Dynamic contrast-enhanced magnetic resonance imaging radiomics analysis based on intratumoral subregions for predicting luminal and nonluminal breast cancer
Introduction
Breast cancer is the most common malignant tumor threatening women’s health worldwide and one of the most common causes of cancer-related death in women. The incidence of breast cancer in most countries has been on the rise over the past few decades (1). Breast cancer is a heterogeneous disease with different morphological and biological characteristics (2,3). The molecular subtype of breast cancer is strongly linked to the treatment and prognosis of patients (4,5). Breast cancer can be divided into the luminal type and nonluminal type according to progesterone receptor (PR) and estrogen receptor (ER) expression (6). The luminal type, which includes luminal A and luminal B types, is sensitive to endocrine therapy and has a low rate of distant metastasis and good prognosis (7). The nonluminal type includes the human epidermal growth factor receptor 2 (HER2) overexpression type and triple-negative (TN) type, which are insensitive to endocrine therapy. Nonluminal breast cancer is more prone to tumor recurrence and metastasis and has a poor prognosis (8,9). The accurate determination of molecular subtypes is thus critical for the targeted treatment of patients with breast cancer.
Dynamic contrast-enhanced magnetic resonance imaging (DCE-MRI) can provide high-resolution tumor anatomical information and functional information related to angiogenesis and blood flow status (10-12). Data from DCE-MRI images have been used to quantitatively evaluate tumor heterogeneity and to establish prediction models for molecular subtypes or the ER/PR expression status of breast cancer (13-18). Lafcı et al. identified radiomics features that could distinguish luminal A from luminal B breast cancer by extracting and analyzing the features from T1-weighted DCE-MRI (16). In another study, Zhong et al. successfully constructed a radiomics score (Rad-score) to valuated ER and PR status in preoperative patients with breast cancer according to the texture features of the functional parameter maps calculated by breast DCE-MRI (17). These studies suggest that quantitative features from DCE-MRI images have the potential to predict lumen types in breast cancer.
Previous studies have obtained valuable information to quantify the extent of tumor heterogeneity based on the analysis of the whole tumor. However, intratumoral regions that exhibit different dynamic patterns may provide useful information that cannot be acquired by radiomics analysis of the whole tumor (19-21). The features extracted from intratumoral subregions have been used to predict the pathological complete response after neoadjuvant chemotherapy (NAC) (22,23). Other studies have examined the use of texture features inside tumors to distinguish between benign and malignant tumors, identify the HER2 status, and predict the amplification status of the Ki-67 index (24-26). However, it remains unclear whether intratumoral regional analysis is more effective than whole tumor analysis for differentiating the luminal types of breast cancer.
The purpose of this study was to establish a nomogram combining Rad-score and clinical factors for the personalized prediction of luminal and nonluminal breast cancer.
Methods
Patients
This study was conducted in accordance with the Declaration of Helsinki (revised in 2013) and approved by the Ethics Review Committee of Shengjing Hospital Affiliated to China Medical University. In view of the retrospective nature of this study, the need for informed consent was waived. Between January 2018 and January 2021, 300 patients with breast lesions detected with DCE-MRI were continuously collected and screened according to the following inclusion and exclusion criteria. The inclusion criteria were as follows: (I) breast DCE-MRI performed before biopsy or resection, and (II) available pathological results. The exclusion criteria were as follows: (I) incomplete pathological results, (II) patients who received chemotherapy or radiotherapy, and (III) presence of image artifacts. Finally, 153 female patients with a mean age of 50.08 years were included in the analysis and randomly divided into a training cohort (108 patients) and a validation cohort (45 patients). Clinical factors including age, HER2 status, Ki-67 status, histological grade, apparent diffusion coefficient (ADC) value, Breast Imaging Reporting & Data System (BI-RADS) category, and time–intensity curve (TIC) type were obtained from the imaging system. The flowchart of this study is shown in Figure 1.
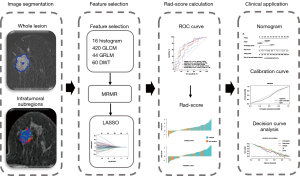
Imaging protocol
Detailed information on DCE-MRI image acquisition methods and imaging parameters are described in the Appendix 1.
Pathologic assessment
ER, PR, and HER2 expression was detected using streptavidin peroxidase immunohistochemistry (IHC). The detailed pathological analysis is presented in Appendix 1.
Tumor segmentation
A total of 3 experienced radiologists used the semiautomatic segmentation method to complete the segmentation of tumors and finally reached a consensus. The specific tumor segmentation methods are described in Appendix 1.
Intratumoral subregion partition
To analyze tumor heterogeneity, the whole tumor area was divided into 3 subregions on the basis of the changes in pixel intensity at different imaging stages according to a previously reported method (26). Briefly, the specific approach proceeded follows:
First, the following equation was used to calculate the relative enhancement via comparing the postcontrast image and the pre-contrast image pixel by pixel:
where I(p, q, t) and I(p, q, t0) represent the signal strength of the pixel (p, q) obtained at times t and t0 (the precontrast moment) (27), and E (p, q, t) is the time signal intensity curve, which depicts the variation of relative enhancement with time (28,29). Time to peak (TTP), the arrival time of peak enhancement, was calculated as follows:
The pixels of the whole tumor region were then partitioned according to the TTP value. The lesion pixels that reached the peak enhancement value in the first to fourth, fifth or sixth, and seventh or eighth stages were divided into rapid, medium, and slow subregions, respectively. Therefore, the whole lesion was divided into 3 subregions representing different TTP values. Figure 2 shows the tumor segmentation results of 2 random cases.
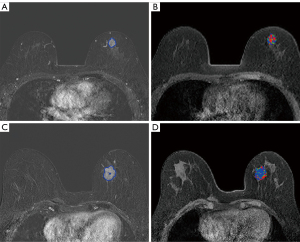
Feature extraction
MATLAB 2018b (MathWorks) was used to extract 540 texture features from 3 intratumoral subregions and the whole tumor region, respectively. The extracted features were summarized into 4 types, information of which are provided in Table 1. For the detailed description of these features, please refer to Appendix 1. In the MRI subtraction image, the features extracted from the precontrast, median (the fourth), and last (the eighth) subtraction MR images were named S-0, S-4, and S-8 respectively. A precontrast sequence was included in the analysis based on previous studies showing that it was associated with the molecular type of breast cancer (15,30).
