref] [PubMed]
- Guth S, Theune U, Aberle J, Galach A, Bamberger CM. Very high prevalence of thyroid nodules detected by high frequency (13 MHz) ultrasound examination. Eur J Clin Invest 2009;39:699-706. [Crossref] [PubMed]
- Haugen BR, Alexander EK, Bible KC, Doherty GM, Mandel SJ, Nikiforov YE, Pacini F, Randolph GW, Sawka AM, Schlumberger M, Schuff KG, Sherman SI, Sosa JA, Steward DL, Tuttle RM, Wartofsky L. 2015 American Thyroid Association Management Guidelines for Adult Patients with Thyroid Nodules and Differentiated Thyroid Cancer: The American Thyroid Association Guidelines Task Force on Thyroid Nodules and Differentiated Thyroid Cancer. Thyroid 2016;26:1-133. [Crossref] [PubMed]
- Moon WJ, Baek JH, Jung SL, Kim DW, Kim EK, Kim JY, Kwak JY, Lee JH, Lee JH, Lee YH, Na DG, Park JS, Park SWKorean Society of Thyroid Radiology. (KSThR); Korean Society of Radiology. Ultrasonography and the ultrasound-based management of thyroid nodules: consensus statement and recommendations. Korean J Radiol 2011;12:1-14. [Crossref] [PubMed]
- OpenAI TJO. Chatgpt: Optimizing language models for dialogue. 2022. Available online: https://autogpt.net/chatgpt-optimizing-language-models-for-dialogue/
- Silver D, Huang A, Maddison CJ, Guez A, Sifre L, van den Driessche G, Schrittwieser J, Antonoglou I, Panneershelvam V, Lanctot M, Dieleman S, Grewe D, Nham J, Kalchbrenner N, Sutskever I, Lillicrap T, Leach M, Kavukcuoglu K, Graepel T, Hassabis D. Mastering the game of Go with deep neural networks and tree search. Nature 2016;529:484-9. [Crossref] [PubMed]
- Brown T, Mann B, Ryder N, Subbiah M, Kaplan JD, Dhariwal P, Neelakantan A, Shyam P, Sastry G, Askell A, Agarwal S. Language models are few-shot learners. Advances in Neural Information Processing Systems 2020;33:1877-901.
- Ferrucci D, Brown E, Chu-Carroll J, Fan J, Gondek D, Kalyanpur AA, Lally A, Murdock JW, Nyberg E, Prager J, Schlaefer N, Welty C. Building Watson: An overview of the DeepQA project. AI Magazine 2010;31:59-79.
- Schrittwieser J, Antonoglou I, Hubert T, Simonyan K, Sifre L, Schmitt S, Guez A, Lockhart E, Hassabis D, Graepel T, Lillicrap T, Silver D. Mastering Atari, Go, chess and shogi by planning with a learned model. Nature 2020;588:604-9. [Crossref] [PubMed]
- Rajkomar A, Dean J, Kohane I. Machine Learning in Medicine. N Engl J Med 2019;380:1347-58. [Crossref] [PubMed]
- Koubaa A. GPT-4 vs. GPT-3.5: A Concise Showdown. Preprints 2023, 2023030422. Available online: https://doi.org/
10.20944/preprints202303.0422.v1 - Shickel B, Tighe PJ, Bihorac A, Rashidi P. Deep EHR: A Survey of Recent Advances in Deep Learning Techniques for Electronic Health Record (EHR) Analysis. IEEE J Biomed Health Inform 2018;22:1589-604. [Crossref] [PubMed]
- Esteva A, Robicquet A, Ramsundar B, Kuleshov V, DePristo M, Chou K, Cui C, Corrado G, Thrun S, Dean J. A guide to deep learning in healthcare. Nat Med 2019;25:24-9. [Crossref] [PubMed]
- Jiang F, Jiang Y, Zhi H, Dong Y, Li H, Ma S, Wang Y, Dong Q, Shen H, Wang Y. Artificial intelligence in healthcare: past, present and future. Stroke Vasc Neurol 2017;2:230-43. [Crossref] [PubMed]
- Grinberg M. Flask web development: developing web applications with python[M]. O'Reilly Media, Inc.; 2018.
