Development of a magnetic resonance imaging-derived radiomics model to predict microvascular invasion in patients with hepatocellular carcinoma
Introduction
Hepatocellular carcinoma (HCC) is the most common malignant tumor of the liver. In past decades, the incidence of HCC has increased (1,2). The incidences of HCC over the 3 decades spanning from 1983 to 2012 extracted from the Surveillance, Epidemiology, and End Results (SEER) database were 1.9, 3.1, and 4.9 per 100,000, respectively (2). The 6-month relative survival rate of HCC increased from 31.0% in the 1980s to 42.9% in the 1990s to 57.2% in the 2000s (2). The increase in the incidence and survival rate is largely due to the rapid development of imaging techniques and treatment strategies.
Microvascular invasion (MVI), also known as microvascular tumor embolism, refers to cancer cell nests in the vascular cavity lined by endothelial cells. Patients with HCC and MVI have a poor prognosis and are more likely to develop HCC recurrence and metastasis (3,4). Accurate prediction of MVI in HCC can facilitate the accurate estimation of patient prognosis, inform the appropriate selection of effective treatment methods (5-7), including anti-recurrence and anti-metastasis therapies (systemic therapy or immunotherapy); guide posttreatment follow-up; and predict the need for additional treatment. Radiomics aims to objectively and quantitatively characterize the structure of tumors and peritumoral tissue (8,9). Doing so can describe tumor heterogeneity and reflect the histopathologic grading and prognosis (10,11). Therefore, radiomics can potentially be effective in the preoperative prediction of MVI (12,13). Some studies suggest that liver radiomics is insufficiently mature to routinely integrate into clinical practice (14,15). In addition, previous radiomic studies have involved multi-sequence and multiphase imaging, resulting in a large amount of data, requiring extensive computational analysis and a challenging and complex operation process. Consequently, the wider application of radiomics has been limited. The purpose of this study was thus to develop and validate a simple, stable, and repeatable radiomics model for MVI in HCC based on T2-weighted imaging (T2WI) radiomic features. We present the following article in accordance with the TRIPOD reporting checklist (available at https://qims.amegroups.com/article/view/10.21037/qims-22-1011/rc).
Methods
Study population
This study was conducted in accordance with the Declaration of Helsinki (as revised in 2013). The study was reviewed and approved by the ethics committee of West China Hospital. Written informed consent was waived owing to the retrospective nature of this study. Data were obtained from patients with HCC who underwent routine preoperative liver magnetic resonance imaging (MRI) examinations within 2 months prior to surgery between January 2012 and January 2021. The inclusion criteria were as follows: (I) a diagnosis of primary HCC according to the Guidelines for Diagnosis and Treatment of Primary Liver Cancer (2017 China Edition) (16); (II) an MRI examination performed within 2 months before the operation; (III) a complete surgical resection and MVI evaluation; and (IV) no previous treatment. The exclusion criteria were as follows: (I) incomplete clinical or pathological information; (II) previous medical history of other malignant tumors; (III) hematologic disease with obvious hepatic iron deposition or other related diseases leading to long-term abnormal liver function, (i.e., the history of repeated blood transfusions leading to liver iron overload and abnormal liver function caused by systemic diseases such as leukemia or lymphoma); and (IV) obvious image artifacts (i.e., poor respiratory coordination of patients leading to obvious image artifacts or uneven magnetic field signals leading to image deformation or signal abnormalities). A total of 104 patients were enrolled in this study.
Pathological criteria for MVI
Surgical treatment was performed within 2 months after the completion of the MRI examination. The histologic presence of cancer cell nests within the lumen of blood vessels lined by endothelial cells was considered diagnostic of MVI. MVI is most commonly detected in the small branching vessels of the portal vein in paracancerous liver tissue and the vessels within the tumor capsules. In this study, the patients were divided into the MVI (MVI-positive) and non-MVI (MVI-negative) groups based on the presence or absence of MVI. Without consulting the patient’s pathological reports and clinical data, 2 pathologists who had specialized in abdominal pathology for more than 5 years reviewed all the pathological sections together.
