Usefulness of longitudinal nodule-matching algorithm in computer-aided diagnosis of new pulmonary metastases on cancer surveillance CT scans
Introduction
The lungs are one of the most common sites for cancers to metastasize. Therefore, the accurate imaging study of lung parenchyma is of clinical importance in preoperative staging and postoperative surveillance. Chest computed tomography (CT) is the most widely used modality for detecting pulmonary metastases, due to its high sensitivity; however, small metastases are often missed at the earliest CT scan and are detected in later CT scans (1). If they are detected at an earlier CT scan as smaller and fewer nodules without other distant metastases, some patients would have a window for different treatment options with better clinical outcome, such as undergoing a modified chemotherapy regimen, or would benefit from pulmonary metastasectomy. Careful selection of pulmonary metastasectomy improves survival rates in a variety of cancers, including colorectal cancer, renal cell carcinoma, breast cancer, otolaryngeal cancer, and uterine malignancies (2-8).
Several efforts have been attempted to improve the pulmonary nodule detection rate by using computer-aided detection (CAD) (9-13). Deep learning-based CAD (DL-CAD) algorithms have recently demonstrated better performance than that of conventional CAD algorithms in detecting small pulmonary nodules on single CT images (14-17). However, in clinical practice, radiologists often assess nodules by comparing sequential CT scans. Specifically, detecting new nodules by comparing surveillance CT scans is crucial because they could potentially be metastases. Nevertheless, this process is a repetitive and time-consuming task that burdens the radiologists’ workload. Therefore, we hypothesized that artificial intelligence (AI)-based solutions capable of automatically assessing the appearance of new nodules with high accuracy would consistently assist radiologists in daily clinical practice. In a previous study (18), a novel algorithm to detect changes in sequential chest X-ray images was suggested. Based on this algorithm, we developed a new algorithm that can match nodules on CT images from different times, and we combined it with a commercially available DL-CAD. In the surveillance CT of patients with cancer whose baseline CT was metastasis-free, new nodules are highly likely to be metastases and knowing whether it is new or pre-existing helps classify nodules as a benign or metastatic nodule. In this setting, we expected that nodule-matching algorithm could assist radiologists in the diagnosis of new pulmonary metastases by automatically categorizing CAD-detected nodules as new or pre-existing. Therefore, we aimed to evaluate the usefulness of our longitudinal nodule-matching algorithm in the CAD of new pulmonary metastases on cancer surveillance CT scans. We present this article in accordance with the STARD reporting checklist (available at https://qims.amegroups.com/article/view/10.21037/qims-23-1174/rc).
Methods
Patient selection
The study was conducted in accordance with the Declaration of Helsinki (as revised in 2013). This retrospective study was approved by institutional review board of Seoul National University Bundang Hospital (IRB No. B-2109-709-103), and individual consent for this retrospective analysis was waived. We selected consecutive patients (n=403) who underwent pulmonary metastasectomy and whose metastases were pathologically confirmed between January 2014 and December 2018 at Seoul National University Bundang Hospital. Two thoracic radiologists (S.H.Y. and Junghoon Kim, with 3 and 6 years of post-training experience, respectively) independently reviewed the CT images and electronic medical records to identify visible but undiagnosed pulmonary metastases. Any disagreements were resolved by discussion and consensus with a third thoracic radiologist (K.W.L., with 25 years of experience). During the image review, patients with CT scans that could potentially interrupt the nodule detection process (e.g., inappropriate image quality, underlying lung disease such as severe interstitial lung disease or emphysema, and previous history of lung surgery) (n=51) and patients without missed pulmonary metastases (n=251) were excluded. Based on the original CT readings, we excluded patients with CT scans in which pulmonary metastases were detected but misinterpreted as benign (n=11). Patients with pulmonary metastases on the first chest CT (n=31) were also excluded. Finally, 59 patients with 65 missed metastases on follow-up CT images were included (Figure 1). The time of the initial CT scan without pulmonary metastasis was designated as Time 1. The time when the pulmonary metastasis was visible in the follow-up CT image but missed by the interpreting radiologist was designated as Time 2.
Image acquisition
CT images were obtained by using a 16-row multidetector CT scanner (Mx8000 IDT; Philips Healthcare, Andover, MA, USA), 64-row multidetector CT scanner [Brilliance 64 and IQon Spectral CT by Philips Healthcare; SOMATOM Definition Edge by Siemens Healthineers (Erlangen, Germany)], or 256-row multidetector CT scanner (iCT 256; Philips Healthcare). We used low-dose unenhanced CT images, which were acquired using a tube voltage of 100–120 kVp and a maximal tube current-time product of 30 mAs with dose modulation. Images were reconstructed using a slice thickness of 1 mm with an increment of 1 mm.
The CAD system and the longitudinal nodule-matching algorithm
The overall workflow of CAD analysis is shown in Figure 2. The nodule-matching CAD system employs two separate steps: nodule detection and longitudinal nodule matching.
