Validation of a deep learning-based software for automated analysis of T2 mapping in cardiac magnetic resonance imaging
Introduction
Myocardial edema is associated with acute myocardial infarction, myocarditis, stress cardiomyopathy, cardiac sarcoidosis, and cardiac allograft rejection, and detecting it aids in diagnosing these diseases. Cardiac magnetic resonance imaging (MRI) with T2-weighted sequences can detect myocardial edema (1-3). Measuring T2 signal intensity in the myocardium using T2-weighted imaging is the conventional procedure for detecting myocardial edema (2,4). However, the ability of this technique to evaluate diffuse or subtle myocardial changes is limited because it requires a reference tissue such as remote myocardium or skeletal muscle (1,5). T2 mapping of the myocardium has emerged as a technique with better diagnostic accuracy than conventional T2-weighted imaging because it provides tissue-specific T2 values without requiring comparison with reference tissue values (1,3,6-9). T2 mapping techniques have advantages over conventional T2-weighted imaging for detecting myocardial edema and inflammation with superior diagnostic performance (3).
Measuring T2 values in T2 mapping sequence requires manual segmentation of the ventricular myocardium. However, manual segmentation is time-consuming, and its accuracy relies on the observer’s experience (10-12). The ability to automatically perform segmentation should improve the reproducibility and conveniency of measuring T2 values. Recent developments in deep learning (DL) models have made automated T2 map analysis possible, but performance evaluations of automated T2 measurements in the left ventricular (LV) myocardium are rare. One study recently reported that convolution neural network-based automated T2 measurements exhibited good agreement with manual measurements (11). However, these results were obtained with a single 1.5-T MRI scanner at a single center. To date, the reliability and diagnostic performance of automated T2 measurements using multi-institutional datasets obtained with multiple 3.0-T scanners have not been investigated.
The purpose of our study is to evaluate the performance of a commercial DL-based software for automated T2 measurements from 3.0-T cardiac MRI scans of healthy subjects and patients with myocarditis at two centers.
Methods
This study was conducted in accordance with the Declaration of Helsinki (as revised in 2013). The study was approved by the institutional review boards of Severance Hospital and Dongsan Hospital, and the informed consent was waived for this retrospective analysis, except for healthy volunteers from Dongsan Hospital who provided the written informed consent for publication. Additionally, the informed consent from healthy volunteers at Severance Hospital was obtained during a prospective study (13), therefore, the informed consent for this retrospective analysis was also waived.
Subjects
We validated a commercial DL-based, automated cardiac MRI analysis software by Myomics (Phantomics, Inc., Seoul, Korea) by retrospectively including consecutive subjects who met the following eligibility criteria (Figure 1): (I) adults (age ≥19 years) who underwent cardiac MRI between October 2018 and May 2021 at center 1 and between March 2020 and June 2021 at center 2; (II) myocarditis was suggested by cardiac MRI and clinical findings. Diagnoses of myocarditis were based on diagnostic criteria recommended by the European Society of Cardiology Working Group for myocardial and pericardial diseases (6,14). Patients with myocarditis had one or more of the clinical presentations (e.g., acute chest pain) and one or more of the diagnostic criteria (e.g., electrocardiographic changes, elevated troponin level, functional abnormalities on cardiac imaging, or imaging abnormalities on cardiac MRI) or two or more diagnostic criteria. Cardiac MRI abnormalities suggesting myocarditis were based on the Lake Louise criteria (15). We also included 42 healthy volunteers who underwent cardiac MRI at either center (13). Subjects were excluded if the quality of the T2 map images was too poor to allow T2 map analysis for the entire myocardial segment. Subjects were included if image artifacts were present in only a portion of myocardial segments. In total, 42 subjects (29 subjects from center 1 and 13 from center 2) were enrolled in the normal group. The myocarditis group consisted of 31 subjects from center 1 and 10 from center 2, for a total of 41 myocarditis patients.
