Development trends and knowledge framework in the application of magnetic resonance imaging in prostate cancer: a bibliometric analysis from 1984 to 2022
Introduction
According to the International Agency for Research on Cancer (IARC) Global Cancer Statistics 2020, prostate cancer (PCa) is the most common tumor of the male genitourinary system. It is the 2nd most frequently diagnosed cancer and the 5th most common cause of cancer death among men worldwide (1). PCa is characterized by insidious onset and asymptomatic or minor symptoms in the early stage, causing delayed diagnosis and loss of optimal treatment window (2). Thus, it is extremely important to accurately identify PCa and benign prostatic hyperplasia with early diagnosis. Furthermore, accurate staging of PCa to guide the choice of treatment modality and prevent undertreatment and overtreatment is of great significance in improving the quality of life of patients and extending their survival time (3).
Imaging is considered indispensable in the clinical management of PCa, and the improved soft tissue contrast of magnetic resonance imaging (MRI) compared with ultrasonography and computed tomography shows unique advantages (4). At present, MRI has played an increasingly important role in the diagnosis and management of PCa (5). MRI is highly sensitive in detecting PCa and can accurately localize lesions (6). The introduction of the Prostate Imaging-Reporting and Data System (PI-RADS) guidelines has improved PCa detection and the accuracy of its imaging staging (7). Meanwhile, the therapeutic ratio could be significantly improved by incorporating MRI into prostate brachytherapy due to its ability to differentiate benign and malignant PCa (8). Machine learning (ML) methods based on MRI scans are used for the diagnosis and discrimination of benign and malignant lesions, and most studies have achieved good results (9,10). Furthermore, it has been reported that MRI can be used to predict the recovery situation and biochemical recurrence (11,12).
Bibliometric analysis is an approach that is used to evaluate published scientific articles in a research field. It uses bibliometric tools to quantitatively analyze and visualize the literature data and measure characteristics, to understand the characteristics, trends, and information utilization of scientific articles (13,14). Meanwhile, by using this method, we can also compare the research status of various countries, institutions, authors, or journals to better understand the different areas of research (15). Therefore, the present study sought to summarize the application and development of MRI in PCa by analyzing relevant studies from 1984 to 2022, through a bibliometric approach. We hope to construct a knowledge framework to present current research hotspots and emerging trends in the field, which can help new researchers to better choose their future research directions.
Methods
Data collection
The Science Citation Index Expanded (SCIE) of the Web of Science Core Collection (WoSCC) database was chosen as the data source. It is the most frequently used and acceptable database for researchers in a variety of fields (16).
All the literature about MRI in PCa published in WoSCC between January 1, 1984, and June 30, 2022 was retrieved on November 6, 2022. The following keywords were used in the search: “prostate cancer” and “magnetic resonance imaging”. Detailed search terms are provided in Appendix 1. The language was limited to English, and the document types were limited to original articles and reviews. Irrelevant documents were excluded. Raw data were downloaded from WoSCC in full-record, plaintext format. The literature feature clustering included titles, keywords, abstracts, authors, and institutions (17). In this study, the exclusion of the articles was based on the WoSCC. The process of inclusion and exclusion is shown in Figure 1. The detailed record of the exclusion process is provided in Appendix 2. Finally, 10,230 articles were included in the present study.
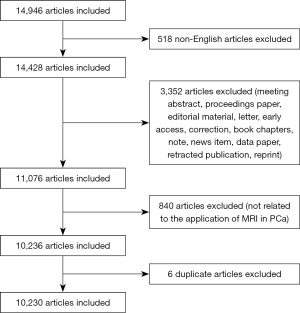
Data analysis and visualization
Microsoft Excel 2016 (Microsoft, Redmond, WA, USA) and the Bibliometrix Biblioshiny R-package software (https://bibliometrix.org/biblioshiny/biblioshiny1.html) were used to export statistical charts and tables of top-cited or productive authors, countries, journals, and institutions, and display the trend of the number of articles published by year (18). CiteSpace (version 6.1.R3; Chaomei Chen, Drexel University, Philadelphia, PA, USA) was used to perform a co-occurrence analysis and visualize the collaboration networks of the authors, institutions, countries, references, and keywords, as well as author co-citation analysis (ACA) and reference co-citation analysis (RCA).
ACA was used to establish the closeness of scholarly relationships among core authors to identify and analyze the scientific community within the disciplinary presentation area. RCA was performed to provide an informative snapshot of the network of articles and the professional domain that numerous co-cited articles converge in (19). The modularity value (Q value) and the mean silhouette value (S value) were also used to evaluate the results of clustering and the rationality of clustering, respectively. The Q value is the clustering module value, and Q>0.3 means that the clustering result is significant. The S value is the average contour value of clustering, and S>0.5 means that the clustering is reasonable (20).
The duplication time and annual growth rate can be used as indicators of scientific literature productivity. Duplication time refers to the amount of time it takes for the output of a subject to double. The annual growth rate refers to the percentage increase compared to the previous year. The equation to calculate the doubling time (D) is expressed by the following equation:
where b is the constant that relates the growth rate with the already acquired size of the discipline. The annual growth rate can be calculated using the following equation:
Lotka’s law, also known as the inverse square law of scientific productivity, can be used to express the frequency distribution of scientific productivity in terms of the number of published articles and is widely used to assess the productivity of authors (21). It suggests that there are more authors who publish fewer papers than those who publish many papers. In mathematics, it is expressed mathematically using the following equation:
According to Lotka’s index, authors are classified into three levels of productivity: small producers (those who publish 1 article); medium-sized producers (those who publish 2–9 articles); and large-scale producers (those who publish 10 or more articles).
Results
Trend of global publications
A total of 10,230 articles were included in subsequent analyses. The number of articles published each year and the cumulative number of articles published are shown in Figure 2, which shows a consistent overall upward trend in the number of annual publications from 1984 to 2022; the fastest annual growth was in 2017, with an increase of 123 articles. To calculate D, the statistical graph is adjusted to fit an exponential curve, which follows the following equation: with a correlation coefficient of 0.9315. This production corresponds to 38 years and a D of 3.13 years.
