Preoperative differentiation of pancreatic cystic neoplasm subtypes on computed tomography radiomics
Introduction
Pancreatic cystic neoplasms (PCNs) represent a heterogeneous group of cystic lesions that mainly consist of serous cystic neoplasms (SCNs), mucinous cystic neoplasms (MCNs), intraductal papillary mucinous neoplasms (IPMNs), solid pseudopapillary neoplasms (SPNs), and cystic pancreatic neuroendocrine tumors (PNETs), in which the IPMN, SCN, and MCN are the major types of PCNs (1,2). Among PCNs, MCN and IPMN are important precursor lesions that provide a greater likelihood for the screening and early diagnosis of pancreatic cancer (3,4). The ability to differentially diagnose the 3 PCN subtypes of SCN, MCN, and IPMN ahead of treatment is necessary to improve the management and clinical outcomes due to the variable potential of malignancy (5). IPMN and MCN patients with high malignant potential are mostly required to either undergo surgical resection or lifelong follow-up for surveillance of disease progression (6,7). Conversely, SCN is considered a benign lesion; surgery and follow-up are not recommended unless specific symptoms exist (8).
The Pancreatic Surgery Department of the Chinese Academic Society of Young Surgeons retrospectively analyzed clinical data of PCN patients in 16 top pancreatic centers in 2018 (9). A total of 2,251 patients with PCN underwent surgical resection, of whom 678 (30.1%) were postoperatively diagnosed with SCN in pathology. The number of correct diagnoses of PCN subtypes based on preoperative computed tomography (CT) examination was only 659 (33.0%). Therefore, most patients could avoid unnecessary surgical resection if SCN could be accurately diagnosed. Presently, the majority of PCNs are incidentally detected on abdominal CT examinations, and thus, these CT scans provide the available imaging dataset for diagnostic studies (10,11). The pancreatic protocol of CT and gadolinium-enhanced magnetic resonance imaging (MRI) with magnetic resonance cholangiopancreatography (MRCP) comprises the main diagnostic imaging modalities for the identification of different PCNs subtypes. Endoscopic ultrasound (EUS)-based techniques for PCNs are recognized as the complementary means to obtain a more precise diagnosis (12-15) Despite promising advances in imaging techniques, the distinction between the various PCN types still represents a great clinical challenge for practicing physicians (16). The correct diagnosis of PCNs before treatment is essential for clinical decision-making.
The exponential advances in medical image analysis have facilitated the progression of the novel field called radiomics, which represents the processes for the high-throughput extraction of numerous quantitative features from medical images (17,18). Radiomics provides great potential to assess the extensive inter- and intra-tumoral heterogeneity hidden in radiologic images and is a well-known noninvasive approach (19). The role of radiomics is committed to becoming an important adjunct to clinical decision making in 2 aspects of diagnosis and prognosis (20-22). Many researchers have provided reviews of the current clinical application of radiomics in the field of pancreatic tumors and concluded that radiomics plays an important role in improving the non-invasive evaluation of pancreatic lesions (23-26). Xie et al. found that CT-based radiomics showed good potential in preoperatively discriminating MCN from atypical serous cystadenomas (ASCN) (27). Shen et al. provided evidence that CT-based radiomics analysis was reliable to differentiate SCN, MCN, and IPMN (28). Wei et al. proposed a preoperative CT-based radiomics diagnosis scheme to differentiate SCN from other PCNs (29). On the basis of previous studies, our study attempted to segment pancreatic cystic tumors automatically with a deep-learning segmentation algorithm, establish binary- and multiclass prediction models, and further improve the diagnostic efficiency. Nevertheless, there is still an urgent demand to improve the diagnostic performance of radiomics-based models.
Our study aimed to construct an automated model to enable precise diagnosis of PCNs, ultimately assisting the involved physicians in making optimized treatment decisions. We present this article in accordance with the TRIPOD reporting checklist (30) (available at https://qims.amegroups.com/article/view/10.21037/qims-22-1192/rc).
Methods
Patients
Between February 2015 and December 2020, 450 consecutive patients with PCNs who underwent curative resection at Nanjing Drum Tower Hospital were retrospectively evaluated (Figure 1). The inclusion criteria were as follows: (I) postoperative pathological diagnosis of MCN, SCN, or IPMN; (II) identifiable cystic lesion in contrast-enhanced CT images within 3 weeks before curative resection; (III) no systemic or focal therapy before CT examination and surgery; and (IV) available clinicopathological parameters. The exclusion criteria were as follows: (I) complicated with other hepato-biliary-pancreatic malignant tumors (n=27); (II) artifacts on CT images in the arterial phase or venous phase (n=9); and (III) difficulty in outlining the margin of the tumor because of the size (maximum diameter <1 cm) and poor contrast of the lesion (n=10).
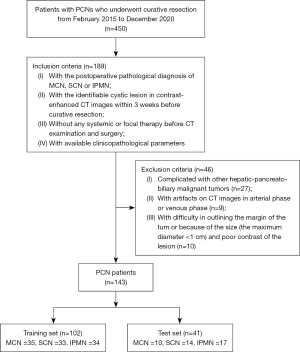
The collected clinical and pathological data included patient age, sex, abdominal symptoms, chronic pancreatitis history, history of diabetes, lesion location (head and neck, body and tail, uncinate, and diffuse), the number of tumors (solitary or multiple), tumor maximum diameter, CT value of tumor, calcification, pancreatic duct dilatation, bile duct dilatation, serum alanine aminotransferase (ALT), serum aspartate aminotransferase (AST), serum albumin (ALB), and serum tumor markers [α-fetoprotein (AFP), carbohydrate antigen 19-9 (CA19-9), carcinoembryonic antigen (CEA), carbohydrate antigen 125 (CA125), carbohydrate antigen 72-4 (CA72-4); carbohydrate antigen 242 (CA242)]. Preoperative clinical data and postoperative pathological information of the included patients were obtained from standard-of-care medical records. The study was conducted in accordance with the Declaration of Helsinki (as revised in 2013). The study was approved by the Ethics Committee of the Nanjing Drum Tower Hospital and the requirement for individual consent for this retrospective analysis was waived.
