Prognostic prediction of left ventricular myocardial noncompaction using machine learning and cardiac magnetic resonance radiomics
Introduction
Left ventricular noncompaction (LVNC) is a rare but widely recognized condition characterized by prominent trabeculations on the luminal surface of the ventricle, deep intertrabecular recesses communicating with the ventricular cavity, and a thin compacted myocardial layer (1). LVNC is remarkably heterogeneous in terms of its causes, clinical presentation, morphology, and prognosis. Despite several published studies on LVNC, its prognosis remains unclear, as many patients have a favorable prognosis (2,3); meanwhile, others may experience progression, with heart failure (HF), ventricular arrhythmias (VAs), and systemic embolisms (SEs) being the most frequent cardiovascular complications (4), and many even die (mortality rate 13.2–48%) (5,6). Although these potential adverse outcomes and factors that indicate illness severity and clinical outcomes have been well described, there remains an urgent need to develop predictive models that can identify and stratify patients with LVNC at risk of major adverse cardiovascular events (MACEs; including HF, VA, SE, and all-cause death) to thus facilitate the management of these patients.
Recent research into imaging analysis technology and artificial intelligence has made remarkable progress in a variety of medical fields, and applications for building discriminative models have been reported (7-10). Radiomics is an emerging analytical method that can extract quantitative pixel-level features from routinely acquired medical images. As the approach can obtain multiple quantifiers of tissue features with no need for additional image acquisitions or changes in protocol, radiomics shows great potential for improving diagnosis, prognosis, and clinical decision-making (11). In addition, an ensemble machine learning framework, which can reduce the bias in a single machine learning algorithm, may help to improve the predictive performance of radiomics methods (12). Cardiac magnetic resonance (CMR) is frequently used for the measurement of left ventricular (LV) function and the identification and assessment of the extent of trabeculations; it outperforms echocardiography in the precise and comprehensive assessment of the heart owing to its higher spatial resolution and better field of view (13). Applications of radiomics based on cine CMR sequences have been reported for the diagnosis of acute, subacute, and chronic myocardial infarction (14,15) and etiologies of LV hypertrophy (16). However, to the best of our knowledge, the performance of cine CMR-based radiomics in predicting MACEs in patients with LVNC has not thus far been examined.
In this study, we aimed to extract and select radiomics features derived from cine CMR images and develop a predictive model using machine learning to predict MACEs in patients with LVNC. We further sought to develop and validate a combined model for predicting MACEs comprising the selected radiomics features and clinical factors to better stratify patients with LVNC.
Methods
This study was conducted in accordance with the Declaration of Helsinki (as revised in 2013) and was approved by the Biomedical Research Ethics Committee of the West China Hospital of Sichuan University (No. 2022-1190). Individual consent for this retrospective analysis was waived. All personal information was kept strictly confidential and only used for research purposes. The study workflow included data acquisition, image segmentation, feature extraction, feature analysis, model construction, and model validation (see Figure 1).
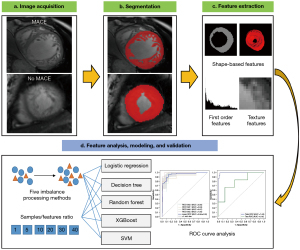
Patient profiles and CMR image acquisition and analysis
We retrospectively screened 129 consecutive patients with LVNC who had undergone CMR from May 2013 to May 2021. LVNC was diagnosed using the Petersen criteria (17). The exclusion criteria were as follows: congenital heart disease; severe valvular disease requiring surgical intervention; known coexisting acquired cardiomyopathy; age <12 years, pregnant women, or athletes; estimated glomerular filtration rate (eGFR) <30 mL/min; and poor image quality. Participants were followed up for MACEs, including HF, VA, SE, and all-cause death (see Table S1 for full details). The follow-up involved clinical visits or telephone interviews. The follow-up duration was not less than 2 months and was calculated as the date of CMR examination to the occurrence of an MACE or the last follow-up without an MACE. Of the 105 patients remaining after the application of the exclusion criteria, 9 were excluded due to being lost to follow-up (>2 months).
The 96 patients included in this study were divided into 2 cohorts, training and validation, in a ratio of 8:2 via simple randomization to construct and validate models, respectively. Thus, 76 patients (44 males and 32 females; mean age 37.12±14.18 years) were enrolled in the training cohort, including 61 non-MACE patients and 15 MACE patients; meanwhile, 20 patients (14 males and 6 females; mean age 38.20±16.68 years) were enrolled in the validation cohort, including 14 non-MACE patients and 6 MACE patients. The patients’ demographic characteristics and clinical data were collected from their electronic medical records. Medical treatments were performed by medical professionals according to the relevant clinical guidelines (18,19). The details of the CMR protocol and CMR image analysis are presented in Appendix 1.
Image segmentation
Segmentation was performed on serial short-axis slices at end diastole by 2 investigators with more than 3 years of CMR experience in consensus. A region of interest (ROI) of the LV myocardium (including trabeculations and excluding papillary muscles) was manually segmented using the pencil tool in ITK-SNAP software (http://www.itksnap.org). Papillary muscles were treated as trabeculation when they were indistinguishable from trabeculation.
To evaluate the interobserver reproducibility of the radiomics features, 2 radiologists (readers 1 and 2) with at least 3 years of cardiology experience who were blinded to the patients’ information independently performed the segmentations. To assess the intraobserver reproducibility of the radiomics features, reader 1 performed a second segmentation 1 month later.