MRI examination
Liver MRI examinations were performed on a 1.5T MRI scanner (GE Healthcare) using a body phased-array coil. All patients underwent pre-enhanced axial and coronal T2WI fat suppression (FS), axial T1-weighted in/out-of-phase imaging, diffusion-weighted imaging (DWI), and post-enhanced axial and coronal multiphase scanning. After bolus injection of gadoteric acid meglumine salt (0.2 mL/kg body weight) at a 1-mL/s flow rate, hepatic arterial, portal, and delayed phase images were obtained using the liver acquisition with volume acceleration (LAVA) sequence, with suspended respiration at 25–30, 50–60, and 110–120 s, respectively. Table 1 lists the parameters of each scan sequence.
Table 1
Name | Orientation | Category | TR (ms) | TE (ms) | FOV (cm) | Matrix | NEX | Seam thickness (mm) | Fat saturation | Breath hold |
---|---|---|---|---|---|---|---|---|---|---|
T1WI in/out phase | TRA | FSPGR | 7.1 | 4.7/2.1 (in/out phase) | 40×36 | 288×224 | 2 | 5 | Yes/no | No |
T2WI-FS | TRA/COR | FRFSE | 6,500–7,000 | 90 | 42×42 | 288×224 | 2 | 5 | Yes | No |
DWI | TRA | SS SE-EPI | 7,500 | 70 | 42×42 | 288×224 | 2 | 5 | Yes | Yes |
T1WI + C | TRA/COR | LAVA | 7.1 | 3.5 | 40×36 | 256×192 | – | 5 | Yes | Yes |
MRI, magnetic resonance imaging; T1WI in/out phase, T1-weighted images in phase/out phase; T2WI, T2-weighted images; FS, fat suppression; DWI, diffusion-weighted imaging; C, contrasted; TRA, transverse axial; COR, coronal axial; FSPGR, fast-spoiled gradient echo; FRFSE, fast relaxation fast spin echo; SS SE-EPI, single shot spin-echo echo-planar imaging; LAVA, liver acquisition with volume acceleration; TR, repetition time; TE, echo time; FOV, field of view; NEX, number of excitations.
Volume of interest (VOI) determination and segmentation
The 3-dimensional (3D) volume area of the lesion was identified and manually delineated using the VOI on T2WI after the consensus of 2 radiologists with more than 3 years of experience in MRI diagnosis of abdominal diseases (Figure 1). This process was completed without consulting the patient’s radiological and pathological reports. T2W images of all patients were preprocessed before delineating the VOI to make the images of each patient more homogeneous. The VOI avoided areas with necrosis, cystic degeneration, and hemorrhage. The lesions showed high signal intensity on T2WI and DWI and low signal intensity on T1WI compared with the normal background liver parenchyma. Enhanced lesions showed enhancement on dynamic contrast-enhanced (DCE)-MRI with various enhancement patterns, including fast wash-in and wash-out, fast wash-in and slow wash-out, or continuous enhancement. The VOI was manually delineated for each layer in all tumor regions.
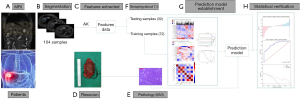
Radiomics feature extraction
Feature extraction was performed using AK software (Artificial Intelligence Kit Version; V. 3.2.0R, GE Healthcare). All radiomic features, including first order, shape, gray-level co-occurrence matrix (GLCM), gray-level size zone matrix (GLSZM), gray-level run length matrix, neighboring gray-tone difference matrix, and gray-level dependence matrix were extracted. Wavelet transform was selected, and a bin width of 25 mm with a distance of 1 mm was set. A total of 851 feature parameters were extracted (for an operational example, see Figure 2).
