Artificial intelligence versus radiologist in the accuracy of fracture detection based on computed tomography images: a multi-dimensional, multi-region analysis
Introduction
According to reports, the incidence of traumatic fractures is as high as 0.32% in the United States (1) and up to 1.3% in China (2), which has become a public health problem of global concern. Avulsion fracture, which is the avulsion and displacement of bone fragments due to the traction of tendons and ligaments after trauma, is easy to be missed clinically. A study by Haraguchi et al. (3) showed that the incidence of avulsion fractures is high in patients with severe inversion injury, especially in children and patients over 40 years old. Diagnosis of avulsion fracture is usually difficult on digital radiography (DR) images. Missed fracture on radiograph in the emergency department is a common cause of medical incident and litigation (4,5). It is reported that the annual litigation rate experienced by orthopedic doctors is 14%, which is higher than the national average of 7% for physicians (6,7).
Recently, convolutional neural networks (CNNs) in deep learning have achieved remarkable results in automatic detection of various non-avulsion fractures, ligament injures, and classification of bone tumors, including upper and lower extremities fractures [e.g., distal radius (8), proximal humerus (9), intertrochanteric hip (10)], calcaneofibular ligament injuries in the ankle joint (11), and pelvic and sacral osteosarcoma classification (12). For avulsion fractures, the imaging presentation of fracture signs is often challenging to determine, making it difficult to obtain a sufficient number of labeled samples for artificial intelligence (AI) training to improve their detection. At present, there only a few studies have reported the application of deep learning in avulsion fractures (13). Moreover, most of the studies used imaging reports or interpretations of DR images by radiology experts as the evaluation reference (14-16), which may be affected by individual experience.
Therefore, this study used computed tomography (CT) images to verify the lesion labeling on X-ray radiographs and then optimize an AI model for detecting extremity fractures. The diagnostic efficiency of the AI model before and after the optimization was evaluated and compared with radiologists, especially in the aspect of avulsion fractures. We present this article in accordance with the STARD reporting checklist (available at https://qims.amegroups.com/article/view/10.21037/qims-23-428/rc).
Methods
Data generation
The CT and DR images of adult limb trauma in China-Japan Friendship Hospital from 2017 to 2020 were retrospectively collected. Each X-ray examination followed the conventional projection views, consisting of 1–4 radiographs. Anatomical areas included the shoulder, elbow, wrist, hand, hip, knee, ankle, and foot. The exclusion criteria were as follows: (I) no initial radiological report (42 cases); (II) no corresponding CT examination of the same anatomical area was performed within 2 weeks (2,317 cases); (III) postoperative radiography of the fracture (937 cases); and (IV) poor radiographic quality (5 cases). Totally, 1,035 cases (anatomical areas) with 3,167 radiographs were extracted in this study, including 666 positive cases and 374 negative cases, which were divided into a training set (n=675), a validation set (n=169), and a test set (n=191) in a balanced joint distribution (Figure 1). The number of individual components of the total datasets is shown in Figure 2. The study was conducted in accordance with the Declaration of Helsinki (as revised in 2013). The study was approved by the China-Japan Friendship Hospital Institutional Review Board (Medical ethics number: 2019-94-k62-1) and the requirement for individual consent for this retrospective analysis was waived.
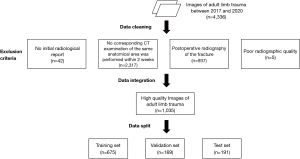
Image labeling
Firstly, 2 radiologists with more than 10 years of experience reviewed the CT images to define the site and scope of the fractures as a gold standard and then labeled the fractures on DR images. DeepWise software (https://label.deepwise.com/) was applied for annotation. A rectangular bounding box was drawn to enclose the fracture line. For a non-avulsion fracture, multiple rectangular bounding boxes can be labeled for 1 fracture site.
Preprocessing
Image arrays in 16-bit integer format were extracted from Digital Imaging and Communications in Medicine (DICOM) files. Then, all the images were resized to the scale so that the short side length was close to 1,200 pixels while maintaining the aspect ratio. Augmentations were employed during training, including random rotation, random flip, random brightness, random crop, and contrast distortion.