Radiomics feature extraction
Image normalization was implemented to eliminate the differences between cine CMR image signals, with a normalized scale of 100. Precropping was performed to reduce the memory footprint, and the bin size was 20. The details of the related parameters and features can be viewed online (https://pyradiomics.readthedocs.io/en/latest/index.html). Three-dimensional (3D) radiomics analysis was chosen, as it provides more comprehensive information than does the 2-dimensional (2D) approach. The radiomics features calculated from 8 to 12 short-axis cine images for each patient were introduced together. Following this, 105 quantitative radiomics features were extracted from each volume of interest (VOI) using Pyradiomics (version 3.0.1; https://pyradiomics.readthedocs.io) and SimpleITK (version 2.0.0; https://simpleitk.org). The detailed features are provided in Table S2. The reproducibility of the extracted radiomics features was based on VOI delineation in interobserver and intraobserver comparisons. In addition, we compared the radiomic features of the 2 different magnetic resonance scanners.
Data analysis strategy
The class imbalance of training samples (non-MACE:MACE ratio: 61:15) would have affected the processing effect of machine learning algorithms. To resolve this issue, we first balanced the MACE data in the training cohort by using oversampled algorithms to synthesize new MACE samples based on interpolation. The non-MACE and MACE samples were then balanced to a ratio of approximately 61:61 in the generated dataset, which served as the final training cohort. Finally, feature analysis and machine learning modeling were implemented in the training cohort, and their diagnostic performance was evaluated in the validation cohort. A radiomics model and clinical model with the top 12 importance-ranked radiomics or clinical features were developed. We also built a combined radiomics-clinical combined model by integrating valuable radiomics features and clinical features. The performance of the prediction models was compared.
Feature analysis, modeling, and validation
We compared 5 oversampling algorithms: the synthetic minority oversampling technique (SMOTE), adaptive synthetic (ADASYN) algorithm, borderline SMOTE, KMeans SMOTE, and support vector machine (SVM) SMOTE. We then used the extreme gradient boosting (XGBoost) algorithm to repeatedly build models and assess the importance of each feature. Previous studies have shown that each feature needs at least 10 samples that can yield reasonably stable estimates (11,20,21). To determine the optimal sample:feature ratio, we implemented 5 different sample:feature ratios (1, 5, 10, 20, 30, and 40) and compared their results. To identify the best-fitting method, the 5 most commonly used methods in the radiomics studies were adopted: logistic regression, decision tree, random forest, XGBoost, and SVM algorithms. Through these experiments, the optimal machine learning pipeline was determined.
Finally, we built the machine learning pipeline by using Python Jupyter Lab (version 3.0.14; https://jupyter.org) for further analysis. Based on the selected radiomics features, clinical features, and their combinations, the radiomics model, clinical model, and combined model were built using 4-fold cross-validation in the training cohort and evaluated in the validation cohort. The receiver operating characteristic (ROC) curves of the 3 models were plotted, and the area under the receiver operating characteristic curve (AUC), sensitivity, specificity, accuracy, precision and F1 score of each were calculated. The point with the largest Youden index (equal to sensitivity + specificity − 1), was defined as the optimal cutoff on each ROC curve. The reported values of sensitivity and specificity were those at the best cutoff point.
Statistical analysis
Statistical analyses were conducted in R (version 3.5.1; https://www.r-project.org) with RStudio (version 1.0.136, https://www.rstudio.com). The machine learning algorithms were implemented using Python (version 3.6.6, https://www.python.org). The Kolmogorov-Smirnov test was used to assess the normality of the distributions of the variables. Normally distributed continuous variables are presented as the mean ± standard deviation, and nonnormally distributed continuous variables are presented as the median with interquartile range (IQR). Categorical variables are presented as frequency (percentage). Student t-, Mann-Whitney, and the chi-squared tests were conducted, as appropriate. The intraclass correlation coefficient (ICC) was calculated to evaluate feature repeatability. ICC values were categorized as poor (ICC <0.40), fair (ICC =0.40–0.59), good (ICC =0.60–0.74), and excellent (ICC =0.75–1.0). Pearson correlation coefficient (r) was calculated between clinical variables and radiomics variables and was evaluated as follows: |r| ≤0.2, no correlation; 0.2< |r| ≤0.4, weak correlation; 0.4< |r| ≤0.6, moderate correlation; 0.6< |r| ≤0.8, strong correlation; and 0.8< |r| ≤1.0, excellent correlation. The AUC was categorized as follows: AUC =0.5, no discrimination; 0.5≤ AUC <0.7, poor discrimination; 0.7≤ AUC <0.8, acceptable discrimination; 0.8≤ AUC <0.9, excellent discrimination; and AUC ≥0.9, outstanding discrimination. The DeLong test was used to assess the difference between ROC curves. P<0.05 indicated statistical significance.
Results
Patient characteristics
The median time to an MACE was 30.6 (IQR, 11.7–51.3) months. MACEs occurred in 21 (21.88%) patients, including 11 (11.46%) cases of HF, 6 (6.25%) of VAs, and 4 (4.17%) of all-cause death (Table S1). The incidence of MACEs was not significantly different between the training and validation cohorts (training: n=15, 19.7%; validation: n=6, 30.0%; P=0.494). The baseline patient characteristics and CMR findings in the 2 cohorts are shown in Tables 1,2, respectively.
