Application of ultrasound-based radiomics models of breast masses to predict invasive components of encapsulated papillary carcinoma
Introduction
Encapsulated papillary carcinoma (EPC) accounts for less than 2% of all breast cancers with a generally good prognosis (1). EPC is often accompanied by an in situ or invasive component (INV) (2). EPC has an axillary lymph node (ALN) metastasis rate of 1.1–18.8% (3,4). Clinical management of EPC may be altered depending on the presence of an INV (5-7). Core needle biopsy is an accurate and commonly used modality to diagnose preoperative pathology of breast cancer. However, it has been reported that the accuracy of core needle biopsy for EPC is low (33.3%), and even the accompanying INV failed to be diagnosed in 60% of cases (3). The presence of an INV in EPC impacts treatment, however, preoperative biopsy is not always accurate.
Ultrasound (US) is an important screening modality for breast cancer. Previous studies (3,8,9) have shown that EPC often presents as a complex cystic and solid mass with circumscribed margin, oval to round shape, and abundant blood flow within the parenchymal component or papillary structures. US has a diagnostic accuracy of 62.0% for EPC (3). Hassan et al. (7) suggested that EPC with uncircumscribed margins predict postoperative pathology with invasion, but core needle biopsy failed to diagnose it. Speer et al. suggested that an irregular mass is more often associated with the diagnosis of invasive EPC (8). However, no US imaging features have been demonstrated to differentiate in situ versus invasive EPC (8,10). Therefore, studies on INVs of EPC are few and limited to descriptive studies, and there is still a gap in predictive studies.
Radiomics is a high-throughput process of extracting a large number of quantitative imaging features from digital medical images and converting them into high-dimensional, mineable data (11). A radiomics approach can surpass the limitations of US images that are limited to subjective US interpretation (11). US-based radiomics has been increasingly developed in the diagnosis, prediction, and prognosis of diseases. For example, Wang et al. (12) used deep learning radiomics of elastography for assessing liver fibrosis stages, with an area under the receiver operating characteristic (ROC) curve (AUC) of 0.85 and above. Yu et al. (13) successfully developed an US-based radiomics nomogram to predict ALN metastasis in early-stage invasive breast cancer, with an AUC of 0.81.
This study aimed to develop a radiomics model for predicting INVs of EPC based on US images. To the best of our knowledge, this is the first article related to the study of breast EPC using a radiomics approach.
Methods
Patients
A total of 111 patients (113 masses) were consecutively included in this retrospective study from January 2016 to April 2021. All masses were diagnosed as EPC by surgical pathology and underwent preoperative breast US examinations in Fudan University Shanghai Cancer Center. The inclusion criteria were as follows: (I) EPC, and (II) visible on US examinations. A total of 6 patients were excluded, 3 due to having undergone breast surgery in other hospitals, 2 because they only exhibited a small amount of EPC (focal area), and 1 because of invisibility on US examinations. Finally, 107 masses (105 patients) were enrolled for analysis. In a ratio of 3:2, 64 masses were randomly assigned to a training set (52 EPC without INV; 12 EPC with INV) and 43 masses were assigned to a test set (36 EPC without INV; 7 EPC with INV).
This retrospective study was conducted in accordance with the Declaration of Helsinki (as revised in 2013). The study was approved by the Ethics Committee of Fudan University Shanghai Cancer Center (No. 2107238-18), and the requirement for individual consent for this retrospective analysis was waived.
Clinicopathological features
Clinicopathological data were obtained from medical records including age, palpable ALN, ALN metastasis, the status of human epidermal growth factor receptor 2 (HER2), estrogen receptor (ER), progesterone receptor (PR), and histological type.
ER or PR positivity refers to immunohistochemistry (IHC) staining ≥1% (14). HER2 positivity is determined by any of the following tests: (I) immunohistochemistry 3+, or complete and intense member staining >30% of invasive cancer cells; (II) HER2/CEP17 ratio >2.2/2.0 measured by fluorescence in situ hybridization test; and (III) HER2 gene copy number >6.0 signal/nucleus by chromogenic in situ hybridization (15,16).