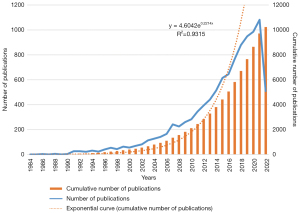
Analysis of authors and co-cited authors
A total of 34,233 authors were included in this study, of which 3,256 authors had no less than 5 articles. Table 1 displays the stratification of authors based on their productivity index grouping. The largest group is composed of authors who have published 1 paper, with a high index of transience (occasional authors) of 69.51. Meanwhile, large producers who have published more than 10 papers make up the smallest part of the group, comprising only 3.45%. Table 2 summarizes the 20 most productive authors and the top 20 co-cited authors with the highest citations. Most of these authors were from the USA. Among them, Turkbey, Emberton, and Choyke were the top 3 productive authors, with 239, 182, and 181 articles, respectively. Figure 3A shows the co-authorship among these representative authors. Co-authorship analysis is an important form to reflect the degree of communication among authors in a given field (22).
Table 1
No. of authors | % | |
---|---|---|
PI ≥1 (10 or more articles) | 1,183 | 3.45 |
0< PI <1 (2–9 articles) | 10,488 | 30.64 |
PI =0 (1 article) | 22,562 | 65.91 |
Total | 34,233 | 100 |
PI, participation index.
Table 2
Rank | Author | Co-cited author | |||||
---|---|---|---|---|---|---|---|
Name | Count | Country | Name | Count | Country | ||
1 | Turkbey | 239 | USA | Barentsz | 10,318 | Netherlands | |
2 | Emberton | 182 | UK | Choyke | 8,626 | USA | |
3 | Choyke | 181 | USA | Turkbey | 8,522 | USA | |
4 | Pinto | 167 | USA | Hricak | 7,514 | USA | |
5 | Hricak | 153 | USA | Emberton | 6,697 | UK | |
6 | Kurhanewicz | 148 | USA | Futterer | 6,300 | Netherlands | |
7 | Ahmed | 142 | UK | Pinto | 6,121 | USA | |
8 | Barentsz | 137 | Netherlands | Kurhanewicz | 5,630 | USA | |
9 | Wood | 128 | USA | Wood | 4,994 | USA | |
10 | Rosenkrantz | 123 | USA | Taneja | 4,806 | USA | |
11 | Futterer | 102 | Netherlands | Rosenkrantz | 4,532 | USA | |
12 | Taneja | 100 | USA | Ahmed | 4,463 | UK | |
13 | Moore | 99 | UK | Merino | 4,264 | USA | |
14 | Freeman | 92 | UK | Villers | 4,223 | France | |
15 | Merino | 89 | USA | Haider | 4,188 | USA | |
16 | Punwani | 88 | USA | Rouviere | 4,135 | France | |
17 | Haider | 87 | USA | Verma | 3,907 | Canada | |
18 | Oto | 87 | USA | Freeman | 3,553 | UK | |
19 | Schlemmer | 86 | Germany | Witjes | 3,491 | Netherlands | |
20 | Vigneron | 81 | USA | Vigneron | 3,394 | USA |
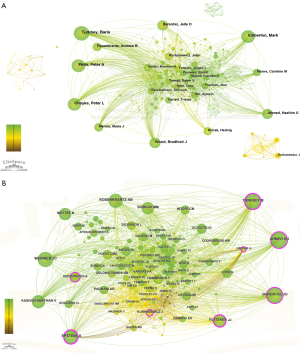
A total of 23,086 citations were recorded in this study. Barentsz, Choyke, and Turkbey were the top 3 most cited authors, with 10,318, 8,626, and 8,522 citations, respectively. ACA uses authors as the units of analysis and the co-citations of pairs of authors as the variable that indicates their “distances” from each other (23). The co-citation relationship of the authors in this study is shown in Figure 3B.
Analysis of countries and institutions
A total of 64 countries published related articles in this field. Table 3 shows the top 20 countries and the number of published articles under the classification of corresponding authors. The USA ranked first with 3,362 articles, accounting for 32.84% of the total number of articles published, followed by China (830, 8.11%), Germany (754, 7.37%), and the UK (737, 7.20%). Multiple country publication (MCP) indicates the number of co-authored papers with authors from other countries, and single country publication (SCP) indicates the number of co-authored papers by authors of the same nationality. Both of MCP and SCP were used to evaluate the degree of international cooperation (24). In addition, 6,144 institutions published articles in this field; Table 4 and Figure 4 summarize the top 20 institutions and their partnerships. Memorial Sloan Kettering Cancer Center published the most with 791 articles, accounting for 2.03%, followed by the University of California San Francisco (599, 1.53%), University of Toronto (498, 1.28%), and University of California Los Angeles (451, 1.16%).
Table 3
Rank | Country | Count | Percentage | SCP | MCP |
---|---|---|---|---|---|
1 | USA | 3,362 | 32.84 | 2,669 | 693 |
2 | China | 830 | 8.11 | 701 | 129 |
3 | Germany | 754 | 7.37 | 562 | 192 |
4 | UK | 737 | 7.20 | 500 | 237 |
5 | Italy | 560 | 5.47 | 416 | 144 |
6 | Netherlands | 535 | 5.23 | 365 | 170 |
7 | Canada | 486 | 4.75 | 349 | 137 |
8 | Japan | 427 | 4.17 | 395 | 32 |
9 | France | 371 | 3.62 | 277 | 94 |
10 | Korea | 316 | 3.09 | 289 | 27 |
11 | Australia | 245 | 2.39 | 163 | 82 |
12 | Switzerland | 166 | 1.62 | 101 | 65 |
13 | Belgium | 131 | 1.28 | 81 | 50 |
14 | Turkey | 113 | 1.10 | 106 | 7 |
15 | Denmark | 111 | 1.08 | 77 | 34 |
16 | Spain | 108 | 1.06 | 76 | 32 |
17 | Sweden | 99 | 0.97 | 70 | 29 |
18 | Austria | 94 | 0.92 | 39 | 55 |
19 | Norway | 94 | 0.92 | 58 | 36 |
20 | India | 89 | 0.87 | 75 | 14 |
SCP: the number of co-authored papers by authors of the same nationality; MCP: the number of co-authored papers with authors from other countries. SCP, single country publication; MCP, multiple country publication.
