Global trends in neonatal MRI brain neuroimaging research over the last decade: a bibliometric analysis
Introduction
Neuroimaging plays a central role in the evaluation, treatment, and prognosis of neonates. In recent years, the exploration of the developing brain has been a major focus of research for researchers and clinicians, and magnetic resonance imaging (MRI) non-invasive neuroimaging methods in particular are critical in demonstrating the link between the brain and behavioral changes in neonates and infants (1,2). MRI not only indirectly reflects the complex dynamic processes observed at the molecular and cellular levels, but also provides information on brain morphology, structural connectivity, microstructural properties of gray and white matter, and brain functional structure (3-5).
Bibliometrics can be used to understand the frontier dynamics and development trend of the profession or research field of neuroimaging through the reading of professional literature, which will help researchers to predict the future research trend (6-11). Therefore, this study endeavored to use bibliometrics methods to statistically analyze the research status of neonatal MRI brain neuroimaging in the past decade, and to evaluate the research hotspot and current status in this field.
Methods
Data sources and search strategies
The Web of Science Core Collection (WoSCC) is widely used because of its ability to provide comprehensive and standardized datasets. In our study, the dataset was based on WoSCC literature retrieval from 2013 to 2022. Original articles and reviews written in English were included in the study. The search terms were as follows: (TS=[newborn OR neonate OR neonatal OR term infant full OR term infant normal OR term infant OR full-term baby OR premature infant OR preterm infant OR prematurity OR post-term infant]) AND (TS=[magnetic resonance imaging OR MRI OR neuroimaging OR functional neuroimaging OR functional magnetic resonance imaging OR functional MRI OR fMRI]) AND (TS=[brain OR cerebrum]). The inclusion criteria were as follows: (I) the literature dataset in this study was based on WoSCC search; (II) the time of literature retrieval was 2013–2022; (III) original papers and reviews written in English. The exclusion criteria were as follows: (I) non-English language; (II) articles that had been published online, meeting abstracts, editorial materials, proceedings papers, book chapters, letters, revisions, and republications. A total of 3,568 documents were finally collected, including 3,206 articles and 362 reviews. Due to the rapid updating of the WoSCC database, data collected on different dates may lead to deviation of results. Therefore, all data in this paper were retrieved on the same day (16 January 2023). The retrieval flow chart of this study is shown in Figure 1.
Data collection
First, we extracted the raw data from the WoSCC database. Detailed records were made of raw data information, including number of publications (Np), citations, year of publication, author, country/region, institution, H-index, references, journals, and keywords. Although inaccurate analysis may not have been completely avoided due to the same abbreviations being used by different authors or multiple versions of cited references, most of the raw data in our study is considered reliable. The data are analyzed using VOSviewer v.1.6.10 (Leiden University, Leiden, Netherlands; https://www.vosviewer.com/) and CiteSpace 6.1.R6 (Drexel University, Philadelphia, PA, USA; http://cluster.cis.drexel.edu/~cchen/citespace/).
Through the statistical analysis function of the WoS system, the annual Np, countries, journals, authors, institutions, and citations were quantitatively analyzed, and Excel software (Microsoft, Redmond, WA, USA) was used to draw charts. In addition, the relevant information of the above 3,568 documents from the WoSCC database was exported and saved in txt format. The cited literature and keywords were analyzed by CiteSpace software to clarify the research hotspots in different periods and future research trends.
Bibliometric analysis
The basic features of publications were retrieved using the intrinsic functions of WoSCC. The quantity and quality of research results were evaluated from the indicators such as the Np, citation frequency, and impact factors (IFs). In this study, Np was used to quantify yield, and number of citations (Nc) was used to show the impact. The Journal Citation Reports (JCR) partition and IF of journals are important indicators to evaluate the academic level of journals and the quality of papers. H-index, also known as h-factor, is a new method for evaluating academic achievement: “h” represents “high citations”. The H-index of a researcher means that at most h papers of his are cited at least h times. The H-index can accurately reflect a person’s academic achievement. The higher a person’s H-index, the greater the influence of his paper. Global Citation Score (GCS) is the Nc of an article in the world. A high GCS of an article indicates that the related field is much concerned by global scientists.
Taking time (year) as the independent variable and the number of documents as the dependent variable, the number of documents and the annual Np were predicted by the fitting curve, so as to reflect the change of annual publication volume. In this study, VOSviewer and CiteSpace software were used to construct bibliometric maps to obtain more comprehensive results according to co-occurrence and co-citation (12,13). VOSviewer v.1.6.10 was used to construct and visualize a bibliometric network diagram. In this study, VOSviewer was used for co-occurrence analysis, whereby the size of the node represents Np, the thickness of the line represents the strength of the relationship, and the color of the node represents different clusters or periods. CiteSpace uses cluster analysis, timeline or time zone view, references, keyword citation bursts, and other methods to visually evaluate knowledge fields and development trends. Cluster analysis can classify references and keywords in order to find research topics and explore new research trends in recent years.
Results
Analysis of global publications
A total of 3,568 documents published from 2013 to 2022 were retrieved. All the included literature were in English, including 3,206 articles and 362 reviews. The retrieval flow chart is displayed in Figure 1. The total Nc was 50,331, the average Nc per paper was 18.24, and the H-index of all publications was 94.