US image acquisition
US images were obtained using several machines, such as SuperSonic Aixplorer US scanner (Supersonic Imagine S.A., Aix en Provence, France) with a 7–15 MHz linear transducer, GE Logiq E9 (GE Healthcare, Waukesha, WI, USA) with a 6–15 MHz linear transducer, Toshiba Aplio 500 (Toshiba Medical Systems Corporation, Tochigi, Japan) with a 5–10 MHz linear transducer. US screening was conducted by several radiologists who have 3–20 years of respective experience.
US image processing and analysis
US features analysis
All US images underwent independent and blinded review by 2 radiologists and were evaluated based on the Breast Imaging-Reporting and Data System 5th edition (17). If the radiologists had inconsistent results, an agreement was reached by consensus. Vascularity was evaluated according to Adler’s index (0, I, II, or III) (18), and 0, I for less flow, II, III for abundant flow. Since 8 masses did not have color Doppler US images, only 99 masses were included.
Region of interest segmentation
Breast masses segmentation was manually delineated along the boundary of the entire lesion by a radiologist with 6-year experience using freely available software (LabelMe; https://github.com/airsimonhan/label-for-dicom-ima).
Feature extraction and selection
A total of 461 radiomics features were extracted from the region of interest (ROI) of each US image. The features include 16 self-made shape features, 16 self-made histogram features, 19 self-made texture features, 23 gray-level co-occurrence matrix (GLCM) features, 13 gray-level run-length matrix (GLRLM) features, 13 gray-level size zone matrix (GLSZM) features, 5 neighborhood gray-tone difference matrix (NGTDM) features, and 356 wavelet features. All radiomics features used mutual information for univariate features selection and 30 features were selected for building prediction models. The names of the selected features can be found in Table 1.
Table 1
Feature type | Feature name |
---|---|
Shape feature | Entropy of normalized radius histogram |
Diameter of equivalent circle | |
Grayscale feature | Histogram range |
Boundary-based texture features | Standard deviation of annular region |
Variance contrast of inside and outside | |
GLRLM | Low gray |
GLSZM | High gray-level zone emphasis |
NGTDM | Complexity |
Wavelet features | 22 features |
INV, invasive component; GLCM, gray-level co-occurrence matrix; GLRLM, gray-level run length matrix; GLSZM, gray-level size zone matrix; NGTDM, neighbor gray tone difference matrix.
Data sampling and building of radiomics models
If our imbalanced dataset is directly used to train the radiomics model, the model will predict all inputs into the majority class (EPC without INV) to maximize the accuracy of the prediction. The minority class (EPC with INV) is usually more important in clinical situations. Therefore, we need to improve the capability of the radiomics model to discriminate the minority class, and data resampling can solve the problem of classes imbalance.
SMOTEENN (19) was used to perform data sampling for the features in the training set. It is a method combing over-sampling and under-sampling using synthetic minority oversampling technique (SMOTE) and edited nearest neighbors (ENN). During the data sampling, firstly SMOTE increases the examples in the minority class by randomly interpolating between the examples. Then, if the class label of one example differs from the class of at least 2 of its 3 nearest neighbors, ENN will remove it from the dataset. In general, SMOTEENN reduces the gap between the numbers of examples in the 2 classes and increases the between-class distance. As a result, the classifier can more easily find the decision boundary between 2 classes, thus improving the prediction performance.
We used 5 ensemble machine learning classifiers to build radiomics models for predicting EPC with INV, including XGBoost, AdaBoost, Balanced Random Forest, Easy Ensemble, and Random Under Sampling (RUS) Boost. We established the new training set after data sampling using SMOTEENN. The classifiers were trained on the new training set and evaluated on both the original training set and the test set.
The radiomics flow chart is shown in Figure 1.
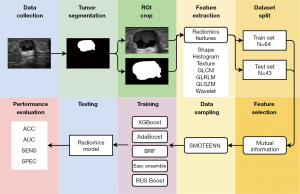
Statistical analysis
All data were analyzed using SPSS version 20 (IBM Corp., Armonk, NY, USA). To determine related clinical and US features with INVs of EPC, continuous variables were analyzed using the t-test or Mann-Whitney U test, whereas categorical variables were analyzed using the chi-square or Fisher’s exact test. This study estimated the performance of the radiomics models using accuracy (ACC), AUC, sensitivity (SENS), and specificity (SPEC). Statistical significance was considered when P<0.05.
