A quantitative analysis framework of placenta accreta spectrum: placenta subtype, intraoperative bleeding, and hysterectomy risk evaluation based on magnetic resonance imaging-anatomical-clinical features
Introduction
Placenta accreta spectrum (PAS) disorders signify the diverse extents of direct infiltration of the uterine myometrium by placental trophoblast cells, impeding the partial or complete detachment of the placenta from the myometrium (1). These conditions have exhibited an augmented frequency over the past few decades (1). PAS can be classified into 3 distinct subtypes, namely accreta, increta, and percreta, based on the extent of trophoblastic infiltration (1,2). The deeper the invasion of placental trophoblast cells, the greater the likelihood of encountering unfavorable pregnancy outcomes, potentially resulting in profound hemorrhage, necessitating hysterectomy, causing damage to the urinary system, and even leading to maternal and fetal mortality. It is noteworthy, however, that not all pregnant women diagnosed with placenta accreta are exposed to extreme risk (2). Consequently, the identification of placental subtypes during prenatal care and the identification of high-risk pregnant individuals vulnerable to intraoperative hemorrhage and hysterectomy carry significant clinical implications (3).
In recent times, machine learning (ML) techniques have exhibited their potential in facilitating intricate medical image or data analysis, thereby enhancing the productivity of physicians (4-6). In the context of PAS, these investigations have predominantly focused on the remarkable diagnostic accuracy and value of magnetic resonance imaging (MRI) radiomics (7,8). Although some studies have reported outstanding performance in methodological metrics, 3 current restrictions remain. Firstly, the model’s dependence on manually annotated regions of interest (ROIs) by radiologists has proven impractical in clinical settings (7-9). Secondly, the algorithm utilized in the studies is excessively simplistic, and the majority of research lacks the inclusion of multicenter data, thereby imposing limitations on the generalizability of the research findings (8,10). Thirdly, due to the inherent “black box” nature of ML methods, interpreting the decision-making process within the model becomes challenging, leading to clinicians’ reluctance in utilizing it (7-10).
To the best of our knowledge, no existing literature has reported a comprehensive framework utilizing ML for the systematic prediction of PAS placenta subtype, intraoperative bleeding, and hysterectomy risk. In this study, we devised an integrated approach that combined MRI-anatomical-clinical features and employed the robust extreme gradient boosting (XGBoost) algorithm. This framework facilitated a quantitative analysis of placenta subtype (invasive vs. non-invasive), intraoperative bleeding (≥1,500 vs. <1,500 mL), and hysterectomy risk (hysterectomy vs. non-hysterectomy). Additionally, we performed a quantitative assessment of the influence of MRI-anatomical-clinical features on each model’s decision-making process, employing SHapley Additive exPlanations (SHAP) values to provide insights into how these decisions are derived.
Methods
Participant cohorts
In this retrospective study, pregnant women who were suspected of having PAS in 2 medical centers (Shenzhen People’s Hospital and The Third Affiliated Hospital of Shenzhen University) from January 2018 to October 2022 were collected. The former hospital is a tertiary referral center under municipal administration, whereas the latter is a tertiary referral center under district administration. Initially, a total of 243 cases were screened for inclusion in this study. We rigorously adhered to the specified inclusion and exclusion criteria, as depicted in Figure 1. The inclusion criteria encompassed the following: (I) gestational age exceeding 28 weeks; (II) singleton pregnancy; and (III) MRI had been conducted prior to the cesarean operation. Conversely, the exclusion criteria were as follows: (I) inadequate availability of complete clinical, surgical, and pathological information; (II) presence of severe motion artifacts or other image-related anomalies; (III) coagulation disorders; (IV) occurrences of placental abruption or uterine rupture; and (V) fetal malformations. The diagram of the analysis framework is shown in Figure 2. The study was conducted in strict accordance with the Declaration of Helsinki (revised in 2013), and approval from the Research Ethics Committee (No. KY2022-038-01) was obtained for this research. The requirement for informed consent documents was waived for this retrospective analysis.
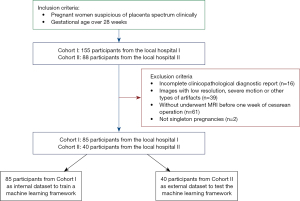
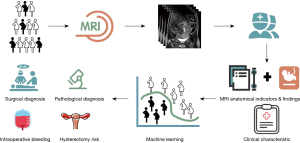
MRI protocol and acquisition
The MRI image acquisition was performed using a 1.5-T scanner with a phased-array body coil at both centers. The T2-weighted sequences were obtained in 3 planes (axial, sagittal, and coronal) during the MRI scanning. The specific parameters used for the MRI scans are provided in Table S1.