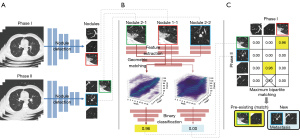
Nodule detection was performed by using a commercially available DL-CAD (LuCAS; Monitor Corporation, Seoul, Korea), which employs convolutional neural network (CNN) models that have demonstrated high performance in relevant applications (19). The LuCAS system was trained on the Lung Image Database Consortium and Image Database Resource Initiative (LIDC/IDRI) database, a publicly available reference for the medical imaging research community. The LIDC/IDRI database contains chest CT scans of 1,018 patients, with annotations of benign and malignant lung nodules by four experienced radiologists (20). This commercially available DL-CAD was employed solely for nodule detection, and no additional training or modifications were performed specifically for this study. The details of the software system are provided in the supplementary appendix. The nodule detection algorithm (Figure 2A) takes three-dimensional (3D) lung CT images from Time 1 and Time 2 as the input to yield a set of center coordinates for nodule candidates. The corresponding cubic patch images are then cropped around these center coordinates. In this study, the target nodule size was assumed to be 30 mm or less, and a cubic patch size of 48 mm was deemed suitable to cover the nodules.
In this study, we used a specialized longitudinal nodule-matching algorithm (Figure 2B) from the same vendor as the DL-CAD algorithm. However, it was not commercially available, being exclusively intended for research purposes. The network architecture closely follows the structure of a previous study (18), with the key difference being the substitution of all two-dimensional (2D) operations with 3D operations. Despite variations in input resolution, the network depth remains consistent because of the fully convolutional nature of the feature extractor in the original network. The only adjustment was adapting the number of nodes in the fully-connected layer that flattens the convolutional feature map for classification score calculation, thereby matching the previous output size. To develop the matching algorithm, 128 CT images from 34 patients were used with 28 images used for training, 3 images used for validation, and 3 images used for testing. Each patient had a minimum of two CT examinations: the positive set consisted of pairings of the identical nodule and the negative set consisted of combinations of different nodules found within the same patient. Interpatient combinations were not considered. A 3D patch image of 48 mm × 48 mm × 48 mm was extracted around the coordinates of the detected nodules. The algorithm takes two patch images from each time to extract feature maps through the squeeze and excitation network (21) and determines whether they represent the identical nodule. We utilized binary cross entropy as the loss function because the algorithm was inherently a binary classification task. The training process was conducted on a GPU machine (TITAN RTX 24GB; NVidia Corporation, Santa Clara, CA, USA), using a batch size of 32. We utilized the Adam optimizer with a learning rate of 0.001 and employed early stopping at the 30-epoch checkpoint where validation loss no longer decreased. In the test set, we achieved a sensitivity of 0.971 and specificity of 0.972, based on an optimal predefined threshold value of 0.126. Pairs with a matching score lower than the predefined threshold were excluded from consideration, and only pairs that exceeded the threshold were selected as matched pairs by using the maximum bipartite matching technique. A nodule patch at Time 2 without a match at Time 1 was considered “new”; otherwise, it was considered “pre-existing” (Figure 2C).
Assessment of DL-CAD and nodule-matching results
Two radiologists (S.H.Y. and Junghoon Kim) independently reviewed the lesions that were detected using CAD at Time 2. These lesions were marked on axial CT images (Figure 3) and viewed using a built-in Digital Imaging and Communications in Medicine (DICOM) viewer. Reviewers classified the lesions as “metastases”, “benign nodules”, or “non-nodules”, based on electronic medical records and follow-up CT images. Non-nodule lesions are lesions that are not actual nodules but are falsely detected as nodules. In cases of pulmonary metastases missed by DL-CAD, the reviewers manually registered them as nodules. The characteristics of all lesions (i.e., metastases, benign nodules, and non-nodules) were recorded, including the longest diameter, location, adjacent parenchymal abnormality, relationship to the pulmonary structures, and matching outcome to nodules detected at Time 1. The location of the nodule was presented in terms of lobar position and centrality. The bilateral upper and right middle lobes were classified as the upper lobes. A nodule located within the outer third of the lung was regarded as peripheral. Any lung parenchymal abnormality around the nodules was also assessed. Reviewers also recorded whether the lesion was in contact with adjacent structures such as the pleura, interlobar fissure, airway, blood vessels, or other structure. If the lesion was not attached to any structures, it was considered isolated.
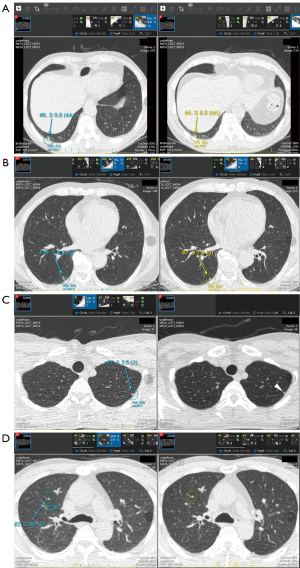
To evaluate the nodule-matching results between the Time 1 and Time 2 CT images by using the longitudinal nodule-matching algorithm, reviewers could compare the two CT images on one screen. The DL-CAD showed matched pairs of nodules on Time 1 and Time 2 CT images, along with information about whether a nodule on the Time 2 CT image was new or pre-existing. The reviewers assessed the matching results and classified matching errors into three categories: (I) mismatching, when a nodule at Time 2 was falsely matched with a different nodule at Time 1; (II) detection failure, when a nodule at Time 2 was not matched with any nodule at Time 1 because the same nodule at Time 1 was not detected; and (III) matching failure, when the same nodules were correctly detected at Time 1 and Time 2, but not matched by the algorithm. Any disagreement during the review was resolved by consensus. The diagnostic performance of DL-CAD was analyzed with and without longitudinal nodule-matching. First, all nodule candidates on Time 2 CT image were regarded as metastasis candidates without nodule matching. Second, by using the longitudinal nodule-matching algorithm, pre-existing Time 2 nodules were regarded as benign and excluded from metastasis candidates. The metastasis candidates confirmed as metastases by the analyses with and without longitudinal comparisons were defined as true-metastasis candidates, whereas metastasis candidates not proven as metastases were considered false-metastasis candidates.