Results
Clinical and pathological characteristics
Within a total of 105 patients, 5 were male and 100 were female. The mean age was 63.71 years (range, 31 to 85 years); the mean size of tumors was 23.40 mm (range, 9 to 120 mm). Of 107 masses, 88 presented EPC without INV, 19 EPC with INV. A total of 70 masses (65.4%) presented isolated EPC (Figure 2), 18 EPC with carcinoma in situ (17 ductal carcinomas in situ, 1 intraductal papillary carcinoma), 19 EPC with INV [14 invasive ductal carcinoma (Figure 3), 3 with synchronous invasive ductal carcinoma and ductal carcinoma in situ, 1 invasive carcinoma with neuroendocrine differentiation, 1 invasive cribriform carcinoma]. Some 65 masses (68.4%) presented luminal A, followed by luminal B (28 masses, 29.5%), and only 2 masses were triple-negative breast cancer. We were unable to subtype 12 masses due to HER2 score 2+ and without a fluorescence in situ hybridization test. Axillary ultrasonography showed no suspicious ALNs in all patients. Of the patients who underwent ALN management, 4 had ALN metastases.
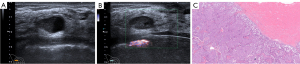

EPC patients with INV had a slightly younger mean age and were less likely to be postmenopausal than EPC patients without INV (61.32 vs. 64.23 years, 73.7% vs. 78.0%) (Table 2). EPC with INV had more luminal B subtype, whereas EPC without INV had slightly more luminal A subtype.
Table 2
Features | All cases (N=107) | EPC | Set | ||||||||
---|---|---|---|---|---|---|---|---|---|---|---|
Without INV (N=88) | With INV (N=19) | χ2/U/t | P value | Training set (N=64) | Test set (N=43) | χ2/U/t | P value | ||||
Age (years) | 63.71±11.98 | 64.23±11.62 | 61.32±13.59 | 0.961 | 0.339 | 53 [45, 61] | 53 [46, 61] | −0.237 | 0.813 | ||
Tumor size on US (mm) | 21 [16, 26] | 20.0 [16, 25] | 25 [18, 32] | −1.942 | 0.052 | 20 [15, 26] | 20.0 [15, 26] | −1.963 | 0.050 | ||
Menopausal status† | 0.167 | 0.683 | 0.297 | 0.586 | |||||||
Premenopausal | 23 (22.8) | 18 (22.0) | 5 (26.3) | 13 (21.0) | 10 (25.6) | ||||||
Postmenopausal | 78 (77.2) | 64 (78.0) | 14 (73.7) | 49 (79.0) | 29 (74.4) | ||||||
Family history of breast cancer | 0.585 | 0.444 | 0.410 | 0.522 | |||||||
No | 83 (77.6) | 67 (76.1) | 16 (84.2) | 51 (79.7) | 32 (74.4) | ||||||
Yes | 24 (22.4) | 21 (23.9) | 3 (15.8) | 13 (20.3) | 11 (25.6) | ||||||
Palpable ALN | 1.132 | 0.287 | 3.459 | 0.063 | |||||||
No | 102 (95.3) | 83 (94.3) | 19 (100.0) | 63 (98.4) | 39 (90.7) | ||||||
Yes | 5 (4.7) | 5 (5.7) | 0 (0.0) | 1 (1.6) | 4 (9.3) | ||||||
Breast tumor management | – | – | – | – | |||||||
Partial mastectomy/breast conserving surgery | 64 (59.8) | 57 (64.8) | 7 (36.8) | 36 (56.3) | 28 (65.1) | ||||||
Total mastectomy | 43 (40.2) | 31 (35.2) | 12 (63.2) | 28 (43.7) | 15 (34.9) | ||||||
ALN management | – | – | – | – | |||||||
SLNB | 50 (46.7) | 39 (44.3) | 11 (57.9) | 30 (46.9) | 20 (46.5) | ||||||
ALN dissection | 8 (7.5) | 5 (5.7) | 3 (15.8) | 7 (10.9) | 1 (2.3) | ||||||
None | 49 (45.8) | 44 (50.0) | 5 (26.3) | 27 (42.2) | 22 (51.2) | ||||||
ALN metastasis‡ | – | – | – | – | |||||||
No | 54 (93.1) | 42 (95.5) | 12 (85.7) | 35 (94.6) | 19 (90.5) | ||||||
Yes | 4 (6.9) | 2 (4.5) | 2 (14.3) | 2 (5.4) | 2 (9.5) | ||||||
Subtypes§ | – | – | – | – | |||||||
Luminal A | 65 (68.4) | 53 (69.8) | 12 (63.2) | 36 (64.3) | 29 (74.4) | ||||||
Luminal B | 28 (29.5) | 21 (27.6) | 7 (36.8) | 19 (33.9) | 9 (23.0) | ||||||
TN | 2 (2.1) | 2 (2.6) | 0 (0.0) | 1 (1.8) | 1 (2.6) |
Age in groups of all cases, EPC with INV and EPC without INV is presented as mean ± standard deviation, age in the training/test set and tumor size on US as median [interquartile range], and other features as number (frequency). †, 5 patients were male, 1 patient was unknown menopausal status, so there were only 101 masses; ‡, 49 patients did not undergo axillary lymph node surgery; §, 12 masses could not be subtyped due to HER2 score 2+ but no fluorescent in situ hybridization test. EPC, encapsulated papillary carcinoma; INV, invasive component; US, ultrasound; ALN, axillary lymph node; SLNB, sentinel lymph node biopsy; TN, triple negative.