Sample size calculation
The primary endpoint of our study was the difference between automated T2 values and manual reference T2 values. As there are no standardized criteria for non-inferiority analysis regarding automated T2 measurements, the non-inferiority margin was set by focusing on the software’s performance for discriminating between normal and abnormal by automated measurement. According to the guidelines, the upper and lower ranges of normal values are defined by the mean ±2 standard deviations (SDs) of normal data (3). The SD of T2 relaxation times in healthy subjects ranged from 1.2 to 5.1 ms in a meta-analysis; thus, the non-inferiority margin for our study was chosen conservatively to be 2.4 ms based on the smallest reported SD (16). The sample size was determined to be a minimum of 14 subjects per group (normal and myocarditis) based on a non-inferiority margin of 2.4 ms that would achieve a type I error with alpha =0.025 and power =80%. The non-inferiority margin and sample size calculation were determined only to compare automated T2 values and manual reference standard T2 values, not to calculate the sensitivity and specificity.
Cardiac MRI acquisition
Cardiac MRI examinations were performed at each institution with a 3-T system [Prismafit (Siemens Healthineers, Erlangen, Germany) for center 1 and Vida (Siemens Healthineers) for center 2]. Quantitative T2 mapping imaging was performed prior to contrast media injection with a T2-prepared steady-state free precession (SSFP) pulse sequence along identical short-axis planes. T2 mapping images were acquired at the end-diastolic cardiac phase with the breath-hold technique, a slice thickness of 8 mm, an inter-slice gap of 10 mm, a field of view of approximately 380×380 mm2, and in-plane resolution of 1.9 mm (Table S1). T2 maps were created with systems provided by the MRI manufacturer.
LV function and mass were assessed by acquiring short-axis images of the LV using a cine balanced steady-state free-precession sequence (17). Three short-axis modified look-locker inversion-recovery (MOLLI) images at the base, mid-cavity, and apex were acquired for native T1 mapping (1,17). A total dose of 0.1 mmol/kg gadolinium agent was then injected. Late gadolinium enhancement (LGE) imaging was acquired 10 min after contrast injection. Subsequently, post-contrast MOLLI T1 mapping was obtained for T1 determination from the exact location used for native T1 mapping.
Cardiac MRI analysis
Cardiac MR images were anonymized and analyzed independently by two experienced observers (cardiac radiologists with 9 and 6 years of cardiac MRI experience) who were blinded to the automated T2 analysis results and clinical information, to establish the reference standard.
T2 mapping images were analyzed using commercial software (cvi42 image analysis software, Circle Cardiovascular Imaging Inc., Calgary, AB, Canada). The manual segmentation of the T2 map was evaluated by the slice. Per segment T2 values were reported according to the American Heart Association 16-segment model. Per slice or per patient T2 values were calculated as the mean T2 value of each segment. Cardiac MRI images of other sequences (e.g., T1 maps or LGE images) were evaluated for the presence of imaging abnormalities to assess myocarditis based on the Lake Louise criteria.
For the assessment of interobserver agreement, T2 mapping images were analyzed by a third observer (a board-certified cardiac radiologist). She independently segmented the LV myocardium of using the same software (cvi42) and was blinded to the reference standards.
DL models for myocardium segmentation on T2 maps
Automated analysis of T2 maps was performed using Myomics (Phantomics), which is a DL-based, automated cardiac MRI analysis software (Figures 2,3). The DL model was developed using T2 map images as input. The details of the DL model are described in the supplementary methods (Appendix 1). Briefly, the training and testing datasets for the DL model included 586 cardiac MRI examinations from center 1 that were acquired using a 3.0-T MRI system (Prismafit, Siemens Healthineers).
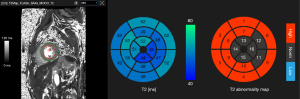
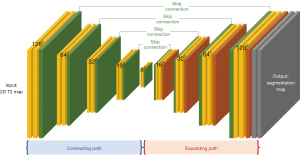
Data analysis
The primary endpoint of our study was to determine whether automated T2 values were non-inferior to manual reference T2 values in a per-segment analyses. The secondary endpoints were to analyze segmentation performance using the Dice similarity coefficient (DSC) and to evaluate the diagnostic performance of the DL-based software for detecting abnormal T2 segments in a per-segment analysis by calculating the sensitivity and specificity.