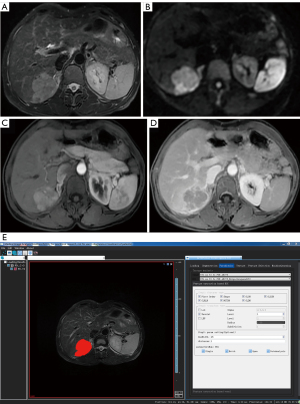
Model configurations
Patients were randomly assigned to the training and verification cohorts using a ratio of about 7:3. We established a prediction model to predict the MVI results using statistical feature de-redundancy. First, a univariate logistic regression analysis was used for feature selection, and features with P<0.05 were retained. Then, the least absolute shrinkage and selection operator (LASSO) regression model was applied, and the minimum λ was selected using 5-fold cross-validation. Heatmap analysis was used to show the correlation between the radiomics features and MVI results. Each patient’s radiomic score was displayed using different colors. Finally, we established a predictive model with several selected features using logistic regression.
Statistical analysis
The Mann-Whitney test was used for continuous variables with an abnormal distribution. Fisher exact test or the chi-squared test was used for nominal variables. All statistical analyses in this study were performed using R version 3.5.1 (The R Foundation for Statistical Computing; https://www.freesoftwarefiles.com/math/r-for-windows-3-5-1-free-download/) and Python version 3.5.6 (Python Software Foundation; https://www.python.org/downloads/release/python-356/). Statistical significance was defined as a 2-tailed P value <0.05.
Continuous variables are presented as the median with interquartile ranges. Categorical variables are presented as numbers and percentages. Hepatitis B DNA was classified as follows: 0, normal; 1, low level [≤105 copies (cps)/mL]; and 2, middle-high level (>105 cps/mL).
The area under the curve (AUC) was obtained from the receiver operating characteristic (ROC) curve analysis. The specificity, sensitivity, and positive and negative predictive values were calculated and verified in the test cohort. The consistency between the model’s prediction and actual results confirmed with pathological diagnosis was evaluated using calibration curve analysis. Decision curve analysis was used to assess the clinical applicability of the model.
Results
Study population
A total of 104 patients were enrolled, including 89 males and 15 females, with a median age of 55 years (range, 25–77 years). Among the 104 patients with HCC included in the study, 40 had histologic evidence of MVI (the MVI-negative group, 49.03%), and 55 did not (the MVI-positive group, 50.97%). There were 34 patients with MVI and 38 without in the training cohort. In the test cohort, 15 patients had MVI and 17 did not. The clinical characteristics are listed in Table 2. Age, carbohydrate antigen199, and carcinoembryonic antigen levels were not significantly different between the MVI-positive and MVI-negative groups. MVI was more likely to be detected in males than in females in our samples. Serum alpha-fetoprotein levels in patients who were MVI-positive were higher than those in patients who were MVI-negative. Lower serum hepatitis B DNA levels were found in patients who were MVI-negative. The incidence of MVI was significantly higher in the cohort with DNA that was positive for hepatitis B, especially in the middle-high level hepatitis B DNA group. The absence of MVI was more likely to be observed in the cohort with DNA that was negative for hepatitis B.
Table 2
Variable | Sample | MVI-negative (n=51, 49.03%) | MVI-positive (n=53, 50.97%) | Statistics | P value |
---|---|---|---|---|---|
Age (years), mean ± SD | 104 | 54.20±11.23 | 53.49±10.42 | 0.332 | 0.74 |
Gender | 9.931 | 0.002 | |||
Male | 89 | 38 (74.51%) | 51 (96.23%) | ||
Female | 15 | 13 (25.49%) | 2 (3.77%) | ||
Hepatitis B DNA (cps/mL) | –2.094 | 0.036 | |||
0 | 50 | 31 (60.78%) | 19 (35.85%) | ||
1 | 15 | 5 (9.80%) | 10 (18.87%) | ||
2 | 39 | 15 (29.41%) | 24 (45.28%) | ||
AFP (ng/mL) | 104 | 18.60 (4.27, 273.92) | 139.40 (17.06, 1,899.10) | –2.725 | 0.006 |
CA199 (U/mL) | 104 | 12.16 (10.62, 30.81) | 12.16 (8.98, 32.01) | 0.016 | 0.987 |
CEA (ng/mL) | 104 | 2.43 (2.08, 3.58) | 2.43 (1.69, 4.30) | –0.065 | 0.948 |
Continuous variables are expressed as the median and interquartile range. Categorical variables are expressed as numbers and percentages. SD, standard deviation; AFP, alpha-fetoprotein; CA199, carbohydrate antigen199; CEA, carcinoembryonic antigen; MVI, microvascular invasion. Hepatitis B DNA classification: 0, normal; 1, low level [≤105 copies (cps)/mL]; 2, middle-high level (>105 cps/mL).