Table 4
Rank | Institution | Countries/regions | Articles | Percentage |
---|---|---|---|---|
1 | Memorial Sloan Kettering Cancer Center | USA | 791 | 2.03 |
2 | University of California San Francisco | USA | 599 | 1.53 |
3 | University of Toronto | Canada | 498 | 1.28 |
4 | University of California, Los Angeles | USA | 451 | 1.16 |
5 | Radboud University Nijmegen | Netherlands | 420 | 1.08 |
6 | The University of Texas MD Anderson Cancer Center | USA | 331 | 0.85 |
7 | The Johns Hopkins University | USA | 317 | 0.81 |
8 | German Cancer Research Center | Germany | 302 | 0.77 |
9 | Harvard University | USA | 275 | 0.70 |
10 | University of Michigan | USA | 267 | 0.68 |
11 | Stanford University | USA | 262 | 0.67 |
12 | The Institute of Cancer Research | UK | 243 | 0.62 |
13 | The University of Chicago | USA | 235 | 0.60 |
14 | University College London Hospitals NHS Foundation Trust | UK | 226 | 0.58 |
15 | Case Western Reserve University | USA | 219 | 0.56 |
16 | Duke University | USA | 211 | 0.54 |
17 | Seoul National University | Korea | 210 | 0.54 |
18 | University of Cambridge | UK | 208 | 0.53 |
19 | Universitair Medisch Centrum Utrecht | Netherlands | 200 | 0.51 |
20 | Division of Surgery & Interventional Science | UK | 198 | 0.51 |
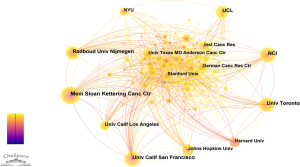
Analysis of journals
A total of 795 journals published related articles in this field, of which 264 journals had no less than 5 articles. The top 20 most prolific journals are listed in Table 5. Of the top 10 journals, 5 were from the United States (USA), 2 from the UK, 2 from the Netherlands, and 1 from Germany. Journal of Magnetic Resonance Imaging [impact factor (IF) 2021: 5.119] published the most (333 publications, 3.26%), followed by the Journal of Urology (IF 2021: 7.641, 308 publications, 3.01%), Radiology (IF 2021: 29.146, 260 publications, 2.54%), and American Journal of Roentgenology (IF 2021: 6.582, 256 publications, 2.51%). H-index is a mixed index that is used as a significant indicator of appraising both the number and level of academic output of a scientific researcher, country, journal, or institution (25). The journal with the highest IF was Radiology.
Table 5
Journal | Country | Number | Percentage | IF 2021 | H-index |
---|---|---|---|---|---|
Journal of Magnetic Resonance Imaging | USA | 333 | 3.26 | 5.119 | 60 |
Journal of Urology | Netherlands | 308 | 3.01 | 7.641 | 74 |
Radiology | USA | 260 | 2.54 | 29.146 | 100 |
American Journal of Roentgenology | USA | 256 | 2.51 | 6.582 | 55 |
European Radiology | Germany | 253 | 2.48 | 7.034 | 56 |
International Journal of Radiation Oncology Biology Physics | Netherlands | 252 | 2.47 | 8.013 | 63 |
BJU International | UK | 248 | 2.43 | 5.969 | 55 |
Medical Physics | UK | 199 | 1.95 | 4.506 | 40 |
Urology | USA | 199 | 1.95 | 2.633 | 36 |
Magnetic Resonance in Medicine | USA | 189 | 1.85 | 3.737 | 44 |
World Journal of Urology | USA | 183 | 1.79 | 3.661 | 28 |
European Urology | Netherlands | 182 | 1.78 | 24.344 | 92 |
European Journal of Radiology | Netherlands | 171 | 1.67 | 4.531 | 39 |
Abdominal Radiology | USA | 168 | 1.64 | 2.886 | 17 |
Urologic Oncology-Seminars and Original Investigations | Netherlands | 166 | 1.62 | 2.954 | 23 |
Radiotherapy and Oncology | Netherlands | 142 | 1.39 | 6.901 | 43 |
British Journal of Radiology | UK | 141 | 1.38 | 3.629 | 26 |
NMR in Biomedicine | USA | 138 | 1.35 | 4.478 | 33 |
Physics in Medicine and Biology | UK | 137 | 1.34 | 4.174 | 36 |
Frontiers in Oncology | Switzerland | 130 | 1.27 | 5.738 | 15 |
IF: an internationally recognized journal evaluation index to evaluate the journals quality; H-index: a composite index can be used as an indicator to evaluate the academic output quantity and level of a journal. IF, impact factor.
Analysis of reference citations and co-citations
Table 6 lists the top 10 articles with the highest citation. Barentsz (26) had the highest total citation frequency with 1,283 citations, followed closely by Weinreb (27) with 1,277 citations.
Table 6
Title | Journals | Authors | Year | Citations |
---|---|---|---|---|
ESUR prostate MR guidelines 2012 | European Radiology | Barentsz et al. | 2012 | 1,283 |
PI-RADS prostate imaging-reporting and data system: 2015, version 2 | European Urology | Weinreb et al. | 2016 | 1,277 |
Diagnostic accuracy of multi-parametric MRI and TRUS biopsy in prostate cancer (PROMIS): a paired validating confirmatory study (PROMIS): a paired validating confirmatory study | Lancet | Ahmed et al. | 2017 | 1,021 |
MRI-targeted or standard biopsy for prostate-cancer diagnosis | The New England Journal of Medicine |
Kasivisvanathan et al. | 2018 | 842 |
Comparison of MR/ultrasound fusion-guided biopsy with ultrasound-guided biopsy for the diagnosis of prostate cancer | The Journal of the American Medical Association | Siddiqui et al. | 2015 | 712 |
EAU-ESTRO-SIOG guidelines on prostate cancer. Part 1: screening, diagnosis, and local treatment with curative intent | European Urology | Mottet et al. | 2017 | 519 |
Prostate Imaging Reporting and Data System version 2.1: 2019 update of Prostate Imaging Reporting and Data System version 2 | European Urology | Turkbey et al. | 2019 | 439 |
The 2014 International Society of Urological Pathology (ISUP) consensus conference on Gleason grading of prostatic carcinoma: definition of grading patterns and proposal for a new grading system | American Journal of Surgical Pathology |
Epstein et al. | 2016 | 429 |
Magnetic resonance imaging for the detection, localization, and characterization of prostate cancer: Recommendations from a European consensus meeting | European Urology | Dickinson et al. | 2011 | 389 |
Can clinically significant prostate cancer be detected with multiparametric magnetic resonance imaging? A systematic review of the literature | European Urology | Futterer et al. | 2015 | 385 |
ESUR, European Society of Urogenital Radiology; MR, magnetic resonance.