CT technique
All CT examinations were performed with a multidetector spiral CT scanner (LightSpeed, VCT, or Discovery HD750; GE Healthcare, Chicago, IL, USA). The parameters of contrast-enhanced CT scans were as follows: tube voltage 120 kVp, tube current 250–300 mAs, slice thickness: 1.25 mm, slice interval: 1.25 mm, matrix: 512×512, rotation time: 0.6 s, helical pitch 1.375, and field of view: 35–40 cm. All patients were required to fast for at least 6–8 hours before the CT scan and were placed in the supine position with their arms raised during the scanning. The scan range was from the dome of the diaphragm to the lower pole of the kidney. After the unenhanced CT scan, iodinated contrast agent (Omnipaque 350 mgI/mL; GE Healthcare, USA) at a rate of 1.5 mL/kg was injected intravenously with a high-pressure syringe (Medrad Stellant CT injector system; Indianola, PA, USA). The pancreatic phase and portal venous phase of contrast enhancement were obtained at 40–50 and 65–70 s, respectively, after the injection of the contrast agent. The mean interval between the CT scan and surgery was 9±4 days (range, 7–20 days).
CT images acquisition and segmentation
The radiomics workflow is shown in Figure 2. Contrast-enhanced CT images of PCNs with 1.25-mm thickness [conforming to digital imaging and communications in medicine (DICOM)] were obtained from the Picture Archiving and Communication System (PACS). The pancreatic phase of the contrast-enhanced CT images revealed the distinct regional difference in contrast sensitivity between the normal pancreas tissue and pancreatic cystic tumor, which was selected for analysis of the tumor heterogeneity and correlation with clinical parameters and imaging features.
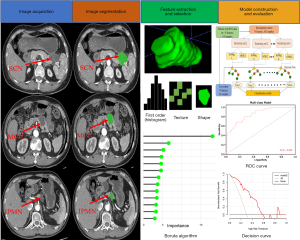
The regions of interest (ROIs) of the whole tumor area were automatically segmented with a deep-learning segmentation algorithm for the study (31). We used a U-Net-like (32) architecture as the base network which is the most popular architecture for medical image segmentation. It contains an encoder to extract multi-scale features from input CT images and a decoder to aggregate the features and generate voxel-wise predictions. The decoder reconstructs the semantic information based on the multi-scale features extracted from the encoder. Skip connections are also used to bridge the encoder and decoder feature maps for better backpropagation and more precise localization of segmentation targets. Moreover, to improve the segmentation accuracy of lesions, we added a level set function regression branch at the end of the decoder (31), which can guide the predicted segmentation contours to lesion boundaries. To train the network, the whole dataset was split into either a training or a testing set with a 4:1 ratio, resulting in a training set and a testing set with 115 and 28 patients, respectively. The network is optimized by the stochastic gradient descent algorithm and initial learning rate is 3e-4 with a minibatch size of 2. The training takes 1,000 epochs and each epoch contains 250 minibatches. The loss function is the unweighted sum between Dice loss and cross-entropy loss, which has been shown to be one of the most robust loss functions (33). To avoid overfitting, we applied data augmentations, including rotation, intensity shifting, gamma correction, and deformation.
After segmented with a deep-learning segmentation algorithm, the ROI of the whole tumor area was corrected on each section by 2 radiologists (Zhang F and Wu J, with 4 and 10 years of experience in abdominal imaging, respectively) using ITK-SNAP (version 3.8.0; http://www.itksnap.org/), avoiding the pancreatic parenchyma and large vessels around the tumor and adjacent abdominal organs. The 2 radiologists were blinded to the clinicopathological information of all the patients during the period of segmentation. The radiologists performed the segmentation twice in a 2-week independent manner to evaluate inter- and intra-observer reproducibility to determine the stability of each radiomic feature extracted from the ROIs.
Radiomic feature extraction and selection
Image preprocessing and radiomic feature extraction were implemented by the open-source PyRadiomics package (version 3.0.1, https://www.radiomics.io/) (34), which is implemented in Python. Resampling-based approaches and normalization techniques were performed to improve the repeatability, stability, and accuracy through the standardized process of feature extraction in PyRadiomics. The voxel size of CT images was resampled to 1×1×1 mm3; image intensity was normalized by discretizing values of voxel intensity as 64 gray levels for the inhomogeneity of spatial intensity. A total of 1,218 radiomics features were extracted from each of the 3-dimensional (3D) segmentations in the pancreatic phase, which consisted of first-order statistics (n=19), shape descriptors (3D, n=16), 75 texture features [gray level co-occurrence matrix (GLCM), gray level size zone matrix (GLSZM), gray level run length matrix (GLRLM), neighbouring gray tone difference matrix (NGTDM), gray level dependence matrix (GLDM); n=75], higher-order features selected by wavelet filter (n=688) and Laplacian of Gaussian (LoG) filter (n=430). Subsequently, all feature values were centered and standardized by z scores for all analyses.
The feature selection process was conducted to follow the 3 steps for identifying the robust characteristics. Before feature screening, all data were normalized to the range [0, 1], avoiding the impact of significant discrepancies between the values. The specific process of feature selection was as follows. In the first step, we excluded the radiomic features with poor stability from the dataset (intraclass correlation coefficients lower than 0.75) in the assessment of test-retest and interobserver agreement. Subsequently, the Pearson correlation test was employed to filter out the redundant features with an average and absolute coefficient higher than 0.8. Ultimately, the Boruta algorithm was applied to select the important features for the establishment of a radiomics signature by the random forest (RF) classifier as highly related to differential diagnosis in PCNs.
Model development and validation
We developed 2 types of preoperative radiomics models by RF classifier: the multiclass prediction model was constructed to discriminate 3 PCN subtypes; the binary-class prediction models made pairwise classifications among the 3 subtypes of MCN, SCN, and IPMN. The above radiomics models included the radiomics signature and significant clinical-radiologic characteristics before surgery. An overview of the RF modeling process is shown in Figure 3. Univariate analysis of clinical-radiologic parameters with a P value lower than 0.1 was entered into multivariable analysis. The final risk factors among the clinical and imaging features were selected using multivariable logistic regression by the stepwise backward method to eliminate the nonsignificant features. All models were validated in an independent test cohort.