Segments with poor quality images due to severe artifacts or failure of automated segmentation were excluded from diagnostic performance analysis. An elevated (abnormal) T2 value was defined as a T2 value higher than two SDs above the mean T2 value for each slice in the normal group (11,16).
Statistical analyses
R software (version 4.1.2; R Foundation for Statistical Computing, Vienna, Austria) with R packages “lmerTest”, “rmcorr”, and “DescTools” and SPSS software (version 25.0, IBM Corp., Armonk, NY, USA) were used for statistical analyses. The independent t-test and chi-square test were used to compare participants’ demographics between centers. For primary endpoint, automated and manual reference T2 values were compared per segment using a linear mixed model with the center and type of measurement (automated or manual) as the fixed effect and the patient as the random effect. The interaction between center and the type of measurement was added to the model to test whether the differences in T2 values between the automated and reference were different between two centers. The upper limit of the 95% confidence interval (CI) of the difference was used to judge non-inferiority. Pearson correlation, linear regression, and Bland-Altman analyses were used to analyze the correlation and agreement between reference and automated T2 values in the per-segment analysis. DSC was compared between slice location, (apex, mid, base), sex, and disease group using one-way analysis of variance with the Bonferroni correction or independent t-test. Inter-observer agreement and the agreement between the observer and automated measurement for T2 values were assessed using Bland-Altman analyses. Logistic regression with a generalized estimating equation was used to assess sensitivity, specificity, and accuracy of DL-based software for detection of segments with elevated T2 value per segment, compared to reference T2 values. P values <0.05 were considered statistically significant.
Results
Clinical characteristics
A total of 83 subjects were enrolled from two centers (41 males; mean age, 41.7±15.8 years; 42 in the normal group and 41 in the myocarditis group). The demographics are summarized in Table 1 and Table S2. There was a significant difference in the proportion of males and age between the two centers (P<0.05). The proportion of patients with myocarditis was not significantly different between the two centers (P>0.05). Cardiac MRI results of myocarditis group are provided in Table S3.
Table 1
Characteristics | Total (n=83) | Center 1 (n=60) | Center 2 (n=23) | P value* |
---|---|---|---|---|
Sex, n (%) | 0.006 | |||
Male | 41 (49.4) | 24 (40.0) | 17 (73.9) | |
Female | 42 (50.6) | 36 (60.0) | 6 (26.1) | |
Age (year) | 41.7±15.8 | 44.7±16.3 | 33.8±11.4 | 0.004 |
BMI (kg/m2) | 23.2±3.6 | 22.9±3.7 | 24.0±3.2 | 0.220 |
Group, n (%) | 0.504 | |||
Normal | 42 (50.6) | 29 (48.3) | 13 (56.5) | |
Myocarditis | 41 (49.4) | 31 (51.7) | 10 (43.5) |
Data are presented as the number of subjects (percentage) or mean ± SD. *, for comparison between two centers. BMI, body mass index; SD, standard deviation.
Performance of DL-based automated T2 analysis software
Automated myocardial segmentation failed in 16 segments of one subject in the myocarditis group from center 2 and in one segment of one healthy subject from center 1. The success rate for automated segmentation of the T2 map was 97.6% (81 of 83) per patient and 98.7% (1,311 of 1,328) per segment.
Comparisons of automated and reference T2 values are summarized in Table 2. The linear mixed model showed that patterns of difference in automated and reference T2 values were dissimilar between center 1 and center 2 (P value for interaction =0.031). Automated and reference T2 values per segment were 45.35 and 44.32 ms, respectively, at center 1 and 44.09 and 42.35 ms at center 2. The upper limit of the 95% CI of the difference was smaller than the predefined non-inferiority margin (2.40 ms) at center 1 (1.38 ms) and center 2 (2.18 ms), suggesting that automated T2 measurement is non-inferior to manually measured T2 values. Automated and reference T2 values were positively correlated at center 1 (R=0.831, slope =0.893) and center 2 (R=0.689, slope =0.678) for per-segment analyses (Figure 4). Bland-Altman plots of automated and reference T2 values showed a mean bias ± 95% limits of agreement of 1.03±6.34 and 1.70±6.76 ms at center 1 and center 2, respectively (Figure 4).