RCA uses reference as the element of analysis to reflect the relationship between the reference by analyzing patterns and trends in co-citation (19). Figure 5A visually represents the 11 main clusters of reference co-citation. From the analysis results, the Q value was 0.7647, and the S value was 0.9253, suggesting that the clustering effect and network homogeneity were reliable. Figure 5B shows the timeline view of the co-citation references, which reflects the evolution of research hotspots through time. “#6 radical retropubic prostatectomy” and “#5 prostate neoplasms” were the earliest research in this field. “#1 multiparametric magnetic resonance imaging”, “#8 psma”, “#10 radiomics”, “#4 pi-rads”, and “#active surveillance” are currently the latest research hotspots.
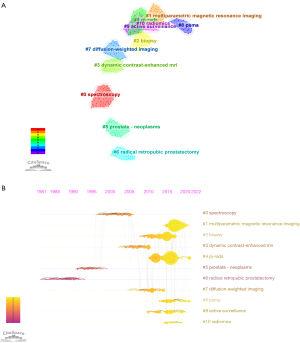
Analysis of keyword burst
Keyword burst analysis can display the changing trend of research topics over time, which may help scholars grasp the development of research hotspots (28). A total of 338 keywords were detected in the analysis of keywords burst. The top 50 keywords are presented in Figure 6, sorted by the initial year of the burst. Among them, “magnetic resonance spectroscopic” had the highest burst intensity, with a burst strength of 114.97. Meanwhile, “machine learning”, “psa density”, “multi parametric mri”, “deep learning”, and “artificial intelligence” appeared frequently in the past 3 years, with burst strengths of 27.01, 14.72, 47.25, 38.14, and 19.49, respectively.
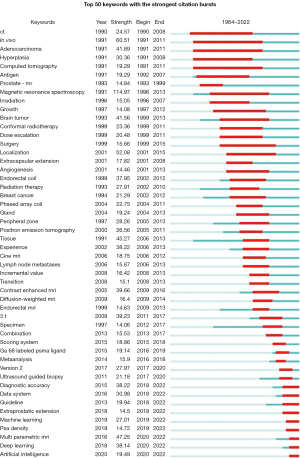
Discussion
The current study analyzed the use of MRI in PCa through bibliometrics and found an overall increasing trend in the number of articles published before June 2022, with a period of pronounced rapid growth after 2003. This rapid growth may be attributed to the emergence and development of multiple MRI-based technologies [such as intravoxel incoherent motion, diffusion kurtosis imaging, dynamic contrast-enhanced (DCE) MRI, etc.] over the past 2 decades. With the advent of the internet age, the increasing number of publications such as journals has led to a corresponding increase in related literature. It can be expected that the number of publications in this field will continue to increase yearly and remain at a high level over the next few years. Future research should also focus on the application of new technologies such as artificial intelligence (AI) and deep learning (DL) combined with MRI in PCa.
From 1984 to 2022, all the top 10 authors in terms of the number of publications published more than 100 articles, of which 8 appear in the list of the top 10 most cited authors. This indicates that articles published by high-producing authors receive more attention from researchers, which suggests that they may be of higher academic value. A total of 8 of the top 12 authors in terms of the number of publications and citations are from the USA, underscoring the significant influence of the USA in this field. Barentsz’s article published in European Radiology received the highest number of citations. It argued cogently that multiparametric MRI (mpMRI) should be an integral part of PCa diagnosis and treatment (26).
All the top 10 contributing countries or regions had a high proportion of SCP in the volume of publications, implying that all countries are primarily engaged in domestic co-authorship. Meanwhile, the USA also had the largest MCP, suggesting that it has strong ties with other countries and is their main collaborator in this field. Although China ranked second after the USA, with 830 publications, the MCP was only 129, and even lower than that of Canada with 486 publications. This indicates that although the number of papers in China is large, there is little collaboration with other countries. Furthermore, 7 of the top 10 institutions were from the USA, which explains why the USA is far more influential than any other country. The disparity between the USA and China may be explained by several factors, including the later start and slow early development in the early stage of MRI in China, few opportunities for communication with the international community, and the language barrier that may impact external collaboration. Therefore, China should actively maintain close cooperative relationships with the international community to increase its influence in this field.
The top 10 journals published 2,497 articles, accounting for 24.43% of the total number of publications. These journals have made a great contribution to the development of the field, indicating that more high-quality articles on the application of MRI in PCa will be published in these journals as a priority in the future. In addition, the Journal of Magnetic Resonance Imaging, Journal of Urology, and American Journal of Roentgenology were extremely prolific journals with great potential to publish high-quality articles to increase the IF in the future.