Model architecture and learning policy
In this study, an AI diagnosis system for fracture was used for auxiliary diagnosis. The algorithm we propose in this paper was based on the ResNeXt-101-32x4d + feature pyramid networks (FPN) + Mask-region-based CNN (RCNN) model (17), and the adaptive optimization was carried out on the above annotation data set of DR images. Firstly, pre-processed training data were sent to the ResNeXt-101 backbone with FPN to extract multi-scale features (P2–P6 in Figure 3). Secondly, these features from different scale levels were fused to generate global features and sent to the region proposal network (RPN) to make preliminary box predictions and obtain the proposals. In this stage, positive and negative samples were balanced by a data balancing method to adapt to the distribution of the dataset. Thirdly, the region of interest (ROI) alignment was applied to obtain the feature map of the possible lesion area, and then the fully connected layers were used for classification and box regression to predict fractures precisely. In addition, the prediction results were optimized through adjustments such as anchor scale and aspect ratio, the FPN stages and channels, and gradient learning strategies. Finally, label balance was added to shield bad gradients, the proposal was relocated and corrected, and then the multi-scale fused features of the corresponding region were obtained, resulting in a more robust model training. A global learning rate of 0.002 was initially used with 0.9 momentum and 0.0001 weight decay, divided by 10 at 15 epochs and 30 epochs, and then the training stopped after 45 epochs.
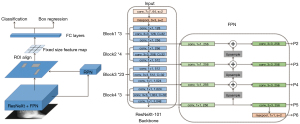
The proposed method was developed using PyTorch 1.7 open-source deep learning library (https://pytorch.org/get-started/previous-versions/), a server with 24 Intel(R) Xeon(R) E5-2685 v3 @ 2.60 GHz CPUs, and 8 NVIDIA TITAN Xp GPU (https://www.nvidia.com/en-au/titan/titan-xp/). The version of CUDA was 10.1.
Model evaluation
The performances of pre-optimized AI model, optimized AI model, and radiologist are evaluated at the lesion and case (anatomical area) level, respectively. For the lesion-based assessment, all fracture lesions were classified as avulsion fractures and non-avulsion fractures. True positive required 1 box of true positive on any of the projected views. For fracture lesion level, since all parts except the fracture can be considered negative, the resulting specificity was meaningless. Therefore, the detection rate was considered as the true positive rate. For case (anatomic area) level, true positive required only 1 box of true positive, regardless of the number of fractures present.
For the performance of the radiologists, initial radiological reports were evaluated. Reports that indicated a fracture with unequivocal diagnosis or probability were rated as positive, whereas description of unspecific signs without conclusion was treated as negative results.
Statistical methods
The study was statistically analyzed using SPSS 26.0 (IBM Corp., Armonk, NY, USA) software, and the count data were expressed as n (%). The detection rates of avulsion fractures and non-avulsion fractures by the pre-optimized AI model, the optimized AI model, and the radiologist were compared, respectively, as well as their accuracy, sensitivity, and specificity for fractures at each anatomic area by using the quality test for paired samples. Significance tests were used to compare detection rate, accuracy, sensitivity, and specificity. Statistical significance was indicated by a two-tailed P<0.05.
Results
Lesion-based diagnostic efficacy
In the test set (191 cases), a total of 266 lesions were identified, including 57 (21.43%) avulsion fractures and 209 (78.57%) non-avulsion fractures. The detection rate of avulsion fracture by the optimized AI was significantly higher than that by pre-optimized AI (57.89% vs. 35.09%, P=0.004), increasing by 22.80%. The detection rate of the optimized AI model for non-avulsion fractures was significantly higher than that of the pre-optimized AI model (85.64% vs. 71.29%, P<0.001), with an increase of 14.35% (Table 1 and Figure 4). The average precision (AP) of optimized AI model for all lesions was higher than that of pre-optimized AI model (0.582 vs. 0.425) (Figure 5).
Table 1
Fracture type | Detection rate | t value | P value | |
---|---|---|---|---|
Pre-optimized AI (%) | Optimized AI (%) | |||
Avulsion fracture (n=57) | 35.09 | 57.89 | −2.871 | 0.004 |
Non-avulsion fracture (n=209) | 71.29 | 85.64 | −4.196 | <0.001 |
AI, artificial intelligence.