Table 1
Category | Texture features | Quantity |
---|---|---|
Histogram | Max, min, range, interquartile range, mean, median, standard, variance, skewness, kurtosis, covariance, 1st percentile, 10th percentile, 50th percentile, 90th percentile, 99th percentile | 16 |
GLCM† | Autocorrelation (ACOR), contrast (CON), correlation (COR), cluster prominence (CP), cluster shade (CS), dissimilarity (DIS), joint energy (JENE), joint entropy (JENT), homogeneity (HOM), maximum probability (MP), sum of squares (SOS), sum average (SA), sum variance (SV), sum entropy (SE), difference variance (DV), difference entropy (DE), information measure of correlation 1 (IMC1), information measure of correlation 2 (IMC2), inverse difference normalized (IDN), inverse different moment (IDM), inverse difference moment normalized (IDMN) | 420 |
GLRLM‡ | Run-length nonuniformity (RLN), gray-level nonuniformity (GLN), long-run emphasis (LRE), short-run emphasis (SRE), run percentage (RP), low gray-level run emphasis (LGLRE), high gray-level run emphasis (HGLRE), short-run low gray-level emphasis (SRLGLE), short-run high gray-level emphasis (SRHGLE), long-run low gray-level emphasis (LRLGLE), long-run high gray-level emphasis (LRHGLE) | 44 |
DWT§ | Harr parameters | 20 |
Daubechies2 parameters | 20 | |
Symlet4 parameters | 20 | |
Total | 540 |
†, GLCM parameters were calculated for 4 distances (1, 2, 3, and 4 pixels) and 4 angles (0°, 45°, 90°, and 135°). (d, 0), (0, d), (d, d), and (–d, –d) represent 0°, 45°, 90°, and 135°, respectively, where d is the distance. For example, CON (0, 1) represents the contrast feature calculated for a distance of 1 and a direction of 90°. ‡, GLRLM parameters were calculated for 4 angles (0°, 45°, 90°, and 135°). §, DWT parameters were calculated for 4 layers and 3 directions (horizontal, vertical, diagonal) to produce low and high frequency components. For example, Harr_4_H_V represents the vertical high frequency component of the fourth layer using the Harr wavelet. DWT, discrete wavelet transformation; GLCM, gray-level co-occurrence matrix; GLRLM, gray-level run-length matrix.
Interobserver variability assessment
Readers 1 and 2 randomly selected 50 cases from all the out-of-order images for region of interest (ROI) segmentation and then extracted the features. The features were then evaluated using the intraclass correlation coefficient (ICC) (31). The features with ICC >0.8 were considered to have good reproducibility and stability and were retained for subsequent radiomics analysis.
Feature selection and radiomics score calculation
From the features with good stability in the training cohort, 100 features with high correlation and low redundancy were selected using the minimum redundancy maximum relevance method. The least absolute shrinkage and selection operator method was then used to select the most useful prediction features from the dataset through 10-fold cross-validation, thereby reducing the dimension of the feature set (32). Support vector machine (SVM) and logistic regression (LR) classifiers were developed based on the features of each subregion, 3 subregions, and the whole region, and their discrimination performance was evaluated using the area under the receiver operating characteristic (ROC) curve (AUC). Finally, the Rad-score of each patient was calculated according to the linear combination of the respective coefficient weights of the selected features.
Development of the nomogram
A nomogram for predicting luminal and nonluminal breast cancer was developed using data from the training cohort. Univariate and multivariate logistic regression analyses were performed to identify important factors associated with the luminal classification of breast cancer. Candidate factors included all clinical factors. Factors with P<0.1 in univariate analyses were included in multivariate analyses (33). The Akaike information criterion was used as the likelihood ratio test of the stop rule, and forward step-by-step selection was applied for multivariate analysis in the training cohort. The variance inflation factor (VIF) was used to estimate the collinearity diagnosis of multivariable logistic regression. Finally, the independently predicted values were combined to obtain a nomogram.
Validation of the nomogram
ROC curves were drawn to assess the performance of the nomogram for differentiating luminal and nonluminal breast cancer in the training and validation cohorts, and the AUC was calculated to quantify discrimination. The calibration curve was drawn to analyze the consistency between the predicted risk and observed risk of luminal breast cancer for determining the prediction accuracy of the nomogram in 2 cohorts (34). By quantifying the net benefit of different threshold probabilities in the validation cohort, decision curve analysis (DCA) was performed to determine the clinical usefulness of the nomogram (35).
Statistical analysis
SPSS 23.0 and R software (version 4.4.1) were used for statistical analyses, while the χ2 test was used for comparative analyses. Continuous variables satisfying the normal distribution were compared with Student t test. The Mann-Whitney test was used to compare continuous variables with nonnormal or unknown distribution. Two-sided P values <0.05 were considered statistically significant. The ROC curve was drawn with the maximum Youden Index as the best cutoff value, and AUC, sensitivity, specificity, accuracy, positive predictive value, and negative predictive value were calculated as comparison indicators (36,37). Delong test was used to statistically compare the AUC values of the 2 models (38). ROC curves were plotted using MedCalc software (version 20.0, MedCalc Software Ltd.).
Results
Clinical characteristics
Table 2 shows the basic information of the study cohort. HER2 status differed significantly between the 2 cohorts (P=0.019), but there was no significant difference between the 2 cohorts in other basic clinical characteristics (P>0.05). Other details of patients are shown in Table 2.