Table 1
Characteristics | Training cohort (n=76) | Validation cohort (n=20) | |||||
---|---|---|---|---|---|---|---|
Non-MACE (n=61) |
MACE (n=15) | P | Non-MACE (n=14) |
MACE (n=6) | P | ||
Age (years) | 34.00 (27.00, 46.00) |
46.00 (29.00, 55.00) |
0.083 | 34.50 (29.25, 40.00) |
48.00 (20.75, 69.25) |
0.141 | |
Sex | 0.564 | 1.000 | |||||
Female | 27 (44.3) | 5 (33.3) | 4 (28.6) | 2 (33.3) | |||
Male | 34 (55.7) | 10 (66.7) | 10 (71.4) | 4 (66.7) | |||
Family history of cardiomyopathy | 2 (3.3) | 0 (0.0) | 1.000 | 0 (0.0) | 1 (16.7) | 0.300 | |
Hypertension | 3 (4.9) | 2 (13.3) | 0.254 | 2 (14.3) | 3 (50.0) | 0.131 | |
Dyslipidemia | 2 (3.3) | 0 (0.0) | 1.000 | 1 (7.1) | 1 (16.7) | 0.521 | |
Diabetes mellitus | 3 (4.9) | 0 (0.0) | 1.000 | 1 (7.1) | 2 (33.3) | 0.202 | |
Smoking | 13 (21.3) | 5 (33.3) | 0.521 | 4 (28.6) | 2 (33.3) | 1.000 | |
BMI (kg/m2) | 23.19 (20.15, 26.33) |
22.03 (20.03, 26.45) |
0.749 | 22.70 (19.85, 25.63) |
20.23 (18.76, 23.00) |
0.269 | |
Symptoms on admission | |||||||
Baseline NYHA functional class | 0.002 | 0.034 | |||||
I | 45 (73.8) | 6 (40.0) | 12 (85.7) | 2 (33.3) | |||
II | 6 (9.8) | 1 (6.7) | 0 (0.0) | 1 (16.7) | |||
III | 8 (13.1) | 3 (20.0) | 0 (0.0) | 2 (33.3) | |||
IV | 2 (3.3) | 5 (33.3) | 2 (14.3) | 1 (16.7) | |||
Stroke or systemic embolization | 1 (1.6) | 1 (6.7) | 0.358 | 1 (7.1) | 0 (0.0) | 1.000 | |
Arrhythmias | 10 (16.4) | 4 (26.7) | 0.584 | 0 (0.0) | 2 (33.3) | 0.079 | |
Cardiomyopathy phenotype | |||||||
Dilated phenotype | 16 (26.2) | 5 (33.3) | 0.819 | 7 (50.0) | 3 (50.0) | 1.000 | |
Hypertrophic phenotype | 1 (1.6) | 3 (20.0) | 0.023 | 0 (0.0) | 1 (16.7) | 0.300 | |
Restrictive phenotype | 1 (1.6) | 0 (0.0) | 1.000 | 0 (0.0) | 0 (0.0) | NA | |
Arrhythmogenic phenotype | 0 (0.0) | 1 (6.7) | 0.197 | 0 (0.0) | 0 (0.0) | NA | |
Co-existing RVNC | 8 (13.1) | 3 (20.0) | 0.788 | 1 (7.1) | 1 (16.7) | 0.521 | |
Medical therapy | |||||||
Beta-blocker | 19 (31.1) | 5 (33.3) | 1.000 | 3 (21.4) | 3 (50.0) | 0.303 | |
Angiotensin-converting enzyme inhibitor | 5 (8.2) | 0 (0.0) | 0.576 | 0 (0.0) | 1 (16.7) | 0.300 | |
Angiotensin receptor blockers | 10 (16.4) | 2 (13.3) | 1.000 | 4 (28.6) | 1 (16.7) | 1.000 | |
Diuretics | 16 (26.2) | 6 (40.0) | 0.462 | 4 (28.6) | 4 (66.7) | 0.161 | |
Ivabradine | 2 (3.3) | 1 (6.7) | 0.488 | 1 (7.1) | 0 (0.0) | 1.000 | |
Calcium antagonists | 0 (0.0) | 0 (0.0) | NA | 0 (0.0) | 1 (16.7) | 0.300 | |
Statins | 2 (3.3) | 0 (0.0) | 1.000 | 1 (7.1) | 1 (16.7) | 0.521 | |
Amiodarone | 3 (4.9) | 2 (13.3) | 0.254 | 0 (0.0) | 0 (0.0) | NA | |
Class 1C antiarrhythmic | 1 (1.6) | 0 (0.0) | 1.000 | 0 (0.0) | 0 (0.0) | NA | |
Oral anticoagulant therapy | 3 (4.9) | 3 (20.0) | 0.160 | 0 (0.0) | 0 (0.0) | NA |
Data are presented as the median with interquartile range and n (%). MACE, major adverse cardiovascular event; BMI, body mass index; NYHA, New York Heart Association; NA, not applicable; RVNC, right ventricular noncompaction.
Table 2
CMR variable | Training cohort (n=76) | Validation cohort (n=20) | |||||
---|---|---|---|---|---|---|---|
Non-MACE (n=61) | MACE (n=15) | P | Non-MACE (n=14) | MACE (n=6) | P | ||
LVEF (%) | 47.36 (21.84, 57.59) | 22.10 (16.80, 34.60) | 0.010 | 44.36 (27.97, 55.07) | 13.01 (10.48, 17.01) | 0.001 | |
LVEDV (mL) | 176.87 (125.20, 279.53) | 313.00 (182.90, 390.60) | 0.016 | 199.71 (155.80, 283.37) | 313.81 (258.80, 424.90) | 0.016 | |
LVESV (mL) | 89.50 (59.86, 200.77) | 273.60 (116.10, 299.30) | 0.011 | 115.90 (68.44, 221.16) | 272.71 (216.33, 381.40) | 0.003 | |
LVSV (mL/m2) | 68.80 (58.50, 89.80) | 59.20 (40.60, 77.50) | 0.251 | 78.57 (67.46, 96.96) | 42.35 (39.52, 48.82) | 0.002 | |
LVMI (g/m2) | 52.98 (41.08, 70.47) | 76.09 (68.99, 105.36) | 0.001 | 62.34 (47.65, 74.09) | 102.62 (62.13, 136.01) | 0.013 | |
Maximal NC:C ratio (%) | 3.55 (2.77, 4.79) | 4.57 (2.73, 6.69) | 0.273 | 3.25 (2.77, 3.75) | 4.67 (3.20, 6.21) | 0.141 | |
Number of noncompacted segments | 9.00 (5.00, 12.00) | 11.00 (7.00, 13.00) | 0.614 | 9.50 (6.00, 11.00) | 12.00 (9.00, 15.00) | 0.205 | |
RV abnormalities (low EF, dilation) | 28 (45.9) | 12 (80.0) | 0.018 | 5 (35.7) | 3 (50.0) | 0.642 | |
LGE | 18 (29.5) | 12 (80.0) | <0.001 | 5 (35.7) | 4 (66.7) | 0.336 |
Data are presented as the median with interquartile range and n (%). CMR, cardiac magnetic resonance; MACE, major adverse cardiovascular event; LVEF, left ventricular ejection fraction; LVEDV, left ventricular end diastolic volume; LVESV, left ventricular end systolic volume; LVSV, left ventricular stroke volume; LVMI, left ventricular mass index; NC:C, noncompacted to compacted ratio; RV, right ventricular; EF, ejection fraction; LGE, late gadolinium enhancement.