MRI-anatomical-clinical features evaluation
Based on the guidelines of Society of Abdominal Radiology (SAR) and European Society of Urogenital Radiology (ESUR) in 2020 for MRI imaging of PAS (11), and taking into consideration clinical practices and related studies (12-16), we incorporated several morphological findings and anatomical indicators about the MRI of PAS. Morphological findings (see Tables S1,S2 and Figure S1) included the following: (I) placental heterogeneity; (II) placental tissue protrusion into the cervical canal (Figure S1A); (III) uterine placental bulge; (IV) intraplacental dark T2 bands (Figure S1B); (V) loss of low T2 retroplacental line (Figure S1C); (VI) myometrial thinning disruption; (VII) bladder wall interruption (Figure S1D); (VIII) focal exophytic placental mass; (IX) placenta previa subtype at MRI; (X) main location of placental attachment; and (XI) location of intraplacental dark T2 bands. Anatomical indicators included the following: (I) cervical canal length; (II) diameter of placental abnormal vasculature (Figure S1E); (III) uterine anteroposterior diameter ratio (Figure S1F); (IV) placental abnormal vasculature area in T2WI; and (V) intraplacental dark T2 bands area.
In our analysis, we derived the area of abnormal placental vasculature through the multiplication of the length and width of the largest dimension observed in T2-weighted imaging (T2WI). Likewise, the area of intraplacental dark T2 bands was determined by measuring the length and width of the largest dimension evident in T2WI. Moreover, we calculated the ratio of the uterine anteroposterior diameters using a systematic approach: Firstly, a straight line was sketched on sagittal T2WI, connecting the endocervix to the highest point of the uterine fundus. Secondly, the anteroposterior diameter of both the upper and lower uterine segments was measured perpendicular to this line. Lastly, the ratio of the anteroposterior diameter of the upper segment to that of the lower segment was employed to evaluate the expansion of the lower uterine segment. MRI morphological findings and anatomical indicators were meticulously analyzed by 2 highly experienced radiologists, namely Doctor A and Doctor B, with 11 and 12 years of expertise in the field of diagnostic imaging of obstetrical and gynecological conditions, respectively. It is important to note that they carried out the analysis in a blinded manner, devoid of any knowledge regarding the clinical, surgical, or pathologic information associated with the cases. In situations where morphological findings were absent, a value of 0 was assigned; if there was a possibility of their existence, a value of 1 was assigned; and if the findings were clearly evident, a value of 2 was assigned. In the event of any disagreement between the 2 radiologists, a thorough discussion ensued, and a final consensus was achieved in consultation with another esteemed radiologist, Doctor C, with 31 years of diagnostic experience.
Furthermore, we incorporated the following clinical characteristics into our analysis: (I) maternal age; (II) gravidity; (III) parity; (IV) history of prior cesarean section; and (V) history of uterine surgeries such as abortion, dilatation and curettage (D&C), and myomectomy. In total, 21 MRI-anatomical-clinical features were amalgamated as input into our framework, encompassing morphological findings, anatomical indicators, and clinical characteristics.
ML algorithm and model comparison
In accordance with the 2019 International Federation of Gynecology and Obstetrics (FIGO) classification (17), the included cases were stratified into 2 groups: non-invasive placenta implantation (comprising normally adherent placenta and FIGO grade 1) and invasive placenta implantation (encompassing FIGO grades 2 and 3). According to the guidelines from the American College of Obstetricians and Gynecologists (ACOG) (18), immediate initiation of transfusion therapy is recommended when maternal blood loss reaches or exceeds 1,500 mL. Therefore, we defined this threshold as the boundary for intraoperative massive bleeding. Different ML classification algorithms [such as logistic regression (LR), support vector machines (SVM), random forest (RF), and extreme gradient boosting (XGBoost)] (19) were adopted to predict the placenta subtype (invasive vs. non-invasive), intraoperative bleeding (≥1,500 vs. <1,500 mL) and hysterectomy risk (hysterectomy vs. non-hysterectomy).
In addition, we conducted a comparative analysis to evaluate the predictive accuracy of placenta subtype (invasive vs. non-invasive) between the proposed model and 4 experienced radiologists (Doctor 1: 31 years of diagnostic experience, Doctor 2: 22 years of diagnostic experience, Doctor 3: 13 years of diagnostic experience, Doctor 4: 9 years of diagnostic experience). It is noteworthy that the radiologists employed a binary classification approach rather than assigning likelihood scores when assessing the placental subtypes. We conducted a comparative analysis of the model and 4 radiologists in terms of accuracy, sensitivity, and specificity in diagnosing different subtypes of PAS. A LR model with random effects (glmer) was utilized to evaluate the diagnostic performance of the 4 radiologists and the model on the same testing dataset.