Radiomics model establishment
The heatmaps of the model for selecting features in the training samples are shown in Figure 3. After univariate logistic regression, 24 features (P<0.05) were retained. The remaining 8 features were selected to establish a predictive model using LASSO regression (Figure 4). The 8 features were as follows: original_shape_elongation, wavelet-HLH_firstorder_Mean, wavelet-LLH_glcm_ClusterShade, wavelet-LLL_glcm_Imc2, wavelet-LHL_glszm_GrayLevelNonUniformity, wavelet-HLH_glcm_MCC, wavelet-LLL_glcm_Imc1, and wavelet-HLH_glcm_Imc1 [Feature naming rules: image type (original image/transformed image)_feature type (shape/texture)_feature name. For the explanation of various radiological features, please refer to the website: https://pyradiomics.readthedocs.io/en/latest/features.html#]. The box map of the distribution of each feature contained in the MVI-negative and MVI-positive population is shown in Figure 5.
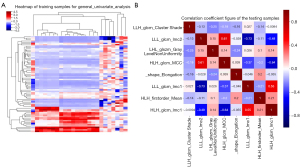
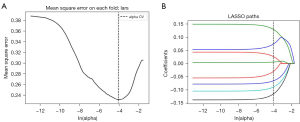
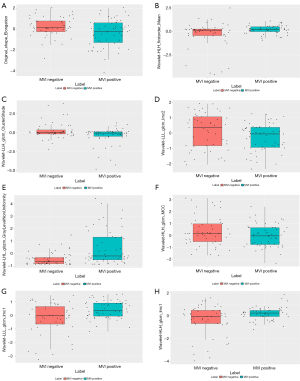
Test of the radiomics model
The AUC, accuracy, specificity, sensitivity, and positive and negative predictive values of the model for predicting MVI in training and test cohorts are presented in Table 3; in the training cohort, these values were 0.867, 72.7%, 84.2%, 64.7%, 72.7%, and 78.6%, respectively, while in the test cohort, they were 0.820, 75%, 70.6%, 73.3%, 75%, and 68.8%, respectively. The AUC in our model (Figure 6) was more than 0.8, which was close to or higher than that in many complex radiomics studies of MVI (17,18)
Table 3
Group | AUC (95% CI) | Accuracy (%) | Sensitivity (%) | Specificity (%) | Positive predictive value (%) | Negative predictive value (%) |
---|---|---|---|---|---|---|
Training cohort | 0.867 (0.789–0.946) | 72.7 | 84.2 | 64.7 | 72.7 | 78.6 |
Test cohort | 0.820 (0.670–0.969) | 75 | 70.6 | 73.3 | 75 | 68.8 |
AUC, area under the curve; CI, confidence interval.
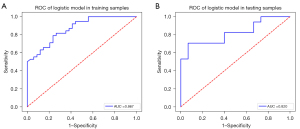
The calibration curves displayed good consistency in both the training and test cohorts for MVI prediction using the model (Figure 7). The radiomics scores of the logistic model in the training and test cohorts are shown in the bar chart in Figure 8. These findings indicated that the MVI-positive group scored higher than did the MVI-negative group.
