Effect of multiple peritumoral regions of interest ranges based on computed tomography radiomics for the prediction of early recurrence of hepatocellular carcinoma after resection
Introduction
Hepatocellular carcinoma (HCC) is one of the most common malignancies and the third leading cause of cancer deaths worldwide (1). Surgical hepatectomy or liver transplantation is the main treatment option for patients with HCC and the predominant curative treatment modality (2). The main problem after resection is the high rate of tumor recurrence. HCC recurrence after resection can be classified as early recurrence (ER), occurring within 2 years, and late recurrence, occurring >2 years after resection (3,4). The ER rate after tumor resection is about 5–60% (5). Currently, multiple HCC staging systems are key for prognosis and treatment guidance (6,7). However, these systems do not provide quantifiable risk measures, and none are sufficient to accurately predict tumor recurrence. Therefore, identifying reliable predictors associated with ER after HCC resection is critical for patient risk stratification, treatment decisions, and improving long-term survival.
Radiomics enables an in-depth description of tumor phenotypes by extracting large and quantitative imaging features and has shown great potential both in providing intratumoral information about heterogeneous tumors and in predicting prognosis after tumor treatment (8,9). Regions of interest (ROI) are the study target regions used to extract radiomics features, and the extent of the ROI largely determines the predictive efficacy of radiomics models (10). In recent years, some studies have been conducted to predict the recurrence and survival, microvascular invasion (MVI) of HCC using radiomics models (11-13). Ji et al. showed that radiomics models based on enhanced computed tomography (CT) could effectively predict early HCC recurrence (14). However, the peritumoral region around the tumor consisting of parenchyma can more effectively reflect the state of the tumor microenvironment (TME) and changes (15,16), which is valuable for assessing the aggressive behavior of tumors. Several studies have included the peritumoral range for ROI analyses, gradually recognizing the importance of this region for tumor radiomics ROI (17-19). Nevertheless, the choice of the peritumoral ROI range for predicting ER of HCC remains controversial (20,21), and the optimal peritumoral ROI range needs to be further explored.
In this study, we hypothesized that different peritumoral range in CT-based radiomics may have different effects on predicting ER. Therefore, the aim of the study was to evaluate the effect of multiple peritumoral regions on ER prediction in HCC and thus to determine the optimal ROI range and to construct a combined clinical radiomics prediction model. We present this article in accordance with the TRIPOD reporting checklist (available at https://qims.amegroups.com/article/view/10.21037/qims-23-226/rc).
Methods
Study population and follow-up
The study was conducted in accordance with the Declaration of Helsinki (as revised in 2013). The Ethics Committee of the Third Xiangya Hospital approved the study and waived the requirement for informed consent of patients due to the retrospective nature of this study. Data were obtained from patients with HCC who underwent routine preoperative contrast-enhanced CT examinations within 1 month prior to surgery between January 2014 and December 2019. The inclusion and exclusion criteria were as follows. Inclusion criteria: (I) enhanced CT examination within 1 month before surgery; (II) postoperative pathological diagnosis of HCC; (III) no other treatment such as radiofrequency ablation, immunotherapy, transarterial chemoembolization and other treatments before surgical resection; and (IV) complete clinical data. Exclusion criteria: (I) a combination of tumors from other sites; (II) metastatic HCC and intrahepatic cholangiocarcinoma; (III) CT image quality did not meet the requirements; (IV) lost to follow-up; (V) tumors were close to the liver border or major blood vessels.
All patients were followed up by outpatient and/or telephone visits to determine ER. Outpatient follow-up was reviewed within 1 month after surgery and every 3 months thereafter. The review included alpha-fetoprotein (AFP), CT, and magnetic resonance imaging (MRI) to determine if the patient had an ER. ER was defined as new intrahepatic lesions and/or extrahepatic metastases within 2 years after HCC resection. The follow-up ended in December 2021 for all patients.
CT image acquisition
Enhanced CT images of all patients were exported from picture archiving and communication system (PACS) system and saved as dicom images in medicine files for analysis. Images were obtained from two different CT scanners. (I) GE Revolution CT and (II) Philips Brilliance 64 CT (Table S1). After the completion of the plain scan, a nonionic contrast agent (iohexol, 300 mg/mL; GE Healthcare) was administered at a dose of 60 to 110 mL (1.5 mL/kg) and an average injection rate of 3.0 mL/s. Using the mass injection tracking technique: images of the arterial phase were acquired 15 s after the abdominal aortic attenuation value reached 100 HU; images of the portal phase and delayed phase were acquired 30 and 180 s after the arterial phase, respectively.