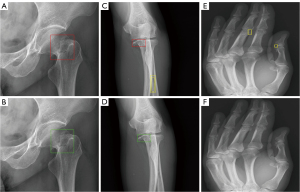
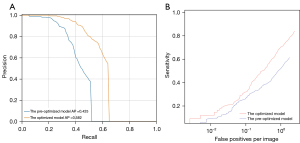
The detection rate of avulsion fracture by the optimized AI model was significantly higher than that by radiologists (57.89% vs. 29.82%, P=0.002), with 28.07% difference. For the non-avulsion fracture, there was no significant difference of detection rate between the optimized AI model and radiologists (P=0.853). The results are presented in Table 2 and Figure 6.
Table 2
Fracture type | Detection rate | t value | P value | |
---|---|---|---|---|
Radiologist (%) | Optimized AI (%) | |||
Avulsion fracture (n=57) | 29.82 | 57.89 | −3.066 | 0.002 |
Non-avulsion fracture (n=209) | 86.12 | 85.64 | 0.185 | 0.853 |
AI, artificial intelligence.
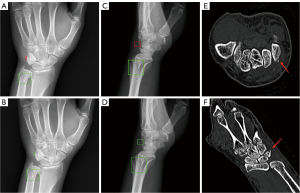
Case-based diagnostic efficacy
At the case level, the accuracy (86.40% vs. 71.93%, P<0.001) and sensitivity (87.29% vs. 73.48%, P<0.001) of the optimized AI were significantly higher than those of the pre-optimized AI model, with an increase of 14.47% and 13.81%, respectively, as shown in Table 3. There was no statistical difference in accuracy, sensitivity, and specificity between the optimized AI and the radiologists (P>0.05) (Table 4).
Table 3
Diagnostic efficacy | Pre-optimized AI (%) | Optimized AI (%) | t value | P value |
---|---|---|---|---|
Accuracy | 71.93 | 86.40 | −4.391 | <0.001 |
Sensitivity | 73.48 | 87.29 | −3.964 | <0.001 |
Specificity | 65.96 | 82.98 | −1.940 | 0.058 |
AI, artificial intelligence.
Table 4
Diagnostic efficacy | Radiologist (%) | Optimized AI (%) | t value | P value |
---|---|---|---|---|
Accuracy | 83.33 | 86.40 | −1.068 | 0.725 |
Sensitivity | 85.64 | 87.29 | −0.538 | 0.725 |
Specificity | 74.47 | 82.98 | −1.159 | 0.252 |
AI, artificial intelligence.
Discussion
The study used CT image-based fracture identification as the “gold standard” to label and train AI models, and then evaluated the diagnostic efficacy of the AI models in detecting avulsion fractures and non-avulsion fractures in multiple anatomical areas on DR images. The results found that the optimized AI model greatly improved the diagnostic performance for different anatomical areas and different types of extremity fractures compared to the pre-optimized AI. The optimized AI model improved the detection rate of avulsion fractures compared to radiologists (29.82% vs. 57.89%, P=0.002). Inagaki et al. reported that an AI model improved the detection rate of sacral fractures compared to specialists by labeling and training an AI model using the results of CT images (accuracy 93.5% vs. 55.3%) (18). This study demonstrated the feasibility of using AI for fracture detection.
This study used CT images as the gold standard to label radiographs for the training set. Most published datasets for automated fracture detection have been labeled by orthopedic surgeons (19), general radiologists (20-23), specialized musculoskeletal radiologists (20,21), or orthopedic specialists (16). Tobler et al. (14) used radiology reports to label distal radius fractures on radiographs for the training set. Another study showed that a considerable part of fractures were missed at the initial diagnosis (24). Since partial radiology reports and clinicians are susceptible to error, the data labeling based on reports had some level of inaccuracy. In contrast, the fracture areas were manually labeled by radiologists based on the visualization of lesions on the corresponding CT images in our study. A previous study suggested that subtle fractures are difficult to detect on plain films, and CT imaging may be required (25). The bounding boxes used to train, validate, and test the models were labeled according to each instance corresponding axial CT and 3-dimensional (3D) reconstruction images in our study; this method could harvest more accurate bounding boxes than expert-based data annotations. It is more difficult to identify subtle imaging findings for avulsion fractures than non-avulsion fractures, so CT localization for small fragments and translucent lines can make the labeling more accurate.
