The differential computed tomography features between small benign and malignant solid solitary pulmonary nodules with different sizes
Introduction
Currently, the detection rate of solitary pulmonary nodules, particularly those with smaller sizes, has dramatically increased with the introduction of multidetector computed tomography (CT). Based on the density observed on CT imaging, solitary pulmonary nodules can be divided into sub-solid and solid nodules (1). Patients with solid solitary pulmonary nodules (SSPNs) demonstrate to have a much poorer prognosis than those with sub-solid nodules, partly because that the CT features of malignant SSPN usually mimic benign lesions, resulting in a higher occurrence of missed or delayed diagnosis (2-4). Therefore, analyzing the CT feature differences between benign and malignant SSPNs, especially those with smaller sizes, is vital to increase the correct diagnosis rate and improve patient’s prognosis.
In clinical practice, the differential diagnosis of benign and malignant SSPNs has become controversial (5-9). Previous studies have investigated the imaging features of SSPNs. Swensen et al. (10) confirmed that larger diameter, spiculation, and upper-lobe location of solitary pulmonary nodules were highly associated with malignancy. Li et al. (11) studied 371 surgically resected nodules measuring ≤30 mm and revealed that the possibility of malignancy markedly increased in nodules with older age, a family history of cancer, larger size, and spiculation, whereas calcification was associated with benignancy. Wang et al. (12) reported that lobulation, spiculation, pleural indentation, and vacuole sign were more common in malignant solitary pulmonary nodules, whereas no significant differences were observed in incidences of vessel convergence, air bronchogram, and satellite lesions between benign and malignant groups. Remarkably, some of these findings reported in the literatures are inconsistent. Among these studies, some investigators focused on SPNs of <15 mm, some on those <20 mm, and others on those <30 mm. Zhang et al. (13) established several models to predict lung cancer risk and identified different independent predictors for solid nodules of ≤15 mm and those between 15 and 30 mm. Therefore, we speculated that different outcomes among CT findings of SSPNs may attribute to the fact that these studies seem to ignore the influence of nodule sizes on CT appearances besides different sample sizes. To date, few scholars have sought to compare CT features of small benign and malignant nodules of different sizes. The Lung Imaging Reporting and Data System (Lung-RADS) by the American College of Radiology (14) is a widely accepted tool that standardizes CT reporting and management recommendations. The Lung-RADS classifies SSPNs into four different risk categories (<1%, 1–2%, 5–15%, and >15%) based on the threshold size of 6, 8, and 15 mm.
Therefore, this study aimed to investigate the differential CT features between small (≤15 mm) benign and malignant SSPNs with different sizes sorted using the Lung-RADS, thereby improving the diagnostic accuracy of SSPNs and reducing unnecessary surgical resections of SSPNs. We present this article in accordance with the STROBE reporting checklist (available at https://qims.amegroups.com/article/view/10.21037/qims-23-995/rc).
Methods
Patients
The study was conducted in accordance with the Declaration of Helsinki (as revised in 2013). The study was approved by the ethics committee of the First Affiliated Hospital of Chongqing Medical University, and the need for informed consent was waived because of the retrospective nature of the study. From May 2018 to November 2021, we collected the clinical and imaging data of 980 consecutive patients who underwent chest CT in the First Affiliated Hospital of Chongqing Medical University and met the following criteria: (I) patients who underwent chest CT scans and thin-section CT images were available; (II) those with SSPNs with an average diameter of ≤15 mm; and (III) those with SSPNs confirmed by surgery or CT follow-up. Among them, 186 patients were excluded based on the following exclusion criteria: (I) poor imaging quality due to obvious respiratory motion artifacts; (II) incomplete clinical data. Finally, 794 patients who met the aforementioned criteria were included in this study.
All patients were divided into benign (473 patients) and malignant groups (321 patients). Furthermore, each group was classified into three cohorts based on the nodule size: cohort I (diameter ≤6 mm; 97/794, 12.22%), cohort II (6 mm < diameter ≤8 mm; 118/794, 14.86%), and cohort III (8 mm < diameter ≤15 mm; 579/794, 72.92%). The flow diagram of this study is shown in Figure 1. The clinical characteristics, such as age, sex, smoking history, respiratory symptoms, and family history of malignant tumors, were collected.