ROI segmentation
Figure 1 shows the flow of the study. After training, two radiologists with 5 years of experience who were unaware of the patient’s clinical information and ER, performed original region of interest (OROI) segmentation of arterial and portal CT images in ITK-SNAP software (Version 3.6.0, www.itksnap.org). The three-dimensional ROI was formed by manually outlining the tumor edge layer by layer, followed by importing the three-dimensional ROI and the original image together into Artificial Intelligent Kit version 3.2.2 (GE Healthcare) using the function of dilation as the basis of the OROI to expand 1–5 mm outward sequentially (Figure 2). For areas with multiple lesions, we selected the one with the largest diameter for segmentation. ROIs were outlined by an experienced radiologist under the guidance of an expert radiologist with 20+ years of experience.
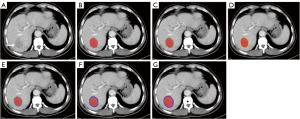
Image preprocessing and feature extraction
Radiomics feature extraction was performed with Artificial Intelligent Kit version 3.2.2 (GE Healthcare), which was in concordance with the Image Biomarker Standardization Initiative (IBSI) guideline (22). Image preprocessing and feature extraction were performed by Artificial Intelligent Kit version 3.2.2. The voxels of all images were resampled at the same size of 1 mm × 1 mm × 1 mm. A gaussian smooth filter algorithm was used for noise reduction. The voxel intensity dispersion range was limited to 64. To evaluate the reproducibility and robustness of tumor delineation or feature extraction, inter- and intraclass correlation coefficients (ICCs) were calculated. After one month, 30 randomly selected cases of which the CT images were delineated by reader were segmented by radiologist A and radiologist B, for evaluation of the intra-observer (radiologist A twice) and inter observer (radiologist A vs. radiologist B) agreement. A total of 792 features were extracted from each patient, including 396 features from each of the arterial and portal phase CT images, with the suffix “_P” added to the portal phase features. Six types of features were extracted from each ROI, including histogram features, gray level co-occurrence matrix (GLCM) features, gray level run length matrix (GLRLM) features, gray level size zone matrix (GLSZM) features, form factor features, and Haralick features (Table S2).
Feature selection and modeling construction
Radiomics features that showed both intra-observer and inter-observer ICC values greater than 0.75 (indicating satisfactory agreement) were selected for subsequent analysis. All features were normalized using the R software “caret” package, and then the features were analyzed for redundancy. Pearson correlation coefficient analysis was performed for the normally distributed features, and Spearman’s rank correlation coefficient analysis was performed for the non-normally distributed features. Least absolute shrinkage and selection operator (LASSO) regression was performed to downscale and filter the remaining features, and the optimal regularization parameter lambda was identified using a fivefold cross-validation method. The radiomics features with nonzero coefficients were screened as the most predictive features, and the radiomics score (Rad score) of the selected features was further calculated. Finally, the Rad score was used to build a logistic regression prediction model.
Statistical analysis
All statistical analyses were performed using R software (Version 3.6.1, https://www.r-project.org). Normally distributed continuous variables were expressed as the mean and standard deviation, and non-normally distributed continuous variables were expressed as the median and quartiles. Categorical variables were expressed as the number of cases and percentages. Continuous variables between the two groups were tested using the Mann-Whitney U test or t-test. Categorical variables were compared by the Chi-square test. Univariate and multivariate logistic regression analyses were performed to identify clinical factors. LASSO regression was used for feature selection. Clinical factors were combined with the optimal ROI to build a logistic combination model. A nomogram was used to visualize the prediction models. The model prediction accuracy in the training and validation cohort was quantified by the area under the curve (AUC), and calibration curves and decision curves were used to evaluate the models. The AUCs of different models were compared using Delong’s test. The cutoff values for high-low risk stratification were determined based on the maximum Youden index of the combined model receiver operator characteristic (ROC) curve, and high-low risk stratification was performed for all patients. All statistical tests were two-tailed, and P<0.05 was considered a statistically significant difference.
Results
Baseline characteristics
Table 1 shows the baseline clinical characteristics of all patients. The 160 HCC patients were randomized in a 7:3 ratio, with 112 patients divided into the training cohort and 48 patients into the validation cohort. Ninety-seven (60.6%) of the patients were ER; 63 (39.4%) did not. None of the variables were significantly different between the groups.