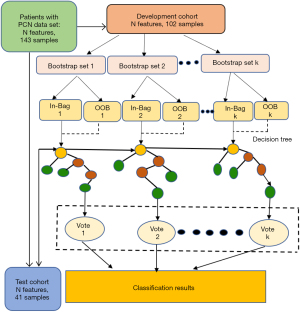
Statistical analysis
The Shapiro-Wilk test and Levene test were used for normality and homogeneity of variances, respectively. Continuous variables were expressed as medians with interquartile ranges in parentheses, and categorical variables were expressed as total numbers and frequencies. Univariate analysis was performed to analyze clinical-radiologic parameters: chi-square test, corrected chi-square test, or Fisher test was used for categorical variables; independent sample t-test or Mann-Whitney U test was used for continuous variables. Multivariate logistic regression analysis was applied to select the important predictors of clinical parameters. The radiomics signature was built based on the selected radiomics features by the package “Boruta” in R. Receiver operating characteristic (ROC) curve analysis was used to evaluate the predictive efficiency of the model. The area under the curve (AUC), sensitivity, and specificity were obtained to assess the performance of the prediction model. Decision curve analysis (DCA) was performed to evaluate the net benefits of the prediction models. The statistical analysis was conducted with software (R, version 4.1.0; R Foundation for Statistical Computing, Vienna, Austria). A two-tailed P value less than 0.05 was considered statistically significant.
Results
Patients characteristics of the study cohorts
Eventually, from February 2015 to December 2020, a total of 143 patients were enrolled in our study cohort. The dataset of patients with PCN was randomly divided into 2 groups at a ratio of 7:3: the development and test cohorts. The development cohort consisted of 102 patients (MCN 35, SCN 33, IPMN 34), and the test cohort consisted of 41 patients (MCN 10, SCN 14, IPMN 17). The preoperative clinical-radiologic characteristics of all PCN patients were not significantly different in the development and test cohorts, as shown in Table 1. The proportions of males and females in the population of MCN, SCN and IPMN patients were 1:6.5, 1:2.9, and 1:0.4, respectively; the median ages with interquartile ranges were 60 (47.75, 68.25) years, 55 (44.00, 62.00) years, and 66 (61.00, 71.00) years, respectively. Comparison of consistency between preoperative radiological and postoperative pathological diagnosis showed that the diagnostic accuracy and precision of preoperative imaging findings in PCN patients were relatively low. The rates of accurate diagnosis were 16.67%, 10.64%, and 56.86%, respectively, for MCN, SCN, and IPMN. Among the 3 subtypes, the percentages of PCN patients with ambiguous diagnoses were 73.33%, 70.21%, and 39.22% (MCN, n=33; SCN, n=33; IPMN, n=22), respectively. In our institution, the misdiagnosis rates of MCN, SCN, and IPMN patients were 10.00%, 19.15%, and 3.92%, respectively.
Table 1
Characteristics | Development cohort (n=102) | Test cohort (n=41) | P value |
---|---|---|---|
Age (years)* | 54.5 (43.0, 66.0) | 61.0 (42.0, 67.0) | 0.449 |
Sex | 0.132 | ||
Female | 59 (57.8) | 18 (43.1) | |
Male | 43 (42.2) | 23 (56.1) | |
Primary tumor type | 0.670 | ||
MCN | 35 (34.3) | 10 (24.4) | |
SCN | 33 (32.4) | 14 (34.1) | |
IPMN | 34 (33.3) | 17 (41.5) | |
Chronic pancreatitis | 0.558 | ||
Present | 7 (6.9) | 4 (9.8) | |
Absent | 95 (93.1) | 37 (90.2) | |
Abdominal symptom | 0.855 | ||
Present | 53 (52.0) | 22 (53.7) | |
Absent | 49 (48.0) | 19 (46.3) | |
History of diabetes | 0.300 | ||
Present | 7 (6.9) | 5 (12.2) | |
Absent | 95 (93.1) | 36 (87.8) | |
Serum AFP (ng/mL)* | 2.0 (1.3, 3.1) | 1.8 (1.3, 2.6) | 0.115 |
Serum CA19-9 (U/mL)* | 10.2 (6.3, 15.5) | 10.4 (6.5, 10.4) | 0.641 |
Serum CEA (ng/mL)* | 0.8 (0.5, 1.8) | 1.0 (0.5, 2.0) | 0.250 |
Serum CA125 (U/mL)* | 8.7 (5.4, 11.1) | 9.3 (6.8, 11.6) | 0.507 |
Serum CA72-4 (U/mL)* | 1.5 (1.0, 2.7) | 1.7 (1.1, 3.1) | 0.809 |
Serum CA242 (U/mL)* | 4.3 (3.0, 7.6) | 4.0 (2.3, 6.1) | 0.724 |
Serum ALT (U/L)* | 15.9 (11.9, 22.4) | 18.7 (15.2, 23.9) | 0.554 |
Serum AST (U/L)* | 18.7 (15.2, 23.9) | 18.8 (16.0, 24.0) | 0.746 |
Serum ALB (g/L)* | 40.1 (39.5, 42.1) | 40.8 (38.6, 42.5) | 0.465 |
Lesion location | 0.370 | ||
Head and neck | 33 (32.4) | 19 (46.3) | |
Body and tail | 50 (49.0) | 16 (39.0) | |
Uncinate | 12 (11.8) | 2 (4.9) | |
Diffuse | 7 (6.9) | 4 (9.8) | |
Tumor diameter (cm)* | 2.83 (1.85, 4.63) | 2.81 (2.17, 4.15) | 0.857 |
Tumor number | 0.400 | ||
Solitary | 79 (77.5) | 29 (70.7) | |
Multiple | 23 (22.5) | 12 (29.3) | |
Mean CT value (HU)* | 37 (−8, 64) | 26 (−10, 59) | 0.381 |
Calcification | 0.595 | ||
Present | 16 (15.7) | 5 (12.2) | |
Absent | 86 (84.3) | 36 (87.8) | |
Pancreatic duct dilatation | 0.060 | ||
Present | 55 (53.9) | 30 (73.2) | |
Absent | 47 (46.1) | 11 (26.8) | |
Bile duct dilatation | 0.808 | ||
Present | 14 (13.7) | 5 (12.2) | |
Absent | 88 (86.3) | 36 (87.8) |
Except where indicated, data are numbers of patients, with percentages in parentheses. *, data are medians, with parentheses are the interquartile range. MCN, mucinous cystic neoplasm; SCN, serous cystic neoplasm; IPMN, intraductal papillary mucinous neoplasm; AFP, a-fetoprotein; CA19-9, carbohydrate antigen 199; CEA, carcinoembryonic antigen; CA125, carbohydrate antigen 125; CA72-4, carbohydrate antigen 72-4; CA242, carbohydrate antigen 242; ALT, alanine aminotransferase; AST, aspartate aminotransferase; ALB, albumin; CT, Computed tomography; HU, Hounsfield units.