Olczak et al. (26) used a Visual Geometry Group (VGG)-16 network to identify fractures. Their dataset contained 256,000 hand, wrist, and ankle radiographs. The model detected fractures with accuracy of 83%. This study is considered the first to show that deep learning works for orthopedic radiographs. The VGG-1618 network is a CNN that classifies entire radiographs into fracture or nonfracture categories. This study used a ResNeXt-101 network and achieved an accuracy of 86.4% in diagnosing extremity fractures, which is higher than that of the study by Olczak et al. Our study also used the Mask-RCNN architecture (17), which is a proposal-based object detection CNN. The region proposal-based models mainly include the RCNN (27), Faster-RCNN (24), and Mask-RCNN (17). In a study performed by Wu et al. (28), the RCNN was used to identify fractures from hand, wrist, elbow, shoulder, pelvic, knee, ankle, and foot radiographs. In addition, a Feature Ambiguity Mitigate Operator (FAMO) model was introduced to mitigate feature ambiguity. This model finally achieved a sensitivity of 77.5% and a specificity of 93.4%. The Mask-RCNN method achieved a sensitivity of 87.29% in our study, which is higher than that in the study by Wu et al. Increased sensitivity is important to reduce the missed diagnosis for clinical practice.
The most important result of this study was the finding that the optimized AI model improved the detection of avulsion fractures, which has important implications for clinical practice given that avulsion fractures are easily missed. Published studies investigating deep learning approaches for fracture detection have focused on non-avulsion fractures, such as those of the hip (29,30), wrist (31), shoulder (9), and ankle (20). However, there have been few applications of deep learning for the detection of avulsion fractures. Ren et al. (13) developed and evaluated a 2-stage deep CNN system that mimics a radiologist’s search pattern for detecting 2 minor fractures: triquetral avulsion fractures and Segond fractures. The specially designed training program has greatly improved the detection rate of the minor fractures. In our study, the total detection rate of avulsion fractures at multiple anatomical areas by the optimized AI model was 57.89%, whereas the detection rate of radiologists was only 29.82%. These avulsion fractures were mostly misdiagnosed in the initial radiological reports, and were only identified by the optimized AI model. Therefore, the results of our study show that CNNs can be trained to improve the detection of avulsion fractures.
Our study had several limitations. Firstly, we concluded that CT-based labeling was superior to labeling based on a team of experts, but we did not further compare the differences between them. Secondly, selection of only examinations that had CT follow-up may have created spectrum bias toward more severe injuries, and the number of negative samples in the test set was relatively small, especially for the hand bones; more multi-center data in the future are required for testing our model. Thirdly, our radiology reports were written by radiology residents and radiologists with varying expertise in musculoskeletal imaging and were unstructured, which may have influenced the diagnostic results. Finally, whether this AI will improve the ability of professionals to diagnose fractures needs to be tested further in clinical practice.
Conclusions
In conclusion, this study showed that the optimized AI model significantly improved the diagnostic efficacy of extremity fractures compared with the pre-optimized AI model, and the optimized AI model significantly outperformed the radiologists in detecting avulsion fractures. Therefore, the AI model trained on CNN with CT-based labels to detect extremity fractures (especially avulsion fractures that are easily missed) on radiographs are suitable as an assisted reading tool.
Acknowledgments
Funding: None.
Footnote
Reporting Checklist: The authors have completed the STARD reporting checklist. Available at https://qims.amegroups.com/article/view/10.21037/qims-23-428/rc
Conflicts of Interest: All authors have completed the ICMJE uniform disclosure form (available at https://qims.amegroups.com/article/view/10.21037/qims-23-428/coif). HC, YY and XL are employees of DeepWise AI Lab Company. The other authors have no conflicts of interest to declare.
Ethical Statement: The authors are accountable for all aspects of the work in ensuring that questions related to the accuracy or integrity of any part of the work are appropriately investigated and resolved. The study was conducted in accordance with the Declaration of Helsinki (as revised in 2013). The study was approved by the China-Japan Friendship Hospital Institutional Review Board (Medical ethics number: 2019-94-k62-1) and the requirement for individual consent for this retrospective analysis was waived.