- French RM. The Turing Test: the first 50 years. Trends Cogn Sci 2000;4:115-22. [Crossref] [PubMed]
- Murdoch TB, Detsky AS. The inevitable application of big data to health care. JAMA 2013;309:1351-2. [Crossref] [PubMed]
- He W, Zha S, Li L. Social media competitive analysis and text mining: A case study in the pizza industry. Int J Inf Manage 2013;33:464-72.
- Wei J, Wang X, Schuurmans D, Bosma M, Ichter B, Xia F, Chi E, Le Q, Zhou D. Chain-of-Thought Prompting Elicits Reasoning in Large Language Models. Part of Advances in Neural Information Processing Systems 35 (NeurIPS 2022); 2022.
- McKnight PE, Najab JJTCeop. Mann‐Whitney U Test. 2010:1-1. Available online: https://doi.org/
10.4135/9781412961288.n228 - Virtanen P, Gommers R, Oliphant TE, Haberland M, Reddy T, Cournapeau D, et al. SciPy 1.0: fundamental algorithms for scientific computing in Python. Nat Methods 2020;17:261-72. [Crossref] [PubMed]
- Gebrael G, Sahu KK, Chigarira B, Tripathi N, Mathew Thomas V, Sayegh N, Maughan BL, Agarwal N, Swami U, Li H. Enhancing Triage Efficiency and Accuracy in Emergency Rooms for Patients with Metastatic Prostate Cancer: A Retrospective Analysis of Artificial Intelligence-Assisted Triage Using ChatGPT 4.0. Cancers (Basel) 2023.
- Secinaro S, Calandra D, Secinaro A, Muthurangu V, Biancone P. The role of artificial intelligence in healthcare: a structured literature review. BMC Med Inform Decis Mak 2021;21:125. [Crossref] [PubMed]
- Petch J, Di S, Nelson W. Opening the Black Box: The Promise and Limitations of Explainable Machine Learning in Cardiology. Can J Cardiol 2022;38:204-13. [Crossref] [PubMed]
- Coskun B, Ocakoglu G, Yetemen M, Kaygisiz O. Can ChatGPT, an Artificial Intelligence Language Model, Provide Accurate and High-quality Patient Information on Prostate Cancer? Urology 2023;180:35-58. [Crossref] [PubMed]
- Vorisek CN, Lehne M, Klopfenstein SAI, Mayer PJ, Bartschke A, Haese T, Thun S. Fast Healthcare Interoperability Resources (FHIR) for Interoperability in Health Research: Systematic Review. JMIR Med Inform 2022;10:e35724. [Crossref] [PubMed]
- Myong Y, Yoon D, Kim BS, Kim YG, Sim Y, Lee S, Yoon J, Cho M, Kim S. Evaluating diagnostic content of AI-generated chest radiography: A multi-center visual Turing test. PLoS One 2023;18:e0279349. [Crossref] [PubMed]
- Sohn E. The reproducibility issues that haunt health-care AI. Nature 2023;613:402-3. [Crossref] [PubMed]
- Liu S, Wright AP, Patterson BL, Wanderer JP, Turer RW, Nelson SD, McCoy AB, Sittig DF, Wright A. Using AI-generated suggestions from ChatGPT to optimize clinical decision support. J Am Med Inform Assoc 2023;30:1237-45. [Crossref] [PubMed]
- Joyce DW, Kormilitzin A, Smith KA, Cipriani A. Explainable artificial intelligence for mental health through transparency and interpretability for understandability. NPJ Digit Med 2023;6:6. [Crossref] [PubMed]
- Frasca F, Piticchio T, Le Moli R, Tumino D, Cannavò S, Ruggeri RM, Campennì A, Giovanella L. Early detection of suspicious lymph nodes in differentiated thyroid cancer. Expert Rev Endocrinol Metab 2022;17:447-54. [Crossref] [PubMed]
- Sharma M, Savage C, Nair M, Larsson I, Svedberg P, Nygren JM. Artificial Intelligence Applications in Health Care Practice: Scoping Review. J Med Internet Res 2022;24:e40238. [Crossref] [PubMed]