The machine learning pipeline
Figure 2 shows the performance of the various machine learning models (mean AUC) when different oversampling methods were used in the training and validation cohorts. The diagnostic performance was mostly improved via the oversampling technique. The ADASYN algorithm showed the best performance, and ADASYN + SVM modeling had the highest AUC in both the training (AUC =0.97) and validation cohorts (AUC =0.87).
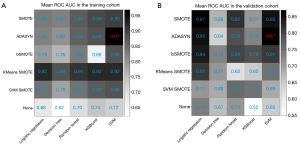
Figure 3 shows the performance of the mean AUC for various machine learning models when different sample:feature ratios were used in the training and validation cohorts. The predictive performance was best when the sample:feature ratio was 10, which is consistent with findings from previous research (11).
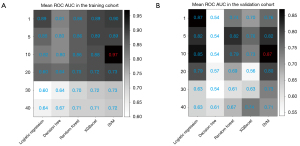
Therefore, the final machine learning pipeline included ADASYN imbalanced processing, XGBoost feature selection with a sample:feature ratio of 10, and SVM modeling. The algorithm details are provided in Table S3.
Feature analysis
The 12 most important radiomics features and 12 most important clinical features were selected for further modeling (Figure 4A,4B). Among all 12 radiomics features, first-order features (n=3), shape-based features (n=3), and gray-level size-zone matrix (GLSZM) features (n=3) had greater importance. Among all clinical variables, the top 5 most important factors were as follows: age, left ventricular mass index (LVMI), body mass index (BMI), maximal noncompacted to compacted (NC:C) ratio, and presence of LV late gadolinium enhancement (LGE).
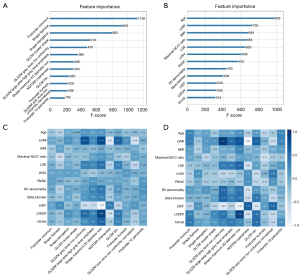
Figure 4C,4D display the correlations between the selected clinical factors and radiomics features in the training and validation cohorts. In the training cohort, LVMI showed strong correlations with GLSZM gray-level nonuniformity (r=0.73), shape maximum 2D diameter row (r=0.79), neighboring gray-tone difference matrix (NGTDM) coarseness (r=−0.62), and gray-level co-occurrence matrix inverse difference normalized (GLCM Idn) (r=0.6). Left ventricular ejection fraction (LVEF) had a strong correlation with the shape maximum of the 2D diameter row (r=−0.77), and left ventricular end diastolic volume (LVEDV) showed an excellent correlation with the shape of the maximum 2D diameter row (r=0.85). In the validation cohort, LVMI had an excellent correlation with GLSZM gray-level nonuniformity (r=0.9) and NGTDM coarseness (r=−0.83), and a strong correlation with the shape maximum of the 2D diameter row (r=0.7) and GLCM Idn (r=0.6). LVEF had strong correlation with the shape of maximum 2D diameter row (r=−0.79) and NGTDM coarseness (r=0.61). LVEDV showed excellent correlation with the shape of the maximum 2D diameter row (r=0.88).
The intraobserver (ICC: 0.934–1.000) and interobserver (ICC: 0.917–0.999) reproducibility for the 12 most important radiomics features was excellent (Table S4). In addition, significant differences in several radiomics features were found between different magnetic resonance scanners (all P values <0.05) (Table S5).
Radiomics, clinical, and combined radiomics-clinical feature models
Based on the top 12 most important radiomics features, clinical features, and their combinations, the radiomics model, clinical model, and combined model were constructed via 4-fold cross-validation in the training cohort and validated in the validation cohort (Figure 5). Details of the machine learning pipeline and its parameters are shown in Table S3. The detailed diagnostic performances of the radiomics, clinical, and combined models are shown in Table 3.
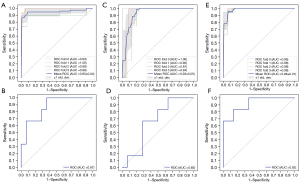
Table 3
Models | AUC | Sensitivity (%) | Specificity (%) | Accuracy (%) | Precision (%) | F1 score |
---|---|---|---|---|---|---|
Radiomics model | ||||||
Training | 0.97 | 100.00 | 70.49 | 76.32 | 45.45 | 0.63 |
Validation | 0.87 | 83.33 | 64.29 | 70.00 | 50.00 | 0.63 |
Clinical model | ||||||
Training | 0.97 | 100.00 | 91.80 | 93.42 | 75.00 | 0.86 |
Validation | 0.65 | 16.67 | 78.57 | 60.00 | 25.00 | 0.20 |
Combined model | ||||||
Training | 0.99 | 93.33 | 93.44 | 93.42 | 77.78 | 0.85 |
Validation | 0.92 | 33.33 | 100.00 | 80.00 | 100.0 | 0.50 |
MACE, major adverse cardiovascular event; AUC, area under the receiver operating characteristic curve.