Statistical analysis
Logistic regression was used to assess whether the nodule size, location, parenchymal abnormality of the surrounding lungs, and relationship to the pulmonary structures were significant factors in successful nodule-matching between the Time 1 and Time 2 CT images. The diagnostic performances of the single-time and longitudinal analysis were assessed and compared using metastasis-based sensitivity, positive predictive value (PPV), and false-metastasis candidates per scan. The McNemar test was performed for metastasis candidates per scan and false-metastasis candidates per scan in the single-time and longitudinal analysis. The PPVs were compared by using the weighted generalized score statistic (22). The 95% confidence intervals for metastasis candidates per scan, false-metastasis candidates per scan, sensitivity, and PPV were obtained from 5,000 bootstrap replications. All statistical tests were two-sided and statistical significance was set at P<0.05. Statistical calculations were performed using R 4.1.2 (R Foundation for Statistical Computing, Vienna, Austria).
Results
Review of missed pulmonary metastases at Time 2
The imaging features and primary cancer type of the 65 missed pulmonary metastases at Time 2 are in Table 1. The largest metastasis missed by the original interpreting radiologist was 9.6 mm.
Table 1
Characteristics | Value |
---|---|
Patients | |
Total number | 59 |
Sex | |
Male | 33 (55.9) |
Female | 26 (44.1) |
Age (years) | 67 [56.8–75] |
Primary cancer types | |
Breast | 6 (10.2) |
Renal | 6 (10.2) |
Colorectal | 33 (55.9) |
Uterus | 3 (5.1) |
Sarcoma | 9 (15.3) |
Head and neck | 2 (3.4) |
Pulmonary metastases | |
Total number | 65 |
Lobe | |
Upper | 27 (41.5) |
Lower | 38 (58.5) |
Location | |
Central | 24 (36.9) |
Peripheral | 41 (63.1) |
Isolated nodule | |
Yes | 32 (49.2) |
No | 33 (50.8) |
Adjacent lung disease | |
Yes | 0 |
No | 65 (100.0) |
Diameter, mm | 4.4 [3.7–5.3] |
<3 | 7 (10.8) |
3≤ to <6 | 48 (73.8) |
≥6 | 10 (15.4) |
Data are presented as n (%), or medians [interquartile ranges].
CAD detection of lung nodules at Time 2
A total of 475 lesions were detected by DL-CAD. The lesions were classified as metastases (n=54), benign nodules (n=392), and non-nodules (n=29) after the radiologist review (Table 2).
Table 2
Characteristics | Metastases (n=54) | Benign nodules (n=392) | Non-nodules (n=29) |
---|---|---|---|
Lobe | |||
Upper | 23 (42.6) | 221 (56.4) | 12 (41.4) |
Lower | 31 (57.4) | 171 (43.6) | 17 (58.6) |
Location | |||
Central | 22 (40.7) | 114 (29.1) | 12 (41.4) |
Peripheral | 32 (59.3) | 278 (70.9) | 17 (58.6) |
Isolated nodule | |||
Yes | 28 (51.9) | 186 (47.4) | 0 |
No | 26 (48.1) | 206 (52.6) | 29 (100.0) |
Adjacent lung disease | |||
Yes | 0 | 24 (6.1) | 0 |
No | 54 (100.0) | 368 (93.9) | 29 (100.0) |
Diameter, mm | 4.7 [3.7–5.4] | 4.1 [3.2–5.4] | 4.8 [2.9–7.1] |
<3 | 5 (9.3) | 77 (19.6) | 8 (27.6) |
3≤ to <6 | 40 (74.1) | 248 (63.3) | 11 (37.9) |
≥6 | 9 (16.7) | 67 (17.1) | 10 (34.5) |
Matching outcome | |||
Success | 54 (100.0) | 256 (65.3) | 0 |
Error | 0 | 136 (34.7) | 29 (100.0) |
Detection failures | – | 113 (83.1) | 28 (96.6) |
Mismatching | 0 | 6 (4.4) | 1 (3.4) |
Matching failures | – | 17 (12.5) | 0 |
Data are presented as n (%), or medians [interquartile ranges].