Among the 473 benign SSPNs, 340 (71.88%) received surgery soon after the initial CT scan, while the remaining 133 (28.12%) underwent sequential follow-up CTs with a total of 200 CT scans and a median observation period of 437 days (range, 51–1,573 days). With regard to the 133 benign SSPNs, 106 (79.70%) underwent surgical resection ultimately, and 27 (20.30%) were absorbed during follow-ups. Among the 321 malignant SSPNs, 254 (79.13%) underwent operation soon after the initial CT scan, whereas 67 (20.87%) received surgical treatment after follow-up CTs with a total of 268 CT scans and a median observation period of 242 days (range, 30–619 days).
CT scanning protocol
Chest CT scan was performed using one of the three following multidetector CT scanners: Somatom Definition FLASH, Siemens Healthcare (Forchheim, Germany); Somatom Perspective, Siemens Healthcare; and Discovery CT750 HD, GE Healthcare (Milwaukee, WI, USA). All patients underwent helical CT scanning in a single breath-hold from the lung apices to the lung base. Scanning parameters were as follows: tube voltage, 100–120 kVp; tube current, 50–250 mAs (automatic tube current modulation technology); rotation time, 0.5 s; and pitch, 0.875–1.50. A total of 215 patients (27.08%, 215/794) underwent contrast-enhanced CT scanning and were injected with nonionic iodinated contrast material [300 mgI/mL iohexol (Omnipaque, GE Healthcare)] into the antecubital vein using an automated injector at a dose of 1.5 mL/kg of body weight at a rate of 3 mL/s, followed by the administration of 45–50 mL of saline solution. The acquisition time for the arterial and delayed phases was triggered at 30 and 120-180 s, respectively. Thin-section CT images were reconstructed with a slice thickness and interval of 0.625–1.25 mm for the GE scanner and 0.6–1.0 mm for the other two Siemens scanners. All CT images were reconstructed with a sharp kernel in lung window setting [window width, 1,500 Hounsfield unit (HU); window level, −600 HU].
CT image interpretation
Two experienced radiologists with >10 years of experience in chest imaging who were blinded to the clinical and pathologic findings interpreted the CT images in lung window setting using a picture-archiving and communication system workstation (Vue PACS, Carestream, Rochester, NY, USA) independently. Any disagreements were resolved by discussion until a consensus was reached. For nodules with multiple CT scans, initial CT images were included and analyzed. The following CT characteristics were carefully analyzed and recorded:
- Nodule size (the average maximal long-axis diameter and perpendicular maximal short-axis diameter of the nodule in the same plane);
- Location (the left upper and lower lobes as well as the right upper, middle, and lower lobes);
- Shape [polygonal (including triangles, quadrilaterals, and trapezoids, etc.) (Figure 2A-2C) and non-polygonal];
- Margin (spiculation and lobulation);
- Intra-nodular characteristics [airspace (air attenuation within the nodule including vacuole sign and cavity) and calcification];
- Peri-nodular characteristics [halo sign (ill-defined ground-glass opacity surrounding the nodule), satellite lesions (one or more nodular shadows surrounding the dominant nodule with a distance of <3 cm), peripheral fibrosis (linear shadows around the nodule caused by the lesion or preexisting fibrosis), pleural retraction (one or more linear-like or cord-like structures connected between the tumor and pleura with pleural end thickening), bronchial adhering sign (bronchus walked along the edge of SSPN with or without compression of the lumen) (Figure 2D), bronchial truncation (bronchus was blocked and interrupted at the edge of SSPN) (Figure 2E), and air bronchogram (a bronchus entered the SSPN with intact, narrow, rigid, or expansive lumen) (Figure 2F)].