The radiomics model yielded an AUC of 0.97 (sensitivity 100.00%, specificity 70.49%) in the training cohort and an AUC of 0.87 (sensitivity 83.33%, specificity 64.29%) in the validation cohort. For the clinical model, the AUC was 0.97 (sensitivity 100.00%, specificity 91.80%) in the training cohort and 0.65 (sensitivity 16.67%, specificity 78.57%) in the validation cohort. The combined model achieved an AUC of 0.99 (sensitivity 93.33%, specificity 93.44%) in the training cohort and an AUC of 0.92 (sensitivity 33.33%, specificity 100.00%) in the validation cohort.
The combined radiomics-clinical feature model showed the best performance, with the highest predictive accuracy. There was no significant difference in the performance of the radiomics model and the clinical model or the combined model (P=0.124 and P=0.621, respectively). The performance (AUC) of the combined model was significantly better than that of the clinical model (P=0.003).
Discussion
In this study, we assessed cine CMR-derived radiomics features and clinical risk factors using XGBoost and SVM algorithms in predicting MACEs in patients with LVNC. Our results showed the following: (I) radiomics analysis is feasible on cine CMR images, and the machine learning radiomics model performed considerably well in MACE prediction for patients with LVNC; (II) the combined radiomics-clinical feature model, integrating the radiomics features and clinical variables, showed a more competitive discrimination performance than did the clinical model. The combined model may facilitate personalized risk stratification and improve treatment decision-making for patients with LVNC.
Patients with LVNC experience different disease courses: many patients have a good prognosis, but some patients have an unfavorable prognosis. Therefore, identifying patients at risk for MACEs may be useful for guiding the frequency of clinical follow-up as well as the timing of interventions. Several studies (3,22-24) have explored predictors of poor outcomes, such as LVEF, presence of LGE, LV end-diastolic dimension, LV posterior wall compaction, decreased strains, and HF at diagnosis, and reports on prognostic factors and a poor outcome of LVNC are numerous, but their indicators are varied and not entirely consistent. There is thus a need to establish models to predict poor outcomes in patients with LVNC, given the heterogeneities of their various risk factors.
Casas et al. (25) designed a risk score model using Cox regression based on variables [including age, sex, cardiovascular risk factors, abnormal electrocardiography signs, LVEF, and noncompaction cardiomyopathy (LVEF <50% and/or family aggregation)] associated with MACEs to improve the risk stratification of patients with LVNC, which showed good discrimination between score tertiles. In our study, using a machine learning pipeline, we found that conventional cardiac structure and function parameters (LVMI, LGE, LVSV, RV abnormality, LVEF, and LVEDV), myocardial morphology assessed with CMR (maximal NC:C ratio, and right ventricular noncompaction), and clinical characteristics (age, BMI, beta-blocker usage, and baseline New York Heart Association functional class) constituted important values in the classification process. However, the clinical model showed poor discriminative performance. This indicates that clinical factors alone are insufficiently predictive and that additional effective tools are needed to predict MACEs in patients with LVNC.
Radiomics has shown potential as a noninvasive and quantitative tool in diagnosis and prognosis via the extraction of effective imaging features (26-28). Izquierdo et al. (29) demonstrated the value of machine learning-based CMR radiomics in the differential diagnosis of LVNC, hypertrophic cardiomyopathy, and dilated cardiomyopathy. Our study further explored the ability of radiomics to risk-stratify patients with LVNC, and our results showed that the radiomics model using nonenhanced cine CMR images had good discriminative capacity comparable to that of the clinical model. Since CMR is becoming more commonly used for the clinical assessment of LVNC and cine imaging is a routine sequence in clinical practice (30), supplemental radiomics analyses could be easily implemented. In this study, although adding radiomics features improved the sensitivity of clinical factors alone to some extent, the sensitivity of both the clinical and combined models was low. This may be due to the low sensitivity of the clinical model itself. However, the combined model had high specificity and accuracy, demonstrating the potential of the model to predict MACEs. In addition, consistent with some radiomics studies (31,32), we found a strong correlation between the selected radiomics features extracted from cine CMR images and the conventional CMR-derived indicator, suggesting the biological interpretability of radiomics features. We also observed several differences in the radiomics features between different magnetic resonance scanners, which was consistent with Lee et al.’s study (33). Further studies are warranted to reduce or avoid this difference to minimize its impact on quantitative analyses.
In our study, the final machine learning pipeline was ADASYN processing, XGBoost feature selection, and SVM modeling. The SMOTE algorithm and SMOTE-based extensions are mature data-imbalance processing algorithms (34) that show good performance in biomedical data analysis (35-37). ADASYN can generate balanced samples depending on the estimate of the local distribution of the class, so it is suitable for generating harder-to-learn examples. In addition, SMOTE, bSMOTE, and KMeans SMOTE also demonstrated good performance, which can provide a reference for other imbalanced biomedical data processing. A recent study (38) reported that machine learning mining results using the SMOTE method were largely consistent with the baseline patterns or trends, and synthetic data generated using machine learning have shown advantages in clinical modeling (39). XGBoost is a novel tree-based algorithm for sparse data processing that provides the gradient-boosted decision tree, which is widely recognized in data mining challenges and tasks (40,41). Some studies (42,43) have indicated that XGBoost performs better than do deep learning methods in tabular data analysis. The optimal sample:feature ratio is 10, which may be a universal conclusion in radiomics research. Our results also showed that the predictive performance was best and most robust under different conditions when the sample:feature ratio was 10. When the ratio approaches 1, almost all features are incorporated into machine learning modeling without selection. Most irrelevant and redundant features will hinder the learning effect as noise. When the ratio is very large, this is equivalent to only a few features being selected for modeling and may lack sufficiently useful information. Our study also tested multiple modeling approaches, and the comparisons of these modeling methods showed that SVM, which maximizes the separating margin (44,45), was the most powerful classifier with high accuracy and robustness.