Among the 65 missed metastases on Time 2 CT images, DL-CAD detected 54 (83.1%) metastases (median longest diameter, 4.7 mm). The detection rates of metastases were not significantly different, whether the lesions were in the upper lobes (P>0.99), centrally located (P=0.8), or isolated from adjacent structures (P=0.9). DL-CAD detected 71% (5/7) of metastases that were less than 3 mm in diameter, 83% (40/48) of metastases that were 3–6 mm in diameter, and 90% (9/10) of metastases that were 6 mm or larger. Multivariate logistic regression revealed that nodule size (P=0.049) was the only significant independent predictor of detection, after controlling for other nodule features (Table 3).
Table 3
Variables | Univariate | Multivariate | |||
---|---|---|---|---|---|
OR (95% CI) | P value | OR (95% CI) | P value | ||
Lobe (upper/lower) | |||||
Upper | – | – | |||
Lower | 0.77 (0.18, 2.87) | 0.703 | 0.59 (0.13, 2.43) | 0.474 | |
Diameter | 1.57 (0.94, 2.96) | 0.126 | 2.09 (1.07, 4.77) | 0.049 | |
Location (central/peripheral) | |||||
Central | – | – | |||
Peripheral | 3.09 (0.71, 21.6) | 0.173 | 2.63 (0.54, 19.9) | 0.273 | |
Contact with adjacent structures | 0.53 (0.13, 1.97) | 0.354 | 0.29 (0.05, 1.29) | 0.116 | |
Adjacent parenchymal abnormalities* | N/A | N/A | N/A | N/A |
*, all cases of metastases have no adjacent parenchymal abnormalities. OR, odds ratio; CI, confidence interval; N/A, not applicable.
Non-nodules (n=29) consisted of vessels (n=24), tiny bronchioles (n=2), interlobular septum (n=1), osteophytes (n=1), and cardiac motion artifacts (n=1), which DL-CAD falsely detected as nodules. All non-nodules were considered to be in contact with structures of their origin, after radiologist review.
Nodule matching between Time 1 and Time 2
The interval between Time 1 and Time 2 CT examinations ranged 84–2,266 days (median, 287 days). In the nodule-matching analysis, the algorithm correctly identified all 54 CAD-detected metastases as new lesions. Among the 392 CAD-detected benign nodules, 63 nodules were confirmed as new nodules, and 329 nodules were determined to be pre-existing nodules, after radiologist review. Of the 63 new benign nodules, 61 (96.8%) nodules were correctly classified as new nodules, whereas the remaining two (3.2%) nodules were falsely classified as pre-existing nodules because of mismatching by the nodule-matching algorithm. For the 117 new nodules detected with DL-CAD, which included new benign nodules and metastases, the nodule-matching algorithm correctly classified 115 (98.3%) nodules as new nodules.
Among the 329 pre-existing benign nodules, the nodule-matching algorithm classified 199 (60.5%) nodules as pre-existing. Among these, the nodule-matching algorithm correctly matched and identified 195 nodules as pre-existing. Four nodules were classified as pre-existing, but incorrectly due to mismatching. The nodule-matching algorithm incorrectly classified the remaining 130 nodules as new nodules, which resulted from detection failure (n=113) and matching failure (n=17). All 29 non-nodules detected with DL-CAD were confirmed as pre-existing lesions via radiologist review. The nodule-matching algorithm incorrectly classified 28 of 29 non-nodules as new lesions because of detection failure. The algorithm classified one non-nodule as a pre-existing lesion, but this result was falsely attributed to mismatching. Of note, all non-nodule lesions encountered matching errors in the nodule-matching process. Finally, among the 358 pre-existing benign nodules and non-nodule lesions detected with DL-CAD, the nodule-matching algorithm classified 200 (55.9%) lesions as pre-existing, with 195 (55.6%) lesions correctly classified without matching errors.
The nodule-matching algorithm yielded 165 matching errors, with most errors attributed to detection failures (n=141, 85.4%). Using multivariate logistic regression, contact with adjacent structures (P=0.018) and presence of adjacent parenchymal abnormalities (P=0.012) were significant independent predictors of matching error, after controlling for other nodule features (Table 4). The process of CAD detection and the classification of the lung nodules are shown in Figure 4.
Table 4
Variables | Univariate | Multivariate | |||
---|---|---|---|---|---|
OR (95% CI) | P value | OR (95% CI) | P value | ||
Lobe (upper/lower) | |||||
Upper | – | – | |||
Lower | 0.78 (0.53, 1.14) | 0.197 | 0.78 (0.53, 1.15) | 0.218 | |
Diameter | 0.98 (0.90, 1.06) | 0.632 | 0.94 (0.85, 1.03) | 0.174 | |
Location (central/peripheral) | |||||
Central | – | – | |||
Peripheral | 1.35 (0.90, 2.01) | 0.143 | 1.36 (0.90, 2.05) | 0.138 | |
Contact with adjacent structures | 1.49 (1.02, 2.19) | 0.040 | 1.63 (1.09, 2.45) | 0.018 | |
Adjacent parenchymal abnormalities | 2.41 (1.05, 5.61) | 0.037 | 3.03 (1.28, 7.34) | 0.012 |
OR, odds ratio; CI, confidence interval.