Table 2
Characteristics | Training cohort (n=108) | Validation cohort (n=45) | P† | |||||
---|---|---|---|---|---|---|---|---|
Nonluminal (n=39) | Luminal (n=69) | P | Nonluminal (n=16) | Luminal (n=29) | P | |||
Age, mean ± SD, years | 51.90±9.86 | 48.17±8.95 | 0.048 | 50.00±7.49 | 52.21±10.50 | 0.463 | 0.278 | |
ADC value, median (IQR) | 1.06 (0.96, 1.23) | 0.98 (0.85, 1.08) | 0.015 | 1.06 (0.90, 1.15) | 0.90 (0.83, 1.08) | 0.046 | 0.322 | |
TIC, % | 0.664 | 0.900 | 0.137 | |||||
Wash-out | 29 (74.36) | 48 (69.57) | 13 (81.25) | 24 (82.75) | ||||
Plateau | 8 (20.51) | 19 (27.53) | 3 (18.75) | 5 (17.25) | ||||
Wash-in | 2 (5.13) | 2 (2.89) | 0 (0.00) | 0 (0.00) | ||||
BI-RADS, % | 0.168 | 0.104 | 0.822 | |||||
4A | 0 (0.00) | 2 (2.90) | 0 (0.00) | 0 (0.00) | ||||
4B | 5 (12.82) | 10 (14.49) | 2 (12.50) | 6 (20.69) | ||||
4C | 22 (56.41) | 44 (63.77) | 8 (50.00) | 19 (65.52) | ||||
5 | 12 (30.77) | 13 (18.84) | 6 (37.50) | 4 (13.79) | ||||
Ki-67, % | 0.730 | 0.742 | 0.716 | |||||
Low | 9 (23.08) | 18 (26.09) | 4 (25.00) | 6 (20.69) | ||||
High | 30 (76.12) | 51 (73.91) | 12 (75.00) | 23 (79.31) | ||||
HER2, % | <0.001 | 0.003 | 0.019 | |||||
Negative | 13 (33.33) | 48 (69.57) | 1 (6.25) | 15 (51.72) | ||||
Positive | 26 (66.67) | 21 (30.43) | 15 (93.75) | 14 (48.28) | ||||
Histologic grade, % | 0.001 | 0.017 | 0.531 | |||||
I and II | 26 (66.67) | 65 (94.20) | 10 (62.50) | 27 (93.10) | ||||
III | 13 (33.33) | 4 (5.80) | 6 (37.50) | 2 (6.90) | ||||
Rad-score, median (IQR) | 0.25 (−0.19, 0.58) | 0.98 (0.37, 1.56) | <0.001 | 0.10 (−0.55, 0.53) | 0.81 (0.25, 1.54) | 0.017 | 0.200 |
P value was obtained from univariate association analysis between each clinicopathological variable and breast cancer type. P† indicates the comparative analysis between the training cohort and the validation cohort. ADC, apparent diffusion coefficient; BI-RADS, breast imaging reporting and data system; HER2, human epidermal growth factor receptor 2; IQR, interquartile range; Rad-score, radiomics score; SD, standard deviation; TIC, time-intensity curve.
Feature selection and radiomics score calculation
Finally, 1,598, 1,603, 1,582, and 1,611 features in the rapid subregion, medium subregion, slow subregion, and whole tumor region showed good stability, respectively, with 5, 1, 5, and 1 effective features being left after the feature selection step, respectively. Table 3 lists each feature in detail and shows their predictive performance. In general, the features belonging to the rapid subregion performed best in the 3 subregions, and the highest AUC values in the training and validation cohort were 0.805 and 0.737, respectively. In the training cohort, the AUC values for skewness (S-0), Daubechies wavelet 24 HV (S-0), and skewness (S-8) from the rapid subregion were higher than those of the whole tumor region, with P values of 0.032, 0.021, and 0.011, respectively.
Table 3
Method | Subregions | Feature | Cohort | AUC | Interval | P† |
---|---|---|---|---|---|---|
Intratumoral Regionalization | Rapid | Skewness (S-0) | Training | 0.796 | 0.705–0.888 | 0.032 |
Validation | 0.726 | 0.570–0.882 | 0.078 | |||
Daubechies2_4_H_V (S-0) | Training | 0.548 | 0.535–0.603 | 0.021 | ||
Validation | 0.515 | 0.315–0.715 | 0.813 | |||
SRHGLE_0 (S-4) | Training | 0.741 | 0.646–0.836 | 0.128 | ||
Validation | 0.584 | 0.397–0.771 | 0.599 | |||
GLN_135 (S-4) | Training | 0.628 | 0.514–0.743 | 0.969 | ||
Validation | 0.621 | 0.434–0.807 | 0.417 | |||
Skewness (S-8) | Training | 0.805 | 0.719–0.892 | 0.011 | ||
Validation | 0.737 | 0.581–0.893 | 0.085 | |||
Medium | Daubechies2_4_H_V (S-4) | Training | 0.724 | 0.624–0.825 | 0.263 | |
Validation | 0.640 | 0.468–0.812 | 0.347 | |||
Slow | Skewness (S-0) | Training | 0.651 | 0.538–0.764 | 0.816 | |
Validation | 0.608 | 0.417–0.799 | 0.546 | |||
Skewness (S-4) | Training | 0.713 | 0.613–0.813 | 0.299 | ||
Validation | 0.616 | 0.436–0.797 | 0.458 | |||
RP_0 (S-4) | Training | 0.604 | 0.493–0.716 | 0.745 | ||
Validation | 0.625 | 0.450–0.800 | 0.414 | |||
Harr_4_H_H (S-4) | Training | 0.639 | 0.528–0.751 | 0.930 | ||
Validation | 0.621 | 0.437–0.805 | 0.444 | |||
Harr_4_H_D (S-4) | Training | 0.742 | 0.638–0.846 | 0.142 | ||
Validation | 0.603 | 0.436–0.771 | 0.451 | |||
Whole Lesion | – | Covariance (S-8) | Training | 0.632 | 0.520–0.744 | – |
Validation | 0.522 | 0.343–0.701 | – |
†, P value represents the performance comparison between the features from the intratumoral subregions and the feature from the whole tumor region. AUC, area under the receiver operating characteristic curve; SRHGLE, short-run high gray-level emphasis; GLN, gray-level nonuniformity; RP, run percentage.
Tables 4,5 show the performance of the 2 classifier models in identifying the luminal and nonluminal breast cancer in 2 cohorts. The relevant ROC curves are shown in Figure 3A-3D. Regardless of the classifier, the features belonging to the rapid subregion showed the most stable classification performance. The Delong test results of each classification model are shown in Table S1.