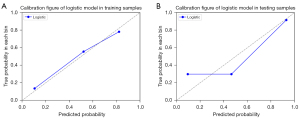
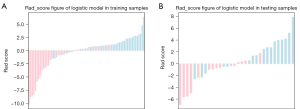
After extracting the features of the lesion image using AK software, we selected 8 key features as mentioned above, and running this model, we established a predictive model which could predict the presence of MVI in the lesions. The decision curve showed that if the threshold probability of a patient in the training cohort was 4%, the prediction of MVI using the radiomics nomogram in our study added more advantages than did the treatment of all patient regimens or non-treatment regimens. The threshold probability of patients in the verification cohort was more than 30% (Figure 9).
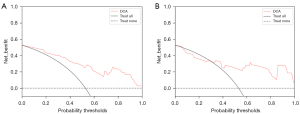
Discussion
In this study, we established a radiomics model to predict preoperative MVI using radiomic features from T2WI. In contrast to the existing complex multi-sequence and multifactor model studies, the single-sequence radiomics model in our study obtained good performance in MVI prediction. The AUC was more than 0.80, and the correction curve showed that the MVI predicted using the model was in good agreement with the pathological results in the training and test cohorts. The main reason that the AUC in our model was higher than 80%, which was close to or higher than that in many complex radiomics studies on MVI (17,18), might be due to the model stability and data repeatability. This finding may be attributable to two main factors. First, it was difficult to guarantee consistency in each patient when checked, and the VOI was manually delineated for each layer in the tumor region. Manual extraction of imaging features has the disadvantage of large deviation, low sensitivity, and poor generalization ability. Deep learning–based techniques for automatic segmentation and radiomic analysis, such as rigorous workflow, manual/semiautomatic lesion annotation, inadequate feature criteria, and multicenter validation, are being analyzed to address limitations, (19). Massive and high-quality medical image data is the basis of deep learning technology that can achieve accurate image diagnosis. However, due to the limitations of imaging equipment and acquisition time, these techniques will inevitably be affected by noise, artifacts, and other factors in the process of medical imaging (19,20). The more sequences that are included, the more unstable the data (14,15,18), which leads to poorer model repeatability. Simple radiomic studies may yield better and more stable results. Second, the accurate discrimination of lesion boundaries is more conducive to radiomics research. T2WI sequence images show very high resolution, and the signal difference between the surrounding normal liver parenchyma and tumor tissue in T2WI was more obvious than in other plain scan sequences. Other sequences, such as DCE-MRI and DWI, show poorer resolution. The objectivity, accuracy, and repeatability of DCE-MRI radiomics are also questionable due to individual differences in circulation. The stability of the diffusion-weighted sequences is relatively poor, with a lack of repeatability. The VOI in our study included all tumor slices on T2WI, which made the features more representative and stable than those on 2-dimensional VOI (17). Furthermore, radiomics studies have the advantages of high repeatability, stable calculation, indefatigability, and freedom from subjective interference (21-23). Our study was based on single-sequence radiomics, and the model is expected to be more stable and repeatable.
In our study, 8 quantitative radiological features associated with MVI in HCC were extracted. Among these 8 features, 6 were matrix-based features (wavelet-LLH_glcm_ClusterShade, wavelet-LLL_glcm_Imc2, wavelet-LHL_glszm_GrayLevelNonUniformity, wavelet-HLH_glcm_MCC, wavelet-LLL_glcm_Imc1, and wavelet-HLH_glcm_Imc1), and 2 were histogram-based features (original_shape_Elongation and wavelet-HLH_firstorder_Mean). The first-order feature describes the characteristics of the voxel intensity distribution in the region of interest (ROI) (24). It is interesting to note that the feature original_shape_Elongation may be associated with the MVI in HCC. The larger the elongation of the tumor, the more apparent the MVI is, which may be associated with the growth pattern of the tumor (25). Matrix-based features or texture features are second-order statistics used to describe tumor heterogeneity. Highly malignant tumors may exhibit greater heterogeneity and obvious intercellular differences, manifesting as diverse gray scales and mixed signals within the tumor.