Co-citation clustering analysis revealed the core issue and developmental process of this field through the cluster analysis chart and the timeline view (29). The earliest studies were focused on “#6 radical retropubic prostatectomy” and “#5 prostate neoplasms”, whereas the current hot topics are “#1 multiparametric magnetic resonance imaging”, “#8 psma”, “#10 radiomics”, “#4 pi-rads”, and “#active surveillance”. In earlier studies, MRI was only used to determine the need for radical retropubic prostatectomy, and thus it was insufficient in achieving accurate preoperative staging of PCa owing to technical limitations (30). With the development of medical imaging technology, the applications of MRI in PCa have evolved into using rich imaging sequences, dynamic enhancement, and even AI to achieve an accurate evaluation of PCa. MpMRI refers to the combination of multiple MRI sequences required to make a diagnosis, it consists of both anatomic [T1-weighted (T1W) and T2-weighted (T2W) MRI] sequences as well as functional sequences, including diffusion-weighted imaging (DWI) MRI, DCE MRI, and magnetic resonance spectroscopy (MRS) (31-33). Meanwhile, the PI-RADS classification as an imaging assessment based on mpMRI can provide more detailed clinical information, leading to the refinement of the clinical evaluation of PCa (34). It has been continuously updated since its initial release by the European Society of Urogenital Radiology (ESUR) in 2012 (26), and the latest version, PI-RADS v2.1, was released in 2019 (27,35). Prostate-specific membrane antigen (PSMA) is a type II transmembrane glycoprotein that is overexpressed on PCa cells, and its expression increases with the tumor grade (36,37). Therefore, PSMA can be used as an excellently targeted probe to combine with MRI molecular contrast agent, advance the capability for early detection of primary and metastatic PCa, and track disease progression and treatment, thereby facilitating image-guided interventional therapy (38,39). A study pointed out that the introduction of imaging agents targeting PSMA has shown high diagnostic accuracy for primary staging and restaging during biochemical recurrence compared with conventional imaging (40). This has received little attention so far. Radiomics is a technique for extracting quantitative features from medical images, providing numerous imaging biomarkers for tumor detection, diagnosis, aggressiveness determination, staging, and prognosis, including for PCa (41,42). Ma et al. confirmed that radiomics combined with the ML classifier model has strong classification performance in identifying benign and PCa lesions in the PI-RADS 4/5 score (43). Furthermore, Zhang et al. showed that nomograms constructed based on radiomics features combined with total prostate-specific antigen (tPSA) levels improved the diagnostic accuracy of clinically significant PCa and reduced unnecessary biopsies (44). It also plays an important role in the treatment and prediction of prognosis in PCa. Shiradkar et al. presented a radiomics-assisted targeted treatment radiotherapy planning method to accurately localize malignant lesions to increase the biological effect of the tumor region while achieving a reduction in dosage to noncancerous tissue (45). Radiomics features predicted the risk of metastatic progression in high-risk PCa (clinical stage ≥ T2c, biopsy Gleason score ≥8, or PSA level >20 ng/mL), and classified high-risk patients into favorable and unfavorable prognostic groups (46). A recent study also found that the majority of low-risk (clinical stage ≤ T2a, biopsy Gleason score ≤6, and PSA level ≤10 ng/mL) were not at risk of death for PCa in a 10-year time frame (47). For these patients, active surveillance (AS) may be a better option than surgery (48). The two main roles of mpMRI in AS are to improve the selection of eligible patients at diagnosis and evaluate significant changes over serial scans that would indicate radiologic progression (49).
Keyword burst analysis can reflect the main research hotspots and the trend of research hotspots in a specific period (50). After 2018, the keywords gradually evolved to use new technology combined with MRI, and “machine learning”, “psa density”, “multi parametric mri”, “deep learning”, and “artificial intelligence” were the keywords with the highest burst strength. AI is a discipline that focuses on using a computer to simulate human intelligent behavior. Applications of medical-image diagnostic systems have expanded the frontiers of AI into the area of medical practice (51). AI has a wide range of applications in the diagnosis, staging, treatment, and prognosis of PCa. The main methods of AI include ML and DL. The ML technique is a representative method for exploring the risk factors or high-risk groups of disease by analyzing medical big data (52). It often combines with radiomics, and plays an important role in the accurate diagnosis and treatment of PCa by building various models (53-55). DL is an important branch of AI that uses networks of simple interconnected units to extract patterns from data to solve complex problems (56). Some researchers use DL methods to achieve highly accelerated MRI processes of the prostate and improved image quality through image reconstruction (57-59). Accurate detection and localization of PCa in men undergoing prostate MRI is the most extensive application of DL (60). DL models based on convolutional neural networks can be used to achieve the semi-automatic classification of PCa on mpMRI (61). Some studies are focused on methods using DL to achieve fully automatic segmentation of prostate lesions and gland through various algorithms (62,63). “PSA density” is a keyword with high emergence intensity. PSA density (relating the serum PSA to the volume of the prostate) is a better indicator than PSA for predicting PCa in patients with a PSA below 10 ng/mL (64,65). A combination of the PI-RADS v2 score and PSA density is considered an important management tool for low-risk PCa (66).
Nonetheless, this study has some limitations. First, all publications included in this study were downloaded from WoSCC; therefore, they may not represent the complete research field of MRI in PCa. Second, our study only included English literature, which may have led to language bias and the consequent omission of high-quality literature from other languages. Finally, it takes time for articles to reach a certain number of citations after publication, which may mean that high-quality articles published in recent years have not yet reached the level of citations commensurate with their quality and may have led to research bias.
Conclusions
In summary, MRI has a wide range of applications in the study of PCa, especially in adjuvant diagnosis and tumor staging. A large number of scholars have devoted their efforts to research related to the MRI of PCa. The USA remains the leader in this field with a large number of core authors and high-level institutions and will continue to lead in the future. Currently, the main research hotspot in this field is to provide an important reference for accurate diagnosis and treatment of PCa through DL, radiomics, and AI.
Acknowledgments
The authors would like to thank all study participants.
Funding: This study was supported by
Footnote
Conflicts of Interest: All authors have completed the ICMJE uniform disclosure form (available at https://qims.amegroups.com/article/view/10.21037/qims-23-446/coif). The authors have no conflicts of interest to declare.
Ethical Statement: The authors are accountable for all aspects of the work in ensuring that questions related to the accuracy or integrity of any part of the work are appropriately investigated and resolved.
Open Access Statement: This is an Open Access article distributed in accordance with the Creative Commons Attribution-NonCommercial-NoDerivs 4.0 International License (CC BY-NC-ND 4.0), which permits the non-commercial replication and distribution of the article with the strict proviso that no changes or edits are made and the original work is properly cited (including links to both the formal publication through the relevant DOI and the license). See: https://creativecommons.org/licenses/by-nc-nd/4.0/.