Table 1
Characteristic | All patients, N=160 | Training cohort, N=112 | Validation cohort, N=48 | P value |
---|---|---|---|---|
Age (years) | 0.576 | |||
≥50 | 109 (68.1) | 76 (67.9) | 33 (68.8) | |
<50 | 51 (31.9) | 36 (32.1) | 15 (31.2) | |
Gender | 0.3 | |||
Female | 18 (11.2) | 15 (13.4) | 3 (6.2) | |
Male | 142 (88.8) | 97 (86.6) | 45 (93.8) | |
ALT (U/L) | 0.25 | |||
≥50 | 45 (28.1) | 35 (31.2) | 10 (20.8) | |
<50 | 115 (71.9) | 77 (68.8) | 38 (79.2) | |
AST (U/L) | 0.535 | |||
≥40 | 81 (50.6) | 59 (52.7) | 22 (45.8) | |
<40 | 79 (79.4) | 53 (47.3) | 26 (54.2) | |
ALB (g/L) | 0.269 | |||
≥45 | 30 (18.8) | 18 (16.1) | 12 (25.0) | |
<45 | 130 (81.2) | 94 (83.9) | 36 (75.0) | |
Tumor size (cm) | 0.397 | |||
≥5 | 97 (60.6) | 65 (58.0) | 32 (66.7) | |
<5 | 63 (39.4) | 47 (42.0) | 16 (33.6) | |
Child-Pugh class | 0.462 | |||
A | 126 (78.8) | 87 (77.7) | 39 (81.2) | |
B | 34 (21.2) | 25 (22.3) | 9 (18.8) | |
HBV | >0.99 | |||
Negative | 23 (14.4) | 16 (14.3) | 7 (14.6) | |
Positive | 137 (85.6) | 96 (85.7) | 41 (85.2) | |
AFP (ng/mL) | 0.224 | |||
≤100 | 90 (56.2) | 67 (59.8) | 23 (47.9) | |
>100 | 70 (43.8) | 45 (40.2) | 25 (52.1) | |
Platelet | 150.0 (101.8–200.5) | 141.0 (97.0–197.0) | 160.5 (105.3–203.8) | 0.335 |
INR | 1.10 (1.03–1.18) | 1.10 (1.03–1.19) | 1.09 (1.04–1.17) | 0.901 |
PT (s) | 12.6 (11.8–13.4) | 12.6 (11.8–13.3) | 12.5 (11.8–13.5) | 0.689 |
Tumor number | 0.93 | |||
Single | 129 (80.6) | 91 (81.2) | 38 (79.2) | |
Multiple | 31 (19.4) | 21 (18.8) | 10 (20.8) | |
Cirrhosis | 0.653 | |||
Negative | 76 (47.5) | 55 (49.1) | 21 (43.8) | |
Positive | 84 (52.5) | 57 (50.9) | 27 (56.2) | |
BCLC | >0.99 | |||
A | 73 (45.6) | 51 (45.5) | 22 (45.8) | |
B | 87 (54.4) | 61 (54.5) | 26 (54.2) | |
MVI | >0.99 | |||
Negative | 97(60.6) | 68 (60.7) | 29 (60.6) | |
Positive | 63 (39.4) | 44 (39.3) | 19 (39.4) | |
Early recurrence | 0.397 | |||
No | 63 (39.4) | 47 (42.0) | 16 (33.3) | |
Yes | 97 (60.6) | 65 (58.0) | 32 (66.7) |
Data are expressed as median (IQR) or number (percentage). ALT, alanine aminotransferase; AST, aspartate aminotransferase; ALB, albumin; AFP, alpha-fetoprotein; INR, international normalized ratio; PT, prothrombin time; HBV, hepatitis B virus; BCLC, Barcelona Clinic Liver Cancer; MVI, microvascular invasion; IQR, interquartile range.
Clinical factors model construction
The results of the univariate and multivariate analyses of clinical factors associated with ER in the training cohort are summarized in Table 2. Univariate and multivariate logistic regression analyses revealed MVI (OR =2.83, P=0.037) and AFP (>100 ng/mL) (OR =3.32, P=0.013) as clinically independent risk factors for ER. The clinical model had AUC values and 95% confidence intervals (CIs) of 0.724 (0.638–0.809) and 0.694 (0.552–0.837) in the training and validation cohorts, respectively.
Table 2
Characteristics | Univariate analysis | Multivariate analysis | |||
---|---|---|---|---|---|
Odds ratio (95% CI) | P value | Odds ratio (95% CI) | P value | ||
Age (years) | |||||
≥50 | |||||
<50 | 0.41 (0.17–0.96) | 0.039 | 0.70 (0.27–1.86) | 0.478 | |
Gender | |||||
Female | |||||
Male | 1.53 (0.49–4.81) | 0.469 | |||
ALB (g/L) | |||||
<45 | |||||
≥45 | 0.52 (0.19–1.44) | 0.207 | |||
ALT (U/L) | |||||
<50 | |||||
≥50 | 0.80 (0.36–1.79) | 0.588 | |||
AST (U/L) | |||||
<40 | |||||
≥40 | 1.30 (0.61–2.75) | 0.5 | |||
PT (s) | 1.05 (0.84–1.31) | 0.682 | |||
Platelet | 1.00 (1.00–1.01) | 0.779 | |||
INR | 1.16 (0.11–12.17) | 0.903 | |||
Tumor size (cm) | 0.914 | ||||
<5 | |||||
≥5 | 1.04 (0.49–2.23) | ||||
Child-Pugh class | |||||
A | |||||
B | 1.18 (0.56–2.48) | 0.663 | |||
HBV | |||||
Negative | |||||
Positive | 0.8 (0.27–2.39) | 0.696 | |||
AFP (ng/mL) | |||||
≤100 | |||||
>100 | 5.24 (2.18–12.58) | <0.001 | 3.32 (1.28–8.58) | 0.013 | |
Tumor number | |||||
Single | |||||
Multiple | 1.22 (0.46–3.23) | 0.69 | |||
Cirrhosis | |||||
Negative | |||||
Positive | 1.14 (0.54–2.43) | 0.725 | |||
BCLC | |||||
A | |||||
B | 0.81 (0.38–1.73) | 0.59 | |||
MVI | |||||
Negative | |||||
Positive | 4.93 (2.05–11.82) | <0.001 | 2.83 (1.06–7.51) | 0.037 |
ALT, alanine aminotransferase; AST, aspartate aminotransferase; ALB, albumin; AFP, alpha-fetoprotein; INR, international normalized ratio; PT, prothrombin time; HBV, hepatitis B virus; BCLC, Barcelona Clinic Liver Cancer; MVI, microvascular invasion.