Annual trend of publication quantity
The annual Np was correlated with the year of publication, and the correlation coefficient R2 reached 0.8883 (Figure 2). Np increased from 263 in 2013 to 453 in 2021, with Np reaching its peak in 2021. Annual Np generally maintained an upward trend. A polynomial fitting curve showing the annual trend of publication is displayed in Figure 2.
Contributions of countries/regions
A total of 82 countries and regions had published related articles. The geographical distribution of the total number of articles included in the study across various countries and regions is shown in Figure 3A. Three quarters of the 3,568 articles were from the top 10 countries. From 2013 to 2022, the top 10 countries in terms of annual publications of this research were the United States, the United Kingdom, Canada, the Netherlands, China, Italy, Australia, France, Germany, and Switzerland (Figure 3B), among which the United States published the most papers, followed by the United Kingdom and Canada.
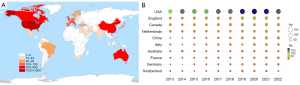
According to the top 10 high-yield countries/regions in Np (Table 1), the United States ranked first with the most articles published (1,443/40.44%). The United Kingdom (444/12.44%) ranked second, and Canada (404/11.32%) ranked third. Articles published in the United States were cited 28,078 times, accounting for 55.79% of the total citations, followed by the United Kingdom (10,017 times), and Canada (8,738 times). In addition, the H-index of the United States is the highest [78], which was more than 3 times that of China [23]. Germany and Switzerland had slightly lower Np, but their H-indexes, Nc, and average citations were higher than those of China.
Table 1
Rank | Country | Np | Nc | H-index | AC |
---|---|---|---|---|---|
1 | USA | 1,443 | 28,078 | 78 | 22.26 |
2 | England | 444 | 10,017 | 54 | 25.15 |
3 | Canada | 404 | 8,738 | 47 | 23.08 |
4 | Netherlands | 284 | 6,434 | 42 | 24.45 |
5 | China | 268 | 2,455 | 23 | 9.65 |
6 | Italy | 222 | 3,328 | 29 | 15.35 |
7 | Australia | 206 | 4,871 | 37 | 25.33 |
8 | France | 194 | 3,703 | 32 | 19.77 |
9 | Germany | 175 | 3,333 | 30 | 19.8 |
10 | Switzerland | 165 | 4,139 | 34 | 26.12 |
Np, total number of publications; Nc, total number of citations; AC, average citations per item.
Analysis of affiliations
The top 10 institutions with the highest Np related to this study are listed in Table 2. The University of London ranked first in terms of Np, followed by Harvard University and the University of Toronto. The Nc [6,909] and H-index [46] of the University of London were also the highest. The top 10 institutions are mostly from the United States and Canada.
Table 2
Rank | Affiliations | Country | Np | Nc | H-index |
---|---|---|---|---|---|
1 | University of London | England | 273 | 6,909 | 46 |
2 | Harvard University | USA | 255 | 6,500 | 43 |
3 | University of Toronto | Canada | 202 | 4,613 | 37 |
4 | Utrecht University | Netherlands | 195 | 4,640 | 36 |
5 | University Toronto Affiliates | Canada | 192 | 4,475 | 37 |
6 | Utrecht University Medical Center | Netherlands | 188 | 4,631 | 36 |
7 | University of California System | USA | 182 | 5,323 | 39 |
8 | King's College London | England | 180 | 4,572 | 39 |
9 | Hospital for Sick Children (SickKids) | Canada | 176 | 3,953 | 35 |
10 | Harvard Medical School | USA | 165 | 3,877 | 35 |
Np, total number of publications; Nc, total number of citations.
Analysis of authors
The top 10 authors with the largest Np in this study are shown in Table 3. The top 10 authors with the most papers had published 775 papers between them, accounting for 21.72% of the total papers. Benders and De Vries from the Netherlands ranked first and second in this research field, respectively, and they were followed by Inder of Harvard University in the US and Edwards of King’s College London in the UK. De Vries had the highest Nc [3,134]. Manon had the highest H-index [34]. This indicates that their research has attracted the attention of more scholars. The top 10 authors are mostly from the Netherlands and the United Kingdom.
Table 3
Rank | Author | Affiliations | Country | Np | Nc | H-index |
---|---|---|---|---|---|---|
1 | Benders, Manon | Utrecht University | Netherlands | 112 | 3,089 | 34 |
2 | De Vries, Linda S | Utrecht University | Netherlands | 103 | 3,134 | 33 |
3 | Inder, Terrie E | Harvard University | USA | 82 | 1,805 | 26 |
4 | Edwards, Anthony C | King’s College London | England | 82 | 2,892 | 32 |
5 | Miller, Steven P | University of Toronto | Canada | 75 | 2,693 | 29 |
6 | Groenendaal, Floris | Utrecht University | Netherlands | 75 | 2,267 | 31 |
7 | Hajnal, Joseph V | King’s College London | England | 69 | 2,038 | 26 |
8 | Counsell, Serena | King’s College London | England | 66 | 1,835 | 24 |
9 | Doyle, Lex | University of Melbourne | Australia | 57 | 2,043 | 26 |
10 | Cheong, Jeanie L. Y. | University of Melbourne | Australia | 54 | 1,028 | 20 |
Np, total number of publications; Nc, total number of citations
Analysis of journals
Top 10 journals with the most published papers are listed in Table 4. NeuroImage published the most related articles (162, IF: 7.4), followed by Pediatric Research (133, IF: 3.953) and NeuroImage: Clinical (98, IF: 4.891). About 25.42% were retrieved from 10 journals (907/25.42%). All of these 10 journals had a relatively high IF (defined as >3.000), and NeuroImage (IF: 7.4) had the highest Nc and H-index.