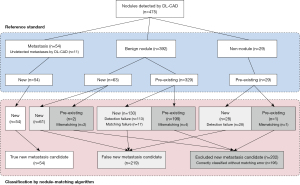
We observed slight differences in the results when CT scanners at Time 1 and Time 2 were the same (n=213) or different (n=273) (Figures S1,S2). Considering any case with a matching error as an inaccurate classification, the accurate classification of new benign nodules was 100% (29 out of 29) when the same CT scanners were used and 94.1% (32 out of 34) when different CT scanners were employed. For pre-existing benign nodules, the accurate classification rates were 65.0% (93 out of 143) and 54.8% (102 out of 186), respectively. For pre-existing non-nodules, the accurate classification rates were 0% (0 out of 15) and 0% (0 out of 14), respectively. The matching error rates were 30.5% (65 out of 213) and 36.6% (100 out of 273), respectively. These differences were not statistically significant (all P>0.05).
Diagnostic performance of DL-CAD and the nodule-matching algorithm for pulmonary metastases
Among the 421 benign lesions detected with DL-CAD, 202 (48.0%) lesions were classified as pre-existing with the nodule-matching algorithm and were subsequently excluded from metastasis candidates. As a result, without any change in sensitivity, the number of false-metastasis candidates per CT scan significantly decreased by 47.9% (P<0.001) from 7.1 (95% CI: 5.1, 9.6) to 3.7 (95% CI: 2.4, 5.2), and the PPV increased significantly (P<0.001) from 11.4% (95% CI: 8.6%, 14.3%) to 19.8% (95% CI: 15.0%, 24.5%) through the utilization of the nodule-matching algorithm. The diagnostic performance of DL-CAD using single Time 2 CT images and the nodule-matching algorithm are compared and summarized in Table 5.
Table 5
Variables | Analysis of single CT image | Analysis using the nodule-matching algorithm | P value |
---|---|---|---|
Metastasis candidates per scan | 8.1 (475/59) [5.9, 10.7] | 4.6 (273/59) [3.3, 6.2] | <0.001 |
False-metastasis candidates per scan | 7.1 (421/59) [5.1, 9.6] | 3.7 (219/59) [2.4, 5.2] | <0.001 |
Sensitivity | 83.1% (54/65) [73.9%, 92.3%] | 83.1% (54/65) [73.9%, 92.3%] | N/A |
PPV | 11.4% (54/475) [8.6%, 14.3%] | 19.8% (54/273) [15.0%, 24.5%] | <0.001 |
The numbers in square brackets are 95% CIs. P values were calculated by using the McNemar test for metastasis candidates per scan and false-metastasis candidates per scan, and by using a weighted generalised score statistic (18). DL-CAD, deep learning-based computer-aided detection; CT, computed tomography; N/A, not applicable; PPV, positive predictive value.
Discussion
In this study, we demonstrated the usefulness of a longitudinal nodule-matching algorithm in the diagnosis of new pulmonary metastasis by using DL-CAD. Among the new pulmonary metastases missed by the interpreting radiologists, DL-CAD detected 83.1% as nodules. The nodule-matching algorithm significantly reduced the number of false-metastasis candidates per scan by 47.9% while maintaining its sensitivity.
With technological advances, CAD is expected to assist radiologists in interpreting chest CT. Previous studies (14,15,23-26) have focused on improving the detection rate of nodule-detection algorithms while reducing false-positive results. Recent studies have also explored using deep learning-based analysis on single chest CT scans to discriminate lung cancer from benign nodules (27-29), and proposed lung cancer prediction through longitudinal analysis (30). A critical objective of chest CT imaging is the detection of metastasis on cancer surveillance, in addition to its role in lung cancer diagnosis. We hypothesized that matching nodules between current and previous CT images could assist radiologists in classifying CAD-detected nodules on cancer surveillance CT scans. In particular, for patients without known pulmonary metastases, the identification of new nodules on serial chest CT scans becomes crucial because they may strongly indicate the presence of metastases. By automatically comparing CT images from different time points, new lesions can be easily differentiated from pre-existing lesions. In our study, we employed the nodule-matching algorithm to identify new pulmonary metastases that were overlooked by the interpreting radiologists. The results of our study suggest that the nodule-matching algorithm used in conjunction with DL-CAD would reduce the effort required to detect new pulmonary metastases.
With regard to the performance in detecting metastases, nodule size was the only significant independent predictor of detection (P<0.05), with larger nodules having higher detection rates. These findings align with those of previous studies focused on pulmonary nodule detection (31-34). The detection rate of DL-CAD for nodules smaller than 3 mm was 71%, surpassing the range of 0–33% reported in one study (32) that utilized several CAD algorithms for detecting missed lung cancers. Chen et al. (1) reported 2.66 mm as the mean size of a missed lung metastasis; when using this criterion, a high detection rate of DL-CAD for nodules smaller than 3 mm would be expected to have significant clinical efficacy. In another study (34), a detection rate of 84.3% was reported for nodules smaller than 5 mm, which aligns closely with our findings of 81.8% for nodules smaller than 6 mm.