Different ranges of ROI radiomics model construction
OROI-ROI 5 had 3, 6, 11, 14, 2, and 12 features used to build radiomics models, respectively (Figure S1, Table S3). The predictive ability of each ROI radiomics model in the training and validation cohort are summarized in Table 3. ROI 3 was determined to be the optimal ROI based on the AUC values in the training and validation cohorts, and the Rad score was calculated and compared for each patient in ROI 3 (Figure 3A,3B). Rad scores were significantly higher in ER-positive patients than in ER-negative patients (Figure 3C,3D). The correlation heatmap depicted the correlation coefficient matrix for the 14 features in the ROI 3 radiomics model in the training cohort (Figure S2). Heatmap depicted the 14 features in the ROI 3 radiomics model in the ER-positive and ER-negative groups within the training cohort (Figure S3).
Table 3
ROI | Cohort | Sensitivity | Specificity | Accuracy | AUC (95% CI) |
---|---|---|---|---|---|
OROI | Training cohort | 0.492 | 0.851 | 0.643 | 0.710 (0.614–0.806) |
Validation cohort | 0.656 | 0.821 | 0.688 | 0.707 (0.547–0.867) | |
ROI 1 | Training cohort | 0.523 | 0.872 | 0.67 | 0.759 (0.669–0.848) |
Validation cohort | 0.625 | 0.812 | 0.688 | 0.695 (0.541–0.850) | |
ROI 2 | Training cohort | 0.662 | 0.83 | 0.732 | 0.784 (0.698–0.870) |
Validation cohort | 0.75 | 0.75 | 0.75 | 0.711 (0.545–0.877) | |
ROI 3 | Training cohort | 0.8 | 0.787 | 0.795 | 0.867 (0.802–0.933) |
Validation cohort | 0.719 | 0.812 | 0.75 | 0.807 (0.682–0.931) | |
ROI 4 | Training cohort | 0.554 | 0.915 | 0.705 | 0.765 (0.677–0.853) |
Validation cohort | 0.844 | 0.75 | 0.812 | 0.752 (0.583–0.921) | |
ROI 5 | Training cohort | 0.677 | 0.766 | 0.714 | 0.777 (0.693–0.862) |
Validation cohort | 0.875 | 0.5 | 0.75 | 0.705 (0.544–0.866) |
ROI, regions of interest; OROI, original region of interest; AUC, area under the curve; CI, confidence intervals.
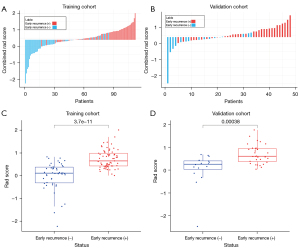
Comparison of radiomic models with different ranges of ROI
The ROC curves for OROI-ROI 5 are shown in Figure 4A,4B. The training cohort had the largest AUC value for ROI 3, which was significantly different from the OROI AUC value (P=0.012). The validation cohort also had the largest AUC value for ROI 3, which was not significantly different from the other ROIs. ROI 3 was determined to be the optimal ROI.
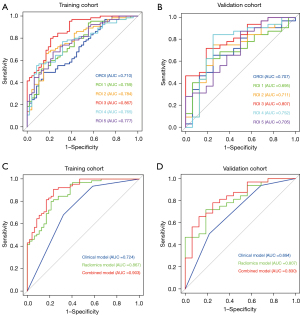
Construction and evaluation of the combined clinical-radiomics model
Based on the above study, two clinical risk factors, AFP and MVI, were combined with ROI 3 Rad score to establish a combined clinical-radiomics model. The ROC curves of the clinical model, the radiomics model and the combined model are shown in Figure 4C,4D. The sensitivity, specificity, accuracy, and AUC values and 95% of the combined model in the training cohort were 0.908, 0.766, 0.848, and 0.903 (0.848–0.957), respectively. The sensitivity, specificity, accuracy, AUC and 95% CIs of the combined model in the validation cohort were 0.781, 0.750, 0.771, and 0.830 (0.709–0.952), respectively. Nomograms were drawn to visualize the predictive ability of the model (Figure 5A). In the training and validation cohort, the ROI 3 radiomics score combined with clinical factors constituted a combined model that further enhanced the predictive model efficacy, and the combined model had the optimal predictive efficacy (Table 4). Delong-test results indicated that there were significant differences between predictive performance of radiomic model, clinical model, and combined model in both cohorts (Figure 5B,5C).