Open Access Statement: This is an Open Access article distributed in accordance with the Creative Commons Attribution-NonCommercial-NoDerivs 4.0 International License (CC BY-NC-ND 4.0), which permits the non-commercial replication and distribution of the article with the strict proviso that no changes or edits are made and the original work is properly cited (including links to both the formal publication through the relevant DOI and the license). See: https://creativecommons.org/licenses/by-nc-nd/4.0/.
References
- DiMaggio CJ, Avraham JB, Lee DC, Frangos SG, Wall SP. The Epidemiology of Emergency Department Trauma Discharges in the United States. Acad Emerg Med 2017;24:1244-56. [Crossref] [PubMed]
- Chen W, Lv H, Liu S, Liu B, Zhu Y, Chen X, Yang G, Liu L, Zhang T, Wang H, Yin B, Guo J, Zhang X, Li Y, Smith D, Hu P, Sun J, Zhang Y. National incidence of traumatic fractures in China: a retrospective survey of 512 187 individuals. Lancet Glob Health 2017;5:e807-17. [Crossref] [PubMed]
- Haraguchi N, Toga H, Shiba N, Kato F. Avulsion fracture of the lateral ankle ligament complex in severe inversion injury: incidence and clinical outcome. Am J Sports Med 2007;35:1144-52. [Crossref] [PubMed]
- Petinaux B, Bhat R, Boniface K, Aristizabal J. Accuracy of radiographic readings in the emergency department. Am J Emerg Med 2011;29:18-25. [Crossref] [PubMed]
- Pinto A, Reginelli A, Pinto F, Lo Re G, Midiri F, Muzj C, Romano L, Brunese L. Errors in imaging patients in the emergency setting. Br J Radiol 2016;89:20150914. [Crossref] [PubMed]
- Jena AB, Seabury S, Lakdawalla D, Chandra A. Malpractice risk according to physician specialty. N Engl J Med 2011;365:629-36. [Crossref] [PubMed]
- Bernstein J. Malpractice: Problems and solutions. Clin Orthop Relat Res 2013;471:715-20. [Crossref] [PubMed]
- Kim DH, MacKinnon T. Artificial intelligence in fracture detection: transfer learning from deep convolutional neural networks. Clin Radiol 2018;73:439-45. [Crossref] [PubMed]
- Chung SW, Han SS, Lee JW, Oh KS, Kim NR, Yoon JP, Kim JY, Moon SH, Kwon J, Lee HJ, Noh YM, Kim Y. Automated detection and classification of the proximal humerus fracture by using deep learning algorithm. Acta Orthop 2018;89:468-73. [Crossref] [PubMed]
- Urakawa T, Tanaka Y, Goto S, Matsuzawa H, Watanabe K, Endo N. Detecting intertrochanteric hip fractures with orthopedist-level accuracy using a deep convolutional neural network. Skeletal Radiol 2019;48:239-44. [Crossref] [PubMed]
- Ni M, Zhao Y, Wen X, Lang N, Wang Q, Chen W, Zeng X, Yuan H. Deep learning-assisted classification of calcaneofibular ligament injuries in the ankle joint. Quant Imaging Med Surg 2023;13:80-93. [Crossref] [PubMed]
- Yin P, Wang W, Wang S, Liu T, Sun C, Liu X, Chen L, Hong N. The potential for different computed tomography-based machine learning networks to automatically segment and differentiate pelvic and sacral osteosarcoma from Ewing's sarcoma. Quant Imaging Med Surg 2023;13:3174-84. [Crossref] [PubMed]
- Ren M, Yi PH. Deep learning detection of subtle fractures using staged algorithms to mimic radiologist search pattern. Skeletal Radiol 2022;51:345-53. [Crossref] [PubMed]
- Tobler P, Cyriac J, Kovacs BK, Hofmann V, Sexauer R, Paciolla F, Stieltjes B, Amsler F, Hirschmann A. AI-based detection and classification of distal radius fractures using low-effort data labeling: evaluation of applicability and effect of training set size. Eur Radiol 2021;31:6816-24. [Crossref] [PubMed]
- Mutasa S, Varada S, Goel A, Wong TT, Rasiej MJ. Advanced Deep Learning Techniques Applied to Automated Femoral Neck Fracture Detection and Classification. J Digit Imaging 2020;33:1209-17. [Crossref] [PubMed]
- Lindsey R, Daluiski A, Chopra S, Lachapelle A, Mozer M, Sicular S, Hanel D, Gardner M, Gupta A, Hotchkiss R, Potter H. Deep neural network improves fracture detection by clinicians. Proc Natl Acad Sci U S A 2018;115:11591-6. [Crossref] [PubMed]
- He K, Gkioxari G, Dollar P, Girshick R. Mask R-CNN. IEEE Trans Pattern Anal Mach Intell 2020;42:386-97. [Crossref] [PubMed]
- Inagaki N, Nakata N, Ichimori S, Udaka J, Mandai A, Saito M. Detection of Sacral Fractures on Radiographs Using Artificial Intelligence. JB JS Open Access 2022.