Age, tumor size on US, family history of breast cancer, palpable ALN, and menopausal status were not significantly different between EPC with and without INV and between the training set and test set. The details of clinicopathological features are shown in Table 2.
US features between EPC without invasive carcinoma and EPC with invasive carcinoma
This study only presented hypoechoic and complex cystic and solid echo patterns. The most common features of EPC were enhanced or combined posterior acoustic features and no accompanying signs of ductal dilatation (both 89.8%). The following common features of EPC were the absence of calcification (86%), parallel orientation (83.2%), uncircumscribed margin (64.5%), abundant flow (63.6%), complex cystic and solid echo pattern (60.7%), and irregular shape (53.3%).
A total of 15 EPC cases with INV (79.0%) demonstrated an irregular shape, whereas those without INV were significantly more likely to have an oval to round shape (52.3%). The shape was statistically significant between EPC with INV and those without (P=0.031). There were no statistically significant differences in other US features between the 2 groups. All details are shown in Table 3.
Table 3
Features | EPC without INV (N=88), n (%) | EPC with INV (N=19), n (%) | χ2 | P value |
---|---|---|---|---|
Shape | 6.931 | 0.031 | ||
Oval | 10 (11.4) | 2 (10.5) | ||
Round | 36 (40.9) | 2 (10.5) | ||
Irregular | 42 (47.7) | 15 (79.0) | ||
Orientation | 0.654 | 0.419 | ||
Parallel | 72 (81.8) | 17 (89.5) | ||
Not parallel | 16 (18.2) | 2 (10.5) | ||
Margin | 2.110 | 0.146 | ||
Circumscribed | 34 (38.6) | 4 (21.1) | ||
Uncircumscribed | 54 (61.4) | 15 (78.9) | ||
Echo pattern | 1.621 | 0.203 | ||
Hypoechoic | 37 (42.0) | 5 (26.3) | ||
Complex cystic and solid | 51 (58.0) | 14 (73.7) | ||
Posterior acoustic features | 0.630 | 0.427 | ||
No changes and shadowing | 10 (11.4) | 1 (5.3) | ||
Enhancement and combined | 78 (88.6) | 18 (94.7) | ||
Calcifications | 0.234 | 0.629 | ||
No | 75 (85.2) | 17 (89.5) | ||
Yes | 13 (14.8) | 2 (10.5) | ||
Vascular† | 0.101 | 0.751 | ||
Less flow | 30 (35.7) | 6 (40.0) | ||
Abundant flow | 54 (64.3) | 9 (60.0) | ||
Ductal dilatation | 0.020 | 0.969 | ||
No | 79 (89.8) | 17 (89.5) | ||
Yes | 9 (10.2) | 2 (10.5) |
†, only 99 patients had color Doppler flow imaging images. US, ultrasound; EPC, encapsulated papillary carcinoma; INV, invasive component.