Table 2
Center | Group | Automated T2 value* (ms) |
Reference T2 value from manual measurement* (ms) | Difference* | P value |
---|---|---|---|---|---|
Center 1 | Total | 45.35 (44.30, 46.40) | 44.32 (43.27, 45.37) | 1.03 (0.68, 1.38) | <0.001 |
Normal | 43.06 (41.90, 44.22) | 41.92 (40.76, 43.08) | 1.15 (0.67, 1.62) | <0.001 | |
Myocarditis | 47.56 (46.43, 48.70) | 46.64 (45.50, 47.78) | 0.92 (0.46, 1.38) | <0.001 | |
Center 2 | Total | 44.09 (42.68, 45.51) | 42.35 (40.95, 43.77) | 1.74 (1.29, 2.18) | <0.001 |
Normal | 42.57 (40.84, 44.30) | 40.42 (38.69, 42.15) | 2.16 (1.44, 2.87) | <0.001 | |
Myocarditis | 46.02 (44.03, 48.01) | 44.87 (42.91, 46.85) | 1.14 (0.29, 1.99) | 0.009 |
*, estimated mean with two-sided 95% confidence interval.
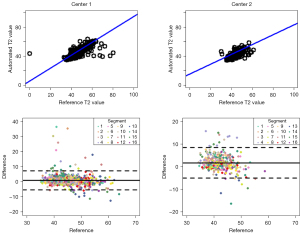
The mean DSC for LV myocardium segmentation on T2 maps was 0.844 (range, 0.355 to 0.971; Figure 5). The DSC was higher at center 1 than center 2 (0.852 vs. 0.823). DSC values were significantly different between slice levels. DSC was lower in apical slices than mid or base slices at both centers (Table 3, P<0.05). No other significant differences for DSC were observed according to sex and disease groups, except that the DSC values for females were slightly lower than those for males at center 1.
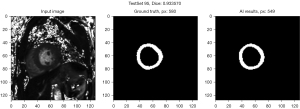
Table 3
Variables | Total (n=246) | Center 1 (n=180) | Center 2 (n=66) | |||||
---|---|---|---|---|---|---|---|---|
Mean ± SD | P value | Mean ± SD | P value | Mean ± SD | P value | |||
Total | 0.844±0.090 | – | 0.852±0.081 | – | 0.823±0.107 | – | ||
Sex | 0.068 | 0.001 | 0.640 | |||||
Male | 0.855±0.083 | 0.874±0.042 | 0.823±0.116 | |||||
Female | 0.834±0.095 | 0.838±0.097 | 0.813±0.083 | |||||
Group | 0.482 | 0.581 | 0.924 | |||||
Normal | 0.840±0.095 | 0.849±0.091 | 0.823±0.101 | |||||
Myocarditis | 0.848±0.085 | 0.855±0.071 | 0.824±0.118 | |||||
Slice | <0.001 | <0.001 | 0.014 | |||||
Apex | 0.805±0.124 | 0.002* | 0.815±0.120 | 0.004* | 0.777±0.134 | 0.465* | ||
Mid | 0.850±0.065 | 0.129† | 0.861±0.045 | 0.523† | 0.821±0.096 | 0.362† | ||
Base | 0.877±0.045 | <0.001‡ | 0.880±0.037 | <0.001‡ | 0.870±0.064 | 0.011‡ |
*, P value for comparison between the apex and mid slices; †, P value for comparison between mid and base slices; ‡, P value for comparison between the base and apex slices. LV, left ventricular; SD, standard deviation.
Interobserver agreement and agreement between the automated measurement and observer for the measurement of T2
Regarding the T2 value, mean bias between the reference standard and the observer was 0.08±2.09 and 0.60±9.54 ms at center 1 and center 2, respectively. Mean bias between the observer and automated measurement 1.12±6.27 and 2.37±11.38 ms at center 1 and center 2, respectively.