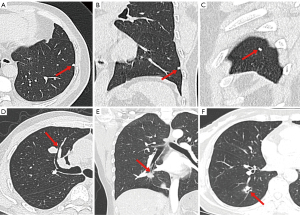
Statistics analyses
Statistical analyses were performed using the statistical analysis system computing software SPSS (version 21.0 for Windows, IBM, Armonk, NY, USA). The diagnostic consistency for CT features between two observers was assessed using the Kappa test, and a Kappa value of >0.80 was considered indicative of a good agreement. When comparing CT features of benign or malignant SSPNs with different sizes, the Chi-square test was performed first. For CT features that differed significantly among the three cohorts, the Bonferroni test was used to perform pairwise comparisons as a post hoc or follow-up analysis.
Multivariable logistic regression analyses (hereinafter referred to as multiparametric analyses) were performed using CT features that differed significantly between both groups in three cohorts to select independent predictors for malignant SSPNs. The final regression models were selected using the enter method and area under the receiver operating characteristic curve (AUC), accuracy, sensitivity, and specificity were used to evaluate the diagnostic performance of models. A two-sided P value of <0.05 was considered statistically significant.
Results
Clinical and pathological manifestations
Totally, 794 patients were included in this study (473 benign SSPNs and 321 malignant SSPNs). The clinical data of patients with benign and malignant SSPNs are displayed in Table 1. The average age of patients with benign SSPNs was 54 years (range, 19–70 years) and that of patients with malignant SSPNs was 60 years (range, 30–83 years). A significant difference in age was observed between the two groups (P<0.001). However, no significant differences in gender, smoking history, respiratory symptoms, and family history of malignant tumors were observed between the two groups (all P>0.05). Among the 446 benign SSPNs confirmed pathologically, 239 (53.59%) were inflammatory lesions, 123 (27.58%) were benign tumors (including 99 pulmonary hamartoma, 16 sclerosing pneumocytoma, 5 solitary fibrous tumor, and 3 pulmonary leiomyoma), 72 (16.14%) were tuberculoma, and 12 (2.69%) were fungal infections. Among the 321 malignant SSPNs (all in pathological stage IA), 292 (90.97%) were adenocarcinoma (including 200 with the acinar-predominant subtype, 55 with the papillary-predominant subtype, 20 with the solid-predominant subtype, and 17 with the micropapillary-predominant subtype), 20 (6.23%) were squamous cell carcinoma, 6 (1.87%) were small cell lung cancer, and 3 (0.93%) were adenosquamous carcinoma.
Table 1
Characteristics | Malignant SSPNs (n=321) | Benign SSPNs (n=473) | P value |
---|---|---|---|
Age (years) | 60±11 | 54±11 | <0.001 |
Sex | |||
Female | 163 (50.8) | 213 (45.0) | 0.111 |
Male | 158 (49.2) | 260 (55.0) | |
Smoking history | 130 (40.5) | 172 (36.4) | 0.239 |
Respiratory symptoms | 85 (26.5) | 125 (26.4) | 0.987 |
Family history of malignant tumors | 35 (10.9) | 47 (9.9) | 0.660 |
The value of age is presented as mean ± SD, and that of other parameters are presented as number (percentage). SSPNs, solid solitary pulmonary nodules; SD, standard deviation.
Consistency assessments
Consistency assessments between the two observers were good for all CT features. Kappa values for the location of lesions, shape, spiculation, lobulation, airspace, calcification, halo sign, satellite lesions, peripheral fibrosis, pleural retraction, bronchial adhering sign, bronchial truncation, and air bronchogram were 1.00, 0.898, 0.862, 0.932, 0.934, 1.00, 0.952, 0.924, 0.887, 0.899, 0.862, 0.895, and 0.911, respectively (all P<0.05).
Comparison of CT features between benign and malignant SSPNs in cohort I
For patients in cohort I, two CT features showed significant differences between both groups (Table 2). Polygonal shape was more common in the benign group (P<0.001), whereas upper-lobe distribution was more common in the malignant group (P=0.016, Figure 3). Multiparametric analysis showed that polygonal shape [adjusted odds ratio (OR): 12.165; 95% confidence interval (CI): 1.512–97.872; P=0.019] was the most effective factor for predicting benign SSPNs, with an AUC of 0.747 (95% CI: 0.640–0.855; P=0.001) and accuracy of 78.35%.