Radiomics models based on US images for predicting invasive carcinoma in the breast EPC
Of 461 radiomics features, 30 were related to INVs of EPC. Among the clinical and US features studied, only irregular shape was significantly associated with INV. However, since shape features were also included in the radiomic features, which overlap with those on US, irregular shape was not included in the final models. We used five ensemble machine learning classifiers to develop models, and RUS Boost had the best performance with AUC [95% confidence interval (CI)], ACC, SENS, and SPEC of 0.867 (0.753–0.96), 0.766, 0.917, and 0.731 in the training set, and 0.875 (0.750–0.974), 0.814, 0.857, and 0.806 in the test set, respectively (Figures 4,5). In addition, the models built by Random Forest, XGBoost, AdaBoost, and Easy Ensemble had moderate or good performance. The performance assessment indicators of the radiomics model are demonstrated in Table 4.
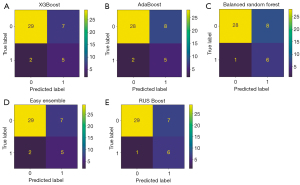
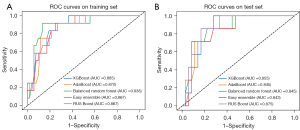
Table 4
Model | Training set | Test set | |||||||
---|---|---|---|---|---|---|---|---|---|
ACC | AUC (95% CI) | SENS | SPEC | ACC | AUC (95% CI) | SENS | SPEC | ||
XGBoost | 0.750 | 0.885 (0.797–0.967) | 0.917 | 0.712 | 0.791 | 0.855 (0.724–0.952) | 0.714 | 0.806 | |
AdaBoost | 0.750 | 0.870 (0.771–0.962) | 0.917 | 0.712 | 0.767 | 0.845 (0.696–0.960) | 0.714 | 0.778 | |
Balanced Random Forest | 0.797 | 0.938 (0.866–0.990) | 1.000 | 0.750 | 0.791 | 0.845 (0.693–0.962) | 0.857 | 0.778 | |
Easy Ensemble | 0.766 | 0.867 (0.771–0.960) | 0.917 | 0.731 | 0.791 | 0.843 (0.679–0.967) | 0.714 | 0.806 | |
RUS Boost | 0.766 | 0.867 (0.753–0.961) | 0.917 | 0.731 | 0.814 | 0.875 (0.750–0.974) | 0.857 | 0.806 |
US, ultrasound; EPC, encapsulated papillary carcinoma; ACC, accuracy; AUC, area under receiver operating characteristic curve; CI, confidence interval; SENS, sensitivity; SPEC, specificity; RUS, Random Under Sampling.
Discussion
This study showed that grayscale and color Doppler US had limited predictive value in identifying an INV of EPC, with only shape predicting an INV. In contrast, various radiomics methods were able to predict the INV of EPC with high predictive performance. In this study, the mean age and tumor size on US were similar to those in another Chinese study sample of 111 EPC cases (63.71 vs. 62 years; 23.40 vs. 22.5 mm) (3).
This study showed that the vast majority of EPC showed posterior acoustic enhancement and the absence of calcification, and more than half showed abundant blood flow, uncircumscribed margins, consistent with previous studies (3,8). However, this study showed that more than half of the EPC had irregularly shaped masses (53.3%), which differed from the aforementioned suggestion that EPCs are mostly oval to round (57–65%) (3,8). We speculate that this discrepancy may be sample biased. In addition, this study found that EPC with INV showed more irregular shape, uncircumscribed margin, and enhanced or combined posterior acoustic feature compared to EPC without INV. Notably, a statistically significant difference in shape between EPC with and without INV was observed in this study. Speer et al. (8) indicated a higher likelihood of an INV in core needle biopsies of irregularly shaped EPC tumors, somewhat supporting the above findings. One of the more distinctive US features of EPC previously reported was that occasional hemorrhage in the cystic component may produce a fluid-debris level (9), however, tumors with higher histological grade are more likely to exhibit hemorrhagic necrosis (20). This may be partially explained by that EPCs with INV have an increased manifestation of cystic-solid mixed echogenicity and combined posterior acoustic features compared to EPC without INV.