High-grade HCC tends to be associated with MVI, which is more prone to necrosis, resulting in a more uneven signal. Therefore, we speculate that the radiological features of MVI-negative HCC are more uniform than those of MVI-positive HCC. Biological functional similarity may affect the similarities of micropathological and subsequent radiological features, which was suggested in our study as a valuable predictor of MVI.
This study did not include tumor margin tissue and intratumoral necrosis, cystic degeneration, or hemorrhage in the radiomics analysis because inclusion of non-tumor parenchyma information would interfere with the model’s effect on tumor parenchyma.
The prediction model in this study was well validated in the test group. It is reasonable to believe that the single-sequence T2WI radiological features of the tumor area are sufficient for predicting MVI before surgery.
Limitations
This was a preliminary study, and there were some limitations. First, we adopted a retrospective design with potential bias, and we excluded some specific cases from the study through the exclusion criteria. Second, the sample size of this study was limited, and our test and training cohorts came from the same center, which may restrict the generalizability of our research results to other centers. Therefore, multicenter research with a larger sample size is needed. Third, the correlation analysis between VOI on MRI and the actual location of MVI in histological specimens was not carried out. Furthermore, there were limitations in pathological sampling and diagnosis, and it is possible that cases that were MVI-positive were not identified. Fourth, it was difficult to avoid differences in the image signal uniformity caused by patient factors. Finally, manual segmentation was used to extract features in our study, which might have affected the accuracy of some features. 3D manual segmentation of tumors is time-consuming, so it is necessary to develop a reliable and repeatable automatic segmentation method.
Conclusions
The radiomic model developed in this study showed high sensitivity, specificity, and accuracy in predicting MVI in both the training and test cohorts. Our results demonstrate that using a single-sequence T2WI-based radiomic model, as an alternative to a complex multi-sequence radiomic model, can also predict MVI without increasing the scanning time or complicating the machine configuration. Moreover, the nomogram developed in this study may be helpful for predicting the individualization of MVI before surgery and assisting clinicians in making preoperative decisions. It is worth exploring its scientific value in tumor lesion grading and prognosis.
Acknowledgments
We would like to thank Editage (www.editage.cn) for English language editing.
Funding: The study was supported by the Foshan 14th Five-Year Plan Key Discipline Foundation (No. FSGSP145036) and the 2021 Medical Science and Technology Research Fund of Guangdong Province (No. B2021393).
Footnote
Reporting Checklist: The authors have completed the TRIPOD reporting checklist. Available at https://qims.amegroups.com/article/view/10.21037/qims-22-1011/rc
Conflicts of Interest: All authors have completed the ICMJE uniform disclosure form (available at https://qims.amegroups.com/article/view/10.21037/qims-22-1011/coif). A GE Healthcare machine was used to complete the patient examination, and the AK software from the same company was used to complete the data extraction. The radiomics model was built by YL, who reports being full-time employee of GE Healthcare during the completion of the study. The other authors have no conflicts of interest to declare.
Ethical Statement: The authors are accountable for all aspects of the work in ensuring that questions related to the accuracy or integrity of any part of the work are appropriately investigated and resolved. The study was conducted in accordance with the Declaration of Helsinki (as revised in 2013) and was approved by the institutional ethics committee of West China Hospital. Individual consent for this retrospective analysis was waived.
Open Access Statement: This is an Open Access article distributed in accordance with the Creative Commons Attribution-NonCommercial-NoDerivs 4.0 International License (CC BY-NC-ND 4.0), which permits the non-commercial replication and distribution of the article with the strict proviso that no changes or edits are made and the original work is properly cited (including links to both the formal publication through the relevant DOI and the license). See: https://creativecommons.org/licenses/by-nc-nd/4.0/.