Table 4
Rank | Journal | Np | Nc | H-index | IF 2021 |
---|---|---|---|---|---|
1 | NeuroImage | 162 | 4,785 | 41 | 7.4 |
2 | Pediatric Research | 133 | 1,951 | 26 | 3.953 |
3 | NeuroImage Clinical | 98 | 1,690 | 24 | 4.891 |
4 | PLoS One | 94 | 1,950 | 26 | 3.752 |
5 | Journal of Pediatrics | 89 | 2,779 | 32 | 6.314 |
6 | Cerebral Cortex | 81 | 2,821 | 33 | 4.861 |
7 | Pediatric Radiology | 68 | 695 | 14 | 3.005 |
8 | Scientific Reports | 63 | 915 | 16 | 4.997 |
9 | American Journal of Neuroradiology | 60 | 863 | 17 | 4.966 |
10 | Pediatric Neurology | 59 | 767 | 17 | 4.21 |
Np, total number of publications; Nc, total number of citations; IF, impact factor.
Analysis of article GCS
The annual GCS number of the top 10 publications is displayed in Figure 4. The article published by Moeskopsin in IEEE Transactions on Medical Imaging in 2016 ranked first, and the total GCS from 2016 to 2022 was 524. In this paper, an automatic segmentation method based on convolutional neural networks (CNN) for anatomical MR brain images is proposed, which can obtain both accurate segmentation details and spatial consistency. This method is applied to the segmentation of neonatal images at different ages (14). This study focuses on the early development of white matter, and reviews imaging studies of fetuses, neonates, and infants. Based on a variety of complementary imaging methods, it is possible to explore brain development at multiple levels, from brain structure development to infant movement and cognition (15). Congenital Zika virus syndrome is a new type of teratogenic disease. In recent years, the research on congenital Zika virus syndrome has been developing rapidly. A study showed that head sizes varied according to the intensity of Zika exposure in the birth cohort, with most suspected cases producing neonates with small heads (16). A large number of studies have shown that magnetic resonance spectroscopy (MRS) has become a clinical neuroimaging method, especially for the clinical evaluation of central nervous system diseases, such as brain tumors, neonatal and childhood diseases (hypoxic-ischemic injury, inherited metabolic diseases, and traumatic brain injury), demyelinating diseases, and infectious brain injury, for which its clinical applications have been proven (17). Research has reviewed the use of behavioral and neuroimaging methods to assess the interaction between language and brain and cognitive processes. Babies born prematurely have a higher risk of cognitive and motor impairments than full-term babies. Early intervention in premature infants has a positive effect on cognitive and motor outcomes in infancy, with cognitive benefits continuing into preschool age (18).
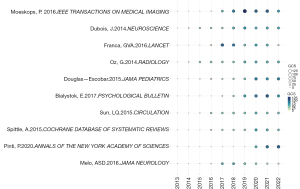
Co-cited reference analysis
In bibliometrics, the research frontier represents the development status of a research field, and the citations of the research frontier articles constitute the discipline knowledge base of the research field. A total of 88,007 documents were cited, and the minimum Nc for each was 49. We selected 200 articles for co-citation analysis. The co-cited literature was divided into different clusters by using different color nodes, as shown in Figure 5A. Figure 5A is a cluster diagram; the cluster was determined by VOSviewer software, which automatically classifies documents with similar research contents into 1 category according to the research content involved in the literature. Cluster 1, red, has 59 articles, mainly focusing on MRI studies on infants, premature delivery, neurodevelopmental imaging, cerebral cortex, and brain structural networks. Cluster 2, green, has 58 articles, mainly focusing on neonatal brain development, severe brain injury, and impaired brain growth patterns. Cluster 3, blue, has 39 articles, mainly focusing on brain development diffusion tensor imaging (DTI), myelination, and white matter tract studies. Cluster 4, yellow, has 33 articles, mainly focusing on the study of neurobehavioral abnormalities and MRI brain injury in hypothermia treatment of neonatal encephalopathy. Cluster 5, purple, has 11 articles, mainly focusing on premature infants and congenital heart disease (CHD) associated with the risk of brain damage and adverse neurodevelopmental outcomes, respectively. Figure 5B is a time diagram, illustrating the most typical references in terms of highlighted length, intensity, and time. As shown in Figure 5B, the 8 groups of documents that have been cited are “#0: diffusion imaging”, “#1: magnetic resonance imaging”, “#2: diffusion MRI”, “#3: functional connectivity”, “#4: segmentation”, “#5: periventricular leukomalacia”, “#6: congenital heart disease”, and “#7: hypothermia”. Figure 5C depicts the top 20 documents with the strongest citation prominence. Among them, Volpe et al. had the highest study intensity (40.01), mainly studying brain injury of premature infants with severe neurodevelopmental disorders (19). In recent years, Makropoulos et al., with a high intensity of research (26.11), focused on the use of structural MRI in the developing neonatal brain (20).