Diagnostic performance for detecting elevated T2 value
A normal range of T2 values was defined for each slice level (Table S4) from reference T2 values of the normal group. T2 values for 17 segments from 2 subjects were excluded from diagnostic performance analysis due to segmentation failures, and 25 segments from 4 subjects were excluded due to poor quality images with severe artifacts (Tables S5,S6). The sensitivity, specificity, and accuracy of automated T2 analysis for detecting elevated T2 values per segment, compared to manual reference values, were 92.8% (193 of 208), 92.0% (668 of 726) and 92.2% (861 of 934), respectively, for center 1 and 83.6% (46 of 55), 82.5% (245 of 297) and 82.7% (291 of 352), respectively, for center 2 (Table 4).
Table 4
Center | Performance metric | Estimate (95% confidence interval) | Fraction |
---|---|---|---|
Center 1* | Sensitivity (%) | 92.8 (88.2, 97.4) | 193/208 |
Specificity (%) | 92.0 (89.7, 94.3) | 668/726 | |
Accuracy (%) | 92.2 (90.3, 94.1) | 861/934 | |
Center 2† | Sensitivity (%) | 83.6 (76.6, 90.7) | 46/55 |
Specificity (%) | 82.5 (74.5, 90.5) | 245/297 | |
Accuracy (%) | 82.7 (76.0, 89.4) | 291/352 |
Data indicate the number of segments. *, 25 segments in four subjects were excluded due to poor quality images with severe artifact, and one segment in one subject was excluded due to failure to automatic segmentation; †, 16 segments in one subject were excluded due to failure to automatic segmentation.
Discussion
Our study compared automated T2 values determined by a DL-based software to reference T2 values determined by manual measurement per segment and evaluated the software’s performance in detecting elevated T2 values per segment. Our study shows that automated T2 values are non-inferior to reference standard T2 values at both centers. The segmentation performance of the automated software is high (DSC >0.8) at both centers. The DL-based software shows good performance (sensitivity: 83.6–92.8%; specificity: 82.5–92.0%; accuracy: 82.7–92.2%) in detecting elevated T2 values in the per-segment analysis.
Several studies have investigated DL-based algorithms for automated myocardium segmentation, primarily using T1 maps (18-22). Some studies used T1-weighted or T2*-weighted images as the input of the DL algorithm (18,21), whereas our study used T2 map as the input. However, to our best knowledge, only one study reported automated segmentation and calculation of T2 values using myocardial T2 maps. A recent study reported the performance of a convolution neural network-based automated T2 analysis platform that was trained on T1 mapping data (11). The automated analysis yielded T2 values that had good agreement with manual measurements (R=0.75, slope =0.99 for the per-segment analysis). The study data were generated by a single 1.5-T MRI scanner at a single center and their study population consisted of patients with known or suspected cardiovascular disease referred for clinical cardiac MRI. Our study evaluated and demonstrated the utility of a DL-based model for calculating automated T2 values acquired with 3.0-T MRI in a study population enrolled from two centers. Our study population consisted of normal and myocarditis groups, in whom the clinical utility of T2 map analysis would be important. Furthermore, we preset the sample size and non-inferiority margin to assess the non-inferiority of automated T2 values relative to manual reference T2 values and used a linear mixed model considering clustered data for data analysis.
The DL-based model used in our study was trained on T2 mapping data from center 1 obtained at 3.0 T and yielded automated T2 values that were non-inferior to manual measurements at centers 1 and 2. However, the automated T2 values tended to be higher than the manual reference T2 values at both centers. We assumed that a larger segmentation mask for the myocardium might have led to higher T2 values because the T2 values of regions adjacent to the myocardium (e.g., lumen of the ventricles, pericardial fat, or pericardial effusion) tended to be higher than those of the myocardium. In addition, manual segmentation of the myocardium was performed by excluding regions with artifacts from the segmentation mask; however, these exclusions were not considered in automated segmentation using the DL-based model. The presence of artifacts is another possible reason for the differences between the manual reference and automated T2 values.