Table 2
Characteristics | Cohort I (n=97) | Cohort II (n=118) | Cohort III (n=579) | |||||||||
---|---|---|---|---|---|---|---|---|---|---|---|---|
Malignant (n=21) | Benign (n=76) | P value | Malignant (n=33) | Benign (n=85) | P value | Malignant (n=267) | Benign (n=312) | P value | ||||
Distribution | ||||||||||||
Upper lobe | 13 (61.9) | 25 (32.9) | 0.016 | 14 (42.4) | 35 (41.2) | 0.902 | 153 (57.3) | 157 (50.3) | 0.093 | |||
Middle/lower lobe | 8 (38.1) | 51 (67.1) | 19 (57.6) | 50 (58.8) | 114 (42.7) | 155 (49.7) | ||||||
Lesion shape | ||||||||||||
Polygonal | 1 (4.8) | 33 (43.4) | <0.001 | 1 (3.0) | 18 (21.2) | 0.016 | 7 (2.6) | 36 (11.5) | <0.001 | |||
Non-polygonal | 20 (95.2) | 43 (56.6) | 32 (97.0) | 67 (78.8) | 260 (97.4) | 276 (88.5) | ||||||
Margin | ||||||||||||
Spiculation | 2 (9.5) | 2 (2.6) | 0.204 | 5 (15.2) | 17 (20.0) | 0.544 | 68 (25.5) | 56 (17.9) | 0.028 | |||
Lobulation | 11 (52.4) | 27 (35.5) | 0.161 | 33 (100.0) | 66 (77.6) | 0.003 | 261 (97.8) | 237 (76.0) | <0.001 | |||
Intra-nodular characteristics | ||||||||||||
Airspace | 0 | 2 (2.6) | >0.99 | 6 (18.2) | 7 (8.2) | 0.222 | 64 (24.0) | 32 (10.3) | <0.001 | |||
Calcification | 0 | 3 (3.9) | >0.99 | 1 (3.0) | 3 (3.5) | >0.99 | 7 (2.6) | 26 (8.3) | 0.003 | |||
Peri-nodular characteristics | ||||||||||||
Halo sign | 0 | 6 (7.9) | 0.414 | 2 (6.1) | 11 (12.9) | 0.457 | 11 (4.1) | 63 (20.2) | <0.001 | |||
Satellite lesions | 0 | 5 (6.6) | 0.516 | 2 (6.1) | 16 (18.8) | 0.084 | 19 (7.1) | 74 (23.7) | <0.001 | |||
Peripheral fibrosis | 3 (14.3) | 26 (34.2) | 0.077 | 11 (33.3) | 39 (45.9) | 0.216 | 157 (58.8) | 146 (46.8) | 0.004 | |||
Pleural retraction | 2 (9.5) | 7 (9.2) | >0.99 | 12 (36.4) | 13 (15.3) | 0.012 | 125 (46.8) | 54 (17.3) | <0.001 | |||
Bronchial adhering sign | 0 | 1 (1.3) | >0.99 | 0 | 6 (7.1) | 0.271 | 7 (2.6) | 34 (10.9) | <0.001 | |||
Bronchial truncation | 2 (9.5) | 2 (2.6) | 0.204 | 4 (12.1) | 6 (7.1) | 0.604 | 94 (35.2) | 31 (9.9) | <0.001 | |||
Air bronchogram | 0 | 3 (3.9) | >0.99 | 10 (30.3) | 11 (12.9) | 0.027 | 93 (34.8) | 42 (13.5) | <0.001 |
CT, computed tomography; SSPNs, solid solitary pulmonary nodules.
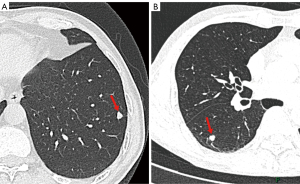
Comparison of CT features between benign and malignant SSPNs in cohort II
For patients in cohort II, four CT features differed significantly between both groups (Table 2). Polygonal shape was more common in benign SSPNs (P=0.016), whereas lobulation, pleural retraction, and air bronchogram were observed more frequently in malignant SSPNs (P=0.003, 0.012, and 0.027, respectively, Figure 4). Multiparametric analysis showed that polygonal shape (OR: 8.870; 95% CI: 1.096–71.772; P=0.041) and the absence of pleural retraction (OR: 0.306; 95% CI: 0.106–0.883; P=0.028) were independent predictors of benign SSPNs, with an AUC of 0.778 (95% CI: 0.694–0.863; P<0.001) and accuracy of 74.58%.