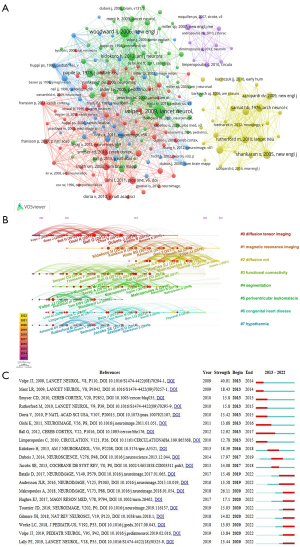
Research hotspots analysis
The keywords in the literature are highly condensed and summarize the content and theme of the literature. Therefore, through the research and analysis of hot keywords, we can better understand the relevant directions and hotspots of research in this field. Keywords in 3,568 papers and abstracts were analyzed using CiteSpace and VOSviewer respectively. As shown in Figure 6A, during the period from 2013 to 2022, 10,728 keywords were used, among which 196 keywords appeared more than 31 times. A total of 5 different color clusters were obtained according to the size, color, and line between the tags. Cluster 1, red, has 73 keywords, mainly focusing on brain structure, brain connectivity, brain development, and cognitive-related studies. Cluster 2, green, has 40 keywords, mainly focusing on the diagnosis, mechanism, and imaging manifestations of the disease. Cluster 3, blue, has 38 keywords, mainly focusing on the disease biomarker- and MRI-related studies of neonatal encephalopathy. Cluster 4, yellow, has 33 keywords, mainly focusing on neonatal cranial nerve injury, clinical manifestations, and influencing factors at birth of premature infants. Cluster 5, purple, has 12 keywords, mainly focusing on cerebral nerve development in neonates with CHD. The size of tags in the co-occurrence knowledge map represents the frequency of keyword occurrence, whereby the larger the tag, the higher frequency of keyword occurrence; the lines between the tags represent the connection between the 2, whereby the closer the lines, the closer the connection, and the same color is clustered into the same category of the study.
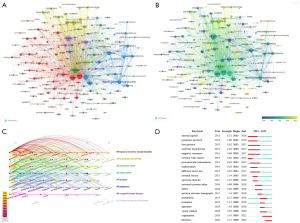
As shown in Figure 6B, VOSviewer classified the colors of all keywords according to their average publication year (APY) and divided the colors of all keywords based on APY. The latest keyword is “neurodevelopmental” (Cluster 2, APY: 2,018.28), followed by “neuroimaging” (Cluster 2, APY: 2,018.47), and “brain development” (Cluster 1, APY: 2,017.74), all of which are associated with neurodevelopmental images of neonates. Further, “abnormalities” (Cluster 1, APY: 2,017.70) and “diffusion tensor imaging” (Cluster 1, APY: 2,017.33) represented hot points of concern in recent years.
Keyword timeline view was used to gather statistics of keyword evolution with the year, and to construct a knowledge map. Through the analysis of the evolution of keywords with the change of years, we were able to understand the evolution of keywords hotspot and phase characteristics; through the comparison of the change of keywords in different time periods, we were able to better elucidate the current research frontier in this field. Figure 6C displays the clusters of keywords and identification of hotspots using CiteSpace. A total of 8 research hotspots were formed: “#0: hypoxic-ischemic encephalopathy”, “#1: functional connectivity”, “#2: premature infant”, “#3: white” matter, “#4: epilepsy”, “#5: pregnancy”, “#6: congenital heart disease”, and “#7: contrast-enhanced ultrasound”.
Keyword emergence refers to the frequency surge of keywords in a certain period of time. Keywords with high frequency change rate can be detected at corresponding time nodes, and the emerging research frontier keywords can be extracted and the keyword prominence year distribution map can be generated to analyze the research hotspots and frontiers in the current research field. In Figure 6D, the top 20 emergent keywords from 2013 to 2022 are analyzed, in which the emergent keywords of “organization”, “thickness”, “young children”, and “prematurity” are with relatively high mutation intensity, which represent the research trends in recent years.
Discussion
Based on WoSCC database, this study conducted bibliometric analysis using VOSviewer and CiteSpace to discuss the hotspots and development trends of neonatal MRI brain neuroimaging research, and retrieved 3,568 original articles and reviews published from 2013 to 2022. Overall, the number of published papers is gradually increasing. According to the polynomial fitting curve, the largest Np was in 2021, which indicates that with the passage of time, the development of this research has been on the rise, and the heat and depth of academic research have been increasing year by year.
From 2013 to 2022, among the top 10 countries in terms of annual publications of this study, the United States published the most articles and ranked first, the United Kingdom ranked second, and Canada ranked third. The United States had more than 3 times as many publications as the United Kingdom or Canada. Articles published in the United States were cited 28,078 times, accounting for 55.79% of the total citations, followed by the United Kingdom and Canada. In addition, the US had the highest H-index [78], more than three times that of China [23]. The institution with the largest Np associated with this study was the University of London, which ranked first in Np, followed by Harvard University and the University of Toronto. The University of London also had the highest Nc and H-index, and most of the top 10 institutions were from the US and Canada. This shows that the United States and the United Kingdom have higher attention in this field, published most of the research in this field, and have good academic ability in this field. The journal that published the most related articles was NeuroImage, followed by Pediatric Research and NeuroImage: Clinical. About 25.42% of the documents were retrieved from 10 journals, with a high IF. This indicates that these journals have published more research breakthroughs in this field, which also reminds researchers interested in this field to pay more attention to these journals. The article published by Moeskops in IEEE Transactions on Medical Imaging in 2016 ranked first, and the total GCS was 524. The proposed automatic segmentation method for anatomical MR brain images based on multi-scale CNN can obtain both accurate segmentation details and spatial consistency. This method has been applied to the segmentation of neonatal images, young and old images at different ages, indicating that the automatic segmentation of brain tissue is the focus of most scholars (14). Further, imaging studies on early white matter development in fetuses, neonates, and infants are reviewed, based on a variety of complementary imaging methods that are able to explore brain development at multiple levels, from brain structural development to infant movement and cognition (15). In addition to normal development, these studies are critical to understanding the pathological mechanisms resulting from early brain abnormalities (e.g., genetic diseases, epilepsy, intellectual disability, learning disabilities) and to evaluating early interventions related to fetal conditions (e.g., growth restriction in utero, teratogenic exposure) or perinatal factors (e.g., premature birth, neonatal stroke). Behavioral and neuroimaging methods can be used to assess the interaction between language and brain and cognitive processes (18).