Some results from the DL-based model performance were different between the two centers. These differences between centers might be because center 2 had a smaller sample size than center 1 (60 in center 1, 23 in center 2), and the DL-based model was trained on a dataset from center 1. DL algorithm performance can degrade with slight variations in the input data. For example, a study of automated quantifications of myocardial scar burden using LGE sequence and cine sequences reported a lower correlation coefficient between manual and automated quantifications of scar burden and many failed segmentations in an external dataset (20). The results were presumed to be due to differences in patient characteristics, imaging parameters, and other implicit differences in implementing the imaging protocol (20).
In our study, the DSC was high (>0.8) at both institutions, but the DSC in apex slices was significantly lower than that in mid or base slices, which is in accordance with the findings of a previous study (11). Furthermore, cases with poor segmentation performance (DSC <0.650) were observed only for apical slices in our study. Possible reasons include the relatively small area of myocardium in the apical slices, somewhat blurred segmentation margins due to the acute angle of the myocardium to the imaging plane, and poor image quality due to motion artifacts (18).
Our study showed that a commercial, DL-based automated algorithm exhibited a higher sensitivity (83.6–92.8%) and slightly lower specificity (82.5–92.0%) for detecting elevated T2 values in a per-segment analysis than a previous study (sensitivity: 71.4%, specificity: 95.4%) (11). As discussed earlier, higher T2 values in automated analysis, possibly related with inclusion of adjacent structures on automated analysis and exclusion of regions with artifacts from manual segmentations may affect the high sensitivity but relatively low specificity of automated T2 map analysis for detecting elevated T2 values. Therefore, a careful review of the automated segmentation results is needed when using the automated T2 values obtained with the DL-based model in actual clinical practice.
The DL-based model in our study allows automatic segmentation of the LV myocardium and automated measurement of T2 values, which is a quick and convenient way to evaluate for myocardial edema without reducing accuracy. Therefore, the DL-based algorithm can be applied to other diseases that require myocardial T2 measurement.
There are several limitations to this study. First, all images were acquired using 3.0-T MRI scanners from a single manufacturer. Additional studies that apply the DL-based software to images obtained from scanners from different vendors and with different field strengths would facilitate the generalizability of the results. Second, although we performed sample size calculations for non-inferiority testing, the sample sizes at each center and number of participating centers were small. Especially, the number of the normal, healthy subjects were relatively small to secure the local reference range. Because the differences between centers can be a concern for clinical implementation of DL algorithm, evaluating the DL-based model with more subjects at more centers would help establish the utility of the DL-based measurement of T2 values. Third, we investigated the accuracy of the DL-based software for detecting segments with elevated T2, but further studies investigating the utility of DL-based automated measurements in combined T1 and T2 mapping sequences may be helpful to expand the clinical application of the DL algorithm.
Conclusions
Automated T2 map analysis using a commercial DL algorithm yields non-inferior measurements and good performance for detecting myocardial segments identified by elevated T2 values compared with manual analysis.
Acknowledgments
Funding: This work was supported by
Footnote
Conflicts of Interest: All authors have completed the ICMJE uniform disclosure form (available at https://qims.amegroups.com/article/view/10.21037/qims-23-375/coif). Two authors (PKK and BWC) are founders of Phantomics, Inc. (Seoul, Korea) and one author (YJY) is an employee of the same company, but the company did not give financial support for this study. The company supported the software (Myomics) for this study. The other authors have no conflicts of interest to declare.
Ethical Statement: The authors are accountable for all aspects of the work in ensuring that questions related to the accuracy or integrity of any part of the work are appropriately investigated and resolved. The study was conducted in accordance with the Declaration of Helsinki (as revised in 2013). The study was approved by the institutional review boards of Severance Hospital and Dongsan Hospital, and the informed consent was waived for this retrospective analysis, except for healthy volunteers from Dongsan Hospital who provided the written informed consent for publication. Additionally, the informed consent from healthy volunteers at Severance Hospital was obtained during a prospective study published before, therefore, the informed consent for this retrospective study was also waived.