References
- Flores YN, Datta GD, Yang L, Corona E, Devineni D, Glenn BA, Bastani R, May FP. Disparities in Hepatocellular Carcinoma Incidence, Stage, and Survival: A Large Population-Based Study. Cancer Epidemiol Biomarkers Prev 2021;30:1193-9. [Crossref] [PubMed]
- Wang S, Sun H, Xie Z, Li J, Hong G, Li D, Mallampati S, Zhou X, Zhou C, Zhang H, Cheng Z, Shan H, Ma H. Improved survival of patients with hepatocellular carcinoma and disparities by age, race, and socioeconomic status by decade, 1983-2012. Oncotarget 2016;7:59820-33. [Crossref] [PubMed]
- Guarino M, Cucchetti A, Pontillo G, Farinati F, Benevento F, Rapaccini GL, et al. Pattern of macrovascular invasion in hepatocellular carcinoma. Eur J Clin Invest 2021;51:e13542. [Crossref] [PubMed]
- Costentin CE, Ferrone CR, Arellano RS, Ganguli S, Hong TS, Zhu AX. Hepatocellular Carcinoma with Macrovascular Invasion: Defining the Optimal Treatment Strategy. Liver Cancer 2017;6:360-74. [Crossref] [PubMed]
- Peng Z, Chen S, Xiao H, Wang Y, Li J, Mei J, Chen Z, Zhou Q, Feng S, Chen M, Qian G, Peng S, Kuang M. Microvascular Invasion as a Predictor of Response to Treatment with Sorafenib and Transarterial Chemoembolization for Recurrent Intermediate-Stage Hepatocellular Carcinoma. Radiology 2019;292:237-47. [Crossref] [PubMed]
- Yang J, Liang H, Hu K, Xiong Z, Cao M, Zhong Z, Yao Z, Deng M. The effects of several postoperative adjuvant therapies for hepatocellular carcinoma patients with microvascular invasion after curative resection: a systematic review and meta-analysis. Cancer Cell Int 2021;21:92. [Crossref] [PubMed]
- Lafaro K, Grandhi MS, Herman JM, Pawlik TM. The importance of surgical margins in primary malignancies of the liver. J Surg Oncol 2016;113:296-303. [Crossref] [PubMed]
- Avanzo M, Wei L, Stancanello J, Vallières M, Rao A, Morin O, Mattonen SA, El Naqa I. Machine and deep learning methods for radiomics. Med Phys 2020;47:e185-202. [Crossref] [PubMed]
- Sung YS, Park B, Park HJ, Lee SS. Radiomics and deep learning in liver diseases. J Gastroenterol Hepatol 2021;36:561-8. [Crossref] [PubMed]
- Sanduleanu S, Woodruff HC, de Jong EEC, van Timmeren JE, Jochems A, Dubois L, Lambin P. Tracking tumor biology with radiomics: A systematic review utilizing a radiomics quality score. Radiother Oncol 2018;127:349-60. [Crossref] [PubMed]
- Chaudhary K, Poirion OB, Lu L, Garmire LX. Deep Learning-Based Multi-Omics Integration Robustly Predicts Survival in Liver Cancer. Clin Cancer Res 2018;24:1248-59. [Crossref] [PubMed]
- Li L, Wu C, Huang Y, Chen J, Ye D, Su Z. Radiomics for the Preoperative Evaluation of Microvascular Invasion in Hepatocellular Carcinoma: A Meta-Analysis. Front Oncol 2022;12:831996. [Crossref] [PubMed]
- Zhang X, Ruan S, Xiao W, Shao J, Tian W, Liu W, Zhang Z, Wan D, Huang J, Huang Q, Yang Y, Yang H, Ding Y, Liang W, Bai X, Liang T. Contrast-enhanced CT radiomics for preoperative evaluation of microvascular invasion in hepatocellular carcinoma: A two-center study. Clin Transl Med 2020;10:e111. [Crossref] [PubMed]