Model explaining and statistical analysis
To assess the significance of the input features in the model’s prediction, we computed the SHAP value for each feature in relation to each sample (20). The SHAP values provide insights into the contribution of each feature towards influencing the predictive model. A higher SHAP value indicates that the corresponding feature has a substantial impact on the model’s decision-making process.
We utilized the original Cohen’s kappa coefficient to assess inter-rater agreement among 2 radiologists for 11 morphological findings. The uncertainty of the estimate such as accuracy, area under the receiver operating characteristic curve (AUROC) was quantified at a 95% confidence interval (CI). The McNemar test was utilized to evaluate the disparities between the diagnostic outcomes of the 4 radiology experts and the model. The receiver operating characteristic (ROC) test (Bootstrap method) was used for statistical comparison between several common-used ML algorithms such as LR, SVM, RF, and XGBoost. All these statistical analyses and experiments were conducted in Python (v3.8; Python Software Foundation, Wilmington, DE, USA) and R (v3.6.3; R Foundation for Statistical Computing, Vienna, Austria). Statistical significance was considered when the P value was <0.05.
Results
Participant characteristics and readers agreement
A total of 125 cases were enrolled and divided into an internal cohort (n=85, a local hospital) and an external testing cohort (n=40, another local hospital). The cases in the internal cohort were stratified shuffle split into a training cohort (n=60, 71%) and an internal validation cohort (n=25, 29%). The basic and clinical information of these participants are shown in Table 1. In this study, the overall prevalence of PAS was found to be 73.60%. The breakdown of the subtypes within PAS was as follows: without placenta accreta accounted for 26.40%, placenta increta for 20.00%, placenta accreta for 35.20%, and placenta percreta for 18.40%. The 2 radiologists (Doctor A and B) had high agreement on the interpretation of morphological findings (all Kappa values greater than 0.7 in Table S3).
Table 1
Characteristics | Internal cohort | External cohort |
---|---|---|
Participants (n) | 85 | 40 |
Maternal age (years), median [range] | 35 [21–45] | 34 [26–45] |
Gravidity (n), median [range] | 3 [1–8] | 3 [2–7] |
Parturition (n), median [range] | 1[0–3] | 1 [1–2] |
Number of prior C-sections, median [range] | 1 [0–3] | 1 [0–2] |
History of uterine surgery (n), median [range] | 1 [0–4] | 0 [0–2] |
Fetal birth weight (g), median [range] | 2,700 [1,250–4,000] | 2,765 [1,400–3,820] |
Gestational age (days), median [range] | 253 [198–276] | 254 [199–276] |
Histologic or surgical outcomes, n (%) | ||
Without placenta accreta | 19 (22.35) | 14 (35.00) |
Placenta increta | 19 (22.35) | 6 (15.00) |
Placenta accreta | 32 (37.65) | 12 (30.00) |
Placenta percreta | 15 (17.65) | 8 (20.00) |
Intraoperative bleeding, n (%) | ||
Small (<1,500 mL) | 33 (38.82) | 24 (60.00) |
Massive (≥1,500 mL) | 52 (61.18) | 16 (40.00) |
Surgery risk, n (%) | ||
Hysterectomy (partial or radical) | 56 (65.88) | 28 (70.00) |
Non-hysterectomy | 29 (34.12) | 12 (30.00) |
The quantitative framework presents robust analytical capability
To verify the robust analytical capability of our algorithms, we compared the model performance trained by the LR, SVM, RF, and XGBoost algorithms, and found that the XGBoost algorithm had the best performance in all 3 classification tasks. Figure 3 demonstrates that none of the alternative algorithms achieved a comparable AUROC to that of the XGBoost algorithm across all classification tasks, despite the lack of statistically significant differences observed in the comparisons with the XGBoost algorithm. Therefore, we selected the XGBoost algorithm as the prediction model for this study.
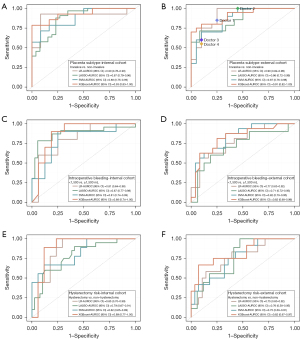
The diagnostic performance of each classification task in the internal and external cohorts are shown in Table 2. As shown in Figure 3A,3B, the AUROC was 0.93 (0.83–1.00) and 0.91 (95% CI: 0.82–1.00) for placenta subtype (invasive vs. non-invasive) classification in the internal validation set and external testing set, respectively. Figure 3C,3D shows that the AUROC was 0.88 (0.74–1.00) and 0.82 (95% CI: 0.68–0.96) for intraoperative bleeding (<1,500 vs. ≥1,500 mL) classification at the internal validation set and external testing set, respectively. As shown in Figure 3E,3F, the AUROC was 0.89 (0.77–1.00) and 0.82 (95% CI: 0.67–0.97) for hysterectomy risk (hysterectomy vs. non-hysterectomy) classification in the internal validation set and external testing set, respectively.