References
- Ferlay J, Colombet M, Soerjomataram I, Parkin DM, Piñeros M, Znaor A, Bray F. Cancer statistics for the year 2020: An overview. Int J Cancer 2021; Epub ahead of print. [Crossref]
- Ye X, Zhao L, Kang J. Expression and significance of PTEN and Claudin-3 in prostate cancer. Oncol Lett 2019;17:5628-34. [Crossref] [PubMed]
- Werneburg GT, Kongnyuy M, Halpern DM, Salcedo JM, Kosinski KE, Haas JA, Schiff JT, Corcoran AT, Katz AE. Patient-reported quality of life progression in men with prostate cancer following primary cryotherapy, cyberknife, or active holistic surveillance. Prostate Cancer Prostatic Dis 2018;21:355-63. [Crossref] [PubMed]
- Ginja M, Pires MJ, Gonzalo-Orden JM, Seixas F, Correia-Cardoso M, Ferreira R, Fardilha M, Oliveira PA, Faustino-Rocha AI. Anatomy and Imaging of Rat Prostate: Practical Monitoring in Experimental Cancer-Induced Protocols. Diagnostics (Basel) 2019;9:68. [Crossref] [PubMed]
- Mendhiratta N, Taneja SS, Rosenkrantz AB. The role of MRI in prostate cancer diagnosis and management. Future Oncol 2016;12:2431-43. [Crossref] [PubMed]
- Citak-Er F, Vural M, Acar O, Esen T, Onay A, Ozturk-Isik E. Final Gleason score prediction using discriminant analysis and support vector machine based on preoperative multiparametric MR imaging of prostate cancer at 3T. Biomed Res Int 2014;2014:690787. [Crossref] [PubMed]
- Nketiah GA, Elschot M, Scheenen TW, Maas MC, Bathen TF, Selnæs KM. PCa-MAP Consortium. Utility of T2-weighted MRI texture analysis in assessment of peripheral zone prostate cancer aggressiveness: a single-arm, multicenter study. Sci Rep 2021;11:2085. [Crossref] [PubMed]
- Dai X, Lei Y, Zhang Y, Qiu RLJ, Wang T, Dresser SA, Curran WJ, Patel P, Liu T, Yang X. Automatic multi-catheter detection using deeply supervised convolutional neural network in MRI-guided HDR prostate brachytherapy. Med Phys 2020;47:4115-24. [Crossref] [PubMed]
- Xu M, Fang M, Zou J, Yang S, Yu D, Zhong L, Hu C, Zang Y, Dong D, Tian J, Fang X. Using biparametric MRI radiomics signature to differentiate between benign and malignant prostate lesions. Eur J Radiol 2019;114:38-44. [Crossref] [PubMed]
- Ji X, Zhang J, Shi W, He D, Bao J, Wei X, Huang Y, Liu Y, Chen JC, Gao X, Tang Y, Xia W. Bi-parametric magnetic resonance imaging based radiomics for the identification of benign and malignant prostate lesions: cross-vendor validation. Phys Eng Sci Med 2021;44:745-54. [Crossref] [PubMed]
- Mobadersany P, Yousefi S, Amgad M, Gutman DA, Barnholtz-Sloan JS, Velázquez Vega JE, Brat DJ, Cooper LAD. Predicting cancer outcomes from histology and genomics using convolutional networks. Proc Natl Acad Sci U S A 2018;115:E2970-9. [Crossref] [PubMed]
- Shiradkar R, Ghose S, Jambor I, Taimen P, Ettala O, Purysko AS, Madabhushi A. Radiomic features from pretreatment biparametric MRI predict prostate cancer biochemical recurrence: Preliminary findings. J Magn Reson Imaging 2018;48:1626-36. [Crossref] [PubMed]
- Donthu N, Kumar S, Mukherjee D, Pandey N, Lim WM. How to conduct a bibliometric analysis: An overview and guidelines. J Bus Res. 2021;133:285-96.
- Hong SJ, Yoon DY, Cho YK, Yoon SJ, Moon JY, Baek S, Lim KJ. Characteristics and Quality of Radiologic Randomized Controlled Trials: A Bibliometric Analysis Between 1995 and 2014. AJR Am J Roentgenol 2016;206:917-23. [Crossref] [PubMed]
- Shen Z, Wu H, Chen Z, Hu J, Pan J, Kong J, Lin T. The Global Research of Artificial Intelligence on Prostate Cancer: A 22-Year Bibliometric Analysis. Front Oncol 2022;12:843735. [Crossref] [PubMed]
- Wei WT, Wei CK, Wu CC. Trends in Research about COVID-19 Vaccine Documented through Bibliometric and Visualization Analysis. Healthcare (Basel) 2022;10:1942. [Crossref] [PubMed]
- Yang DW, Wang XP, Wang ZC, Yang ZH, Bian XF. A scientometric analysis on hepatocellular carcinoma magnetic resonance imaging research from 2008 to 2017. Quant Imaging Med Surg 2019;9:465-76. [Crossref] [PubMed]
- Xiong F, Shen P, Li Z, Huang Z, Liang Y, Chen X, Li Y, Chai X, Feng Z, Li M. Bibliometric Analysis of Post-Stroke Pain Research Published from 2012 to 2021. J Pain Res 2023;16:1-20. [Crossref] [PubMed]
- Zheng J, Zhou R, Meng B, Li F, Liu H, Wu X. Knowledge framework and emerging trends in intracranial aneurysm magnetic resonance angiography: a scientometric analysis from 2004 to 2020. Quant Imaging Med Surg 2021;11:1854-69. [Crossref] [PubMed]
- Deng YJ, Liu SJ, Zhao M, Zhao F, Guo J, Huang YX. Research trends and hotspots of recurrent pregnancy loss with thrombophilia: a bibliometric analysis. BMC Pregnancy Childbirth 2022;22:944. [Crossref] [PubMed]
- Lotka AJ. The frequency distribution of scientific productivity. Journal of Washington Academy Sciences. 1926;16:317-23.
- Yin M, Xu C, Ma J, Ye J, Mo W. A Bibliometric Analysis and Visualization of Current Research Trends in the Treatment of Cervical Spondylotic Myelopathy. Global Spine J 2021;11:988-98. [Crossref] [PubMed]
- Andrews JE. An author co-citation analysis of medical informatics. J Med Libr Assoc 2003;91:47-56.