The longitudinal nodule-matching algorithm classified 55.9% (200/358) of pre-existing benign nodules and non-nodules as pre-existing lesions. As a result, a significant reduction in the number of metastasis candidates was achieved, accompanied by a decrease in false-metastasis candidates per scan and a significant increase in the PPV (P<0.001). In addition, the occurrence of mismatching errors was minimal with only five (1.7%) cases indicating a low-risk misinterpreting metastases as benign. Our nodule-matching algorithm remarkably resulted in no mismatch for metastases, thus minimizing adverse effect on the diagnosis of metastasis.
Among the matching errors encountered during the nodule detection and matching processes, most were specifically attributed to detection failures (85.5%) rather than to failures in the matching stage. This observation highlights the importance of addressing detection challenges in improving overall performance. Owing to the small size of the targeted lesions, the subtle differences arising from variations in CT machines and parameters between the Time 1 and Time 2 CT images likely contributed to the inconsistency in nodule detection. Minimizing these variations and ensuring a more standardized CT scan protocol are essential for achieving higher consistency in nodule detection and in reducing matching errors resulting from detection failures. However, when excluding the detection failures and focusing solely on the correct matching performance, our nodule-matching algorithm achieved a detection rate of 92.8% (310/334 nodules), which is superior or comparable with that reported in other relevant studies (35,36) that simply focused on the nodule-matching process. Logistic regression analysis revealed that contact with adjacent structures (P=0.02) and the presence of adjacent parenchymal abnormalities (P=0.01) were significant independent predictors of matching error. These two factors are presumed to interfere with the detection process of DL-CAD, considering that detection failure was the major matching error in our study. A previous study (9) has also suggested that contact with normal anatomical structures lowers the CAD detection rate. This finding may explain why the nodule-matching algorithm failed to correctly match all 29 non-nodules (detection failure, n=28; mismatching, n=1). All non-nodules were in contact with adjacent structures because each lesion was a continuation of these structures. Because of this contact, distinguishing lesions from the background and determining whether they are actually nodules are difficult when using DL-CAD, which eventually leads to inconsistent detection. Achieving the precise detection of pulmonary nodules, regardless of adjacent structures and parenchymal changes, emerges as a critical factor in reducing matching errors and enhancing the overall performance of DL-CAD and the subsequent nodule-matching algorithm.
Our study had several limitations. First, this study included a relatively small number of metastases. Despite this limitation, DL-CAD with the nodule-matching algorithm demonstrated its usefulness in diagnosing new metastases, particularly in reducing the number of false-metastasis candidates. Second, we did not include patients with underlying lung diseases such as severe interstitial lung disease and emphysema or a previous history of lung surgery. This decision was made to minimize potential interference of these conditions with our algorithm, especially the nodule detection process. To increase the clinical benefit, the nodule detection and matching algorithms should be trained and tested using the CT images of various patient groups with and without pulmonary parenchymal diseases. Third, we did not incorporate nodule size and volume change in differentiating benign and metastatic nodules. This decision was based on the small size of the missed metastatic nodules, which may introduce low reproducibility in the measurement of size and volume by DL-CAD. To effectively apply these changes in the classification of small pulmonary nodules on cancer surveillance CT scans, achieving higher reproducibility in measuring the size and volume of small nodules by DL-CAD is essential. As a consequence, in our study, we specifically focused on newly developed pulmonary metastases on surveillance CT scans with a metastasis-free baseline CT scan and incorporated the information of whether the nodules were new or pre-existing into the classification process. We believe that this approach of targeting newly developed pulmonary metastases on surveillance CT scans can be applicable in a significant proportion of cancer surveillance cases. Finally, we did not consider the experience level of the original interpreting radiologists. However, this omission is unlikely to have introduced significant bias because missed metastases consistently exhibited small longest diameters (median, 4.4 mm; IQR, 3.7–5.3 mm).
In conclusion, DL-CAD detected new pulmonary metastases that were missed by interpreting radiologists with high sensitivity, and the nodule-matching algorithm significantly lowered the number of false-metastasis candidates without compromising the sensitivity of DL-CAD. The nodule-matching algorithm may potentially reduce radiologists’ workload by decreasing the number of false-metastasis candidates encountered during the computer-assisted interpretation of cancer surveillance chest CT scans.
Acknowledgments
Funding: This work was supported by funding from
Footnote
Reporting Checklist: The authors have completed the STARD reporting checklist. Available at https://qims.amegroups.com/article/view/10.21037/qims-23-1174/rc
Conflicts of Interest: All authors have completed the ICMJE uniform disclosure form (available at https://qims.amegroups.com/article/view/10.21037/qims-23-1174/coif). Co-authors Jihang.K., MD and K.J.L., PhD both are employees and stockholders of Monitor Corporation (Seoul, Korea). The other authors have no conflicts of interest to declare.
Ethical Statement: The authors are accountable for all aspects of the work in ensuring that questions related to the accuracy or integrity of any part of the work are appropriately investigated and resolved. The study was conducted in accordance with the Declaration of Helsinki (as revised in 2013). The study was approved by institutional review board of Seoul National University Bundang Hospital (IRB No. B-2109-709-103) and individual consent for this retrospective analysis was waived.