Table 4
Regions | Cohort | AUC (95% CI) | SEN (%) | SPE (%) | ACC (%) | PPV (%) | NPV (%) |
---|---|---|---|---|---|---|---|
Rapid | Training | 0.731 (0.635, 0.826) | 59.42 | 82.05 | 69.44 | 69.14 | 71.43 |
Validation | 0.736 (0.626, 0.900) | 51.72 | 100 | 66.67 | 70.59 | 54.55 | |
Medium | Training | 0.619 (0.509, 0.729) | 92.75 | 28.21 | 67.59 | 67.00 | 75.00 |
Validation | 0.681 (0.519, 0.843) | 51.72 | 81.25 | 55.56 | 60.98 | 0.00 | |
Slow | Training | 0.754 (0.653, 0.855) | 78.26 | 69.23 | 71.30 | 70.21 | 78.57 |
Validation | 0.656 (0.494, 0.817) | 41.38 | 93.75 | 64.44 | 71.88 | 46.15 | |
Combined | Training | 0.780 (0.690, 0.854) | 63.77 | 76.82 | 72.97 | 75.78 | 78.60 |
Validation | 0.692 (0.537, 0.821) | 52.46 | 56.25 | 61.20 | 65.23 | 50.20 | |
Whole lesion | Training | 0.657 (0.539, 0.776) | 88.41 | 43.59 | 71.30 | 69.39 | 90.00 |
Validation | 0.616 (0.448, 0.785) | 55.17 | 75.00 | 55.56 | 60.97 | 0.00 |
ACC, accuracy; AUC, area under the receiver operating characteristic curve; CI, confidence interval; NPV, negative predictive value; PPV, positive predictive value; SEN, sensitivity; SPE, specificity; SVM, support vector machine.
Table 5
Regions | Cohort | AUC (95% CI) | SEN (%) | SPE (%) | ACC (%) | PPV (%) | NPV (%) |
---|---|---|---|---|---|---|---|
Rapid | Training | 0.744 (0.651, 0.823) | 63.77 | 82.05 | 70.37 | 86.27 | 56.14 |
Validation | 0.718 (0.564, 0.842) | 65.52 | 75.00 | 68.89 | 82.61 | 54.55 | |
Medium | Training | 0.623 (0.525, 0.715) | 62.32 | 58.97 | 61.11 | 72.88 | 46.94 |
Validation | 0.578 (0.421, 0.723) | 48.28 | 81.25 | 60.00 | 82.35 | 46.43 | |
Slow | Training | 0.755 (0.663, 0.832) | 66.67 | 76.92 | 70.37 | 86.27 | 56.14 |
Validation | 0.616 (0.460, 0.757) | 48.28 | 81.25 | 60.00 | 82.35 | 46.43 | |
Combined | Training | 0.804 (0.716, 0.874) | 73.54 | 66.25 | 74.87 | 90.06 | 69.65 |
Validation | 0.634 (0.477, 0.772) | 59.45 | 72.50 | 62.69 | 84.37 | 41.84 | |
Whole lesion | Training | 0.597 (0.498, 0.690) | 17.39 | 100.00 | 47.22 | 100 | 40.63 |
Validation | 0.571 (0.415, 0.718) | 72.41 | 56.25 | 66.67 | 75.00 | 52.94 |
ACC, accuracy; AUC, area under the receiver operating characteristic curve; CI, confidence interval; LR, logistic regression; NPV, negative predictive value; PPV, positive predictive value; SEN, sensitivity; SPE, specificity.
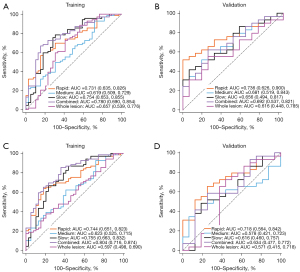
According to the analysis results, we selected the features extracted from the rapid subregion to calculate the Rad-score. The corresponding calculation formula is provided in the Appendix 1. There was no significant difference in the distribution of Rad-scores between the training and validation cohort (P=0.200). Patients with luminal breast cancer had higher Rad-scores (Table 2). The bar graph in Figure S1 shows the good discrimination performance of the Rad-score.
Development of the nomogram
Rad-score, HER2 status, and histological grade were identified as independent predictors according to the results of logistic regression analysis. The VIF was 1.050–1.117, indicating that all variables were not collinear. The independent predictors identified were used to develop a prediction model in the form of a nomogram (Figure 4A).
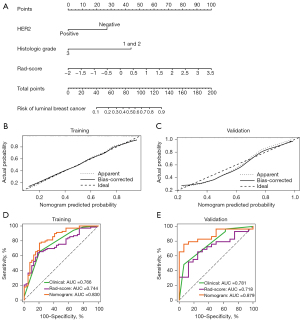
Validation of the nomogram
Table 6 shows the performance of the nomogram, clinical factors, and Rad-score for distinguishing the luminal types of breast cancer. The AUC values for the nomogram, clinical factors, and Rad-score were 0.830 (95% CI: 0.746–0.896), 0.766 (95% CI: 0.675–0.842), and 0.744 (95% CI: 0.651–0.823) in the training cohort and 0.879 (95% CI: 0.748–0.957), 0.781 (95% CI: 0.633–0.891), and 0.718 (95% CI: 0.564–0.842) in the validation cohort, respectively. In the training cohort, the performance of the nomogram was better than that of any single clinical variable (P=0.016) and Rad-score (P=0.023). The performance of the nomogram in the validation cohort was also better than that of the Rad-score (P=0.017). Clinical predictors and Rad-score showed similar discrimination performance in the 2 cohorts. The calibration curves (Figure 4B,4C) showed good consistency between predictions and observations. Figure 4D,4E showed the ROC curves of the nomogram, clinical factors, and Rad-score performance in differentiating luminal types in each cohort.