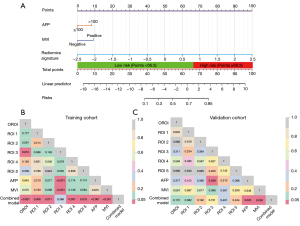
Table 4
Models | Cohort | Sensitivity | Specificity | Accuracy | AUC (95% CI) |
---|---|---|---|---|---|
Clinical model | Training cohort | 0.677 | 0.681 | 0.679 | 0.724 (0.638–0.809) |
Validation cohort | 0.781 | 0.5 | 0.688 | 0.694 (0.552–0.837) | |
Radiomics model | Training cohort | 0.8 | 0.787 | 0.795 | 0.867 (0.802–0.933) |
Validation cohort | 0.719 | 0.812 | 0.75 | 0.807 (0.682–0.931) | |
Combined model | Training cohort | 0.908 | 0.766 | 0.848 | 0.903 (0.848–0.957) |
Validation cohort | 0.781 | 0.75 | 0.771 | 0.830 (0.709–0.952) |
AUC, area under the curve; CI, confidence intervals.
The calibration curves showed high agreement between the predicted and actual probabilities in both the training and validation cohorts (Figure 6A,6B). Within a range, the decision curves showed that the combined model had a higher net benefit rate than both the radiomics model and the clinical model (Figure 6C,6D). Net reclassification improvement (NRI) and integrated discrimination improvement (IDI) were measured to quantify the prediction accuracy of the radiomics models and combined model (Table S4). Analysis of accuracy showed that the IDI and NRI of combined models compared with clinical model in training and validation cohorts were all larger than 0 with all P<0.001, indicating a better prediction power of the combined model compared with clinical model.
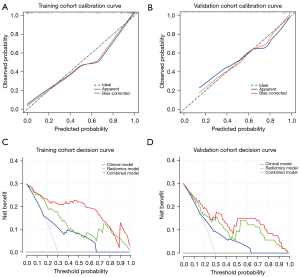
Risk stratification and subgroup analysis
The predicted total score for each patient was calculated based on the nomogram, and the cutoff value determined, based on the ROC maximum Youden index of the combined model in the training cohort, was 66.5. High- and low-risk stratification was performed based on the cutoff value (Figure S4A,S4B). Patients with a score <66.5 in the training cohort and validation cohort were considered to be in the low-risk group, and patients with a score ≥66.5 were considered to be at high-risk group, with a statistically significant difference in the total score between the two groups (Figure S4C,S4D). The difference in the proportion of predicted ER between the low-risk and high-risk subgroups was statistically significant in the training cohort (2.1% vs. 80.0%; P<0.001) and the validation cohort (12.5% vs. 58.1%; P<0.001) (Table S5).
Discussion
In this study, we first elucidated that enhanced CT-based intratumoral and peritumoral ROI radiomics models of different ranges could improve the predictive efficacy of ER after HCC resection. We then compared the predictive efficacy of peritumoral ROI radiomics models of different ranges and determined ROI 3 as the optimal ROI. Next, we developed a combined clinical-radiomics model based on the peritumoral 3 mm ROI radiomics, and the combined model showed the optimal predictive efficacy. Finally, the combination-based model effectively stratified all patients into high- and low-risk categories.
In terms of clinical factors, previous studies have also shown that high AFP levels are associated with ER in HCC (23,24), which is consistent with our results. MVI is an important prognostic factor for HCC (25,26). According to our study, MVI positivity was strongly associated with ER after HCC resection, suggesting aggressive behavior and poorer survival outcomes in HCC, probably because peritumoral tissues are the first and most susceptible to tumor cells, and their surrounding MVI further acts as a major hematogenous dissemination pathway for portal vein tumor thrombosis and metastasis (27). Our clinical model showed only moderate predictive performance, suggesting that the basic clinicopathological factors are not sufficiently predictive and that additional factors are needed to improve the prediction of ER after HCC surgery.
In terms of radiomics, Previous studies have found that peritumoral radiomics is more valuable in assessing tumor heterogeneity (28,29). However, existing radiomic studies are controversial with regard to the range of peritumoral ROIs for predicting HCC recurrence (20,30,31). Therefore, we constructed multiple models with different ranges of ROI radiomics to explore the highly aggressive peritumoral region. Considering that the actual boundary of the tumor is larger than the tumor boundary on imaging, we formed five different peritumoral ROIs by extending the intratumoral OROI by 1–5 mm. The results of the study showed that the AUC of peritumoral 3 mm ROI 3 was 0.865 and 0.803 in the training cohort and validation cohort, respectively, which was the optimal ROI; and the peritumoral 3 mm range was determined to be the optimal ROI.