In recent years, radiomics has been increasingly integrated with US, widely in breast cancer diagnosis, differential diagnosis, and molecular marker prediction. Previously, two-dimensional (2D) US-based radiomics features have been shown to have better efficacy in classifying breast cancer (AUC >0.9) (21,22) as well as in differentiating phyllodes tumors or triple negative breast cancer from fibroadenoma (23,24) or identifying the presence of microinvasion in ductal carcinoma in situ (25). There were also elastography-based radiomics studies with good breast cancer identification efficacy, including shear wave elastography radiomics with AUC of 0.917 (22) and strain US radiomics with AUC of 0.917 (26). The investigators also successfully explored the correlation of biological features in ultrastructural breast cancer, finding significant associations of quantified US features with hormone receptor status, molecular subtype, histological grade, Ki-67, and P53 (27,28). Compared to breast magnetic resonance images (MRI), US radiomics has been less studied because of the relative difficulty in extracting features due to the low resolution of US, the blurred boundaries of some lesions, the irregularity of the imaged area, the high level of noise, and the high level of operator dependency. However, the development of MRI has be somewhat limited because of its high price and imaging complexity. US is widely used in clinical diagnosis because of its low price and rapid imaging. With the development of radiomics algorithms, including the use of new machine learning and deep learning algorithms, and the construction of US image homogenization algorithms, the development of US will continue to advance in the future.
To the best of our knowledge, this study is the first to use a radiomics approach to predict the presence or absence of accompanying INVs in EPC. In this study, 30 radiomics features related to INVs were selected from a large number of radiomics features (n=461), including 7 categories of shape, grayscale, boundary-based texture, wavelet features, GLRLM, GLSZM, and NGTDM. The best performing model was based on RUS Boost, which had good performance in both training and test sets, with AUCs (95% CI) of 0.867 (0.753–0.961) and 0.875 (0.750–0.974), respectively. The 4 remaining methods also had good predictive power with an AUC in the test set of 0.843–0.855. Therefore, the prediction ACC of several radiomics models developed in this study was higher than 0.767 for INV, which was significantly higher than that of core needle biopsy (40%). The present study showed that radiomics methods have a significant advantage in the prediction of INVs and several radiomics methods demonstrated high predictive accuracy. Thus, it suggested that US images contain microscopic features that reflect the pathological component, which can be explored and provided to the clinic using the radiomics approach.
The current treatment modality for EPC is surgical wide excision. However, it has also been suggested that treatment needs to consider its accompanying components. When cases with an INV are present, more attention needs to be paid to the incision margins and vigilant scanning of the ALNs to avoid missing suspicious ALNs or other important features. Ultimately, it has the potential to advance individualized patient care and reduce the need to consider additional procedures such as re-resection or sentinel lymph node biopsy due to the presence of an INV.
Our study has some limitations. First, the study was conducted retrospectively. Since US images were performed by several examiners and obtained from several US machines, image homogeneity was poor. However, given that future clinical applications will also be performed with different radiologists and US machines, this deficiency reflects real-world clinical practice with varied image quality. Of course, considering that the acquisition of radiomics features is greatly influenced by the quality of the images, it is still necessary to standardize the acquisition criteria of US images as much as possible in the future, including grayscale, depth, and cross-section, and also to increase the number of images saved to make the extracted features more stable and avoid outliers. Liu et al. (29) designed a 3 normalization module to normalize multicenter data. Therefore, image processing can also be performed using image normalization algorithms to homogenize images from different sources before studying them, which may reduce the influence of the subjectivity of US images to some extent. Second, this study was conducted in a single-center and lacked an external validation set. After we develop a unified image standard, we will continue to add cases from other hospitals in the future to train the model to improve accuracy and stability.
Conclusions
The US-based radiomics model has good performance in predicting EPC with an INV and can provide additional information to clinicians when determining the treatment management for EPC.
Acknowledgments
The authors would like to thank Ming Li (Department of Pathology, Fudan University Shanghai Cancer Center, Shanghai, China) for providing help with the pathology content.
Funding: This work was supported by
Footnote
Conflicts of Interest: All authors have completed the ICMJE uniform disclosure form (available at https://qims.amegroups.com/article/view/10.21037/qims-22-1069/coif). The authors have no conflicts of interest to declare.
Ethical Statement: The authors are accountable for all aspects of the work in ensuring that questions related to the accuracy or integrity of any part of the work are appropriately investigated and resolved. The study was conducted in accordance with the Declaration of Helsinki (as revised in 2013). The study was approved by the Ethics Board of Fudan University Shanghai Cancer Center (No. 2107238-18) and the requirement for individual consent for this retrospective analysis was waived.