Our results further indicated that the combined model, which integrated radiomics features and clinical risk factors, achieved a better prediction of MACEs than did the clinical model alone. These findings suggest that the combination of quantitative cine CMR image-based radiomics features with clinical characteristics can maximize the predictive performance for MACEs. This clinical parameter plus radiomics and machine learning pipeline may aid clinicians in deciding upon the appropriate management and early intervention for patients with LVNC. These results also indicate that radiomics yielded valuable information that provided complementary prognostic value in LVNC beyond routine clinical data. This may be because radiomics analyses obtained more information than that obtained with radiologists’ conventional imaging interpretation. Larger studies are needed to verify the complementary values of the radiomics features in identifying patients with LVNC at high risk of MACEs. In addition, although the sensitivity was improved via the addition of radiomics features to clinical factors, it was still relatively low. However, the combined model yielded an outstanding AUC and specificity, while the radiomics model had good sensitivity but low specificity. The tradeoff between predictive models depends on the weight of the sensitivity or specificity in different clinical or research scenarios.
Limitations
A few limitations to this study should be mentioned. First, we employed a single-center design, which might have introduced patient selection biases. Second, the small number of patients (especially those who experienced MACEs) is a limitation of the evaluation. Although we used data oversampling for data preprocessing, our results still need to be verified in a larger-sample study. Third, the types of CMR techniques and devices were limited in this single-center study. Evaluation of the different techniques and devices used across a number of centers can provide a more comprehensive understanding of the stability and heterogeneity of the radiomics features. Multicenter and multiscanner studies are needed to validate and generalize the performance of the proposed predictive models. Fourth, although the radiomics model and combined model performed well at predicting MACEs in this study, our future research is aimed at constructing predictive models for different adverse events in patients with LVNC for achieving a more effective prediction, thus allowing for individualized prevention and precise treatment.
Conclusions
Machine learning-based radiomics of cine CMR images provided a useful quantitative prognostic tool for predicting MACEs in patients with LVNC. Integrating radiomics features with clinical risk factors of these patients can achieve a more accurate and individualized prediction of poor prognostic outcomes, which may assist in their management and surveillance.
Acknowledgments
Funding: This work was supported by
Footnote
Conflicts of Interest: All authors have completed the ICMJE uniform disclosure form (available at https://qims.amegroups.com/article/view/10.21037/qims-23-372/coif). The authors have no conflicts of interest to declare.
Ethical Statement: The authors are accountable for all aspects of the work in ensuring that questions related to the accuracy or integrity of any part of the work are appropriately investigated and resolved. The study was conducted in accordance with the Declaration of Helsinki (as revised in 2013) and was approved by the Biomedical Research Ethics Committee of the West China Hospital of Sichuan University (No. 2022-1190). Individual consent for this retrospective analysis was waived.
Open Access Statement: This is an Open Access article distributed in accordance with the Creative Commons Attribution-NonCommercial-NoDerivs 4.0 International License (CC BY-NC-ND 4.0), which permits the non-commercial replication and distribution of the article with the strict proviso that no changes or edits are made and the original work is properly cited (including links to both the formal publication through the relevant DOI and the license). See: https://creativecommons.org/licenses/by-nc-nd/4.0/.
References
- van Waning JI, Caliskan K, Hoedemaekers YM, van Spaendonck-Zwarts KY, Baas AF, Boekholdt SM, et al. Genetics, Clinical Features, and Long-Term Outcome of Noncompaction Cardiomyopathy. J Am Coll Cardiol 2018;71:711-22. [Crossref] [PubMed]
- Mavrogeni S, Sfendouraki E, Theodorakis G, Kolovou G. Diagnosis, severity grading and prognosis of left ventricular non-compaction using cardiovascular magnetic resonance. Int J Cardiol 2013;167:598-9. [Crossref] [PubMed]
- Andreini D, Pontone G, Bogaert J, Roghi A, Barison A, Schwitter J, et al. Long-Term Prognostic Value of Cardiac Magnetic Resonance in Left Ventricle Noncompaction: A Prospective Multicenter Study. J Am Coll Cardiol 2016;68:2166-81. [Crossref] [PubMed]