Table 2
Classification task | AUROC | Sensitivity | Specificity | Cut-off value | Accuracy |
---|---|---|---|---|---|
Placenta subtype | |||||
Internal | 0.93 (0.83–1.00) | 0.79 (0.71–0.87) | 0.99 (0.97–1.00) | 0.51 (0.41–0.61) | 0.85 (0.78–0.92) |
External | 0.91 (0.82–1.00) | 0.80 (0.72–0.88) | 0.90 (0.83–0.95) | 0.61 (0.51–0.91) | 0.83 (0.76–0.90) |
Intraoperative bleeding | |||||
Internal | 0.88 (0.74–1.00) | 0.90 (0.84–0.96) | 0.81 (0.72–0.88) | 0.25 (0.16–0.34) | 0.85 (0.79–0.93) |
External | 0.82 (0.68–0.96) | 0.81 (0.73–0.89) | 0.75 (0.67–0.84) | 0.392 (0.29–0.49) | 0.78 (0.70–0.86) |
Hysterectomy risk | |||||
Internal | 0.89 (0.77–1.00) | 0.89 (0.83–0.95) | 0.88 (0.82–0.94) | 0.39 (0.30–0.48) | 0.85 (0.77–0.91) |
External | 0.82 (0.67–0.97) | 0.67 (0.58–0.76) | 0.93 (0.88–0.98) | 0.52 (0.42–0.62) | 0.83 (0.77–0.90) |
Data are presented as the numerical values of model performance parameters and their 95% confidence intervals. AUROC, area under the receiver operating characteristic curve.
Diagnostic performance of the model versus radiologists
We conducted a comprehensive evaluation of the performance (accuracy, sensitivity, and specificity) in predicting the placenta subtype (invasive vs. non-invasive) between our proposed model and 4 radiologists. Interestingly, none of the radiologists (0.80, 0.78, 0.75, and 0.73) attained the level of accuracy demonstrated by our model (0.83) in the external testing set (Figure S2A). The sensitivity and specificity results of the 4 radiologists can be observed in Figure 3B and Figure S2B,S2C, respectively. Notably, none of the radiologists (0.77, 0.69, 0.86, and 0.85) managed to match the impressive level of specificity demonstrated by our model (0.90) in the external testing set (Figure S2B). Furthermore, in the external testing set, the 4 radiologists achieved sensitivities of 0.88, 1.00, 0.69, and 0.67, whereas our model exhibited a commendable sensitivity of 0.80 (Figure S2C).
In addition, a LR model with random effects was utilized to evaluate the diagnostic performance of the 4 radiologists and the model on the same testing dataset. The generalized linear mixed model demonstrated significant effects of the predictor variable on the outcome, with estimated coefficients of 3.2941 (P<0.01) for the Doctor 1 grouping, 2.990 (P<0.01) for the Doctor 2 grouping, 3.5835 (P<0.01) for the Doctor 3 grouping, and 3.5835 (P<0.01) for the Doctor 4 grouping. Overall, the generalized linear mixed model revealed a significant effect of the predictor variable on the outcome, with estimated coefficients ranging from 2.990 to 3.5835 (P<0.01), while accounting for the random effects of the Doctor 1, Doctor 2, Doctor 3, and Doctor 4 groupings.
Meaningful features contribution evaluation by SHAP values
To assess the impact of imaging features on the model’s prediction, the SHAP value was calculated to analyze the individual influences of each feature for every sample. The SHAP values of all the relevant features were presented in bar plots for each classification task. Positive SHAP values indicate a greater likelihood of the respective prediction. Irrespective of the classification tasks at hand, these influential features encompass various aspects, including subcategories of morphological findings, anatomical indicators, and clinical characteristics. Notably, all anatomical indicators played a substantial role in the decision-making process of each model. As shown in Figure 4A, the area of intraplacental dark T2 bands, the diameter of placental abnormal vasculature, and the presence of intraplacental dark T2 bands emerged as pivotal factors in distinguishing placenta subtype (invasive vs. non-invasive). Figure 4B shows that parturition, bladder wall interruption, and cervical canal length exhibited significant contributions to the classification of intraoperative bleeding [≥1,500 vs. <1,500 mL (massive vs. small)]. Likewise, in the classification of hysterectomy risk (hysterectomy vs. non-hysterectomy), prior section history, maternal age, and the area of intraplacental dark T2 bands assumed an indispensable role in the decision-making process of the model (Figure 4C).