Open Access Statement: This is an Open Access article distributed in accordance with the Creative Commons Attribution-NonCommercial-NoDerivs 4.0 International License (CC BY-NC-ND 4.0), which permits the non-commercial replication and distribution of the article with the strict proviso that no changes or edits are made and the original work is properly cited (including links to both the formal publication through the relevant DOI and the license). See: https://creativecommons.org/licenses/by-nc-nd/4.0/.
References
- Kim PK, Hong YJ. Im DJ, Suh YJ, Park CH, Kim JY, Chang S, Lee HJ, Hur J, Kim YJ, Choi BW. Myocardial T1 and T2 Mapping: Techniques and Clinical Applications. Korean J Radiol 2017;18:113-31. [Crossref] [PubMed]
- Abdel-Aty H, Boyé P, Zagrosek A, Wassmuth R, Kumar A, Messroghli D, Bock P, Dietz R, Friedrich MG, Schulz-Menger J. Diagnostic performance of cardiovascular magnetic resonance in patients with suspected acute myocarditis: comparison of different approaches. J Am Coll Cardiol 2005;45:1815-22. [Crossref] [PubMed]
- Messroghli DR, Moon JC, Ferreira VM, Grosse-Wortmann L, He T, Kellman P, Mascherbauer J, Nezafat R, Salerno M, Schelbert EB, Taylor AJ, Thompson R, Ugander M, van Heeswijk RB, Friedrich MG. Clinical recommendations for cardiovascular magnetic resonance mapping of T1, T2, T2* and extracellular volume: A consensus statement by the Society for Cardiovascular Magnetic Resonance (SCMR) endorsed by the European Association for Cardiovascular Imaging (EACVI). J Cardiovasc Magn Reson 2017;19:75.
- Kindermann I, Barth C, Mahfoud F, Ukena C, Lenski M, Yilmaz A, Klingel K, Kandolf R, Sechtem U, Cooper LT, Böhm M. Update on myocarditis. J Am Coll Cardiol 2012;59:779-92. [Crossref] [PubMed]
- Luetkens JA, Doerner J, Thomas DK, Dabir D, Gieseke J, Sprinkart AM, Fimmers R, Stehning C, Homsi R, Schwab JO, Schild H, Naehle CP. Acute myocarditis: multiparametric cardiac MR imaging. Radiology 2014;273:383-92. [Crossref] [PubMed]
- Luetkens JA, Faron A, Isaak A, Dabir D, Kuetting D, Feisst A, Schmeel FC, Sprinkart AM, Thomas D. Comparison of Original and 2018 Lake Louise Criteria for Diagnosis of Acute Myocarditis: Results of a Validation Cohort. Radiol Cardiothorac Imaging 2019;1:e190010. [Crossref] [PubMed]
- von Knobelsdorff-Brenkenhoff F, Schüler J, Dogangüzel S, Dieringer MA, Rudolph A, Greiser A, Kellman P, Schulz-Menger J. Detection and Monitoring of Acute Myocarditis Applying Quantitative Cardiovascular Magnetic Resonance. Circ Cardiovasc Imaging 2017;10:e005242. [Crossref] [PubMed]
- Luetkens JA, Homsi R, Sprinkart AM, Doerner J, Dabir D, Kuetting DL, Block W, Andrié R, Stehning C, Fimmers R, Gieseke J, Thomas DK, Schild HH, Naehle CP. Incremental value of quantitative CMR including parametric mapping for the diagnosis of acute myocarditis. Eur Heart J Cardiovasc Imaging 2016;17:154-61. [Crossref] [PubMed]
- Giri S, Chung YC, Merchant A, Mihai G, Rajagopalan S, Raman SV, Simonetti OP. T2 quantification for improved detection of myocardial edema. J Cardiovasc Magn Reson 2009;11:56. [Crossref] [PubMed]
- Hann E, Popescu IA, Zhang Q, Gonzales RA, Barutçu A, Neubauer S, Ferreira VM, Piechnik SK. Deep neural network ensemble for on-the-fly quality control-driven segmentation of cardiac MRI T1 mapping. Med Image Anal 2021;71:102029. [Crossref] [PubMed]