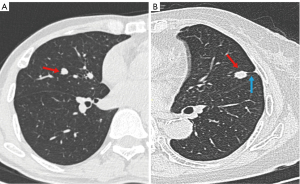
Comparison of CT features between benign and malignant SSPNs in cohort III
For patients in cohort III, 12 CT features differed significantly between both groups (Table 2). Polygonal shape (P<0.001), calcification (P=0.003), halo sign (P<0.001), satellite lesions (P<0.001), and bronchial adhering sign (P<0.001) were favor of benign lesions, while spiculation (P=0.028), lobulation (P<0.001), air space (P<0.001), peripheral fibrosis (P=0.004), pleural retraction (P<0.001), bronchial truncation (P<0.001), and air bronchogram (P<0.001) were favor of malignant SSPNs (Figure 5). Multiparametric analysis showed that polygonal shape (OR: 3.953; 95% CI: 1.508–10.361; P=0.005); calcification (OR: 3.710; 95% CI: 1.305–10.551; P=0.014); halo sign (OR: 6.237; 95% CI: 2.838–13.710; P<0.001); satellite lesions (OR: 6.554; 95% CI: 3.225–13.318; P<0.001); and the absence of lobulation (OR: 0.066; 95% CI: 0.026–0.167; P<0.001), air space (OR: 0.405; 95% CI: 0.215–0.764; P=0.005), pleural retraction (OR: 0.297; 95% CI: 0.179–0.493; P<0.001), bronchial truncation (OR: 0.165; 95% CI: 0.090–0.303; P<0.001), and air bronchogram (OR: 0.363; 95% CI: 0.208–0.633; P<0.001) were independent predictors of benign SSPNs, with an AUC of 0.869 (95% CI: 0.840–0.897; P<0.001) and accuracy of 79.10%.
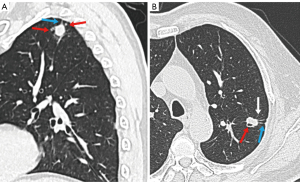
Discussion
Recently, several studies have focused on the differentiation of benign and malignant SSPNs based on morphological imaging and quantitative parameters (15-19). However, most of them seemed not having considered the influence of the nodule size on CT features of SSPNs. In this study, we divided small SSPNs (≤15 mm) into three cohorts (cohort I: diameter ≤6 mm; cohort II: 6–8 mm; and cohort III: 8–15 mm) based on the SSPN classification criteria of Lung-RADS and gained insights into the differential CT features between benign and malignant SSPNs with different sizes. Several major findings were also noted in this study.
Firstly, the present study showed that the smaller the SSPN, the lesser the differential CT signs observed between benign and malignant SSPNs. For benign SSPNs with different sizes, the morphological differences may be attributed to the fact that nodules have various pathological changes in different stages, while for malignancy with different sizes, the morphological differences may be that the tumor invasiveness increases with its size.
For SSPNs with a diameter ≤6 mm, two CT features differed significantly between groups and multiparametric analysis showed that polygonal shape was the most effective variation for predicting benign SSPNs. This pattern was commonly observed in inflammatory nodules and might be best perceptible by multiplanar reconstruction interpretation. We speculated that this sign might be a result of inflammatory absorption. In the healing process, inflammatory lesions will produce some collagen fibers that shrink along the lines of stress (e.g., interstitium), resulting in one or more flat edges along the lung stroma, and then the polygonal shape are formed (20,21). Previous investigators have revealed that the CT pattern of “knife-like change” and polygonal shape usually occur in inflammatory lesions, which are similar to our results (20-22).