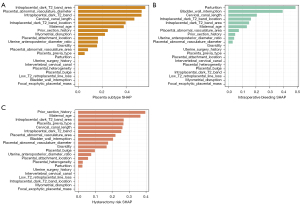
To validate the importance of these significant features in the model’s decision, we eliminated features with SHAP values equal to zero and retrained the model. Remarkably, the retrained model achieved comparable performance to the proposed model in classifying placenta subtype (invasive vs. non-invasive), intraoperative bleeding (≥1,500 vs. <1,500 mL), and hysterectomy risk (hysterectomy vs. non-hysterectomy). The corresponding AUROC values remained at 0.93, 0.88, and 0.90, respectively (Figure S3A-S3C).
Discussion
In this study, we developed an analysis framework utilizing MRI-anatomical-clinical features, employing ML techniques as a quantitative approach for predicting PAS during prenatal stages. To enhance visualization and facilitate clinical application and decision-making, we conducted a detailed quantitative analysis of the influence of MRI-anatomical-clinical features on the model’s decision-making process using SHAP algorithms. Our analysis framework offers a more robust prediction of placenta subtype, intraoperative bleeding, and hysterectomy risk compared to traditional MRI radiomics and evaluation methods, which primarily rely on high-throughput feature extraction or morphological findings.
In recent years, the integration of big data and artificial intelligence has led to the application of radiomics in clinical research (4,21). However, in the context of PAS, research on ML methods has primarily focused on using traditional radiomics to construct simple models for predicting the presence or absence of PAS (7-10). Radiomics involves the extraction of a large number of imaging features from medical images, enabling a more in-depth analysis of these images to assist doctors in making accurate diagnoses (22). Nevertheless, radiomics primarily emphasizes heterogeneous image texturing, particularly texture analysis, with limited emphasis on the anatomical structure of the lesion and surrounding tissues or organs (10). This approach is more suitable for identifying diseases with high heterogeneity, such as tumors, rather than diseases with low heterogeneity. Therefore, in our study, we opted to utilize MRI-anatomical-clinical features instead of radiomic features to predict the placenta subtype (invasive vs. non-invasive), intraoperative bleeding (≥1,500 vs. <1,500 mL), and hysterectomy risk (hysterectomy vs. non-hysterectomy).
In the realm of ML, many models exhibit weaknesses including a lack of interpretability, functioning as enigmatic “black boxes”. In an effort to address this issue, our study conducted a quantitative analysis, delving into the relationship between each feature and the model’s decision through the utilization of SHAP algorithms (20). Contrasted with alternative methods of interpretation, such as class activation diagrams (CAM) (23), SHAP values offer a comprehensive assessment of the impact that each feature has on individual cases and the overall dataset. This in-depth analysis proves instrumental in comprehending the model’s decisions and even identifying the underlying causes of misclassification. To ascertain the reliability of our findings, we selectively excluded features with SHAP values of zero and subsequently retrained the model. As depicted in Figure S3A-S3C, the performance of the retrained model, following feature selection, was tantamount to that of the original model across all classification tasks.
In this study, it became apparent that all anatomical indicators assumed a significant role in the model’s decision-making process. This suggests that these anatomical indices possess a potentially greater importance as markers for determining the placenta subtype, intraoperative bleeding, and hysterectomy risk associated with PAS, surpassing the significance of morphological findings. As is widely acknowledged, the presence of intraplacental dark T2 bands serves as a direct indication of myometrial invasion by the placenta, with the extent of placental accretion directly proportional to the size of the area covered by intraplacental dark T2 bands (24-26). Our findings further validate the indispensability of features related to intraplacental dark T2 bands, such as the presence, area, and location of these bands, in accurately predicting the placenta subtype (invasive vs. non-invasive).
As shown in the Figure 4B, the cervical canal length and uterine anteroposterior diameter ratio played a vital role in the model decision of intraoperative bleeding (≥1,500 vs. <1,500 mL) classification. Placental intrusion into the cervical canal commonly induces cervical canal shortening, resulting in a widened lower uterine segment and restricted surgical visibility, thereby exacerbating the surgical complexity (27-29). This aspect could potentially contribute to the undesirable consequence of significant intraoperative bleeding and serves as a noteworthy anatomical parameter for capturing the presence of lower segment bulging. In a typical healthy pregnancy, the uterine morphology exhibits a characteristic pear-shaped contour, whereas diffuse lower segment distension signifies the occurrence of placental implantation (26).