- Zhu Y, Fahmy AS, Duan C, Nakamori S, Nezafat R. Automated Myocardial T2 and Extracellular Volume Quantification in Cardiac MRI Using Transfer Learning-based Myocardium Segmentation. Radiol Artif Intell 2020;2:e190034. [Crossref] [PubMed]
- Zhang Q, Hann E, Werys K, Wu C, Popescu I, Lukaschuk E, Barutcu A, Ferreira VM, Piechnik SK. Deep learning with attention supervision for automated motion artefact detection in quality control of cardiac T1-mapping. Artif Intell Med 2020;110:101955. [Crossref] [PubMed]
- Suh YJ, Kim PK, Park J, Park EA, Jung JI, Choi BW. Phantom-based correction for standardization of myocardial native T1 and extracellular volume fraction in healthy subjects at 3-Tesla cardiac magnetic resonance imaging. Eur Radiol 2022;32:8122-30. [Crossref] [PubMed]
- Caforio AL, Pankuweit S, Arbustini E, Basso C, Gimeno-Blanes J, Felix SB, et al. Current state of knowledge on aetiology, diagnosis, management, and therapy of myocarditis: a position statement of the European Society of Cardiology Working Group on Myocardial and Pericardial Diseases. Eur Heart J 2013;34:2636-48, 2648a-2648d.
- Ferreira VM, Schulz-Menger J, Holmvang G, Kramer CM, Carbone I, Sechtem U, Kindermann I, Gutberlet M, Cooper LT, Liu P, Friedrich MG. Cardiovascular Magnetic Resonance in Nonischemic Myocardial Inflammation: Expert Recommendations. J Am Coll Cardiol 2018;72:3158-76. [Crossref] [PubMed]
- Hanson CA, Kamath A, Gottbrecht M, Ibrahim S, Salerno M. T2 Relaxation Times at Cardiac MRI in Healthy Adults: A Systematic Review and Meta-Analysis. Radiology 2020;297:344-51. [Crossref] [PubMed]
- Jo Y, Kim J, Park CH, Lee JW, Hur JH, Yang DH, Lee BY, Im DJ, Hong SJ, Kim EY, Park EA, Kim PK, Yong HS. Guideline for Cardiovascular Magnetic Resonance Imaging from the Korean Society of Cardiovascular Imaging-Part 1: Standardized Protocol. Korean J Radiol 2019;20:1313-33. [Crossref] [PubMed]
- Fahmy AS, El-Rewaidy H, Nezafat M, Nakamori S, Nezafat R. Automated analysis of cardiovascular magnetic resonance myocardial native T(1) mapping images using fully convolutional neural networks. J Cardiovasc Magn Reson 2019;21:7. [Crossref] [PubMed]
- Farrag NA, Lochbihler A, White JA, Ukwatta E. Evaluation of fully automated myocardial segmentation techniques in native and contrast-enhanced T1-mapping cardiovascular magnetic resonance images using fully convolutional neural networks. Med Phys 2021;48:215-26. [Crossref] [PubMed]
- Fahmy AS, Rowin EJ, Chan RH, Manning WJ, Maron MS, Nezafat R. Improved Quantification of Myocardium Scar in Late Gadolinium Enhancement Images: Deep Learning Based Image Fusion Approach. J Magn Reson Imaging 2021;54:303-12. [Crossref] [PubMed]
- Martini N, Meloni A, Positano V, Latta DD, Keilberg P, Pistoia L, Spasiano A, Casini T, Barone A, Massa A, Ripoli A, Cademartiri F. Fully Automated Regional Analysis of Myocardial T2* Values for Iron Quantification Using Deep Learning. Electronics 2022;11:2749.
- Wang Y, Zhang Y, Wen Z, Tian B, Kao E, Liu X, Xuan W, Ordovas K, Saloner D, Liu J. Deep learning based fully automatic segmentation of the left ventricular endocardium and epicardium from cardiac cine MRI. Quant Imaging Med Surg 2021;11:1600-12. [Crossref] [PubMed]