For SSPNs with a 6–8-mm-diameter, four CT features differed significantly between the two groups and multiparametric analysis showed polygonal shape and the absence of pleural retraction were independent predictors of benign SSPNs. Moreover, for SSPNs with an 8–15-mm-diameter, more valuable CT features in multiparametric analysis, which could assist in discriminating between malignant and benign SSPNs, were found. Specifically, SSPNs with polygonal shape, calcification, halo sign, and satellite lesions are more likely to indicate benign SSPNs, whereas SSPNs with lobulation, airspace, pleural retraction, bronchial truncation, and air bronchogram increase the suspicion of cancer, which are consistent with some previous studies (23-26). The Lung-RADS suggested that SSPNs of different categories have various risks of malignancy, CT follow-up strategies, and management decisions (27,28). The Fleischner society suggested that high-risk patients with SSPN of diameter <6 mm should consider a follow-up scan, particularly those with suspicious nodule morphology, including irregular or spiculated margins and upper-lobe location (29-31). Recommendations proposed by these guidelines are based mainly on clinical risk factors, nodule sizes, and attenuation; however, morphological characteristics of SSPNs are less involved. Some guideline ambiguities have been observed based on the risk assessment of SSPNs, bringing great challenges to radiologists and other clinicians. As already known, CT plays an important role in discriminating between benign and malignant SSPNs. This study clarified the differential CT features of benign and malignant SSPNs of various sizes in detail, which may serve as a valuable supplementary for the risk evaluation of SSPNs and assist clinicians to early select the optimal management strategy.
Several limitations of this study should be considered. First, given that most patients included in the study underwent surgical resection, some selection biases are inevitable. Second, SSPN heterogeneity with different pathological types in each group was not evaluated. We are planning to compare the CT characteristics of SSPNs among various diseases in each group for a more comprehensive analysis. Finally, we did not analyze the dynamic changes of SSPNs during follow-up CTs and future studies are needed.
Conclusions
In conclusion, the differential CT signs vary between benign and malignant SSPNs with different sizes. Clarifying the differential CT features based on different diameter ranges may help to minimize ambiguities and discriminate the benign SSPNs from malignant ones.
Acknowledgments
Funding: This work was supported by
Footnote
Reporting Checklist: The authors have completed the STROBE reporting checklist. Available at https://qims.amegroups.com/article/view/10.21037/qims-23-995/rc
Conflicts of Interest: All authors have completed the ICMJE uniform disclosure form (available at https://qims.amegroups.com/article/view/10.21037/qims-23-995/coif). The authors have no conflicts of interest to declare.
Ethical Statement: The authors are accountable for all aspects of the work in ensuring that questions related to the accuracy or integrity of any part of the work are appropriately investigated and resolved. The study was conducted in accordance with the Declaration of Helsinki (as revised in 2013). The study was approved by the ethics committee of the First Affiliated Hospital of Chongqing Medical University and individual consent for this retrospective analysis was waived.
Open Access Statement: This is an Open Access article distributed in accordance with the Creative Commons Attribution-NonCommercial-NoDerivs 4.0 International License (CC BY-NC-ND 4.0), which permits the non-commercial replication and distribution of the article with the strict proviso that no changes or edits are made and the original work is properly cited (including links to both the formal publication through the relevant DOI and the license). See: https://creativecommons.org/licenses/by-nc-nd/4.0/.