In terms of assessing the risk of hysterectomy (hysterectomy vs. non-hysterectomy), it was found that prior section history and maternal age played the most significant roles in the model’s decision-making process. These factors are likely influenced by a pregnant woman’s individual inclination to mitigate risks and her preference to preserve her uterus. The presence of bladder wall interruption serves as a direct indication of placental percreta and the invasion of placental tissue into surrounding tissues and organs (26). When placental tissue penetrates the uterine wall and reaches the bladder or abdominal wall, the separation becomes exceedingly challenging, thus increasing the risks of substantial bleeding and a higher likelihood of hysterectomy. In our study, the presence of bladder wall interruption emerged as a crucial contributing factor in both the classification of intraoperative bleeding (≥1,500 vs. <1,500 mL) and the classification of hysterectomy risk (hysterectomy vs. non-hysterectomy).
The diagnosis of PAS using MRI is known to exhibit significant variability among different readers. The incorporation of quantitative ML techniques would have enhanced the strength of the analysis results. In comparison to the prediction accuracy of 4 radiologists in differentiating placental subtypes (invasive and non-invasive), our model demonstrated significantly higher accuracy in the external testing cohort. Consequently, our proposed model exhibited superior accuracy when compared to the radiologists. These findings hold considerable significance in guiding the prediction and diagnosis of placenta accreta prior to surgical intervention.
In practical application scenarios, a reliable model should not only demonstrate adaptability to various data sets but also provide interpretable results (30). Both the internal training cohort and the external test cohort encompassed a heterogeneous range of cases, which enhanced the generalizability and interpretability of our model. Even in cases where the “over-fit” phenomenon was observed, the external testing cohort for placenta subtype (invasive vs. non-invasive), intraoperative bleeding (≥1,500 vs. <1,500 mL), and hysterectomy risk (hysterectomy vs. non-hysterectomy) prediction models still achieved impressive AUROC values of 0.91, 0.82, and 0.82, respectively. Hence, our framework can be readily applied to new data sets with varying structures.
This study had several limitations. Firstly, the study population consisted of pregnant women with suspected PAS, excluding those without suspicion of PAS in prenatal examinations. This exclusion of cases where placenta accreta was not detected during prenatal assessments may have led to underestimation of the predictive performance of the model due to sampling bias. Secondly, the selection of total hysterectomy is contingent upon the technical expertise available at the hospital and the individual preferences of the patient, thus our model can only serve as a reference in such scenarios. Thirdly, it is important to acknowledge that the inherent subjectivity and inter-reader variability in assessing MRI features cannot be entirely eradicated, representing a potential confounding factor. Lastly, the practicality of this framework in clinical settings and its potential to improve patient outcomes remain unknown as this is an exploratory study based on retrospective medical records. The generalizability of this framework and its value in practical clinical applications need to be further validated through subsequent prospective cohort studies.
Conclusions
Our quantitative analysis framework, which incorporates MRI-anatomical-clinical features, demonstrated strong predictive performance in determining the placenta subtype (invasive vs. non-invasive), intraoperative bleeding (≥1,500 vs. <1,500 mL), and hysterectomy risk (hysterectomy vs. non-hysterectomy) associated with PAS. This research not only offers a promising adjunctive method for clinical management and decision-making in PAS pregnancies but also establishes a foundation for future anatomy-based clinical approaches in similar diseases.
Acknowledgments
We extend our heartfelt appreciation to Shenzhen People’s Hospital and The Third Affiliated Hospital of Shenzhen University for generously providing the data for this project.
Funding: This work was supported by
Footnote
Conflicts of Interest: All authors have completed the ICMJE uniform disclosure form (available at https://qims.amegroups.com/article/view/10.21037/qims-23-142/coif). The authors have no conflicts of interest to declare.
Ethical Statement: The authors are accountable for all aspects of the work in ensuring that questions related to the accuracy or integrity of any part of the work are appropriately investigated and resolved. The study was conducted in accordance with the Declaration of Helsinki (revised in 2013), and approval from the Research Ethics Committee (No. KY2022-038-01) was obtained for this research. The requirement for informed consent documents was waived for this retrospective analysis.
Open Access Statement: This is an Open Access article distributed in accordance with the Creative Commons Attribution-NonCommercial-NoDerivs 4.0 International License (CC BY-NC-ND 4.0), which permits the non-commercial replication and distribution of the article with the strict proviso that no changes or edits are made and the original work is properly cited (including links to both the formal publication through the relevant DOI and the license). See: https://creativecommons.org/licenses/by-nc-nd/4.0/.