Co-cited reference analysis emphasizes research closely related to specific fields. The co-cited literature co-display network map in Figure 5A shows that Cluster 1 mainly focuses on MRI research on infant, premature birth, developmental neuroimaging, cerebral cortex, and brain structural network, followed by MRI prediction on neural development, selection of new neonatal tissue segmentation model, and the modeling that supports new model of brain connectivity. For example, the disruption of network structure after hypoxic-ischemic injury in full-term infants, although less studied, may also directly affect the results. However, a direct link between changes in structural and functional connectivity patterns and task performance has not been proven in these populations. Future research must continue to focus on determining whether this relationship exists and determining its applicability in predicting outcomes in brain-damaged infants and other high-risk neonatal populations. Cluster 2 focuses primarily on neonatal brain development, studying severe brain injury and impaired brain growth patterns independently associated with perinatal risk factors and cognitive disability. Cluster 3 mainly focuses on DTI of brain development, myelination, and white matter tracts. Brain development depends on complex and intertwined pathological mechanisms, and the relationship between brain maturation and behavioral changes is unclear and requires non-invasive in vivo imaging studies such as MRI. It is important to understand early pathological mechanisms and to assess the influence of fetal or perinatal factors on later life. Brain development depends on complex and intertwined pathological mechanisms, and the relationship between brain maturation and behavioral changes is not well understood, requiring non-invasive in vivo imaging studies such as MRI to understand early pathological mechanisms and assess the impact of fetal or perinatal factors on later life. Cluster 4 mainly discusses the effects of hypothermia therapy on mortality, long-term neurodevelopmental disorders and clinically important side effects of neonatal encephalopathy, and neurobehavioral abnormalities and MRI brain injury in neonatal encephalopathy treated with hypothermia. Neonatal animal studies and preliminary human studies have shown that mild hypothermia following perinatal hypoxic-ischemia in newborns can reduce neurological sequelae without adverse effects. Cluster 5 focuses on the risk of premature birth and CHD associated with brain damage and adverse neurodevelopmental outcomes, respectively. Cluster 1, cluster 2, and cluster 3 are closely related and connected, whereas cluster 4 and cluster 5 are not very close. Based on atlas analysis, we found that most studies focused on neonatal brain development imaging and brain structural network connectivity studies. Sustained brain damage during the neonatal period may disrupt the development of key structural and functional connectivity networks, leading to subsequent neurodevelopmental disorders in affected newborns. These networks can be characterized by both structural [diffusion MRI (dMRI)] and functional [resting-state functional MRI (rs-fMRI)] neuroimaging techniques. Studies using diffuse MRI, rs-fMRI, and other complementary neuroimaging modalities to characterize structural and functional connectivity development in infants with brain injury. Figure 5B shows the time axis distribution of cluster analysis. “#1: magnetic resonance imaging” and “#2: diffusion MRI” are still the current research trends. The dMRI technique is the key to the study of early brain microstructure development abnormalities; it calculates information about cell integrity and tissue microstructure by applying gradient pulses of different parameters to measure micrometer-scale differences in the diffusion of water molecules. Therefore, we can carry out more exploration in this field of research in the future. Figure 5C cites the top 20 most prominent papers, in which Volpe et al. mainly study brain injury in premature infants with severe neurodevelopmental disorders, including severe cognitive deficits and motor impairments. This type of brain injury is generally thought to consist mainly of periventricular leukomalacia (PVL), which is often accompanied by neuron/axon disease. It affects the white matter of the brain, thalamus, basal ganglia, cerebral cortex, brainstem, and cerebellum. This review incorporates new insights into brain damage and brain development in human prematurity (19). Makropoulos et al. mainly studied structural MRI for developing neonatal brains. In recent years, various segmentation methods have been used to automatically delineate neonatal brain MRI. Different methods to evaluate segmentation accuracy and benchmarks have been used to improve the quality of segmentation, supporting the modeling of new model of brain connectivity (20).