- Aung N, Doimo S, Ricci F, Sanghvi MM, Pedrosa C, Woodbridge SP, Al-Balah A, Zemrak F, Khanji MY, Munroe PB, Naci H, Petersen SE. Prognostic Significance of Left Ventricular Noncompaction: Systematic Review and Meta-Analysis of Observational Studies. Circ Cardiovasc Imaging 2020;13:e009712. [Crossref] [PubMed]
- Shi WY, Moreno-Betancur M, Nugent AW, Cheung M, Colan S, Turner C, Sholler GF, Robertson T, Justo R, Bullock A, King I, Davis AM, Daubeney PEF, Weintraub RG. Long-Term Outcomes of Childhood Left Ventricular Noncompaction Cardiomyopathy: Results From a National Population-Based Study. Circulation 2018;138:367-76. [Crossref] [PubMed]
- Sedaghat-Hamedani F, Haas J, Zhu F, Geier C, Kayvanpour E, Liss M, et al. Clinical genetics and outcome of left ventricular non-compaction cardiomyopathy. Eur Heart J 2017;38:3449-60. [Crossref] [PubMed]
- Li Y, Liu X, Xu K, Qian Z, Wang K, Fan X, Li S, Wang Y, Jiang T. MRI features can predict EGFR expression in lower grade gliomas: A voxel-based radiomic analysis. Eur Radiol 2018;28:356-62. [Crossref] [PubMed]
- Zhang R, Zhang Q, Ji A, Lv P, Zhang J, Fu C, Lin J. Identification of high-risk carotid plaque with MRI-based radiomics and machine learning. Eur Radiol 2021;31:3116-26. [Crossref] [PubMed]
- Jiang YQ, Cao SE, Cao S, Chen JN, Wang GY, Shi WQ, Deng YN, Cheng N, Ma K, Zeng KN, Yan XJ, Yang HZ, Huan WJ, Tang WM, Zheng Y, Shao CK, Wang J, Yang Y, Chen GH. Preoperative identification of microvascular invasion in hepatocellular carcinoma by XGBoost and deep learning. J Cancer Res Clin Oncol 2021;147:821-33. [Crossref] [PubMed]
- Petresc B, Lebovici A, Caraiani C, Feier DS, Graur F, Buruian MM. Pre-Treatment T2-WI Based Radiomics Features for Prediction of Locally Advanced Rectal Cancer Non-Response to Neoadjuvant Chemoradiotherapy: A Preliminary Study. Cancers (Basel) 2020;12:1894. [Crossref] [PubMed]
- Gillies RJ, Kinahan PE, Hricak H. Radiomics: Images Are More than Pictures, They Are Data. Radiology 2016;278:563-77. [Crossref] [PubMed]
- Suarez-Ibarrola R, Basulto-Martinez M, Heinze A, Gratzke C, Miernik A. Radiomics Applications in Renal Tumor Assessment: A Comprehensive Review of the Literature. Cancers (Basel) 2020;12:1387. [Crossref] [PubMed]
- Jacquier A, Thuny F, Jop B, Giorgi R, Cohen F, Gaubert JY, Vidal V, Bartoli JM, Habib G, Moulin G. Measurement of trabeculated left ventricular mass using cardiac magnetic resonance imaging in the diagnosis of left ventricular non-compaction. Eur Heart J 2010;31:1098-104. [Crossref] [PubMed]
- Larroza A, Materka A, López-Lereu MP, Monmeneu JV, Bodí V, Moratal D. Differentiation between acute and chronic myocardial infarction by means of texture analysis of late gadolinium enhancement and cine cardiac magnetic resonance imaging. Eur J Radiol 2017;92:78-83. [Crossref] [PubMed]
- Baessler B, Mannil M, Oebel S, Maintz D, Alkadhi H, Manka R. Subacute and Chronic Left Ventricular Myocardial Scar: Accuracy of Texture Analysis on Nonenhanced Cine MR Images. Radiology 2018;286:103-12. [Crossref] [PubMed]
- Schofield R, Ganeshan B, Fontana M, Nasis A, Castelletti S, Rosmini S, Treibel TA, Manisty C, Endozo R, Groves A, Moon JC. Texture analysis of cardiovascular magnetic resonance cine images differentiates aetiologies of left ventricular hypertrophy. Clin Radiol 2019;74:140-9. [Crossref] [PubMed]
- Petersen SE, Selvanayagam JB, Wiesmann F, Robson MD, Francis JM, Anderson RH, Watkins H, Neubauer S. Left ventricular non-compaction: insights from cardiovascular magnetic resonance imaging. J Am Coll Cardiol 2005;46:101-5. [Crossref] [PubMed]
- Ponikowski P, Voors AA, Anker SD, Bueno H, Cleland JG, Coats AJ, et al. 2016 ESC Guidelines for the diagnosis and treatment of acute and chronic heart failure: The Task Force for the diagnosis and treatment of acute and chronic heart failure of the European Society of Cardiology (ESC). Developed with the special contribution of the Heart Failure Association (HFA) of the ESC. Eur J Heart Fail 2016;18:891-975.
- McMurray JJ, Adamopoulos S, Anker SD, Auricchio A, Böhm M, Dickstein K, et al. ESC Guidelines for the diagnosis and treatment of acute and chronic heart failure 2012: The Task Force for the Diagnosis and Treatment of Acute and Chronic Heart Failure 2012 of the European Society of Cardiology. Developed in collaboration with the Heart Failure Association (HFA) of the ESC. Eur Heart J 2012;33:1787-847. [Crossref] [PubMed]
- Chalkidou A, O'Doherty MJ, Marsden PK. False Discovery Rates in PET and CT Studies with Texture Features: A Systematic Review. PLoS One 2015;10:e0124165. [Crossref] [PubMed]
- Liu J, Guo W, Zeng P, Geng Y, Liu Y, Ouyang H, Lang N, Yuan H. Vertebral MRI-based radiomics model to differentiate multiple myeloma from metastases: influence of features number on logistic regression model performance. Eur Radiol 2022;32:572-81. [Crossref] [PubMed]
- Ramchand J, Podugu P, Obuchowski N, Harb SC, Chetrit M, Milinovich A, Griffin B, Burrell LM, Wilson Tang WH, Kwon DH, Flamm SD. Novel Approach to Risk Stratification in Left Ventricular Non-Compaction Using A Combined Cardiac Imaging and Plasma Biomarker Approach. J Am Heart Assoc 2021;10:e019209. [Crossref] [PubMed]