Table 6
Cohort | Nomogram | Clinical | Rad-score | P | ||
---|---|---|---|---|---|---|
Nomogram vs. clinical | Nomogram vs. Rad-score | Clinical vs. Rad-score | ||||
Training cohort (n=108) | 0.016 | 0.023 | 0.708 | |||
AUC (95% CI) | 0.830 (0.746, 0. 896) | 0.766 (0.675, 0.842) | 0.744 (0.651, 0.823) | |||
ACC | 0.778 | 0.704 | 0.704 | |||
SEN | 0.768 | 0.652 | 0.638 | |||
SPE | 0.795 | 0.795 | 0.821 | |||
Validation cohort (n=45) | 0.068 | 0.017 | 0.546 | |||
AUC (95% CI) | 0.879 (0.748, 0.957) | 0.781 (0.633, 0.891) | 0.718 (0.564, 0.842) | |||
ACC | 0.822 | 0.644 | 0.689 | |||
SEN | 0.759 | 0.483 | 0.655 | |||
SPE | 0.938 | 0.75 | 0.938 |
ACC, accuracy; AUC, area under the receiver operating characteristic curve; CI, confidence interval; Rad-score, radiomics score; SEN, sensitivity; SPE, specificity.
As shown in Figure 5, the DCA results supported the clinical usefulness of the nomogram. The greatest benefits of the nomogram model were obtained when the threshold probability was in the range of 3–82%. The use of the nomogram to identify luminal breast cancer was more effective than was using only clinical variables or Rad-score.
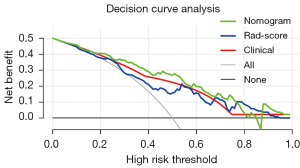
Discussion
In this study, we used the intratumoral partitioning method to divide the tumor region into 3 subregions and extract features to differentiate between luminal and nonluminal breast cancer. The ROC curves indicated that the features belonging to the rapid subregion had the best performance. The Rad-score was calculated with these features, and a nomogram model was established including Rad-score, HER2 status, and histological grade. The results demonstrated that the nomogram had good discrimination and calibration performance, and DCA supported its clinical usefulness.
In the analysis of tumor heterogeneity, most previous studies included the whole tumor region through various sequences based on DCE-MRI (13,15,39,40). However, tumor subregions with different dynamic enhancement patterns are associated with different biological processes and thus different prognoses, and they may have valuable information that cannot be captured by analyzing the whole tumor (19,27,41,42). Wu et al. collected 4 quantitative Haralick texture features in each tumor subregion based on gray-level co-occurrence matrix (GLCM) and used the changes of texture features to predict the pathological complete response after neoadjuvant chemotherapy. The AUC of the features from the intratumor subregion was between 0.75 and 0.80, which was significantly better than the texture analysis based on the whole lesion (AUC =0.65) (22). In this study, we extracted features from the whole tumor lesion and 3 intratumoral subregions segmented based on TTP values and then established classifier models to distinguish between luminal and nonluminal breast cancer. Finally, the predictive performance of the LR model constructed with features from the rapid and slow subregions in the training cohort was found to be better than the model based on the whole tumor region (P=0.023, P=0.047). One possible explanation for this result is that these tumor subregions may embody angiogenesis, which may reflect the biomarker status related to different types of tumors (13,43).
Intratumoral heterogeneity refers to differences in biological characteristics, such as gene expression, angiogenesis, and metabolism (20,44). The close correlation between the microscopic characteristics of medical images and the microstructure and biological characteristics of tumors has been confirmed (45,46). However, the correlation between tumor biological characteristics and radiomics features is complex, and identifying suitable biomarkers to understand the relationship between radiomics features and biological behavior is challenging (47). One useful approach is to use radiomics to identify multiple parameters for the construction of prediction models (48,49). In this study, a Rad-score was developed using 5 features from the rapid subregion. The features used to calculate Rad-score were mainly histogram and gray-level run length matrix (GLRLM). Histogram features can describe the distribution of voxel intensity in the image area, and GLRLM can quantify the gray-level run of continuous pixels with the same gray value. Rad-score, which combines these features, performed well as an independent predictor in distinguishing luminal types, with AUC values in the training and validation cohorts of 0.744 and 0.718, respectively.
Routine clinical testing of molecular subtypes of breast cancer relies on invasive tissue sampling and genetic analysis, which is inherently limited because biopsies performed at a single point in time do not reflect genetic heterogeneity within the breast tumor (50,51). At present, the prediction of breast molecular subtypes requires not only a doctor’s visual examination but simultaneous IHC as well. IHC is a method of tissue sampling that both time-consuming invasive to patients. In addition, in order to more accurately determine the molecular typing of the breast, further fluorescence in situ hybridization analysis is needed, further increasing the time and cost of the procedure. In our study, quantitative features were extracted and analyzed from medical images, and the extracted features were used for screening modeling to predict breast cancer luminal types noninvasively. Meanwhile, a nomogram combining clinicopathological factors and Rad-score was also proposed. The use of nomograms for predicting prognosis and results is common. Previous studies have shown that a nomogram model, that combined Rad-score and clinical factors had better predictive performance than radiomics model or clinical model alone (52-55). In Luo et al.’s study (52), Rad-score, HER2 status, and tumor histological grade were independent predictors of luminal breast cancer. HER2-positive status was significantly associated with nonluminal breast cancer, which was consistent with previous research (56). The nomogram combined these 3 variables to differentiate luminal and nonluminal breast cancer and showed good discrimination performance (AUC =0.879), which was significantly better than that of the Rad-score in the validation cohort (P=0.017). This suggests that the combination of the Rad-score and clinical factors can improve the diagnostic performance of the radiomics model. Several recent studies support the value of combining the Rad-score and clinical factors in establishing radiomics models to predict tumor molecular type (56-58). The results of DCA confirmed the usefulness of the nomogram for clinical application and demonstrated that the nomogram was more effective for predicting breast tumor types than was the all-treatment scheme (assuming that all breast tumors were luminal) or no-treatment scheme (assuming that all breast tumors were nonluminal).
This study had several limitations. First, the sample size was small, and patient data were obtained from a single hospital. Second, we analyzed single-layer images obtained at the largest cross-section of the representative tumor, which might have led to the omission of useful information regarding the tumor (59). Third, the inclusion of a greater number of radiomics features and the combination of DCE-MRI with other imaging methods could improve the accuracy of prediction. Finally, the complexity of the process for calculating the Rad-score in this study may present obstacles to its implementation in daily practice. In future research, efforts will be made to find more simplified biomarkers.