The ROI 3 radiomics model contained the radiomics features with the most predictive properties. The combination of radiomics features from different categories provides the optimal predictive performance. Although explaining the complex relationship between pathophysiological processes and structural features of tumors remains a challenge to be overcome, tumors with higher structural heterogeneity tend to be more aggressive (32). The higher is the heterogeneity of HCC, the more likely it is to break through the envelope and infiltrate into the surrounding area. This is manifested in the image as an increase in image grayscale inhomogeneity and an increase in image complexity. These features may reflect the aggressive tendency of tumors breaking through the envelope and invading peritumoral nontumor tissues, resulting in increased intra- and peritumoral heterogeneity, which may potentially reflect tumor biology and heterogeneity. Compared to a single intratumor, intratumoral features combined with peritumoral radiomic features were more advantageous, indicating the importance of the fusion of intratumoral and peritumoral features. There were significant differences in texture, intensity, and grayness between normal liver and intratumoral and peritumoral tissues, and the intratumoral combined with peritumoral ROI had a synergistic enhancement effect in predicting ER. The TME consisting of peritumor parenchyma contains rich biological information (33), and radiomics can assess subtle alterations in tumor pathophysiology as well as the TME that we cannot identify with the naked eye. We speculate that this is an important reason for the improved predictive efficacy of the ROI 3 compared to the intratumoral OROI. When peritumoral liver tissue extending 4–5 mm outward along the tumor border formed ROI 4 and ROI 5, the number of features used for model construction decreased, and the predictive performance of the model decreased, suggesting that a larger peritumoral range does not necessarily have better predictive performance. This is because some features with predictive value may be interfered with by normal liver tissue and become redundant features, which ultimately leads to a decrease in predictive model performance.
Finally, we constructed a combined clinical-radiomics model that included MVI, AFP, and ROI 3. In this study, the combined model was the best in terms of AUC, accuracy, and discrimination in predicting ER compared with a single radiomics or clinical model. Our study is one of the very few to explore and compare the heterogeneity of multiple different ranges of radiomics ROIs around the tumor perimeter (1–5 mm) in the same study to determine the optimal ROI range for predicting ER after HCC resection as well as effective risk stratification for all patients.
However, there are several limitations in this study. (I) This study was a single-center retrospective study with a small number of included patients and a small sample size, and a multicenter study is needed to validate our findings. (II) We did not combine specific indicators of the TME (immune cell) with radiomics features to further explore the link between radiomics and the peritumor microenvironment, and the combination of both may explain the specificity and importance of the peritumor region more rationally.
Conclusions
In summary, peritumoral ROI radiomics can improve the predictive efficacy of the model, the range of peritumoral 3 mm was determined to be the optimal ROI. The combined clinical-radiomics model has the optimal predictive efficacy, and can achieve effective stratification of patients with high- and low-risk for timely clinical guidance and decision making.
Acknowledgments
Funding: None.
Footnote
Reporting Checklist: The authors have completed the TRIPOD reporting checklist. Available at https://qims.amegroups.com/article/view/10.21037/qims-23-226/rc
Conflicts of Interest: All authors have completed the ICMJE uniform disclosure form (available at https://qims.amegroups.com/article/view/10.21037/qims-23-226/coif). The authors have no conflicts of interest to declare.
Ethical Statement: The authors are accountable for all aspects of the work in ensuring that questions related to the accuracy or integrity of any part of the work are appropriately investigated and resolved. This retrospective was conducted in accordance with the Declaration of Helsinki (as revised in 2013). The study was approved by Ethics Committee of the Third Xiangya Hospital, waiving the need for written informed consent due to the retrospective nature of this study.
Open Access Statement: This is an Open Access article distributed in accordance with the Creative Commons Attribution-NonCommercial-NoDerivs 4.0 International License (CC BY-NC-ND 4.0), which permits the non-commercial replication and distribution of the article with the strict proviso that no changes or edits are made and the original work is properly cited (including links to both the formal publication through the relevant DOI and the license). See: https://creativecommons.org/licenses/by-nc-nd/4.0/.