- Gan K, Xu D, Lin Y, Shen Y, Zhang T, Hu K, Zhou K, Bi M, Pan L, Wu W, Liu Y. Artificial intelligence detection of distal radius fractures: a comparison between the convolutional neural network and professional assessments. Acta Orthop 2019;90:394-400. [Crossref] [PubMed]
- Choi JW, Cho YJ, Lee S, Lee J, Lee S, Choi YH, Cheon JE, Ha JY. Using a Dual-Input Convolutional Neural Network for Automated Detection of Pediatric Supracondylar Fracture on Conventional Radiography. Invest Radiol 2020;55:101-10. [Crossref] [PubMed]
- Kitamura G, Chung CY, Moore BE 2nd. Ankle Fracture Detection Utilizing a Convolutional Neural Network Ensemble Implemented with a Small Sample, De Novo Training, and Multiview Incorporation. J Digit Imaging 2019;32:672-7. [Crossref] [PubMed]
- Blüthgen C, Becker AS, Vittoria de Martini I, Meier A, Martini K, Frauenfelder T. Detection and localization of distal radius fractures: Deep learning system versus radiologists. Eur J Radiol 2020;126:108925. [Crossref] [PubMed]
- Thian YL, Li Y, Jagmohan P, Sia D, Chan VEY, Tan RT. Convolutional Neural Networks for Automated Fracture Detection and Localization on Wrist Radiographs. Radiol Artif Intell 2019;1:e180001. [Crossref] [PubMed]
- Ren S, He K, Girshick R, Sun J. Faster R-CNN: Towards Real-Time Object Detection with Region Proposal Networks. IEEE Trans Pattern Anal Mach Intell 2017;39:1137-49. [Crossref] [PubMed]
- Hallas P, Ellingsen T. Errors in fracture diagnoses in the emergency department--characteristics of patients and diurnal variation. BMC Emerg Med 2006;6:4.
- Olczak J, Fahlberg N, Maki A, Razavian AS, Jilert A, Stark A, Sköldenberg O, Gordon M. Artificial intelligence for analyzing orthopedic trauma radiographs. Acta Orthop 2017;88:581-6. [Crossref] [PubMed]
- Girshick R, Donahue J, Darrell T, Malik J. Region-Based Convolutional Networks for Accurate Object Detection and Segmentation. IEEE Trans Pattern Anal Mach Intell 2016;38:142-58. [Crossref] [PubMed]
- Wu HZ, Yan LF, Liu XQ, Yu YZ, Geng ZJ, Wu WJ, Han CQ, Guo YQ, Gao BL. The Feature Ambiguity Mitigate Operator model helps improve bone fracture detection on X-ray radiograph. Sci Rep 2021;11:1589. [Crossref] [PubMed]
- Oakden-Rayner L, Gale W, Bonham TA, Lungren MP, Carneiro G, Bradley AP, Palmer LJ. Validation and algorithmic audit of a deep learning system for the detection of proximal femoral fractures in patients in the emergency department: a diagnostic accuracy study. Lancet Digit Health 2022;4:e351-8.
- Badgeley MA, Zech JR, Oakden-Rayner L, Glicksberg BS, Liu M, Gale W, McConnell MV, Percha B, Snyder TM, Dudley JT. Deep learning predicts hip fracture using confounding patient and healthcare variables. NPJ Digit Med 2019;2:31. [Crossref] [PubMed]
- Yahalomi E, Chernofsky M, Werman M. Detection of distal radius fractures trained by a small set of X-ray images and Faster R-CNN. In: Arai K, Bhatia R, Kapoor S. editors. Intelligent Computing. Proceedings of the 2019 Computing Conference, Volume 1. Springer International Publishing; 2019:971-981.