Conclusions
In conclusion, this study proposed a nomogram combining clinical factors and Rad-score, which performed well in distinguishing luminal and nonluminal breast cancer. Further validation of the present results is needed before the clinical application of the model.
Acknowledgments
We would like to thank International Science Editing (http://www.internationalscienceediting.com) for editing this manuscript.
Funding: None.
Footnote
Conflicts of Interest: Both authors have completed the ICMJE uniform disclosure form (available at https://qims.amegroups.com/article/view/10.21037/qims-22-1073/coif). The authors have no conflicts of interest to declare.
Ethical Statement: The authors are accountable for all aspects of the work in ensuring that questions related to the accuracy or integrity of any part of the work are appropriately investigated and resolved. The study was conducted in accordance with the Declaration of Helsinki (as revised in 2013) and was approved by the Ethics Review Committee of Shengjing Hospital Affiliated to China Medical University. In view of the retrospective nature of this study, individual consent for this retrospective analysis was waived.
Open Access Statement: This is an Open Access article distributed in accordance with the Creative Commons Attribution-NonCommercial-NoDerivs 4.0 International License (CC BY-NC-ND 4.0), which permits the non-commercial replication and distribution of the article with the strict proviso that no changes or edits are made and the original work is properly cited (including links to both the formal publication through the relevant DOI and the license). See: https://creativecommons.org/licenses/by-nc-nd/4.0/.
References
- Siegel RL, Miller KD, Jemal A. Cancer statistics, 2020. CA Cancer J Clin 2020;70:7-30. [Crossref] [PubMed]
- Weigelt B, Geyer FC, Reis-Filho JS. Histological types of breast cancer: how special are they? Mol Oncol 2010;4:192-208. [Crossref] [PubMed]
- Abramson VG, Lehmann BD, Ballinger TJ, Pietenpol JA. Subtyping of triple-negative breast cancer: implications for therapy. Cancer 2015;121:8-16. [Crossref] [PubMed]
- Sanges F, Floris M, Cossu-Rocca P, Muroni MR, Pira G, Urru SAM, et al. Histologic subtyping affecting outcome of triple negative breast cancer: a large Sardinian population-based analysis. BMC Cancer 2020;20:491. [Crossref] [PubMed]
- Dawood S, Hu R, Homes MD, Collins LC, Schnitt SJ, Connolly J, Colditz GA, Tamimi RM. Defining breast cancer prognosis based on molecular phenotypes: results from a large cohort study. Breast Cancer Res Treat 2011;126:185-92. [Crossref] [PubMed]
- Dai X, Xiang L, Li T, Bai Z. Cancer Hallmarks, Biomarkers and Breast Cancer Molecular Subtypes. J Cancer 2016;7:1281-94. [Crossref] [PubMed]
- Schnitt SJ. Classification and prognosis of invasive breast cancer: from morphology to molecular taxonomy. Mod Pathol 2010;23:S60-4. [Crossref] [PubMed]
- Su Y, Zheng Y, Zheng W, Gu K, Chen Z, Li G, Cai Q, Lu W, Shu XO. Distinct distribution and prognostic significance of molecular subtypes of breast cancer in Chinese women: a population-based cohort study. BMC Cancer 2011;11:292. [Crossref] [PubMed]
- Engstrøm MJ, Opdahl S, Hagen AI, Romundstad PR, Akslen LA, Haugen OA, Vatten LJ, Bofin AM. Molecular subtypes, histopathological grade and survival in a historic cohort of breast cancer patients. Breast Cancer Res Treat 2013;140:463-73. [Crossref] [PubMed]
- Nagy JA, Chang SH, Dvorak AM, Dvorak HF. Why are tumour blood vessels abnormal and why is it important to know? Br J Cancer 2009;100:865-9. [Crossref] [PubMed]
- Karahaliou A, Vassiou K, Arikidis NS, Skiadopoulos S, Kanavou T, Costaridou L. Assessing heterogeneity of lesion enhancement kinetics in dynamic contrast-enhanced MRI for breast cancer diagnosis. Br J Radiol 2010;83:296-309. [Crossref] [PubMed]
- Pinker K, Helbich TH, Morris EA. The potential of multiparametric MRI of the breast. Br J Radiol 2017;90:20160715. [Crossref] [PubMed]
- Mazurowski MA, Zhang J, Grimm LJ, Yoon SC, Silber JI. Radiogenomic analysis of breast cancer: luminal B molecular subtype is associated with enhancement dynamics at MR imaging. Radiology 2014;273:365-72. [Crossref] [PubMed]
- Yamaguchi K, Abe H, Newstead GM, Egashira R, Nakazono T, Imaizumi T, Irie H. Intratumoral heterogeneity of the distribution of kinetic parameters in breast cancer: comparison based on the molecular subtypes of invasive breast cancer. Breast Cancer 2015;22:496-502. [Crossref] [PubMed]
- Sutton EJ, Dashevsky BZ, Oh JH, Veeraraghavan H, Apte AP, Thakur SB, Morris EA, Deasy JO. Breast cancer molecular subtype classifier that incorporates MRI features. J Magn Reson Imaging 2016;44:122-9. [Crossref] [PubMed]
- Lafcı O, Celepli P, Seher Öztekin P, Koşar PN. DCE-MRI Radiomics Analysis in Differentiating Luminal A and Luminal B Breast Cancer Molecular Subtypes. Acad Radiol 2023;30:22-9. [Crossref] [PubMed]
- Zhong S, Wang F, Wang Z, Zhou M, Li C, Yin J. Multiregional Radiomic Signatures Based on Functional Parametric Maps from DCE-MRI for Preoperative Identification of Estrogen Receptor and Progesterone Receptor Status in Breast Cancer. Diagnostics (Basel) 2022.