Open Access Statement: This is an Open Access article distributed in accordance with the Creative Commons Attribution-NonCommercial-NoDerivs 4.0 International License (CC BY-NC-ND 4.0), which permits the non-commercial replication and distribution of the article with the strict proviso that no changes or edits are made and the original work is properly cited (including links to both the formal publication through the relevant DOI and the license). See: https://creativecommons.org/licenses/by-nc-nd/4.0/.
References
- Grabowski J, Salzstein SL, Sadler GR, Blair S. Intracystic papillary carcinoma: a review of 917 cases. Cancer 2008;113:916-20. [Crossref] [PubMed]
- Steponavičienė L, Gudavičienė D, Briedienė R, Petroška D, Garnelytė A. Diagnosis, treatment, and outcomes of encapsulated papillary carcinoma: a single institution experience. Acta Med Litu 2018;25:66-75. [Crossref] [PubMed]
- Zhang J, Zhang T, Wu N, Zhao X, Wang Q, Jiang Y, Gao M, Gu L. Intracystic papillary carcinoma of the breast: Experience of a major Chinese cancer center. Pathol Res Pract 2018;214:579-85. [Crossref] [PubMed]
- Hashmi AA, Iftikhar SN, Munawar S, Shah A, Irfan M, Ali J. Encapsulated Papillary Carcinoma of Breast: Clinicopathological Features and Prognostic Parameters. Cureus 2020;12:e11282. [Crossref] [PubMed]
- Fayanju OM, Ritter J, Gillanders WE, Eberlein TJ, Dietz JR, Aft R, Margenthaler JA. Therapeutic management of intracystic papillary carcinoma of the breast: the roles of radiation and endocrine therapy. Am J Surg 2007;194:497-500. [Crossref] [PubMed]
- Ding J, Wu W, Gan Y. Analysis of clinical-pathological features and prognosis of patients with encapsulated papillary carcinoma of the breast. China Oncol 2013;23:357-61.
- Hassan Z, Boulos F, Abbas J, El Charif MH, Assi H, Sbaity E. Intracystic papillary carcinoma: clinical presentation, patterns of practice, and oncological outcomes. Breast Cancer Res Treat 2020;182:317-23. [Crossref] [PubMed]
- Speer ME, Adrada BE, Arribas EM, Hess KR, Middleton LP, Whitman GJ. Imaging of Intracystic Papillary Carcinoma. Curr Probl Diagn Radiol 2019;48:348-52. [Crossref] [PubMed]
- Bonnet SE, Carter GJ, Berg WA. Encapsulated Papillary Carcinoma of the Breast: Imaging Features with Histopathologic Correlation. J Breast Imaging 2020;2:590-7.
- McCulloch GL, Evans AJ, Yeoman L, Wilson AR, Pinder SE, Ellis IO, Elston CW. Radiological features of papillary carcinoma of the breast. Clin Radiol 1997;52:865-8. [Crossref] [PubMed]
- Bodalal Z, Trebeschi S, Nguyen-Kim TDL, Schats W, Beets-Tan R. Radiogenomics: bridging imaging and genomics. Abdom Radiol (NY) 2019;44:1960-84. [Crossref] [PubMed]
- Wang K, Lu X, Zhou H, Gao Y, Zheng J, Tong M, Wu C, Liu C, Huang L, Jiang T, Meng F, Lu Y, Ai H, Xie XY, Yin LP, Liang P, Tian J, Zheng R. Deep learning Radiomics of shear wave elastography significantly improved diagnostic performance for assessing liver fibrosis in chronic hepatitis B: a prospective multicentre study. Gut 2019;68:729-41. [Crossref] [PubMed]
- Yu FH, Wang JX, Ye XH, Deng J, Hang J, Yang B. Ultrasound-based radiomics nomogram: A potential biomarker to predict axillary lymph node metastasis in early-stage invasive breast cancer. Eur J Radiol 2019;119:108658. [Crossref] [PubMed]
- Goldhirsch A, Winer EP, Coates AS, Gelber RD, Piccart-Gebhart M, Thürlimann B, Senn HJ. Panel members. Personalizing the treatment of women with early breast cancer: highlights of the St Gallen International Expert Consensus on the Primary Therapy of Early Breast Cancer 2013. Ann Oncol 2013;24:2206-23. [Crossref] [PubMed]
- Raji A. Human epidermal growth factor receptor 2 testing recommendation. J Clin Oncol 2007;25:4020-1; author reply 4021-3. [Crossref] [PubMed]
- Wolff AC, Hammond MEH, Allison KH, Harvey BE, Mangu PB, Bartlett JMS, Bilous M, Ellis IO, Fitzgibbons P, Hanna W, Jenkins RB, Press MF, Spears PA, Vance GH, Viale G, McShane LM, Dowsett M. Human Epidermal Growth Factor Receptor 2 Testing in Breast Cancer: American Society of Clinical Oncology/College of American Pathologists Clinical Practice Guideline Focused Update. J Clin Oncol 2018;36:2105-22. [Crossref] [PubMed]
- ACR BI-RADS® Ultrasound. Breast Imaging Reporting & Data System (BI-RADS®). Reston: American College of Radiology; 2013.