Univariate and multivariate analysis of clinico-radiologic parameters
Table 1 shows that the researchers retrospectively collected and analyzed baseline characteristics that were considered clinically relevant to the outcome of diagnosis. Univariate logistic regression analysis of the clinical data and radiological features indicated that age, sex, abdominal symptoms, AFP, CEA, ALT, AST, tumor diameter, calcification, bile duct dilatation, and lesion location were statistically significant (P<0.1) between the 3 subtypes in the development cohort. Next, the significant variables were entered into multivariate logistic regression to obtain the risk factors for the diagnosis of PCNs. The statistical data of the multivariate analysis are shown in Table 2. The results indicated that age (P=0.001, 0.005, 0.041), sex (P=0.005, 0.041, 0.017), and tumor diameter (P<0.001, 0.020, 0.021) were independent risk factors for differential diagnosis between MCN and SCN, MCN and IPMN, and SCN and IPMN, respectively.
Table 2
Characteristics | MCN vs. SCN | MCN vs. IPMN | SCN vs. IPMN | |||||
---|---|---|---|---|---|---|---|---|
OR (95% CI) | P value | OR (95% CI) | P value | OR (95% CI) | P value | |||
Age (years) | 0.863 (0.792, 0.941) | 0.001* | 0.598 (0.418, 0.857) | 0.005* | 0.693 (0.487, 0.985) | 0.041* | ||
Sex (M/F) | 0.122 (0.010, 1.549) | 0.005* | 32.017 (1.564, 65.520) | 0.041* | 262.102 (9.525, 725.121) | 0.017* | ||
Abdominal symptom | 1.353 (0.296, 6.180) | 0.696 | 0.042 (0.000, 4.422) | 0.182 | 0.031 (0.000, 2.951) | 0.135 | ||
CEA (ng/mL) | 3.660 (0.831, 16.112) | 0.086 | 2.236 (0.089, 56.235) | 0625 | 0.611 (0.026, 14.307) | 0.759 | ||
AFP (ng/mL) | 2.261 (0.958, 5.336) | 0.062 | 1.266 (0.429, 3.730) | 0.669 | 0.560 (0.192, 1.629) | 0.287 | ||
ALT (U/L) | 0.828 (0.668, 1.026) | 0.084 | 1.022 (0.720, 1.450) | 0.905 | 1.233 (0.893, 1.703) | 0.202 | ||
AST (U/L) | 1.151 (0.914, 1.448) | 0.232 | 0.779 (0.484, 1.254) | 0.303 | 0.677 (0.415, 1.103) | 0.118 | ||
Tumor diameter (cm) | 1.310 (1.160, 11.126) | <0.001* | 33.913 (1.732, 66.388) | 0.020* | 33.446 (1.709, 645.413) | 0.021* | ||
Calcification | 3.234 (0.534, 19.577) | 0.201 | 1.463 (0.000, 30.172) | 0.994 | 45.249 (0.000, 94.340) | 0.994 | ||
Bile duct dilatation | 2.316 (0.075, 60.672) | 0.657 | 0.368 (0.004, 34.070) | 0.665 | 0.172 (0.003, 11.115) | 0.408 | ||
Lesion location | 2.136 (0.075, 60.672) | 0.657 | 17.143 (0.001, 214.380) | 0.242 | 69.886 (0.001, 403.815) | 0.483 |
*, P<0.05. MCN, mucinous cystic neoplasm; SCN, serous cystic neoplasm; IPMN, intraductal papillary mucinous neoplasm; OR, odds ratio; CI, confidence interval; M, male; F, female; CEA, carcinoembryonic antigen; AFP, α-fetoprotein; ALT, alanine aminotransferase; AST, aspartate aminotransferase.
Radiomic feature selection and signature construction
Among 1,218 radiomics features extracted from CT images, 866 features were selected by evaluation of the intraclass correlation coefficient (ICC >0.75). Then, the Pearson correlation test was used to exclude 722 features with high correlation coefficients.
The Boruta algorithm was conducted to screen 13 features to construct a radiomics signature by RF analysis. The important features selected from the Boruta algorithm are presented in Figure 4. The radiomics signature demonstrated good prediction ability with an out-of-bag (OOB) error of 0.317 and a C-index of 0.772 in the test cohort, and the diagnostic performance is summarized in Table 3.
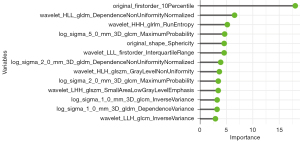
Table 3
P/T | Development cohort | Test cohort | |||||||||||
---|---|---|---|---|---|---|---|---|---|---|---|---|---|
SCN | MCN | IPMN | Pre | Rec | F1 | SCN | MCN | IPMN | Pre | Rec | F1 | ||
SCN | 23 | 5 | 4 | 0.719 | 0.697 | 0.708 | 6 | 0 | 2 | 0.750 | 0.429 | 0.546 | |
MCN | 7 | 29 | 1 | 0.784 | 0.829 | 0.806 | 2 | 9 | 2 | 0.692 | 0.692 | 0.692 | |
IPMN | 3 | 1 | 29 | 0.879 | 0.853 | 0.866 | 6 | 1 | 13 | 0.650 | 0.765 | 0.698 | |
Total | 33 | 35 | 34 | OA: 0.794 | 14 | 10 | 17 | OA: 0.683 |
P, predicted type; T, true type; SCN, serous cystic neoplasm; MCN, mucinous cystic neoplasm; IPMN, intraductal papillary mucinous neoplasm; Pre, precision; Rec, recall; OA, overall accuracy. Precision = true positive/(true positive + false positive); recall = true positive/(true positive + false negative); F1-score = 2 × precision × recall/(precision + recall).