Keywords are the extraction of literature research content and represent the theme of the article. When the frequency of a keyword is high, it reveals the distribution of the research as well as the hotspot and trend of the research. In addition, keyword analysis can determine when frequency-changing keywords first appear in a node. As shown in Figure 6A, the research fields mainly focus on neuroimaging research related to brain structure, brain connectivity, brain development, and cognition (21-24). As shown in Figure 6B, the latest keyword is “neurodevelopmental”, followed by “neuroimaging” and “brain development”, all of which are related to neurodevelopmental images of neonates. The maturation of brain microstructure is a long and complex process, generally lasting from the fetal period to adolescence. The cerebral cortex is composed of various neurons, glia, and a large number of nerve fibers entering and leaving the cortex, and the cerebral white matter is mainly composed of nerve fibers. Nerve fibers transmit and encode information by connecting neurons in different brain regions, and their integrity reflects the development and maturity of cerebral cortex. MRI not only indirectly reflects the complex dynamic processes observed at the molecular and cellular levels, but also provides information on brain morphology, structural connectivity, microstructure properties of gray and white matter, and functional structure of the brain. In addition, “abnormalities” and “diffusion tensor imaging” have been the focus of recent years, attracting the attention of interested scholars. Diffusion imaging applied to neonates provides information on tissue physiology (damage) and microstructure. Diffusion parameters vary during early brain development, reflecting underlying changes in white and gray matter microstructure. DTI can identify the movement of water molecules within tissues, reconstruct white matter fiber bundles, and provide high-resolution microstructures of brain tissue. The basic parameters of DTI, such as fractional anisotropy (FA) and mean diffusivity (MD), can reflect many important information of brain tissue, such as neuron density, fiber orientation diffusion, degree of myelination, free water content, and axon diameter. Early diffusion imaging can accurately indicate the extent and location of brain injury in full-term neonates with neonatal encephalopathy. In premature infants, abnormalities in white matter diffusion anisotropy reflect the extensive disruption of white matter development that accompanies premature birth (25-30). As shown in Figure 6C, “hypoxic-ischemic encephalopathy”, “functional connectivity”, “white matter”, and “pregnancy” have also attracted scholars’ attention in the past 3 years. Although brain MRI has been considered the gold standard for detecting brain injury in preterm infants in recent years, neuroultrasound has clear advantages such as cost-effectiveness, diagnostic practicality, and convenience. Contrast-enhanced ultrasound (CEUS) is a valuable bedside imaging technique for qualitative and quantitative assessment of cerebral perfusion. The risk of hypoxic-ischemic injury, intraventricular hemorrhage, PVL, and hemorrhagic hydrocephalus is significantly higher in preterm infants, which is an important tool for diagnosis and monitoring of brain injury in preterm infants. Therefore, studies have used MRI as the reference standard to evaluate the feasibility, safety, and diagnostic value of transfontanel cerebral CEUS in newborns (31,32). As shown in Figure 6D, the top 20 keywords that appear most frequently have new research directions in different stages with the deepening of the research, and their influence and research activity deserve attention. From 2019 to 2022, the keywords were “organization” and “thickness”, which mainly focused on the study of brain tissue and cerebral cortex (33,34). These keywords represent a new research hotspot and trend in this field. Quantitative measurement of brain volume using structural MRI is helpful to evaluate the brain growth trajectory of children. The sulci, fold, surface area, and volume of the outer surface of the cerebral cortex will undergo complex changes during development, which can be used as markers of brain maturity. In the future, the field of neonatal neuroimaging will still be a hot topic for researchers to focus on. However, as the research focus of a field is constantly changing, researchers still need to explore new research fields and innovate to open up new paths and technical methods.
Multimodal MRI technology has played an important role in the study of neonatal neurodevelopment. Structural MRI, dMRI, MRS, fMRI, and so on, can provide structural, functional, and metabolic information for the evaluation of neonatal brain injury and brain development, and further explore its potential neuropathological mechanism. Although this study did not systematically analyze each category, it can be seen from the information extracted from the software cluster classification that each subtype is also interrelated. Based on a variety of complementary imaging methods, brain development can be explored at multiple levels, from brain structure development to infant motor and cognition. In addition to normal development, it is vital to understand the pathological mechanisms that result from early brain abnormalities such as genetic disorders, epilepsy, intellectual disability, and learning disabilities. In addition, considering the influence of neonatal growth and development factors, longitudinal MRI studies using multiple time points are more likely to elucidate the relationship between brain development and future neurocognitive outcomes. With the rapid development of artificial intelligence technology, the field of neonates has ushered in the era of artificial intelligence. Artificial intelligence analyzes and learns data through logical thinking similar to that of the human brain, and constantly produces new algorithms and new models, which continuously improves its performance. At present, deep learning algorithms, especially convolutional networks, have achieved great success in the application of medical image analysis. There are studies that can predict the segmentation of infant brain tissue and neurodevelopmental disorders at the stage of equal intensity. For example, some studies have treated the brain structure connectome as a 2-dimensional image and applied the established deep CNN to learn the spatial and topological information of the brain connectome. In addition, using transfer learning techniques to mitigate the lack of training data, a transfer learning-enhanced CNN (TL-CNN) model was developed for early prediction of cognitive assessment of extremely preterm infants at age 2 using brain structural connectomes. With the continuous application of new algorithms based on large databases and machine learning, and the increasing cross-collaboration between doctors and professionals, MRI neuroimaging plays an important role in the assessment, detection, and characterization of a wide range of neonatal diseases (34-37). In addition, with the application of artificial intelligence in the field of neonates, the clinical data and image data of neonates will be more accurate and efficient, which is of great significance for the improvement of clinical precision treatment.
Limitations
Through bibliometric and visualization analysis of publications, we can to some extent understand the research hotspots and development trends in this field. However, there are some limitations to this study. First, only articles and reviews in English were included in the SCIE database that we retrieved. In addition, the different selection of search terms will also affect the search results. Second, our study excluded the most recent articles published in 2023. Therefore, there may be a delay in our research to some extent.