- Sweileh WM. Global research output in the health of international Arab migrants (1988-2017). BMC Public Health 2018;18:755. [Crossref] [PubMed]
- Wang M, Liu P, Zhang R, Li Z, Li X. A Scientometric Analysis of Global Health Research. Int J Environ Res Public Health 2020;17:2963. [Crossref] [PubMed]
- Barentsz JO, Richenberg J, Clements R, Choyke P, Verma S, Villeirs G, Rouviere O, Logager V, Fütterer JJEuropean Society of Urogenital Radiology. ESUR prostate MR guidelines 2012. Eur Radiol 2012;22:746-57. [Crossref] [PubMed]
- Weinreb JC, Barentsz JO, Choyke PL, Cornud F, Haider MA, Macura KJ, Margolis D, Schnall MD, Shtern F, Tempany CM, Thoeny HC, Verma S. PI-RADS Prostate Imaging - Reporting and Data System: 2015, Version 2. Eur Urol 2016;69:16-40. [Crossref] [PubMed]
- Meng F, Deng S, Wang L, Zhou Y, Zhao M, Li H, Liu D, Gao G, Liao X, Wang J. Bibliometric analysis and visualization of literature on assisted reproduction technology. Front Med (Lausanne) 2022;9:1063040. [Crossref] [PubMed]
- Liu Y, Li Q, Li W, Zhang Y, Pei X. Progress in urban resilience research and hotspot analysis: a global scientometric visualization analysis using CiteSpace. Environ Sci Pollut Res Int 2022;29:63674-91. [Crossref] [PubMed]
- McSherry SA, Levy F, Schiebler ML, Keefe B, Dent GA, Mohler JL. Preoperative prediction of pathological tumor volume and stage in clinically localized prostate cancer: comparison of digital rectal examination, transrectal ultrasonography and magnetic resonance imaging. J Urol 1991;146:85-9. [Crossref] [PubMed]
- Li X, Li C, Chen M. Patients With "Gray Zone" PSA Levels: Application of Prostate MRI and MRS in the Diagnosis of Prostate Cancer. J Magn Reson Imaging 2023;57:992-1010. [Crossref] [PubMed]
- Turkbey B, Brown AM, Sankineni S, Wood BJ, Pinto PA, Choyke PL. Multiparametric prostate magnetic resonance imaging in the evaluation of prostate cancer. CA Cancer J Clin 2016;66:326-36. [Crossref] [PubMed]
- Zhang VY, Westphalen A, Delos Santos L, Tabatabai ZL, Shinohara K, Vigneron DB, Kurhanewicz J. The role of metabolic imaging in radiation therapy of prostate cancer. NMR Biomed 2014;27:100-11. [Crossref] [PubMed]
- Gupta RT, Mehta KA, Turkbey B, Verma S. PI-RADS: Past, present, and future. J Magn Reson Imaging 2020;52:33-53. [Crossref] [PubMed]
- Turkbey B, Rosenkrantz AB, Haider MA, Padhani AR, Villeirs G, Macura KJ, Tempany CM, Choyke PL, Cornud F, Margolis DJ, Thoeny HC, Verma S, Barentsz J, Weinreb JC. Prostate Imaging Reporting and Data System Version 2.1: 2019 Update of Prostate Imaging Reporting and Data System Version 2. Eur Urol 2019;76:340-51. [Crossref] [PubMed]
- Jeitner TM, Babich JW, Kelly JM. Advances in PSMA theranostics. Transl Oncol 2022;22:101450. [Crossref] [PubMed]
- Plichta KA, Graves SA, Buatti JM. Prostate-Specific Membrane Antigen (PSMA) Theranostics for Treatment of Oligometastatic Prostate Cancer. Int J Mol Sci 2021;22:12095. [Crossref] [PubMed]
- Pu F, Salarian M, Xue S, Qiao J, Feng J, Tan S, Patel A, Li X, Mamouni K, Hekmatyar K, Zou J, Wu D, Yang JJ. Prostate-specific membrane antigen targeted protein contrast agents for molecular imaging of prostate cancer by MRI. Nanoscale 2016;8:12668-82. [Crossref] [PubMed]
- Zhu Y, Sun Y, Chen Y, Liu W, Jiang J, Guan W, Zhang Z, Duan Y. In Vivo Molecular MRI Imaging of Prostate Cancer by Targeting PSMA with Polypeptide-Labeled Superparamagnetic Iron Oxide Nanoparticles. Int J Mol Sci 2015;16:9573-87. [Crossref] [PubMed]
- Mihatsch PW, Beissert M, Pomper MG, Bley TA, Seitz AK, Kübler H, Buck AK, Rowe SP, Serfling SE, Hartrampf PE, Werner RA. Changing Threshold-Based Segmentation Has No Relevant Impact on Semi-Quantification in the Context of Structured Reporting for PSMA-PET/CT. Cancers (Basel) 2022;14:270. [Crossref] [PubMed]
- Gillies RJ, Kinahan PE, Hricak H. Radiomics: Images Are More than Pictures, They Are Data. Radiology 2016;278:563-77. [Crossref] [PubMed]
- Mayerhoefer ME, Materka A, Langs G, Häggström I, Szczypiński P, Gibbs P, Cook G. Introduction to Radiomics. J Nucl Med 2020;61:488-95. [Crossref] [PubMed]
- Ma L, Zhou Q, Yin H, Ang X, Li Y, Xie G, Li G. Texture analysis based on PI-RADS 4/5-scored magnetic resonance images combined with machine learning to distinguish benign lesions from prostate cancer. Transl Cancer Res 2022;11:1146-61. [Crossref] [PubMed]
- Zhang H, Li X, Zhang Y, Huang C, Wang Y, Yang P, Duan S, Mao N, Xie H. Diagnostic nomogram based on intralesional and perilesional radiomics features and clinical factors of clinically significant prostate cancer. J Magn Reson Imaging 2021;53:1550-8. [Crossref] [PubMed]
- Shiradkar R, Podder TK, Algohary A, Viswanath S, Ellis RJ, Madabhushi A. Radiomics based targeted radiotherapy planning (Rad-TRaP): a computational framework for prostate cancer treatment planning with MRI. Radiat Oncol 2016;11:148. [Crossref] [PubMed]
- Franzese C, Cozzi L, Badalamenti M, Baldaccini D, D'Agostino G, Fogliata A, Navarria P, Franceschini D, Comito T, Clerici E, Reggiori G, Tomatis S, Scorsetti M. Radiomics-based prognosis classification for high-risk prostate cancer treated with radiotherapy. Strahlenther Onkol 2022;198:710-8. [Crossref] [PubMed]
- Gugliandolo SG, Pepa M, Isaksson LJ, Marvaso G, Raimondi S, Botta F, et al. MRI-based radiomics signature for localized prostate cancer: a new clinical tool for cancer aggressiveness prediction? Sub-study of prospective phase II trial on ultra-hypofractionated radiotherapy (AIRC IG-13218). Eur Radiol 2021;31:716-28.