Open Access Statement: This is an Open Access article distributed in accordance with the Creative Commons Attribution-NonCommercial-NoDerivs 4.0 International License (CC BY-NC-ND 4.0), which permits the non-commercial replication and distribution of the article with the strict proviso that no changes or edits are made and the original work is properly cited (including links to both the formal publication through the relevant DOI and the license). See: https://creativecommons.org/licenses/by-nc-nd/4.0/.
References
- Chen H, Huang S, Zeng Q, Zhang M, Ni Z, Li X, Xu X. A retrospective study analyzing missed diagnosis of lung metastases at their early stages on computed tomography. J Thorac Dis 2019;11:3360-8. [Crossref] [PubMed]
- Petrella F, Diotti C, Rimessi A, Spaggiari L. Pulmonary metastasectomy: an overview. J Thorac Dis 2017;9:S1291-8. [Crossref] [PubMed]
- Kanzaki R, Fukui E, Kanou T, Ose N, Funaki S, Minami M, Shintani Y, Okumura M. Preoperative evaluation and indications for pulmonary metastasectomy. J Thorac Dis 2021;13:2590-602. [Crossref] [PubMed]
- Shimizu H, Endo S, Natsugoe S, Doki Y, Hirata Y, et al. Thoracic and cardiovascular surgery in Japan in 2016: Annual report by The Japanese Association for Thoracic Surgery. Gen Thorac Cardiovasc Surg 2019;67:377-411. [Crossref] [PubMed]
- Kondo H, Okumura T, Ohde Y, Nakagawa K. Surgical treatment for metastatic malignancies. Pulmonary metastasis: indications and outcomes. Int J Clin Oncol 2005;10:81-5. [Crossref] [PubMed]
- Treasure T, Milošević M, Fiorentino F, Macbeth F. Pulmonary metastasectomy: what is the practice and where is the evidence for effectiveness? Thorax 2014;69:946-9. [Crossref] [PubMed]
- Erhunmwunsee L, D'Amico TA. Surgical management of pulmonary metastases. Ann Thorac Surg 2009;88:2052-60. [Crossref] [PubMed]
- Quiros RM, Scott WJ. Surgical treatment of metastatic disease to the lung. Semin Oncol 2008;35:134-46. [Crossref] [PubMed]
- Cai J, Xu D, Liu S, Cham MD. The Added Value of Computer-aided Detection of Small Pulmonary Nodules and Missed Lung Cancers. J Thorac Imaging 2018;33:390-5. [Crossref] [PubMed]
- Logullo P, MacCarthy A, Dhiman P, Kirtley S, Ma J, Bullock G, Collins GS. Artificial intelligence in lung cancer diagnostic imaging: a review of the reporting and conduct of research published 2018-2019. BJR Open 2023;5:20220033. [Crossref] [PubMed]
- Ewals LJS, van der Wulp K, van den Borne BEEM, Pluyter JR, Jacobs I, Mavroeidis D, van der Sommen F, Nederend J. The Effects of Artificial Intelligence Assistance on the Radiologists' Assessment of Lung Nodules on CT Scans: A Systematic Review. J Clin Med 2023;12:3536. [Crossref] [PubMed]
- Chassagnon G, De Margerie-Mellon C, Vakalopoulou M, Marini R, Hoang-Thi TN, Revel MP, Soyer P. Artificial intelligence in lung cancer: current applications and perspectives. Jpn J Radiol 2023;41:235-44. [Crossref] [PubMed]
- Cellina M, Cè M, Irmici G, Ascenti V, Khenkina N, Toto-Brocchi M, Martinenghi C, Papa S, Carrafiello G. Artificial Intelligence in Lung Cancer Imaging: Unfolding the Future. Diagnostics (Basel) 2022.
- da Silva GLF, Valente TLA, Silva AC, de Paiva AC, Gattass M. Convolutional neural network-based PSO for lung nodule false positive reduction on CT images. Comput Methods Programs Biomed 2018;162:109-18. [Crossref] [PubMed]
- Dou Q, Chen H, Yu L, Qin J, Heng PA. Multilevel Contextual 3-D CNNs for False Positive Reduction in Pulmonary Nodule Detection. IEEE Trans Biomed Eng 2017;64:1558-67. [Crossref] [PubMed]
- Li W, Cao P, Zhao D, Wang J. Pulmonary Nodule Classification with Deep Convolutional Neural Networks on Computed Tomography Images. Comput Math Methods Med 2016;2016:6215085. [Crossref] [PubMed]
- Murphy A, Skalski M, Gaillard F. The utilisation of convolutional neural networks in detecting pulmonary nodules: a review. Br J Radiol 2018;91:20180028. [Crossref] [PubMed]
- Oh DY, Kim J, Lee KJ. Longitudinal Change Detection on Chest X-rays Using Geometric Correlation Maps. Medical Image Computing and Computer Assisted Intervention – MICCAI 2019: 22nd International Conference, Shenzhen, China, October 13–17, 2019, Proceedings, Part VI; Shenzhen, China: Springer-Verlag; 2019:748-56.