Conclusions
In this study, bibliometrics was used to analyze the literature on neonatal MRI brain neuroimaging in the past 10 years. At present, the research development of this field is on the rise. Especially, the United States and the United Kingdom have paid much attention to this field and published most of the research in this field. Neonatal neurodevelopment, brain structural network, cerebral cortex, DTI, and deep learning are currently hotspots of research. Our study can help researchers to better understand the research status, hotspots, and frontier development trends in this field from a macro perspective, and provide certain references for topic selection and research development direction in the future.
Acknowledgments
Funding: This study received funding by
Footnote
Conflicts of Interest: All authors have completed the ICMJE uniform disclosure form (available at https://qims.amegroups.com/article/view/10.21037/qims-23-880/coif). The authors have no conflicts of interest to declare.
Ethical Statement: The authors are accountable for all aspects of the work in ensuring that questions related to the accuracy or integrity of any part of the work are appropriately investigated and resolved.
Open Access Statement: This is an Open Access article distributed in accordance with the Creative Commons Attribution-NonCommercial-NoDerivs 4.0 International License (CC BY-NC-ND 4.0), which permits the non-commercial replication and distribution of the article with the strict proviso that no changes or edits are made and the original work is properly cited (including links to both the formal publication through the relevant DOI and the license). See: https://creativecommons.org/licenses/by-nc-nd/4.0/.
References
- Dubois J, Alison M, Counsell SJ, Hertz-Pannier L, Hüppi PS, Benders MJNL. MRI of the Neonatal Brain: A Review of Methodological Challenges and Neuroscientific Advances. J Magn Reson Imaging 2021;53:1318-43. [Crossref] [PubMed]
- Miller JH, Bardo DME, Cornejo P. Neonatal Neuroimaging. Semin Pediatr Neurol 2020;33:100796. [Crossref] [PubMed]
- Wisnowski JL, Wintermark P, Bonifacio SL, Smyser CD, Barkovich AJ, Edwards AD, de Vries LS, Inder TE, Chau V. Neuroimaging in the term newborn with neonatal encephalopathy. Semin Fetal Neonatal Med 2021;26:101304. [Crossref] [PubMed]
- Barkovich AJ. Imaging of the Newborn Brain. Semin Pediatr Neurol 2019;32:100766. [Crossref] [PubMed]
- Brenner RG, Wheelock MD, Neil JJ, Smyser CD. Structural and functional connectivity in premature neonates. Semin Perinatol 2021;45:151473. [Crossref] [PubMed]
- Zhang J, Zhang Y, Hu L, Huang X, Liu Y, Li J, Hu Q, Xu J, Yu H. Global Trends and Performances of Magnetic Resonance Imaging Studies on Acupuncture: A Bibliometric Analysis. Front Neurosci 2020;14:620555. [Crossref] [PubMed]
- Xia MH, Li A, Gao RX, Li XL, Zhang Q, Tong X, Zhao WW, Cao DN, Wei ZY, Yue J. Research hotspots and trends of multimodality MRI on vascular cognitive impairment in recent 12 years: A bibliometric analysis. Medicine (Baltimore) 2022;101:e30172. [Crossref] [PubMed]
- Karagianni MD, Brotis AG, Gatos C, Kalamatianos T, Vrettou C, Stranjalis G, Fountas KN. Neuromonitoring in Severe Traumatic Brain Injury: A Bibliometric Analysis. Neurocrit Care 2022;36:1044-52. [Crossref] [PubMed]
- Deora H, Ferini G, Garg K, Narayanan MDK, Umana GE. Evaluating the Impact of Intraoperative MRI in Neuro-Oncology by Scientometric Analysis. Life (Basel) 2022.
- Huang L, Xu G, He J, Tian H, Zhou Z, Huang F, Liu Y, Sun M, Liang F. Bibliometric Analysis of Functional Magnetic Resonance Imaging Studies on Acupuncture Analgesia Over the Past 20 Years. J Pain Res 2021;14:3773-89. [Crossref] [PubMed]
- Yan S, Zhang H, Wang J. Trends and hot topics in radiology, nuclear medicine and medical imaging from 2011-2021: a bibliometric analysis of highly cited papers. Jpn J Radiol 2022;40:847-56. [Crossref] [PubMed]
- van Eck NJ, Waltman L. Software survey: VOSviewer, a computer program for bibliometric mapping. Scientometrics 2010;84:523-38. [Crossref] [PubMed]
- Chen C. CiteSpace II: Detecting and visualizing emerging trends and transient patterns in scientific literature. Journal of the American Society for Information Science and Technology 2006;57:359-377.