References
- Hansell DM, Bankier AA, MacMahon H, McLoud TC, Müller NL, Remy J. Fleischner Society: glossary of terms for thoracic imaging. Radiology 2008;246:697-722. [Crossref] [PubMed]
- Patel VK, Naik SK, Naidich DP, Travis WD, Weingarten JA, Lazzaro R, Gutterman DD, Wentowski C, Grosu HB, Raoof S. A practical algorithmic approach to the diagnosis and management of solitary pulmonary nodules: part 1: radiologic characteristics and imaging modalities. Chest 2013;143:825-39. [Crossref] [PubMed]
- Pinsky PF, Gierada DS, Nath PH, Munden R. Lung Cancer Risk Associated With New Solid Nodules in the National Lung Screening Trial. AJR Am J Roentgenol 2017;209:1009-14. [Crossref] [PubMed]
- Wang X, Han R, Guo F, Li X, Zheng W, Wang Q, Song W, Yu T, Wang Y. Analysis of Growth Curve Type in Pulmonary Nodules with Different Characteristics. Zhongguo Fei Ai Za Zhi 2017;20:334-40. [Crossref] [PubMed]
- Chu ZG, Zhang Y, Li WJ, Li Q, Zheng YN, Lv FJ. Primary solid lung cancerous nodules with different sizes: computed tomography features and their variations. BMC Cancer 2019;19:1060. [Crossref] [PubMed]
- Walter JE, Heuvelmans MA, Bock GH, Yousaf-Khan U, Groen HJM, Aalst CMV, Nackaerts K, Ooijen PMAV, Koning HJ, Vliegenthart R, Oudkerk M. Characteristics of new solid nodules detected in incidence screening rounds of low-dose CT lung cancer screening: the NELSON study. Thorax 2018;73:741-7. [Crossref] [PubMed]
- Walter JE, Heuvelmans MA, de Jong PA, Vliegenthart R, van Ooijen PMA, Peters RB, Ten Haaf K, Yousaf-Khan U, van der Aalst CM, de Bock GH, Mali W, Groen HJM, de Koning HJ, Oudkerk M. Occurrence and lung cancer probability of new solid nodules at incidence screening with low-dose CT: analysis of data from the randomised, controlled NELSON trial. Lancet Oncol 2016;17:907-16. [Crossref] [PubMed]
- Xu DM, van Klaveren RJ, de Bock GH, Leusveld AL, Dorrius MD, Zhao Y, Wang Y, de Koning HJ, Scholten ET, Verschakelen J, Prokop M, Oudkerk M. Role of baseline nodule density and changes in density and nodule features in the discrimination between benign and malignant solid indeterminate pulmonary nodules. Eur J Radiol 2009;70:492-8. [Crossref] [PubMed]
- Sim YT, Poon FW. Imaging of solitary pulmonary nodule-a clinical review. Quant Imaging Med Surg 2013;3:316-26. [Crossref] [PubMed]
- Swensen SJ, Silverstein MD, Ilstrup DM, Schleck CD, Edell ES. The probability of malignancy in solitary pulmonary nodules. Application to small radiologically indeterminate nodules. Arch Intern Med 1997;157:849-55.
- Li Y, Chen KZ, Wang J. Development and validation of a clinical prediction model to estimate the probability of malignancy in solitary pulmonary nodules in Chinese people. Clin Lung Cancer 2011;12:313-9. [Crossref] [PubMed]
- Wang X, Lv L, Zheng Q, Huang X, Li B. Differential diagnostic value of 64-slice spiral computed tomography in solitary pulmonary nodule. Exp Ther Med 2018;15:4703-8. [Crossref] [PubMed]
- Zhang R, Tian P, Chen B, Zhou Y, Li W. Predicting Lung Cancer Risk of Incidental Solid and Subsolid Pulmonary Nodules in Different Sizes. Cancer Manag Res 2020;12:8057-66. [Crossref] [PubMed]
- Chelala L, Hossain R, Kazerooni EA, Christensen JD, Dyer DS, White CS. Lung-RADS Version 1.1: Challenges and a Look Ahead, From the AJR Special Series on Radiology Reporting and Data Systems. AJR Am J Roentgenol 2021;216:1411-22. [Crossref] [PubMed]
- Xu DM, van Klaveren RJ, de Bock GH, Leusveld A, Zhao Y, Wang Y, Vliegenthart R, de Koning HJ, Scholten ET, Verschakelen J, Prokop M, Oudkerk M. Limited value of shape, margin and CT density in the discrimination between benign and malignant screen detected solid pulmonary nodules of the NELSON trial. Eur J Radiol 2008;68:347-52. [Crossref] [PubMed]