References
- Silver RM, Branch DW. Placenta Accreta Spectrum. N Engl J Med 2018;378:1529-36. [Crossref] [PubMed]
- Matsuzaki S, Mandelbaum RS, Sangara RN, McCarthy LE, Vestal NL, Klar M, Matsushima K, Amaya R, Ouzounian JG, Matsuo K. Trends, characteristics, and outcomes of placenta accreta spectrum: a national study in the United States. Am J Obstet Gynecol 2021;225:534.e1-534.e38. [Crossref] [PubMed]
- Garmi G, Salim R. Epidemiology, etiology, diagnosis, and management of placenta accreta. Obstet Gynecol Int 2012;2012:873929. [Crossref] [PubMed]
- Yang H, Wu K, Liu H, Wu P, Yuan Y, Wang L, Liu Y, Zeng H, Li J, Liu W, Wu S. An automated surgical decision-making framework for partial or radical nephrectomy based on 3D-CT multi-level anatomical features in renal cell carcinoma. Eur Radiol 2023; Epub ahead of print. [Crossref]
- Ardila D, Kiraly AP, Bharadwaj S, Choi B, Reicher JJ, Peng L, Tse D, Etemadi M, Ye W, Corrado G, Naidich DP, Shetty S. End-to-end lung cancer screening with three-dimensional deep learning on low-dose chest computed tomography. Nat Med 2019;25:954-61. [Crossref] [PubMed]
- McKinney SM, Sieniek M, Godbole V, Godwin J, Antropova N, Ashrafian H, et al. International evaluation of an AI system for breast cancer screening. Nature 2020;577:89-94. [Crossref] [PubMed]
- Sun H, Qu H, Chen L, Wang W, Liao Y, Zou L, Zhou Z, Wang X, Zhou S. Identification of suspicious invasive placentation based on clinical MRI data using textural features and automated machine learning. Eur Radiol 2019;29:6152-62. [Crossref] [PubMed]
- Romeo V, Ricciardi C, Cuocolo R, Stanzione A, Verde F, Sarno L, Improta G, Mainenti PP, D'Armiento M, Brunetti A, Maurea S. Machine learning analysis of MRI-derived texture features to predict placenta accreta spectrum in patients with placenta previa. Magn Reson Imaging 2019;64:71-6. [Crossref] [PubMed]
- Wu Q, Yao K, Liu Z, Li L, Zhao X, Wang S, Shang H, Lin Y, Wen Z, Zhang X, Tian J, Wang M. Radiomics analysis of placenta on T2WI facilitates prediction of postpartum haemorrhage: A multicentre study. EBioMedicine 2019;50:355-65. [Crossref] [PubMed]
- Do QN, Lewis MA, Xi Y, Madhuranthakam AJ, Happe SK, Dashe JS, Lenkinski RE, Khan A, Twickler DM. MRI of the Placenta Accreta Spectrum (PAS) Disorder: Radiomics Analysis Correlates With Surgical and Pathological Outcome. J Magn Reson Imaging 2020;51:936-46. [Crossref] [PubMed]
- Jha P, Pōder L, Bourgioti C, Bharwani N, Lewis S, Kamath A, Nougaret S, Soyer P, Weston M, Castillo RP, Kido A, Forstner R, Masselli G. Society of Abdominal Radiology (SAR) and European Society of Urogenital Radiology (ESUR) joint consensus statement for MR imaging of placenta accreta spectrum disorders. Eur Radiol 2020;30:2604-15.
- Ueno Y, Kitajima K, Kawakami F, Maeda T, Suenaga Y, Takahashi S, Matsuoka S, Tanimura K, Yamada H, Ohno Y, Sugimura K. Novel MRI finding for diagnosis of invasive placenta praevia: evaluation of findings for 65 patients using clinical and histopathological correlations. Eur Radiol 2014;24:881-8. [Crossref] [PubMed]
- Ueno Y, Maeda T, Tanaka U, Tanimura K, Kitajima K, Suenaga Y, Takahashi S, Yamada H, Sugimura K. Evaluation of interobserver variability and diagnostic performance of developed MRI-based radiological scoring system for invasive placenta previa. J Magn Reson Imaging 2016;44:573-83. [Crossref] [PubMed]
- Tanimura K, Morizane M, Deguchi M, Ebina Y, Tanaka U, Ueno Y, Kitajima K, Maeda T, Sugimura K, Yamada H. A novel scoring system for predicting adherent placenta in women with placenta previa. Placenta 2018;64:27-33. [Crossref] [PubMed]
- Bourgioti C, Zafeiropoulou K, Fotopoulos S, Nikolaidou ME, Theodora M, Daskalakis G, Tzavara C, Chatoupis K, Panourgias E, Antoniou A, Konstantinidou A, Moulopoulos LA. MRI prognosticators for adverse maternal and neonatal clinical outcome in patients at high risk for placenta accreta spectrum (PAS) disorders. J Magn Reson Imaging 2019;50:602-18. [Crossref] [PubMed]
- Chen D, Xu J, Ye P, Li M, Duan X, Zhao F, Liu X, Wang X, Peng B. Risk scoring system with MRI for intraoperative massive hemorrhage in placenta previa and accreta. J Magn Reson Imaging 2020;51:947-58. [Crossref] [PubMed]
- Jauniaux E, Ayres-de-Campos D, Langhoff-Roos J, Fox KA, Collins S. FIGO Placenta Accreta Diagnosis and Management Expert Consensus Panel. FIGO classification for the clinical diagnosis of placenta accreta spectrum disorders. Int J Gynaecol Obstet 2019;146:20-4. [Crossref] [PubMed]
- Practice Bulletin No. 183: Postpartum Hemorrhage. Obstet Gynecol 2017;130:e168-86.