References
- Vogel A, Meyer T, Sapisochin G, Salem R, Saborowski A. Hepatocellular carcinoma. Lancet 2022;400:1345-62. [Crossref] [PubMed]
- Sapisochin G, Bruix J. Liver transplantation for hepatocellular carcinoma: outcomes and novel surgical approaches. Nat Rev Gastroenterol Hepatol 2017;14:203-17. [Crossref] [PubMed]
- Tang ZY, Ye SL, Liu YK, Qin LX, Sun HC, Ye QH, Wang L, Zhou J, Qiu SJ, Li Y, Ji XN, Liu H, Xia JL, Wu ZQ, Fan J, Ma ZC, Zhou XD, Lin ZY, Liu KD. A decade's studies on metastasis of hepatocellular carcinoma. J Cancer Res Clin Oncol 2004;130:187-96. [Crossref] [PubMed]
- Yang Y, Nagano H, Ota H, Morimoto O, Nakamura M, Wada H, Noda T, Damdinsuren B, Marubashi S, Miyamoto A, Takeda Y, Dono K, Umeshita K, Nakamori S, Wakasa K, Sakon M, Monden M. Patterns and clinicopathologic features of extrahepatic recurrence of hepatocellular carcinoma after curative resection. Surgery 2007;141:196-202. [Crossref] [PubMed]
- Papaconstantinou D, Tsilimigras DI, Pawlik TM. Recurrent Hepatocellular Carcinoma: Patterns, Detection, Staging and Treatment. J Hepatocell Carcinoma 2022;9:947-57. [Crossref] [PubMed]
- Kulik L, El-Serag HB. Epidemiology and Management of Hepatocellular Carcinoma. Gastroenterology 2019;156:477-491.e1. [Crossref] [PubMed]
- Bruix J, Reig M, Sherman M. Evidence-Based Diagnosis, Staging, and Treatment of Patients With Hepatocellular Carcinoma. Gastroenterology 2016;150:835-53. [Crossref] [PubMed]
- Bera K, Braman N, Gupta A, Velcheti V, Madabhushi A. Predicting cancer outcomes with radiomics and artificial intelligence in radiology. Nat Rev Clin Oncol 2022;19:132-46. [Crossref] [PubMed]
- Pinker K, Chin J, Melsaether AN, Morris EA, Moy L. Precision Medicine and Radiogenomics in Breast Cancer: New Approaches toward Diagnosis and Treatment. Radiology 2018;287:732-47. [Crossref] [PubMed]
- Lambin P, Leijenaar RTH, Deist TM, Peerlings J, de Jong EEC, van Timmeren J, Sanduleanu S, Larue RTHM, Even AJG, Jochems A, van Wijk Y, Woodruff H, van Soest J, Lustberg T, Roelofs E, van Elmpt W, Dekker A, Mottaghy FM, Wildberger JE, Walsh S. Radiomics: the bridge between medical imaging and personalized medicine. Nat Rev Clin Oncol 2017;14:749-62. [Crossref] [PubMed]
- Ji GW, Zhu FP, Xu Q, Wang K, Wu MY, Tang WW, Li XC, Wang XH. Machine-learning analysis of contrast-enhanced CT radiomics predicts recurrence of hepatocellular carcinoma after resection: A multi-institutional study. EBioMedicine 2019;50:156-65. [Crossref] [PubMed]
- Dai H, Lu M, Huang B, Tang M, Pang T, Liao B, Cai H, Huang M, Zhou Y, Chen X, Ding H, Feng ST. Considerable effects of imaging sequences, feature extraction, feature selection, and classifiers on radiomics-based prediction of microvascular invasion in hepatocellular carcinoma using magnetic resonance imaging. Quant Imaging Med Surg 2021;11:1836-53. [Crossref] [PubMed]
- Yuan C, Wang Z, Gu D, Tian J, Zhao P, Wei J, Yang X, Hao X, Dong D, He N, Sun Y, Gao W, Feng J. Prediction early recurrence of hepatocellular carcinoma eligible for curative ablation using a Radiomics nomogram. Cancer Imaging 2019;19:21. [Crossref] [PubMed]
- Ji GW, Zhu FP, Xu Q, Wang K, Wu MY, Tang WW, Li XC, Wang XH. Radiomic Features at Contrast-enhanced CT Predict Recurrence in Early Stage Hepatocellular Carcinoma: A Multi-Institutional Study. Radiology 2020;294:568-79. [Crossref] [PubMed]
- Franzén AS, Raftery MJ, Pecher G. Implications for Immunotherapy of Breast Cancer by Understanding the Microenvironment of a Solid Tumor. Cancers (Basel) 2022.