- Larici AR, Farchione A, Franchi P, Ciliberto M, Cicchetti G, Calandriello L, Del Ciello A, Bonomo L. Lung nodules: size still matters. Eur Respir Rev 2017;26:170025. [Crossref] [PubMed]
- Mazzone PJ, Lam L. Evaluating the Patient With a Pulmonary Nodule: A Review. JAMA 2022;327:264-73. [Crossref] [PubMed]
- Ni Y, Xie Z, Zheng D, Yang Y, Wang W. Two-stage multitask U-Net construction for pulmonary nodule segmentation and malignancy risk prediction. Quant Imaging Med Surg 2022;12:292-309. [Crossref] [PubMed]
- Wen Q, Yue Y, Shang J, Lu X, Gao L, Hou Y. The application of dual-layer spectral detector computed tomography in solitary pulmonary nodule identification. Quant Imaging Med Surg 2021;11:521-32. [Crossref] [PubMed]
- Ahn MI, Gleeson TG, Chan IH, McWilliams AM, Macdonald SL, Lam S, Atkar-Khattra S, Mayo JR. Perifissural nodules seen at CT screening for lung cancer. Radiology 2010;254:949-56. [Crossref] [PubMed]
- de Hoop B, van Ginneken B, Gietema H, Prokop M. Pulmonary perifissural nodules on CT scans: rapid growth is not a predictor of malignancy. Radiology 2012;265:611-6. [Crossref] [PubMed]
- Xu DM, van der Zaag-Loonen HJ, Oudkerk M, Wang Y, Vliegenthart R, Scholten ET, Verschakelen J, Prokop M, de Koning HJ, van Klaveren RJ. Smooth or attached solid indeterminate nodules detected at baseline CT screening in the NELSON study: cancer risk during 1 year of follow-up. Radiology 2009;250:264-72. [Crossref] [PubMed]
- McWilliams A, Tammemagi MC, Mayo JR, Roberts H, Liu G, Soghrati K, et al. Probability of cancer in pulmonary nodules detected on first screening CT. N Engl J Med 2013;369:910-9. [Crossref] [PubMed]
- Qu H, Zhang W, Yang J, Jia S, Wang G. The value of the air bronchogram sign on CT image in the identification of different solitary pulmonary consolidation lesions. Medicine (Baltimore) 2018;97:e11985. [Crossref] [PubMed]
- Lin RY, Lv FJ, Fu BJ, Li WJ, Liang ZR, Chu ZG. Features for Predicting Absorbable Pulmonary Solid Nodules as Depicted on Thin-Section Computed Tomography. J Inflamm Res 2021;14:2933-9. [Crossref] [PubMed]
- Jiang B, Takashima S, Miyake C, Hakucho T, Takahashi Y, Morimoto D, Numasaki H, Nakanishi K, Tomita Y, Higashiyama M. Thin-section CT findings in peripheral lung cancer of 3 cm or smaller: are there any characteristic features for predicting tumor histology or do they depend only on tumor size? Acta Radiol 2014;55:302-8.
- McKee BJ, Regis SM, McKee AB, Flacke S, Wald C. Performance of ACR Lung-RADS in a clinical CT lung screening program. J Am Coll Radiol 2015;12:273-6. [Crossref] [PubMed]
- Dyer SC, Bartholmai BJ, Koo CW. Implications of the updated Lung CT Screening Reporting and Data System (Lung-RADS version 1.1) for lung cancer screening. J Thorac Dis 2020;12:6966-77. [Crossref] [PubMed]
- MacMahon H, Naidich DP, Goo JM, Lee KS, Leung ANC, Mayo JR, Mehta AC, Ohno Y, Powell CA, Prokop M, Rubin GD, Schaefer-Prokop CM, Travis WD, Van Schil PE, Bankier AA. Guidelines for Management of Incidental Pulmonary Nodules Detected on CT Images: From the Fleischner Society 2017. Radiology 2017;284:228-43. [Crossref] [PubMed]
- Bueno J, Landeras L, Chung JH. Updated Fleischner Society Guidelines for Managing Incidental Pulmonary Nodules: Common Questions and Challenging Scenarios. Radiographics 2018;38:1337-50. [Crossref] [PubMed]
- Gould MK, Donington J, Lynch WR, Mazzone PJ, Midthun DE, Naidich DP, Wiener RS. Evaluation of individuals with pulmonary nodules: when is it lung cancer? Diagnosis and management of lung cancer, 3rd ed: American College of Chest Physicians evidence-based clinical practice guidelines. Chest 2013;143:e93S-e120S.