- Chen T, Guestrin C. Xgboost: A scalable tree boosting system. Proceedings of the 22nd ACM SIGKDD international conference on knowledge discovery and data mining. Association for Computing Machinery; 2016:785-94.
- Lundberg SM, Erion G, Chen H, DeGrave A, Prutkin JM, Nair B, Katz R, Himmelfarb J, Bansal N, Lee SI. From Local Explanations to Global Understanding with Explainable AI for Trees. Nat Mach Intell 2020;2:56-67. [Crossref] [PubMed]
- Yang H, Lin J, Liu H, Yao J, Lin Q, Wang J, Jiang F, Wei L, Lin C, Wu K, Wu S. Automatic analysis framework based on 3D-CT multi-scale features for accurate prediction of Ki67 expression levels in substantial renal cell carcinoma. Insights Imaging 2023;14:130. [Crossref] [PubMed]
- Chu C, Liu M, Zhang Y, Zhao S, Ge Y, Li W, Gao C. MRI-Based Radiomics Analysis for Intraoperative Risk Assessment in Gravid Patients at High Risk with Placenta Accreta Spectrum. Diagnostics (Basel) 2022;12:485. [Crossref] [PubMed]
- Yang W, Huang H, Zhang Z, Chen X, Huang K, Zhang S. Towards rich feature discovery with class activation maps augmentation for person re-identification. 2019 IEEE/CVF Conference on Computer Vision and Pattern Recognition (CVPR); Long Beach, CA, USA; 15-20 June 2019. IEEE; 2019:1389-98.
- Sato T, Mori N, Hasegawa O, Shigihara T, Fujimori K, Tasaki K, Shishido F. Placental recess accompanied by a T2 dark band: a new finding for diagnosing placental invasion. Abdom Radiol (NY) 2017;42:2146-53. [Crossref] [PubMed]
- Zhang J, Xu H, Xin Y, Zhang C, Liu Z, Han X, Liu Q, Li Y, Huang Z. Assessment of the massive hemorrhage in placenta accreta spectrum with magnetic resonance imaging. Exp Ther Med 2020;19:2367-76. [Crossref] [PubMed]
- Goergen SK, Posma E, Wrede D, Collett J, Pyman J, Alibrahim E, Keene J, Dobrotwir A. Interobserver agreement and diagnostic performance of individual MRI criteria for diagnosis of placental adhesion disorders. Clin Radiol 2018;73:908.e1-9. [Crossref] [PubMed]
- Fukushima K, Fujiwara A, Anami A, Fujita Y, Yumoto Y, Sakai A, Morokuma S, Wake N. Cervical length predicts placental adherence and massive hemorrhage in placenta previa. J Obstet Gynaecol Res 2012;38:192-7. [Crossref] [PubMed]
- Polat M, Kahramanoglu I, Senol T, Ozkaya E, Karateke A. Shorter the cervix, more difficult the placenta percreta operations. J Matern Fetal Neonatal Med 2016;29:2327-31. [Crossref] [PubMed]
- Stafford IA, Dashe JS, Shivvers SA, Alexander JM, McIntire DD, Leveno KJ. Ultrasonographic cervical length and risk of hemorrhage in pregnancies with placenta previa. Obstet Gynecol 2010;116:595-600. [Crossref] [PubMed]
- van der Ploeg T, Austin PC, Steyerberg EW. Modern modelling techniques are data hungry: a simulation study for predicting dichotomous endpoints. BMC Med Res Methodol 2014;14:137. [Crossref] [PubMed]