Prediction models development and validation
The radiomics-based models were established by 3 significant clinical-radiologic parameters at multivariable analysis and a radiomics signature in the development cohort.
The multiclass prediction model indicated that the classification error (OOB estimate) reached stability with a minimum value of 19.61% when the number of trees was more than 500, and 3 variables were tried at each split. Figure 5 illustrates the relationship between the error rate and the number of trees in the process of multiclass model construction. In the development dataset, the multiclass radiomics model had an overall accuracy of 0.804 and precision of 0.800, 0.727, and 0.929 for SCN, MCN, and IPMN, respectively (Table 4). In the test dataset, the overall accuracy to classify triple tumors was 0.707, and the precision of identification for SCN, MCN, and IPMN was 0.750, 0.667, and 0.722, respectively.
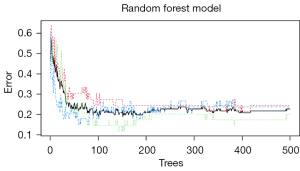
Table 4
P/T | Development cohort | Test cohort | |||||||||||
---|---|---|---|---|---|---|---|---|---|---|---|---|---|
SCN | MCN | IPMN | Pre | Rec | F1 | SCN | MCN | IPMN | Pre | Rec | F1 | ||
SCN | 24 | 2 | 4 | 0.800 | 0.727 | 0.762 | 6 | 0 | 2 | 0.750 | 0.429 | 0.546 | |
MCN | 8 | 32 | 4 | 0.727 | 0.914 | 0.810 | 3 | 10 | 2 | 0.667 | 1.000 | 0.800 | |
IPMN | 1 | 1 | 26 | 0.929 | 0.765 | 0.839 | 5 | 0 | 13 | 0.722 | 0.765 | 0.743 | |
Total | 33 | 35 | 34 | OA: 0.804 | 14 | 10 | 17 | OA: 0.707 |
Precision = true positive/(true positive + false positive); recall = true positive/(true positive + false negative); F1-score = 2 × precision × recall/(precision + recall). P, predicted type; T, true type; SCN, serous cystic neoplasm; MCN, mucinous cystic neoplasm; IPMN, intraductal papillary mucinous neoplasm; Pre, precision; Rec, recall; OA, overall accuracy.
The binary-class radiomics models were composed of 3 classification models to distinguish between SCN and MCN, MCN and IPMN, SCN and IPMN. The SCN-MCN model, MCN-IPMN model and SCN-IPMN model showed overall accuracies of 0.853, 0.928, and 0.866 in the development cohort and 0.750, 0.889, and 0.839 in the test cohort, respectively (Tables 5-7). For the SCN-MCN model, the precision was 0.871 and 0.838 in the development dataset (Table 5). For the MCN-IPMN model, the precision was 0.917 and 0.939 in the development cohort (Table 6). Meanwhile, the precision of the SCN-IPMN model was 0.853 and 0.879 in the development cohort, as shown in Table 7.
Table 5
P/T | Development cohort | Test cohort | |||||||||
---|---|---|---|---|---|---|---|---|---|---|---|
SCN | MCN | Pre | Rec | F1 | SCN | MCN | Pre | Rec | F1 | ||
SCN | 27 | 4 | 0.871 | 0.818 | 0.844 | 8 | 0 | 1.000 | 0.571 | 0.727 | |
MCN | 6 | 31 | 0.838 | 0.886 | 0.861 | 6 | 10 | 0.625 | 1.000 | 0.769 | |
Total | 33 | 35 | OA: 0.853 | 14 | 10 | OA: 0.750 |
Precision = true positive/(true positive + false positive); recall = true positive/(true positive + false negative); F1-score = 2 × precision × recall/(precision + recall). SCN, serous cystic neoplasm; MCN, mucinous cystic neoplasm; P, predicted type; T, true type; Pre, precision; Rec, recall; OA, overall accuracy.
Table 6
P/T | Development cohort | Test cohort | |||||||||
---|---|---|---|---|---|---|---|---|---|---|---|
MCN | IPMN | Pre | Rec | F1 | MCN | IPMN | Pre | Rec | F1 | ||
MCN | 33 | 3 | 0.917 | 0.943 | 0.930 | 10 | 3 | 0.769 | 1.000 | 0.869 | |
IPMN | 2 | 31 | 0.939 | 0.912 | 0.925 | 0 | 14 | 1.000 | 0.823 | 0.903 | |
Total | 35 | 34 | OA: 0.928 | 10 | 17 | OA: 0.889 |
Precision = true positive/(true positive + false positive); recall = true positive/(true positive + false negative); F1-score = 2 × precision × recall/(precision + recall). MCN, mucinous cystic neoplasm; IPMN, intraductal papillary mucinous neoplasm; P, predicted type; T, true type; Pre, precision; Rec, recall; OA, overall accuracy.
Table 7
P/T | Development cohort | Test cohort | |||||||||
---|---|---|---|---|---|---|---|---|---|---|---|
SCN | IPMN | Pre | Rec | F1 | SCN | IPMN | Pre | Rec | F1 | ||
SCN | 29 | 5 | 0.853 | 0.879 | 0.866 | 11 | 2 | 0.846 | 0.786 | 0.828 | |
IPMN | 4 | 29 | 0.879 | 0.853 | 0.866 | 3 | 15 | 0.833 | 0.882 | 0.857 | |
Total | 33 | 34 | OA: 0.866 | 14 | 17 | OA: 0.839 |
Precision = true positive/(true positive + false positive); recall = true positive/(true positive + false negative); F1-score = 2 × precision × recall/(precision + recall). SCN, serous cystic neoplasm; IPMN, intraductal papillary mucinous neoplasm; P, predicted type; T, true type; Pre, precision; Rec, recall; OA, overall accuracy.