- Ding GY, Ma JQ, Yun JP, Chen X, Ling Y, Zhang S, Shi JY, Chang YQ, Ji Y, Wang XY, Tan WM, Yuan KF, Yan B, Zhang XM, Liang F, Zhou J, Fan J, Zeng Y, Cai MY, Gao Q. Distribution and density of tertiary lymphoid structures predict clinical outcome in intrahepatic cholangiocarcinoma. J Hepatol 2022;76:608-18. [Crossref] [PubMed]
- Chong HH, Yang L, Sheng RF, Yu YL, Wu DJ, Rao SX, Yang C, Zeng MS. Multi-scale and multi-parametric radiomics of gadoxetate disodium-enhanced MRI predicts microvascular invasion and outcome in patients with solitary hepatocellular carcinoma ≤ 5 cm. Eur Radiol 2021;31:4824-38. [Crossref] [PubMed]
- Wu L, Gao C, Ye J, Tao J, Wang N, Pang P, Xiang P, Xu M. The value of various peritumoral radiomic features in differentiating the invasiveness of adenocarcinoma manifesting as ground-glass nodules. Eur Radiol 2021;31:9030-7. [Crossref] [PubMed]
- Chen Q, Shao J, Xue T, Peng H, Li M, Duan S, Feng F. Intratumoral and peritumoral radiomics nomograms for the preoperative prediction of lymphovascular invasion and overall survival in non-small cell lung cancer. Eur Radiol 2023;33:947-58. [Crossref] [PubMed]
- Shan QY, Hu HT, Feng ST, Peng ZP, Chen SL, Zhou Q, Li X, Xie XY, Lu MD, Wang W, Kuang M. CT-based peritumoral radiomics signatures to predict early recurrence in hepatocellular carcinoma after curative tumor resection or ablation. Cancer Imaging 2019;19:11. [Crossref] [PubMed]
- Wang F, Cheng M, Du B, Li LM, Huang WP, Gao JB. Use of radiomics containing an effective peritumoral area to predict early recurrence of solitary hepatocellular carcinoma ≤5 cm in diameter. Front Oncol 2022;12:1032115. [Crossref] [PubMed]
- Zwanenburg A, Vallières M, Abdalah MA, Aerts HJWL, Andrearczyk V, Apte A, et al. The Image Biomarker Standardization Initiative: Standardized Quantitative Radiomics for High-Throughput Image-based Phenotyping. Radiology 2020;295:328-38. [Crossref] [PubMed]
- Zhang Z, Jiang H, Chen J, Wei Y, Cao L, Ye Z, Li X, Ma L, Song B. Hepatocellular carcinoma: radiomics nomogram on gadoxetic acid-enhanced MR imaging for early postoperative recurrence prediction. Cancer Imaging 2019;19:22. [Crossref] [PubMed]
- Zhu HB, Zheng ZY, Zhao H, Zhang J, Zhu H, Li YH, Dong ZY, Xiao LS, Kuang JJ, Zhang XL, Liu L. Radiomics-based nomogram using CT imaging for noninvasive preoperative prediction of early recurrence in patients with hepatocellular carcinoma. Diagn Interv Radiol 2020;26:411-9. [Crossref] [PubMed]
- Jiang C, Zhao L, Xin B, Ma G, Wang X, Song S. (18)F-FDG PET/CT radiomic analysis for classifying and predicting microvascular invasion in hepatocellular carcinoma and intrahepatic cholangiocarcinoma. Quant Imaging Med Surg 2022;12:4135-50. [Crossref] [PubMed]
- Xu X, Zhang HL, Liu QP, Sun SW, Zhang J, Zhu FP, Yang G, Yan X, Zhang YD, Liu XS. Radiomic analysis of contrast-enhanced CT predicts microvascular invasion and outcome in hepatocellular carcinoma. J Hepatol 2019;70:1133-44. [Crossref] [PubMed]
- Lee S, Kang TW, Song KD, Lee MW, Rhim H, Lim HK, Kim SY, Sinn DH, Kim JM, Kim K, Ha SY. Effect of Microvascular Invasion Risk on Early Recurrence of Hepatocellular Carcinoma After Surgery and Radiofrequency Ablation. Ann Surg 2021;273:564-71. [Crossref] [PubMed]
- Zhang L, Hu J, Hou J, Jiang X, Guo L, Tian L. Radiomics-based model using gadoxetic acid disodium-enhanced MR images: associations with recurrence-free survival of patients with hepatocellular carcinoma treated by surgical resection. Abdom Radiol (NY) 2021;46:3845-54. [Crossref] [PubMed]
- Xu H, Liu J, Chen Z, Wang C, Liu Y, Wang M, Zhou P, Luo H, Ren J. Intratumoral and peritumoral radiomics based on dynamic contrast-enhanced MRI for preoperative prediction of intraductal component in invasive breast cancer. Eur Radiol 2022;32:4845-56. [Crossref] [PubMed]
- Kim S, Shin J, Kim DY, Choi GH, Kim MJ, Choi JY. Radiomics on Gadoxetic Acid-Enhanced Magnetic Resonance Imaging for Prediction of Postoperative Early and Late Recurrence of Single Hepatocellular Carcinoma. Clin Cancer Res 2019;25:3847-55. [Crossref] [PubMed]
- Li N, Wan X, Zhang H, Zhang Z, Guo Y, Hong D. Tumor and peritumor radiomics analysis based on contrast-enhanced CT for predicting early and late recurrence of hepatocellular carcinoma after liver resection. BMC Cancer 2022;22:664. [Crossref] [PubMed]
- Craig AJ, von Felden J, Garcia-Lezana T, Sarcognato S, Villanueva A. Tumour evolution in hepatocellular carcinoma. Nat Rev Gastroenterol Hepatol 2020;17:139-52. [Crossref] [PubMed]
- Galon J, Bruni D. Approaches to treat immune hot, altered and cold tumours with combination immunotherapies. Nat Rev Drug Discov 2019;18:197-218. [Crossref] [PubMed]