- Adler DD, Carson PL, Rubin JM, Quinn-Reid D. Doppler ultrasound color flow imaging in the study of breast cancer: preliminary findings. Ultrasound Med Biol 1990;16:553-9. [Crossref] [PubMed]
- Batista G, Prati RC, Monard MC. A study of the behavior of several methods for balancing machine learning training data. ACM SIGKDD Explorations Newsletter 2004;6:20-9.
- Stavros AT, Thickman D, Rapp CL, Dennis MA, Parker SH, Sisney GA. Solid breast nodules: use of sonography to distinguish between benign and malignant lesions. Radiology 1995;196:123-34. [Crossref] [PubMed]
- Luo WQ, Huang QX, Huang XW, Hu HT, Zeng FQ, Wang W. Predicting Breast Cancer in Breast Imaging Reporting and Data System (BI-RADS) Ultrasound Category 4 or 5 Lesions: A Nomogram Combining Radiomics and BI-RADS. Sci Rep 2019;9:11921. [Crossref] [PubMed]
- Youk JH, Kwak JY, Lee E, Son EJ, Kim JA. Grayscale Ultrasound Radiomic Features and Shear-Wave Elastography Radiomic Features in Benign and Malignant Breast Masses. Ultraschall Med 2020;41:390-6. [Crossref] [PubMed]
- Lee SE, Han K, Kwak JY, Lee E, Kim EK. Radiomics of US texture features in differential diagnosis between triple-negative breast cancer and fibroadenoma. Sci Rep 2018;8:13546. [Crossref] [PubMed]
- Niu S, Huang J, Li J, Liu X, Wang D, Wang Y, Shen H, Qi M, Xiao Y, Guan M, Li D, Liu F, Wang X, Xiong Y, Gao S, Wang X, Yu P, Zhu J. Differential diagnosis between small breast phyllodes tumors and fibroadenomas using artificial intelligence and ultrasound data. Quant Imaging Med Surg 2021;11:2052-61. [Crossref] [PubMed]
- Zhu M, Pi Y, Jiang Z, Wu Y, Bu H, Bao J, Chen Y, Zhao L, Peng Y. Application of deep learning to identify ductal carcinoma in situ and microinvasion of the breast using ultrasound imaging. Quant Imaging Med Surg 2022;12:4633-46. [Crossref] [PubMed]
- Zhang Q, Xiao Y, Suo J, Shi J, Yu J, Guo Y, Wang Y, Zheng H. Sonoelastomics for Breast Tumor Classification: A Radiomics Approach with Clustering-Based Feature Selection on Sonoelastography. Ultrasound Med Biol 2017;43:1058-69. [Crossref] [PubMed]
- Guo Y, Hu Y, Qiao M, Wang Y, Yu J, Li J, Chang C. Radiomics Analysis on Ultrasound for Prediction of Biologic Behavior in Breast Invasive Ductal Carcinoma. Clin Breast Cancer 2018;18:e335-44.
- Cui H, Zhang D, Peng F, Kong H, Guo Q, Wu T, Wen X, Zhang L, Tian J. Identifying ultrasound features of positive expression of Ki67 and P53 in breast cancer using radiomics. Asia Pac J Clin Oncol 2021;17:e176-84.
- Liu C, Qiao M, Jiang F, Guo Y, Jin Z, Wang Y. TN-USMA Net: Triple normalization-based gastrointestinal stromal tumors classification on multicenter EUS images with ultrasound-specific pretraining and meta attention. Med Phys 2021;48:7199-214. [Crossref] [PubMed]