- Nam CM, Kim J, Lee KJ, editors. Lung nodule segmentation with convolutional neural network trained by simple diameter information. Medical Imaging with Deep Learning; 2018.
- Armato SG 3rd, McLennan G, Bidaut L, McNitt-Gray MF, Meyer CR, Reeves AP, et al. The Lung Image Database Consortium (LIDC) and Image Database Resource Initiative (IDRI): a completed reference database of lung nodules on CT scans. Med Phys 2011;38:915-31. [Crossref] [PubMed]
- Hu J, Shen L, Sun G, editors. Squeeze-and-Excitation Networks. Proceedings of the IEEE Conference on Computer Vision and Pattern Recognition (CVPR); 2018:7132-41.
- Kosinski AS. A weighted generalized score statistic for comparison of predictive values of diagnostic tests. Stat Med 2013;32:964-77. [Crossref] [PubMed]
- Filho AOC, Silva AC, de Paiva AC, Nunes RA, Gattass M. 3D shape analysis to reduce false positives for lung nodule detection systems. Med Biol Eng Comput 2017;55:1199-213. [Crossref] [PubMed]
- Lu X, Gu Y, Yang L, Zhang B, Zhao Y, Yu D, Zhao J, Gao L, Zhou T, Liu Y, Zhang W. Multi-level 3D Densenets for False-positive Reduction in Lung Nodule Detection Based on Chest Computed Tomography. Curr Med Imaging 2020;16:1004-21. [Crossref] [PubMed]
- Gu Y, Lu X, Zhang B, Zhao Y, Yu D, Gao L, Cui G, Wu L, Zhou T. Automatic lung nodule detection using multi-scale dot nodule-enhancement filter and weighted support vector machines in chest computed tomography. PLoS One 2019;14:e0210551. [Crossref] [PubMed]
- Tran GS, Nghiem TP, Nguyen VT, Luong CM, Burie JC. Improving Accuracy of Lung Nodule Classification Using Deep Learning with Focal Loss. J Healthc Eng 2019;2019:5156416. [Crossref] [PubMed]
- Xie Y, Xia Y, Zhang J, Song Y, Feng D, Fulham M, Cai W. Knowledge-based Collaborative Deep Learning for Benign-Malignant Lung Nodule Classification on Chest CT. IEEE Trans Med Imaging 2019;38:991-1004. [Crossref] [PubMed]
- Huang H, Wu R, Li Y, Peng C. Self-Supervised Transfer Learning Based on Domain Adaptation for Benign-Malignant Lung Nodule Classification on Thoracic CT. IEEE J Biomed Health Inform 2022;26:3860-71. [Crossref] [PubMed]
- Yang K, Liu J, Tang W, Zhang H, Zhang R, Gu J, Zhu R, Xiong J, Ru X, Wu J. Identification of benign and malignant pulmonary nodules on chest CT using improved 3D U-Net deep learning framework. Eur J Radiol 2020;129:109013. [Crossref] [PubMed]
- Paez R, Kammer MN, Balar A, Lakhani DA, Knight M, Rowe D, Xiao D, Heideman BE, Antic SL, Chen H, Chen SC, Peikert T, Sandler KL, Landman BA, Deppen SA, Grogan EL, Maldonado F. Longitudinal lung cancer prediction convolutional neural network model improves the classification of indeterminate pulmonary nodules. Sci Rep 2023;13:6157. [Crossref] [PubMed]
- Brown MS, Goldin JG, Suh RD, McNitt-Gray MF, Sayre JW, Aberle DR. Lung micronodules: automated method for detection at thin-section CT--initial experience. Radiology 2003;226:256-62. [Crossref] [PubMed]
- Liang M, Tang W, Xu DM, Jirapatnakul AC, Reeves AP, Henschke CI, Yankelevitz D, Low-Dose CT. Screening for Lung Cancer: Computer-aided Detection of Missed Lung Cancers. Radiology 2016;281:279-88. [Crossref] [PubMed]
- Park EA, Goo JM, Lee JW, Kang CH, Lee HJ, Lee CH, Park CM, Lee HY, Im JG. Efficacy of computer-aided detection system and thin-slab maximum intensity projection technique in the detection of pulmonary nodules in patients with resected metastases. Invest Radiol 2009;44:105-13. [Crossref] [PubMed]
- Li L, Liu Z, Huang H, Lin M, Luo D. Evaluating the performance of a deep learning-based computer-aided diagnosis (DL-CAD) system for detecting and characterizing lung nodules: Comparison with the performance of double reading by radiologists. Thorac Cancer 2019;10:183-92. [Crossref] [PubMed]
- Lee KW, Kim M, Gierada DS, Bae KT. Performance of a computer-aided program for automated matching of metastatic pulmonary nodules detected on follow-up chest CT. AJR Am J Roentgenol 2007;189:1077-81.
- Tao C, Gierada DS, Zhu F, Pilgram TK, Wang JH, Bae KT. Automated matching of pulmonary nodules: evaluation in serial screening chest CT. AJR Am J Roentgenol 2009;192:624-8. [Crossref] [PubMed]