- Łuczak-Woźniak K, Werner B. Left Ventricular Noncompaction-A Systematic Review of Risk Factors in the Pediatric Population. J Clin Med 2021;10:1232. [Crossref] [PubMed]
- Wang C, Takasaki A, Watanabe Ozawa S, Nakaoka H, Okabe M, Miyao N, Saito K, Ibuki K, Hirono K, Yoshimura N, Yu X, Ichida F. Long-Term Prognosis of Patients With Left Ventricular Noncompaction - Comparison Between Infantile and Juvenile Types. Circ J 2017;81:694-700. [Crossref] [PubMed]
- Casas G, Limeres J, Oristrell G, Gutierrez-Garcia L, Andreini D, Borregan M, et al. Clinical Risk Prediction in Patients With Left Ventricular Myocardial Noncompaction. J Am Coll Cardiol 2021;78:643-62. [Crossref] [PubMed]
- Aerts HJ, Velazquez ER, Leijenaar RT, Parmar C, Grossmann P, Carvalho S, Bussink J, Monshouwer R, Haibe-Kains B, Rietveld D, Hoebers F, Rietbergen MM, Leemans CR, Dekker A, Quackenbush J, Gillies RJ, Lambin P. Decoding tumour phenotype by noninvasive imaging using a quantitative radiomics approach. Nat Commun 2014;5:4006. [Crossref] [PubMed]
- Lin Z, Wang T, Li H, Xiao M, Ma X, Gu Y, Qiang J. Magnetic resonance-based radiomics nomogram for predicting microsatellite instability status in endometrial cancer. Quant Imaging Med Surg 2023;13:108-20. [Crossref] [PubMed]
- Shang J, Guo Y, Ma Y, Hou Y. Cardiac computed tomography radiomics: a narrative review of current status and future directions. Quant Imaging Med Surg 2022;12:3436-53. [Crossref] [PubMed]
- Izquierdo C, Casas G, Martin-Isla C, Campello VM, Guala A, Gkontra P, Rodríguez-Palomares JF, Lekadir K. Radiomics-Based Classification of Left Ventricular Non-compaction, Hypertrophic Cardiomyopathy, and Dilated Cardiomyopathy in Cardiovascular Magnetic Resonance. Front Cardiovasc Med 2021;8:764312. [Crossref] [PubMed]
- Ross SB, Jones K, Blanch B, Puranik R, McGeechan K, Barratt A, Semsarian C. A systematic review and meta-analysis of the prevalence of left ventricular non-compaction in adults. Eur Heart J 2020;41:1428-36. [Crossref] [PubMed]
- Rauseo E, Izquierdo Morcillo C, Raisi-Estabragh Z, Gkontra P, Aung N, Lekadir K, Petersen SE. New Imaging Signatures of Cardiac Alterations in Ischaemic Heart Disease and Cerebrovascular Disease Using CMR Radiomics. Front Cardiovasc Med 2021;8:716577. [Crossref] [PubMed]
- Cetin I, Raisi-Estabragh Z, Petersen SE, Napel S, Piechnik SK, Neubauer S, Gonzalez Ballester MA, Camara O, Lekadir K. Radiomics Signatures of Cardiovascular Risk Factors in Cardiac MRI: Results From the UK Biobank. Front Cardiovasc Med 2020;7:591368. [Crossref] [PubMed]
- Lee J, Steinmann A, Ding Y, Lee H, Owens C, Wang J, Yang J, Followill D, Ger R, MacKin D, Court LE. Radiomics feature robustness as measured using an MRI phantom. Sci Rep 2021;11:3973. [Crossref] [PubMed]
- Fernández A, García S, Herrera F, Chawla NV. SMOTE for learning from imbalanced data: progress and challenges, marking the 15-year anniversary. J Artif Intell Res 2018;61:863-905.
- Blagus R, Lusa L. SMOTE for high-dimensional class-imbalanced data. BMC Bioinformatics 2013;14:106.
- Sreejith S, Khanna Nehemiah H, Kannan A. Clinical data classification using an enhanced SMOTE and chaotic evolutionary feature selection. Comput Biol Med 2020;126:103991. [Crossref] [PubMed]
- Xu Z, Shen D, Nie T, Kou Y. A hybrid sampling algorithm combining M-SMOTE and ENN based on Random forest for medical imbalanced data. J Biomed Inform 2020;107:103465. [Crossref] [PubMed]
- Wang L, Wu X, Tian R, Ma H, Jiang Z, Zhao W, Cui G, Li M, Hu Q, Yu X, Xu W. MRI-based pre-Radiomics and delta-Radiomics models accurately predict the post-treatment response of rectal adenocarcinoma to neoadjuvant chemoradiotherapy. Front Oncol 2023;13:1133008. [Crossref] [PubMed]
- Gao C, Killeen BD, Hu Y, Grupp RB, Taylor RH, Armand M, Unberath M. Synthetic data accelerates the development of generalizable learning-based algorithms for X-ray image analysis. Nature Machine Intelligence 2023;5:294-308.
- Liu P, Fu B, Yang SX, Deng L, Zhong X, Zheng H. Optimizing Survival Analysis of XGBoost for Ties to Predict Disease Progression of Breast Cancer. IEEE Trans Biomed Eng 2021;68:148-60. [Crossref] [PubMed]
- Le NQK, Do DT, Chiu FY, Yapp EKY, Yeh HY, Chen CY. XGBoost Improves Classification of MGMT Promoter Methylation Status in IDH1 Wildtype Glioblastoma. J Pers Med 2020;10:128. [Crossref] [PubMed]
- Shwartz-Ziv R, Armon A. Tabular data: deep learning is not all you need. Inform Fusion 2022;81:84-90.
- Fayaz SA, Zaman M, Kaul S, Butt MA. Is deep learning on tabular data enough? an assessment. International Journal of Advanced Computer Science and Applications 2022;13:466-73.
- Huang S, Cai N, Pacheco PP, Narrandes S, Wang Y, Xu W. Applications of Support Vector Machine (SVM) Learning in Cancer Genomics. Cancer Genomics Proteomics 2018;15:41-51. [Crossref] [PubMed]
- Huang MW, Chen CW, Lin WC, Ke SW, Tsai CF. SVM and SVM Ensembles in Breast Cancer Prediction. PLoS One 2017;12:e0161501. [Crossref] [PubMed]