- Monti S, Aiello M, Incoronato M, Grimaldi AM, Moscarino M, Mirabelli P, Ferbo U, Cavaliere C, Salvatore M. DCE-MRI Pharmacokinetic-Based Phenotyping of Invasive Ductal Carcinoma: A Radiomic Study for Prediction of Histological Outcomes. Contrast Media Mol Imaging 2018;2018:5076269. [Crossref] [PubMed]
- Chaudhury B, Zhou M, Goldgof DB, Hall LO, Gatenby RA, Gillies RJ, Patel BK, Weinfurtner RJ, Drukteinis JS. Heterogeneity in intratumoral regions with rapid gadolinium washout correlates with estrogen receptor status and nodal metastasis. J Magn Reson Imaging 2015;42:1421-30. [Crossref] [PubMed]
- Marusyk A, Polyak K. Tumor heterogeneity: causes and consequences. Biochim Biophys Acta 2010;1805:105-17. [Crossref] [PubMed]
- Carvalho ED, Filho AOC, Silva RRV, Araújo FHD, Diniz JOB, Silva AC, Paiva AC, Gattass M. Breast cancer diagnosis from histopathological images using textural features and CBIR. Artif Intell Med 2020;105:101845. [Crossref] [PubMed]
- Wu J, Gong G, Cui Y, Li R. Intratumor partitioning and texture analysis of dynamic contrast-enhanced (DCE)-MRI identifies relevant tumor subregions to predict pathological response of breast cancer to neoadjuvant chemotherapy. J Magn Reson Imaging 2016;44:1107-15. [Crossref] [PubMed]
- Braman NM, Etesami M, Prasanna P, Dubchuk C, Gilmore H, Tiwari P, Plecha D, Madabhushi A. Intratumoral and peritumoral radiomics for the pretreatment prediction of pathological complete response to neoadjuvant chemotherapy based on breast DCE-MRI. Breast Cancer Res 2017;19:57. [Crossref] [PubMed]
- Zhang B, Song L, Yin J. Texture Analysis of DCE-MRI Intratumoral Subregions to Identify Benign and Malignant Breast Tumors. Front Oncol 2021;11:688182. [Crossref] [PubMed]
- Lu H, Yin J. Texture Analysis of Breast DCE-MRI Based on Intratumoral Subregions for Predicting HER2 2+ Status. Front Oncol 2020;10:543.
- Fan M, Cheng H, Zhang P, Gao X, Zhang J, Shao G, Li L. DCE-MRI texture analysis with tumor subregion partitioning for predicting Ki-67 status of estrogen receptor-positive breast cancers. J Magn Reson Imaging 2018;48:237-47. [Crossref] [PubMed]
- Ashraf A, Gaonkar B, Mies C, DeMichele A, Rosen M, Davatzikos C, Kontos D. Breast DCE-MRI Kinetic Heterogeneity Tumor Markers: Preliminary Associations With Neoadjuvant Chemotherapy Response. Transl Oncol 2015;8:154-62. [Crossref] [PubMed]
- Gu J, Polley EC, Denis M, Carter JM, Pruthi S, Gregory AV, Boughey JC, Fazzio RT, Fatemi M, Alizad A. Early assessment of shear wave elastography parameters foresees the response to neoadjuvant chemotherapy in patients with invasive breast cancer. Breast Cancer Res 2021;23:52. [Crossref] [PubMed]
- Lee SH, Kim JH, Cho N, Park JS, Yang Z, Jung YS, Moon WK. Multilevel analysis of spatiotemporal association features for differentiation of tumor enhancement patterns in breast DCE-MRI. Med Phys 2010;37:3940-56. [Crossref] [PubMed]
- Fan M, Li H, Wang S, Zheng B, Zhang J, Li L. Radiomic analysis reveals DCE-MRI features for prediction of molecular subtypes of breast cancer. PLoS One 2017;12:e0171683. [Crossref] [PubMed]
- Xue C, Yuan J, Lo GG, Chang ATY, Poon DMC, Wong OL, Zhou Y, Chu WCW. Radiomics feature reliability assessed by intraclass correlation coefficient: a systematic review. Quant Imaging Med Surg 2021;11:4431-60. [Crossref] [PubMed]
- Sauerbrei W, Royston P, Binder H. Selection of important variables and determination of functional form for continuous predictors in multivariable model building. Stat Med 2007;26:5512-28. [Crossref] [PubMed]
- Cai J, Zheng J, Shen J, Yuan Z, Xie M, Gao M, Tan H, Liang Z, Rong X, Li Y, Li H, Jiang J, Zhao H, Argyriou AA, Chua MLK, Tang Y. A Radiomics Model for Predicting the Response to Bevacizumab in Brain Necrosis after Radiotherapy. Clin Cancer Res 2020;26:5438-47. [Crossref] [PubMed]
- Coutant C, Olivier C, Lambaudie E, Fondrinier E, Marchal F, Guillemin F, Seince N, Thomas V, Levêque J, Barranger E, Darai E, Uzan S, Houvenaeghel G, Rouzier R. Comparison of models to predict nonsentinel lymph node status in breast cancer patients with metastatic sentinel lymph nodes: a prospective multicenter study. J Clin Oncol 2009;27:2800-8. [Crossref] [PubMed]
- Vickers AJ, Cronin AM, Elkin EB, Gonen M. Extensions to decision curve analysis, a novel method for evaluating diagnostic tests, prediction models and molecular markers. BMC Med Inform Decis Mak 2008;8:53. [Crossref] [PubMed]
- Ruopp MD, Perkins NJ, Whitcomb BW, Schisterman EF. Youden Index and optimal cut-point estimated from observations affected by a lower limit of detection. Biom J 2008;50:419-30. [Crossref] [PubMed]
- Zhou Y, Xu J, Liu Q, Li C, Liu Z, Wang M, Zheng H, Wang S. A Radiomics Approach With CNN for Shear-Wave Elastography Breast Tumor Classification. IEEE Trans Biomed Eng 2018;65:1935-42. [Crossref] [PubMed]
- DeLong ER, DeLong DM, Clarke-Pearson DL. Comparing the areas under two or more correlated receiver operating characteristic curves: a nonparametric approach. Biometrics 1988;44:837-45.