All binary class prediction models virtually presented higher overall accuracy and F1-score than the multiclass prediction model in both the development and test cohorts, especially the models for diagnosis classification MCN and IPMN. By analyzing the ROC curve in the test cohort, the multiclass radiomics model integrating radiomic and clinical-radiologic features improved the diagnostic accuracy and efficacy compared with the radiomics signature (Figure 6). The value of AUC was 0.772 (0.589, 0.955) and 0.850 (0.696, 1.000), respectively. However, the binary-class radiomics model showed the best discriminatory ability, with AUC values of 0.914 (0.786, 1.000) for SCN and MCN, 0.863 (0.714, 0.941) for SCN and IPMN, and 0.926 (0.824, 1.000) for MCN and IPMN in the test cohort.
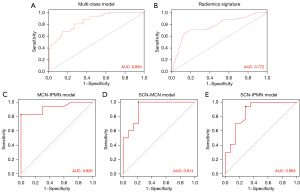
The calibration curve demonstrated that the model-predicted subtype was calibrated with the pathologically confirmed subtype in the binary-class radiomics models (Figure 7). With DCA, 3 binary-class prediction models displayed promising net benefit under the suitable range of threshold probabilities in the test dataset. As shown in Figure 8, the nomogram was performed to visualize the binary-class radiomics models and provide the predicted probability of tumor subtypes for the individuals.
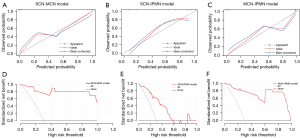
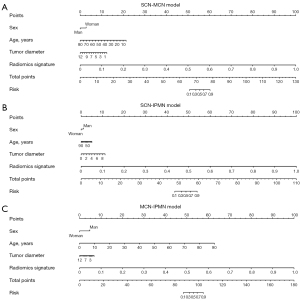
Discussion
The study retrospectively enrolled patients pathologically diagnosed with 3 subtypes of PCNs to predict the precisive histological types preoperatively. The RF classifier was the optimal algorithm selected from the multiple radiomics methods used to construct all the models. Ultimately, all the classification models were composed of 3 significant clinico-radiologic characteristics (age, sex, tumor diameter) and a radiomics signature with 13 radiomics features in the development cohort.
The objective of our research was to enhance the diagnostic efficacy of the preoperative prediction model compared with other clinical and radiomics models in previous study. Dmitriev et al. described an automatic classification algorithm to classify the subtypes of PCNs based on CT images. Their study contained 134 patients and the ensemble classifier, combining 14 selected quantitative features (age, sex, cyst location, intensity, and shape features), established by the RF and convolutional neural network algorithm, showed good accuracy in the differential diagnosis of PCNs (accuracy 83.6%) (35). Shen et al. aimed to investigate the feasibility of using CT based radiomics to preoperatively classify SCN, MCN, and IPMN. In their study, 164 patients were enrolled and they found that the RF model including 4 important clinical characteristics (age, sex, serum CA19-9, serum CEA) and 5 radiomics features presented the highest discrimination ability of differential diagnosis for SCN, MCN, and IPMN than the support vector machine (SVM) model and the artificial neural network (ANN) model (84% in the training dataset and 80% in the validation dataset) (28). In our study, although distinctive clinical and radiologic characteristics were selected to establish the model for diagnosis prediction of the 3 subtypes, our study found similar results to the previous study. The wavelet-based and log-based radiomics features occupied an important place in the signature. According to previous reports, the above radiomics features represent the heterogeneity of tumor tissue and the tumor microenvironment from multiple dimensions of the space. The binary class model showed a higher overall accuracy of 85.3%, 86.6%, and 92.8% in the development cohort and 75.0%, 83.9%, and 88.9% in the test cohort. The radiomics models all achieved good AUC values and calibration results in the test cohort.
In view of clinical practicability, we further developed 3 binary-class models for SCN, MCN, and IPMN. In clinical work, many radiologists are often able to exclude a type of cystic tumor based on the imaging findings, and make mistakes in the diagnosis of the remaining 2 types of cystic tumors. Apparently, the binary-class radiomics models showed better predictive performances with high AUC values than the multiclass radiomics model in the test cohort. Among the 3 binary-class classification models, the SCN-IPMN model provided the highest AUC value and presented excellent net benefit in the DCA in the test cohort. Using the nomogram to visualize diagnosis prediction models, the analysis of the nomogram indicated that the binary-class radiomics models had a potential ability to differentiate the diagnosis of cystic pancreatic neoplasms. The binary-class prediction models displayed a higher overall accuracy in both the development and test datasets than the multiclass prediction model and it can help radiologists make accurate diagnosis.
Compared to previous studies, our study had a distinct advantage in the image segmentation method. The ROI of cystic neoplasms was achieved by using automatic segmentation via a new deep-learning network system. The explicit introduction of targets’ geometric information in the deep learning network was helpful to obtain a better segmentation boundary (31). The proposed segmentation method leads to significant improvements in the stability and robustness of radiomic features.
Our research has some limitations. First, all the datasets were from a single medical center, and the number of included PCN patients was slightly limited, although internal validation was applied to enhance the reliability of the radiomics models. The absence of external validation was the main limitation. Second, the study was a retrospective analysis, which resulted in potential selection bias. Third, all of the contrast-enhanced CT images were acquired with the same CT scanner and fixed parameters. Therefore, the reproducibility and stability of radiomics features extracted from CT images requires further validation in multiple brands of CT scanners. Finally, the dataset was only committed to classifying the 3 most common PCNs. In the future, the other cystic subtypes will be included in the dataset.
Conclusions
The radiomics model consisting of clinical-radiologic characteristics and radiomics features based on enhanced CT images demonstrates promising diagnostic performance and discrimination ability for subtypes of PCNs. The binary-class prediction models are superior to the multiclass prediction model in terms of the overall accuracy of diagnosis. Further studies are required to improve the clinical utility of the radiomics models to help surgeons provide precise diagnostic information for PCN patients.