- Moeskops P, Viergever MA, Mendrik AM, de Vries LS, Benders MJ, Isgum I. Automatic Segmentation of MR Brain Images With a Convolutional Neural Network. IEEE Trans Med Imaging 2016;35:1252-61. [Crossref] [PubMed]
- Dubois J, Dehaene-Lambertz G, Kulikova S, Poupon C, Hüppi PS, Hertz-Pannier L. The early development of brain white matter: a review of imaging studies in fetuses, newborns and infants. Neuroscience 2014;276:48-71. [Crossref] [PubMed]
- França GV, Schuler-Faccini L, Oliveira WK, Henriques CM, Carmo EH, Pedi VD, Nunes ML, Castro MC, Serruya S, Silveira MF, Barros FC, Victora CG. Congenital Zika virus syndrome in Brazil: a case series of the first 1501 livebirths with complete investigation. Lancet 2016;388:891-7. [Crossref] [PubMed]
- Oz G, Alger JR, Barker PB, Bartha R, Bizzi A, Boesch C, et al. Clinical proton MR spectroscopy in central nervous system disorders. Radiology 2014;270:658-79. [Crossref] [PubMed]
- Bialystok E. The bilingual adaptation: How minds accommodate experience. Psychol Bull 2017;143:233-62. [Crossref] [PubMed]
- Volpe JJ. Dysmaturation of Premature Brain: Importance, Cellular Mechanisms, and Potential Interventions. Pediatr Neurol 2019;95:42-66. [Crossref] [PubMed]
- Makropoulos A, Robinson EC, Schuh A, Wright R, Fitzgibbon S, Bozek J, et al. The developing human connectome project: A minimal processing pipeline for neonatal cortical surface reconstruction. Neuroimage 2018;173:88-112. [Crossref] [PubMed]
- Wang Q, Xu Y, Zhao T, Xu Z, He Y, Liao X. Individual Uniqueness in the Neonatal Functional Connectome. Cereb Cortex 2021;31:3701-12. [Crossref] [PubMed]
- Chen M, Li H, Wang J, Yuan W, Altaye M, Parikh NA, He L. Early Prediction of Cognitive Deficit in Very Preterm Infants Using Brain Structural Connectome With Transfer Learning Enhanced Deep Convolutional Neural Networks. Front Neurosci 2020;14:858. [Crossref] [PubMed]
- Ball G, Aljabar P, Arichi T, Tusor N, Cox D, Merchant N, Nongena P, Hajnal JV, Edwards AD, Counsell SJ. Machine-learning to characterise neonatal functional connectivity in the preterm brain. Neuroimage 2016;124:267-75. [Crossref] [PubMed]
- Baker S, Kandasamy Y. Machine learning for understanding and predicting neurodevelopmental outcomes in premature infants: a systematic review. Pediatr Res 2023;93:293-9. [Crossref] [PubMed]
- Triplett RL, Smyser CD. Neuroimaging of structural and functional connectivity in preterm infants with intraventricular hemorrhage. Semin Perinatol 2022;46:151593. [Crossref] [PubMed]
- Neil JJ, Smyser CD. Diffusion Magnetic Resonance Imaging of Infants. Magn Reson Imaging Clin N Am 2021;29:185-93. [Crossref] [PubMed]
- Banihani R, Seesahai J, Asztalos E, Terrien Church P. Neuroimaging at Term Equivalent Age: Is There Value for the Preterm Infant? A Narrative Summary. Children (Basel) 2021.
- Pecheva D, Kelly C, Kimpton J, Bonthrone A, Batalle D, Zhang H, Counsell SJ. Recent advances in diffusion neuroimaging: applications in the developing preterm brain. F1000Res 2018;7:eF1000 Faculty Rev-1326.
- Vo Van P, Alison M, Morel B, Beck J, Bednarek N, Hertz-Pannier L, Loron G. Advanced Brain Imaging in Preterm Infants: A Narrative Review of Microstructural and Connectomic Disruption. Children (Basel) 2022.
- Smyser CD, Wheelock MD, Limbrick DD Jr, Neil JJ. Neonatal brain injury and aberrant connectivity. Neuroimage 2019;185:609-23. [Crossref] [PubMed]
- Hwang M. Introduction to contrast-enhanced ultrasound of the brain in neonates and infants: current understanding and future potential. Pediatr Radiol 2019;49:254-62. [Crossref] [PubMed]
- Squires JH, Beluk NH, Lee VK, Yanowitz TD, Gumus S, Subramanian S, Panigrahy A. Feasibility and Safety of Contrast-Enhanced Ultrasound of the Neonatal Brain: A Prospective Study Using MRI as the Reference Standard. AJR Am J Roentgenol 2022;218:152-61. [Crossref] [PubMed]
- Makropoulos A, Counsell SJ, Rueckert D. A review on automatic fetal and neonatal brain MRI segmentation. Neuroimage 2018;170:231-48. [Crossref] [PubMed]
- Romberg J, Wilke M, Allgaier C, Nägele T, Engel C, Poets CF, Franz A. MRI-based brain volumes of preterm infants at term: a systematic review and meta-analysis. Arch Dis Child Fetal Neonatal Ed 2022;107:520-6. [Crossref] [PubMed]
- Neumane S, Gondova A, Leprince Y, Hertz-Pannier L, Arichi T, Dubois J. Early structural connectivity within the sensorimotor network: Deviations related to prematurity and association to neurodevelopmental outcome. Front Neurosci 2022;16:932386. [Crossref] [PubMed]
- He L, Li H, Chen M, Wang J, Altaye M, Dillman JR, Parikh NA. Deep Multimodal Learning From MRI and Clinical Data for Early Prediction of Neurodevelopmental Deficits in Very Preterm Infants. Front Neurosci 2021;15:753033. [Crossref] [PubMed]
- van Boven MR, Henke CE, Leemhuis AG, Hoogendoorn M, van Kaam AH, Königs M, Oosterlaan J. Machine Learning Prediction Models for Neurodevelopmental Outcome After Preterm Birth: A Scoping Review and New Machine Learning Evaluation Framework. Pediatrics 2022;150:e2021056052. [Crossref] [PubMed]