- Sun R, Hou X, Li X, Xie Y, Nie S. Transfer Learning Strategy Based on Unsupervised Learning and Ensemble Learning for Breast Cancer Molecular Subtype Prediction Using Dynamic Contrast-Enhanced MRI. J Magn Reson Imaging 2022;55:1518-34. [Crossref] [PubMed]
- Chou SS, Gombos EC, Chikarmane SA, Giess CS, Jayender J. Computer-aided heterogeneity analysis in breast MR imaging assessment of ductal carcinoma in situ: Correlating histologic grade and receptor status. J Magn Reson Imaging 2017;46:1748-59. [Crossref] [PubMed]
- Gerlinger M, Rowan AJ, Horswell S, Math M, Larkin J, Endesfelder D, et al. Intratumor heterogeneity and branched evolution revealed by multiregion sequencing. N Engl J Med 2012;366:883-92. [Crossref] [PubMed]
- Mahrooghy M, Ashraf AB, Daye D, Mies C, Feldman M, Rosen M, Kontos D. Heterogeneity wavelet kinetics from DCE-MRI for classifying gene expression based breast cancer recurrence risk. Med Image Comput Comput Assist Interv 2013;16:295-302.
- Li L, Wang K, Sun X, Wang K, Sun Y, Zhang G, Shen B. Parameters of dynamic contrast-enhanced MRI as imaging markers for angiogenesis and proliferation in human breast cancer. Med Sci Monit 2015;21:376-82. [Crossref] [PubMed]
- Bedard PL, Hansen AR, Ratain MJ, Siu LL. Tumour heterogeneity in the clinic. Nature 2013;501:355-64. [Crossref] [PubMed]
- Guo Y, Hu Y, Qiao M, Wang Y, Yu J, Li J, Chang C. Radiomics Analysis on Ultrasound for Prediction of Biologic Behavior in Breast Invasive Ductal Carcinoma. Clin Breast Cancer 2018;18:e335-44.
- Grossmann P, Stringfield O, El-Hachem N, Bui MM, Rios Velazquez E, Parmar C, Leijenaar RT, Haibe-Kains B, Lambin P, Gillies RJ, Aerts HJ. Defining the biological basis of radiomic phenotypes in lung cancer. Elife 2017;6:e23421. [Crossref] [PubMed]
- Tran B, Dancey JE, Kamel-Reid S, McPherson JD, Bedard PL, Brown AM, Zhang T, Shaw P, Onetto N, Stein L, Hudson TJ, Neel BG, Siu LL. Cancer genomics: technology, discovery, and translation. J Clin Oncol 2012;30:647-60. [Crossref] [PubMed]
- Kuo MD, Gollub J, Sirlin CB, Ooi C, Chen X. Radiogenomic analysis to identify imaging phenotypes associated with drug response gene expression programs in hepatocellular carcinoma. J Vasc Interv Radiol 2007;18:821-31. [Crossref] [PubMed]
- Rutman AM, Kuo MD. Radiogenomics: creating a link between molecular diagnostics and diagnostic imaging. Eur J Radiol 2009;70:232-41. [Crossref] [PubMed]
- Zardavas D, Irrthum A, Swanton C, Piccart M. Clinical management of breast cancer heterogeneity. Nat Rev Clin Oncol 2015;12:381-94. [Crossref] [PubMed]
- Orlando L, Viale G, Bria E, Lutrino ES, Sperduti I, Carbognin L, Schiavone P, Quaranta A, Fedele P, Caliolo C, Calvani N, Criscuolo M, Cinieri S. Discordance in pathology report after central pathology review: Implications for breast cancer adjuvant treatment. Breast 2016;30:151-5. [Crossref] [PubMed]
- Luo WQ, Huang QX, Huang XW, Hu HT, Zeng FQ, Wang W. Predicting Breast Cancer in Breast Imaging Reporting and Data System (BI-RADS) Ultrasound Category 4 or 5 Lesions: A Nomogram Combining Radiomics and BI-RADS. Sci Rep 2019;9:11921. [Crossref] [PubMed]
- Yu Y, Tan Y, Xie C, Hu Q, Ouyang J, Chen Y, et al. Development and Validation of a Preoperative Magnetic Resonance Imaging Radiomics-Based Signature to Predict Axillary Lymph Node Metastasis and Disease-Free Survival in Patients With Early-Stage Breast Cancer. JAMA Netw Open 2020;3:e2028086. [Crossref] [PubMed]
- Wang X, Xie T, Luo J, Zhou Z, Yu X, Guo X. Radiomics predicts the prognosis of patients with locally advanced breast cancer by reflecting the heterogeneity of tumor cells and the tumor microenvironment. Breast Cancer Res 2022;24:20. [Crossref] [PubMed]
- Xie Y, Chen F, Li H, Wu Y, Fu H, Zhong Q, Chen J, Wang X. Development and validation of a clinical-radiomics nomogram for predicting a poor outcome and 30-day mortality after a spontaneous intracerebral hemorrhage. Quant Imaging Med Surg 2022;12:4900-13. [Crossref] [PubMed]
- Li C, Yin J. Radiomics Nomogram Based on Radiomics Score from Multiregional Diffusion-Weighted MRI and Clinical Factors for Evaluating HER-2 2+ Status of Breast Cancer. Diagnostics (Basel) 2021.
- Nie P, Yang G, Wang Z, Yan L, Miao W, Hao D, Wu J, Zhao Y, Gong A, Cui J, Jia Y, Niu H. A CT-based radiomics nomogram for differentiation of renal angiomyolipoma without visible fat from homogeneous clear cell renal cell carcinoma. Eur Radiol 2020;30:1274-84. [Crossref] [PubMed]
- Tang TY, Li X, Zhang Q, Guo CX, Zhang XZ, Lao MY, Shen YN, Xiao WB, Ying SH, Sun K, Yu RS, Gao SL, Que RS, Chen W, Huang DB, Pang PP, Bai XL, Liang TB. Development of a Novel Multiparametric MRI Radiomic Nomogram for Preoperative Evaluation of Early Recurrence in Resectable Pancreatic Cancer. J Magn Reson Imaging 2020;52:231-45. [Crossref] [PubMed]
- Zhou J, Luo LY, Dou Q, Chen H, Chen C, Li GJ, Jiang ZF, Heng PA. Weakly supervised 3D deep learning for breast cancer classification and localization of the lesions in MR images. J Magn Reson Imaging 2019;50:1144-51. [Crossref] [PubMed]