Acknowledgments
We would like to express our great appreciation to the R Development Core Team and contributors for R packages used in our study. We thank Jun Ma from the Department of Mathematics, Nanjing University of Science and Technology for supporting the new deep-learning network system to obtain a better segmentation boundary.
Funding: This project was supported by
Footnote
Reporting Checklist: The authors have completed the TRIPOD reporting checklist. Available at https://qims.amegroups.com/article/view/10.21037/qims-22-1192/rc
Conflicts of Interest: All authors have completed the ICMJE uniform disclosure form (available at https://qims.amegroups.com/article/view/10.21037/qims-22-1192/coif). The authors report that this project was supported by China’s Ministry of Science and Technology (No. 2020YFA0713800) and the National Natural Science Foundation of China (No. 12090023). The authors have no other conflicts of interest to declare.
Ethical Statement: The authors are accountable for all aspects of the work in ensuring that questions related to the accuracy or integrity of any part of the work are appropriately investigated and resolved. The study was conducted in accordance with the Declaration of Helsinki (as revised in 2013). The study was approved by the Ethics Committee of the Nanjing Drum Tower Hospital and the requirement for individual consent for this retrospective analysis was waived.
Open Access Statement: This is an Open Access article distributed in accordance with the Creative Commons Attribution-NonCommercial-NoDerivs 4.0 International License (CC BY-NC-ND 4.0), which permits the non-commercial replication and distribution of the article with the strict proviso that no changes or edits are made and the original work is properly cited (including links to both the formal publication through the relevant DOI and the license). See: https://creativecommons.org/licenses/by-nc-nd/4.0/.
References
- Kromrey ML, Bülow R, Hübner J, Paperlein C, Lerch MM, Ittermann T, Völzke H, Mayerle J, Kühn JP. Prospective study on the incidence, prevalence and 5-year pancreatic-related mortality of pancreatic cysts in a population-based study. Gut 2018;67:138-45. [Crossref] [PubMed]
- van Huijgevoort NCM, Del Chiaro M, Wolfgang CL, van Hooft JE, Besselink MG. Diagnosis and management of pancreatic cystic neoplasms: current evidence and guidelines. Nat Rev Gastroenterol Hepatol 2019;16:676-89. [Crossref] [PubMed]
- Lennon AM, Wolfgang CL, Canto MI, Klein AP, Herman JM, Goggins M, Fishman EK, Kamel I, Weiss MJ, Diaz LA, Papadopoulos N, Kinzler KW, Vogelstein B, Hruban RH. The early detection of pancreatic cancer: what will it take to diagnose and treat curable pancreatic neoplasia? Cancer Res 2014;74:3381-9. [Crossref] [PubMed]
- Munigala S, Gelrud A, Agarwal B. Risk of pancreatic cancer in patients with pancreatic cyst. Gastrointest Endosc 2016;84:81-6. [Crossref] [PubMed]
- Keane MG, Afghani E. A Review of the Diagnosis and Management of Premalignant Pancreatic Cystic Lesions. J Clin Med 2021;10:1284. [Crossref] [PubMed]
- European evidence-based guidelines on pancreatic cystic neoplasms. Gut 2018;67:789-804.
- Tanaka M. Clinical Management and Surgical Decision-Making of IPMN of the Pancreas. Methods Mol Biol 2019;1882:9-22.
- Yoon JG, Smith D, Ojili V, Paspulati RM, Ramaiya NH, Tirumani SH. Pancreatic cystic neoplasms: a review of current recommendations for surveillance and management. Abdom Radiol (NY) 2021;46:3946-62. [Crossref] [PubMed]
- The current status of diagnosis and treatment of pancreatic cystic neoplasm in China: a report of 2 251 cases. Zhonghua Wai Ke Za Zhi 2018;56:24-9.
- Okasha HH, Awad A, El-Meligui A, Ezzat R, Aboubakr A, AbouElenin S, El-Husseiny R, Alzamzamy A. Cystic pancreatic lesions, the endless dilemma. World J Gastroenterol 2021;27:2664-80. [Crossref] [PubMed]
- Pandey P, Pandey A, Luo Y, Aliyari Ghasabeh M, Khoshpouri P, Ameli S, O'Broin-Lennon AM, Canto M, Hruban RH, Goggins MS, Wolfgang C, Kamel IR. Follow-up of Incidentally Detected Pancreatic Cystic Neoplasms: Do Baseline MRI and CT Features Predict Cyst Growth? Radiology 2019;292:647-54. [Crossref] [PubMed]
- Facciorusso A, Kovacevic B, Yang D, Vilas-Boas F, Martínez-Moreno B, Stigliano S, et al. Predictors of adverse events after endoscopic ultrasound-guided through-the-needle biopsy of pancreatic cysts: a recursive partitioning analysis. Endoscopy 2022;54:1158-68. [Crossref] [PubMed]
- Crinò SF, Bernardoni L, Gabbrielli A, Capelli P, Salvia R, Rusev BC, Scarpa A, Manfrin E. Beyond Pancreatic Cyst Epithelium: Evidence of Ovarian-Like Stroma in EUS-Guided Through-the-Needle Micro-Forceps Biopsy Specimens. Am J Gastroenterol 2018;113:1059-60. [Crossref] [PubMed]
- Li SY, Wang ZJ, Pan CY, Wu C, Li ZS, Jin ZD, Wang KX. Comparative Performance of Endoscopic Ultrasound-Based Techniques in Patients With Pancreatic Cystic Lesions: A Network Meta-Analysis. Am J Gastroenterol 2023;118:243-55. [Crossref] [PubMed]