- Wakabayashi T, Ouhmich F, Gonzalez-Cabrera C, Felli E, Saviano A, Agnus V, Savadjiev P, Baumert TF, Pessaux P, Marescaux J, Gallix B. Radiomics in hepatocellular carcinoma: a quantitative review. Hepatol Int 2019;13:546-59. [Crossref] [PubMed]
- Xue C, Yuan J, Lo GG, Chang ATY, Poon DMC, Wong OL, Zhou Y, Chu WCW. Radiomics feature reliability assessed by intraclass correlation coefficient: a systematic review. Quant Imaging Med Surg 2021;11:4431-60. [Crossref] [PubMed]
- Bureau of Medical Administration, National Health Commission of the People's Republic of China. Standardization for diagnosis and treatment of hepatocellular carcinoma (2022 edition). Zhonghua Gan Zang Bing Za Zhi 2022;30:367-88. [PubMed]
- Zhang R, Xu L, Wen X, Zhang J, Yang P, Zhang L, Xue X, Wang X, Huang Q, Guo C, Shi Y, Niu T, Chen F. A nomogram based on bi-regional radiomics features from multimodal magnetic resonance imaging for preoperative prediction of microvascular invasion in hepatocellular carcinoma. Quant Imaging Med Surg 2019;9:1503-15. [Crossref] [PubMed]
- Dai H, Lu M, Huang B, Tang M, Pang T, Liao B, Cai H, Huang M, Zhou Y, Chen X, Ding H, Feng ST. Considerable effects of imaging sequences, feature extraction, feature selection, and classifiers on radiomics-based prediction of microvascular invasion in hepatocellular carcinoma using magnetic resonance imaging. Quant Imaging Med Surg 2021;11:1836-53. [Crossref] [PubMed]
- Zhang X, Zhang Y, Zhang G, Qiu X, Tan W, Yin X, Liao L. Deep Learning With Radiomics for Disease Diagnosis and Treatment: Challenges and Potential. Front Oncol 2022;12:773840. [Crossref] [PubMed]
- Cao C, Liu F, Tan H, Song D, Shu W, Li W, Zhou Y, Bo X, Xie Z. Deep Learning and Its Applications in Biomedicine. Genomics Proteomics Bioinformatics 2018;16:17-32. [Crossref] [PubMed]
- Lundsgaard Hansen M, Fallentin E, Axelsen T, Lauridsen C, Norling R, Svendsen LB, Nielsen MB. Interobserver and Intraobserver Reproducibility with Volume Dynamic Contrast Enhanced Computed Tomography (DCE-CT) in Gastroesophageal Junction Cancer. Diagnostics (Basel) 2016.
- Gillies RJ, Kinahan PE, Hricak H. Radiomics: Images Are More than Pictures, They Are Data. Radiology 2016;278:563-77. [Crossref] [PubMed]
- Aerts HJ, Velazquez ER, Leijenaar RT, Parmar C, Grossmann P, Carvalho S, Bussink J, Monshouwer R, Haibe-Kains B, Rietveld D, Hoebers F, Rietbergen MM, Leemans CR, Dekker A, Quackenbush J, Gillies RJ, Lambin P. Decoding tumour phenotype by noninvasive imaging using a quantitative radiomics approach. Nat Commun 2014;5:4006. [Crossref] [PubMed]
- Feng ST, Jia Y, Liao B, Huang B, Zhou Q, Li X, Wei K, Chen L, Li B, Wang W, Chen S, He X, Wang H, Peng S, Chen ZB, Tang M, Chen Z, Hou Y, Peng Z, Kuang M. Preoperative prediction of microvascular invasion in hepatocellular cancer: a radiomics model using Gd-EOB-DTPA-enhanced MRI. Eur Radiol 2019;29:4648-59. [Crossref] [PubMed]
- Renzulli M, Brocchi S, Cucchetti A, Mazzotti F, Mosconi C, Sportoletti C, Brandi G, Pinna AD, Golfieri R. Can Current Preoperative Imaging Be Used to Detect Microvascular Invasion of Hepatocellular Carcinoma? Radiology 2016;279:432-42. [Crossref] [PubMed]