- Klotz L. Active surveillance in intermediate-risk prostate cancer. BJU Int 2020;125:346-54.
- Lee CH, Tan TW, Tan CH. Multiparametric MRI in Active Surveillance of Prostate Cancer: An Overview and a Practical Approach. Korean J Radiol 2021;22:1087-99. [Crossref] [PubMed]
- Xiao Y, Qiu M, Huang W, Hu S, Tan C, Nan F, Jiang X, Wu D, Li M, Li Q, Qin C. Global status of research on radiotherapy for rectal cancer: A bibliometric and visual analysis. Front Public Health 2022;10:962256. [Crossref] [PubMed]
- Yu KH, Beam AL, Kohane IS. Artificial intelligence in healthcare. Nat Biomed Eng 2018;2:719-31. [Crossref] [PubMed]
- Byeon H. Screening dementia and predicting high dementia risk groups using machine learning. World J Psychiatry 2022;12:204-11.
- Gaudiano C, Mottola M, Bianchi L, Corcioni B, Cattabriga A, Cocozza MA, Palmeri A, Coppola F, Giunchi F, Schiavina R, Fiorentino M, Brunocilla E, Golfieri R, Bevilacqua A. Beyond Multiparametric MRI and towards Radiomics to Detect Prostate Cancer: A Machine Learning Model to Predict Clinically Significant Lesions. Cancers (Basel) 2022;14:6156. [Crossref] [PubMed]
- Min X, Li M, Dong D, Feng Z, Zhang P, Ke Z, You H, Han F, Ma H, Tian J, Wang L. Multi-parametric MRI-based radiomics signature for discriminating between clinically significant and insignificant prostate cancer: Cross-validation of a machine learning method. Eur J Radiol 2019;115:16-21. [Crossref] [PubMed]
- Nketiah GA, Bathen TF. Editorial for "MRI Radiomics-Based Machine Learning for Predict of Clinically Significant Prostate Cancer in Equivocal PI-RADS 3 Lesions". J Magn Reson Imaging 2021;54:1474-5.
- Mazurowski MA, Buda M, Saha A, Bashir MR. Deep learning in radiology: An overview of the concepts and a survey of the state of the art with focus on MRI. J Magn Reson Imaging 2019;49:939-54. [Crossref] [PubMed]
- Harder FN, Weiss K, Amiel T, Peeters JM, Tauber R, Ziegelmayer S, Burian E, Makowski MR, Sauter AP, Gschwend JE, Karampinos DC, Braren RF. Prospectively Accelerated T2-Weighted Imaging of the Prostate by Combining Compressed SENSE and Deep Learning in Patients with Histologically Proven Prostate Cancer. Cancers (Basel) 2022;14:5741. [Crossref] [PubMed]
- Johnson PM, Tong A, Donthireddy A, Melamud K, Petrocelli R, Smereka P, Qian K, Keerthivasan MB, Chandarana H, Knoll F. Deep Learning Reconstruction Enables Highly Accelerated Biparametric MR Imaging of the Prostate. J Magn Reson Imaging 2022;56:184-95. [Crossref] [PubMed]
- Ueda T, Ohno Y, Yamamoto K, Murayama K, Ikedo M, Yui M, Hanamatsu S, Tanaka Y, Obama Y, Ikeda H, Toyama H. Deep Learning Reconstruction of Diffusion-weighted MRI Improves Image Quality for Prostatic Imaging. Radiology 2022;303:373-81. [Crossref] [PubMed]
- Zabihollahy F, Ukwatta E, Krishna S, Schieda N. Fully automated localization of prostate peripheral zone tumors on apparent diffusion coefficient map MR images using an ensemble learning method. J Magn Reson Imaging 2020;51:1223-34. [Crossref] [PubMed]
- Aldoj N, Lukas S, Dewey M, Penzkofer T. Semi-automatic classification of prostate cancer on multi-parametric MR imaging using a multi-channel 3D convolutional neural network. Eur Radiol 2020;30:1243-53. [Crossref] [PubMed]
- Bleker J, Kwee TC, Rouw D, Roest C, Borstlap J, de Jong IJ, Dierckx RAJO, Huisman H, Yakar D. A deep learning masked segmentation alternative to manual segmentation in biparametric MRI prostate cancer radiomics. Eur Radiol 2022;32:6526-35. [Crossref] [PubMed]
- Montagne S, Hamzaoui D, Allera A, Ezziane M, Luzurier A, Quint R, Kalai M, Ayache N, Delingette H, Renard-Penna R. Challenge of prostate MRI segmentation on T2-weighted images: inter-observer variability and impact of prostate morphology. Insights Imaging 2021;12:71. [Crossref] [PubMed]
- Djavan B, Zlotta AR, Byttebier G, Shariat S, Omar M, Schulman CC, Marberger M. Prostate specific antigen density of the transition zone for early detection of prostate cancer. J Urol 1998;160:411-8; discussion 418-9.
- Nordström T, Akre O, Aly M, Grönberg H, Eklund M. Prostate-specific antigen (PSA) density in the diagnostic algorithm of prostate cancer. Prostate Cancer Prostatic Dis 2018;21:57-63. [Crossref] [PubMed]
- Washino S, Okochi T, Saito K, Konishi T, Hirai M, Kobayashi Y, Miyagawa T. Combination of prostate imaging reporting and data system (PI-RADS) score and prostate-specific antigen (PSA) density predicts biopsy outcome in prostate biopsy naïve patients. BJU Int 2017;119:225-33.