- Lisotti A, Napoleon B, Facciorusso A, Cominardi A, Crinò SF, Brighi N, Gincul R, Kitano M, Yamashita Y, Marchegiani G, Fusaroli P. Contrast-enhanced EUS for the characterization of mural nodules within pancreatic cystic neoplasms: systematic review and meta-analysis. Gastrointest Endosc 2021;94:881-889.e5. [Crossref] [PubMed]
- Carmicheal J, Patel A, Dalal V, Atri P, Dhaliwal AS, Wittel UA, Malafa MP, Talmon G, Swanson BJ, Singh S, Jain M, Kaur S, Batra SK. Elevating pancreatic cystic lesion stratification: Current and future pancreatic cancer biomarker(s). Biochim Biophys Acta Rev Cancer 2020;1873:188318. [Crossref] [PubMed]
- Gillies RJ, Kinahan PE, Hricak H. Radiomics: Images Are More than Pictures, They Are Data. Radiology 2016;278:563-77. [Crossref] [PubMed]
- Lambin P, Leijenaar RTH, Deist TM, Peerlings J, de Jong EEC, van Timmeren J, Sanduleanu S, Larue RTHM, Even AJG, Jochems A, van Wijk Y, Woodruff H, van Soest J, Lustberg T, Roelofs E, van Elmpt W, Dekker A, Mottaghy FM, Wildberger JE, Walsh S. Radiomics: the bridge between medical imaging and personalized medicine. Nat Rev Clin Oncol 2017;14:749-62. [Crossref] [PubMed]
- Lambin P, Rios-Velazquez E, Leijenaar R, Carvalho S, van Stiphout RG, Granton P, Zegers CM, Gillies R, Boellard R, Dekker A, Aerts HJ. Radiomics: extracting more information from medical images using advanced feature analysis. Eur J Cancer 2012;48:441-6. [Crossref] [PubMed]
- Limkin EJ, Sun R, Dercle L, Zacharaki EI, Robert C, Reuzé S, Schernberg A, Paragios N, Deutsch E, Ferté C. Promises and challenges for the implementation of computational medical imaging (radiomics) in oncology. Ann Oncol 2017;28:1191-206. [Crossref] [PubMed]
- Liu Z, Wang S, Dong D, Wei J, Fang C, Zhou X, Sun K, Li L, Li B, Wang M, Tian J. The Applications of Radiomics in Precision Diagnosis and Treatment of Oncology: Opportunities and Challenges. Theranostics 2019;9:1303-22. [Crossref] [PubMed]
- Salmanpour MR, Shamsaei M, Hajianfar G, Soltanian-Zadeh H, Rahmim A. Longitudinal clustering analysis and prediction of Parkinson's disease progression using radiomics and hybrid machine learning. Quant Imaging Med Surg 2022;12:906-19. [Crossref] [PubMed]
- Virarkar M, Wong VK, Morani AC, Tamm EP, Bhosale P. Update on quantitative radiomics of pancreatic tumors. Abdom Radiol (NY) 2022;47:3118-60. [Crossref] [PubMed]
- Machicado JD, Koay EJ, Krishna SG. Radiomics for the Diagnosis and Differentiation of Pancreatic Cystic Lesions. Diagnostics (Basel) 2020.
- Dalal V, Carmicheal J, Dhaliwal A, Jain M, Kaur S, Batra SK. Radiomics in stratification of pancreatic cystic lesions: Machine learning in action. Cancer Lett 2020;469:228-37. [Crossref] [PubMed]
- Deng Y, Li Y, Wu JL, Zhou T, Tang MY, Chen Y, Zuo HD, Tang W, Chen TW, Zhang XM. Radiomics models based on multi-sequence MRI for preoperative evaluation of MUC4 status in pancreatic ductal adenocarcinoma: a preliminary study. Quant Imaging Med Surg 2022;12:5129-39. [Crossref] [PubMed]
- Xie T, Wang X, Zhang Z, Zhou Z. CT-Based Radiomics Analysis for Preoperative Diagnosis of Pancreatic Mucinous Cystic Neoplasm and Atypical Serous Cystadenomas. Front Oncol 2021;11:621520. [Crossref] [PubMed]
- Shen X, Yang F, Yang P, Yang M, Xu L, Zhuo J, Wang J, Lu D, Liu Z, Zheng SS, Niu T, Xu X. A Contrast-Enhanced Computed Tomography Based Radiomics Approach for Preoperative Differentiation of Pancreatic Cystic Neoplasm Subtypes: A Feasibility Study. Front Oncol 2020;10:248. [Crossref] [PubMed]
- Wei R, Lin K, Yan W, Guo Y, Wang Y, Li J, Zhu J. Computer-Aided Diagnosis of Pancreas Serous Cystic Neoplasms: A Radiomics Method on Preoperative MDCT Images. Technol Cancer Res Treat 2019;18:1533033818824339. [Crossref] [PubMed]
- Collins GS, Reitsma JB, Altman DG, Moons KG. Transparent Reporting of a multivariable prediction model for Individual Prognosis or Diagnosis (TRIPOD): the TRIPOD statement. Ann Intern Med 2015;162:55-63. Erratum in: Ann Intern Med 2015;162:600.
- Ma J, He J, Yang X. Learning Geodesic Active Contours for Embedding Object Global Information in Segmentation CNNs. IEEE Trans Med Imaging 2021;40:93-104. [Crossref] [PubMed]
- Isensee F, Jaeger PF, Kohl SAA, Petersen J, Maier-Hein KH. nnU-Net: a self-configuring method for deep learning-based biomedical image segmentation. Nat Methods 2021;18:203-11. [Crossref] [PubMed]
- Ma J, Chen J, Ng M, Huang R, Li Y, Li C, Yang X, Martel AL. Loss odyssey in medical image segmentation. Med Image Anal 2021;71:102035. [Crossref] [PubMed]
- van Griethuysen JJM, Fedorov A, Parmar C, Hosny A, Aucoin N, Narayan V, Beets-Tan RGH, Fillion-Robin JC, Pieper S, Aerts HJWL. Computational Radiomics System to Decode the Radiographic Phenotype. Cancer Res 2017;77:e104-7. [Crossref] [PubMed]
- Dmitriev K, Kaufman AE, Javed AA, Hruban RH, Fishman EK, Lennon AM, Saltz JH. Classification of Pancreatic Cysts in Computed Tomography Images Using a Random Forest and Convolutional Neural Network Ensemble. Med Image Comput Comput Assist Interv 2017;10435:150-8.