Predicting isocitrate dehydrogenase genotype, histological phenotype, and Ki-67 expression level in diffuse gliomas with an advanced contrast analysis of magnetic resonance imaging sequences
Introduction
Diffuse glioma, the most common primary brain neoplasia, has a relatively poor prognosis. The 2016 World Health Organization (WHO) classification of tumors of the central nervous system (CNS) (1) advanced the classification of CNS tumors, and the entity was classified according to the integrated phenotypic and genotypic parameters. The most remarkable refinement of the upgraded classification was to regard isocitrate dehydrogenase (IDH) as an important genetic factor for diagnosing diffuse glioma, which highlighted an important role of IDH mutation in tumor metabolism, genesis, and proliferation (2-5). Histological phenotype is also a basic factor for defining glioma. Lower-grade glioma (LGG; WHO II and WHO III) has a slower progression than glioblastoma (GBM) and responds differently to therapeutic strategies. The Ki-67 labeling index (LI), a widely known index that reflects the proliferation of tumors, is of noticeable value. Many studies have reported the relationship between glioma grade and Ki-67 LI. In general, tumors with a higher WHO grade have a higher Ki-67 LI (6). Diffuse gliomas with a higher Ki-67 LI suggest a lower overall survival (7) and a worse response to clinical treatment, such as the relief of seizures after surgery, even if the neoplasms are of the same grade (8). However, predicting these gene characteristics before surgery is difficult based only on conventional image characteristics.
The radiogenomics-based classification model is an alternative that noninvasively and preoperatively distinguishes the histological and molecular factors of gliomas, and researchers have made progress on this model (9,10). Compared to computed tomography (CT) and positron emission tomography (PET), magnetic resonance imaging (MRI) achieves high tissue contrast without radiation. Conventional head MRI, which is widely used in clinical practice, has become a fundamental method for these studies. A previous study has demonstrated that among conventional MRI sequences, a T1C-based classifier achieves the highest predictive performance to identify the histological phenotype, but the best sequence combinations have not yet been recommended (11). For the classification of the IDH status in LGG, a previous study has demonstrated that T2-weighted images (T2WI) may be more important (12). Therefore, the actual contribution of different MRI sequences needs further exploration to build more precise models. In addition, the classification of IDH and WHO grade has been separately investigated in most studies. Due to unbalanced sampling because GBM with IDH mutation is rare in clinical practice, it is difficult to build an appropriate model to classify the IDH and WHO grade at the same time.
The present study aimed to build a radiogenomics-based classification model with machine learning (ML) to predict the IDH mutation status, histological phenotype, and Ki-67 expression level for diffuse glioma. In addition, we further explored the disparate contribution of different MRI sequences to the classification. Finally, we aimed to develop a full four-subtype classification system in terms of the WHO classification of CNS tumors to compensate for the unbalanced distribution of sampling that widely occurs at the histological and IDH levels. The present study was both a development and internal validation study. We present the following article in accordance with the TRIPOD reporting checklist (available at https://qims.amegroups.com/article/view/10.21037/qims-22-887/rc).
Methods
Patients
The present study was a retrospective cross-sectional study. Consecutive patients with suspected glioma in the First Medical Center of Chinese PLA General Hospital (a tertiary care center) from November 2015 to October 2019 were recruited, and those with histologically confirmed diffuse gliomas were included. The present study was conducted in accordance with the Declaration of Helsinki (as revised in 2013). The study was approved by the Ethics Committee of the First Medical Center of Chinese PLA General Hospital. Individual consent for this retrospective analysis was waived.
The inclusion criteria were as follows: (I) adult patients aged more than 18 years; (II) available conventional head MR images 2 weeks before surgical resection; (III) no biopsy, radiotherapy, chemotherapy, or any other clinical intervention before MRI scan; and (IV) patients with one of the required statuses, namely, histologically confirmed WHO grade results, immunohistochemically analyzed IDH1 status, and Ki-67 LI results. The exclusion criteria were as follows: (I) patients with marked artifacts on MR images; and (II) any of T1-weighted images (T1WI), T2WI, diffusion-weighted images (DWIs; two sequences with b value equal to 0 and 1,000), or postcontrast T1WI (T1C) missing. The detailed patient selection workflow is shown in Figure 1.
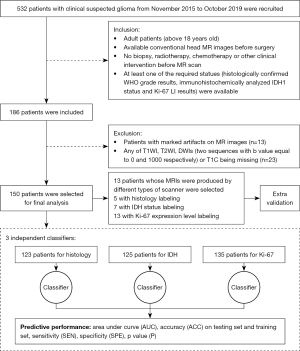
A total of 150 cases, including the validation set, were evaluated in the present study. A total of 123, 125, and 135 patients had histological results, IDH status results, and Ki-67 expression results, respectively. Thirteen patients whose MRIs were produced by different types of scanners were included in the validation group (5 patients with histological results, 7 patients with IDH status results, and 13 patients with Ki-67 expression results).
Histology and immunohistochemistry
The standard protocols of histological analysis and EnVision Plus immunohistochemical staining were conducted to examine IDH1 mutation, histologic phenotype, and Ki-67 LI. Gliomas with histologically confirmed WHO II and WHO III were defined as LGG. The following antibodies were used: R132H-mutant IDH and UMAB107 (Zhongshanjinqiao, Beijing, China). Because different cutoff values have been utilized in previous studies (13,14), we set two different thresholds (10% and 25%) to classify Ki-67 LI in the present study. Ki-67 protein expression in glioma cells lower than the threshold (10% or 25%) was defined as low-Ki-67 expression; otherwise, it was defined as high-Ki-67 expression.
MRI acquisition
The MRI data were mainly produced using three types of scanners as follows: 1.5 T Signa HDxt, Discovery MR750w, and Discovery MR750 (GE Healthcare, Milwaukee, WI, USA). The remaining cases were acquired with several other types of scanners that were also used in our hospital as follows: 3.0 T Signa HDxt and 1.5 T Signa Excite (GE Healthcare); and uMR770 and uMR560 (United Imaging, Shanghai, China). T1WI, T2WI, T1C, and DWI images were acquired using routine diagnostic procedures using 3.0 T and 1.5 T MRI scanners with eight-channel head coils. Detailed information on scanner parameters is provided in the Supplementary Materials (Appendix 1). T1C was acquired from T1WI in axial planes. The apparent diffusion coefficient (ADC) maps were calculated from DWI using an in-house method described in Supplementary Materials (Appendix 2). The DWIs whose b value were equal to 1,000 were used to extract features and build classifiers.
Data postprocessing
In the present study, manual segmentation of the tumor was used. Radiologist No. 1, with neuroradiological experience of more than 6 years, delineated the tumors without knowing the histological and immunohistochemical results using 3D-slicer on every slice of T2W images. Peritumor edema was carefully avoided. A total of 40 patients were randomly selected to repeat the delineation process. Radiologist No. 1 repeated the process with an interval of more than 1 month. Radiologist No. 2, with neuroradiology experience of more than 4 years, repeated the same delineation process and was blinded to the segmentation results of radiologist No. 1. The radiomics features extracted from these 40 patients on the region of interest (ROI) were assessed using the intraclass correlation coefficient (ICC). Examples of MRI images and manual segmentation of ROIs are shown in Figure 2.
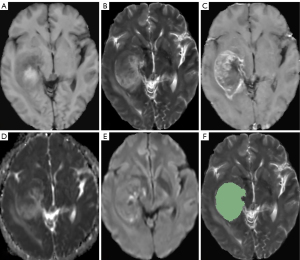
A postprocessing pipeline was built to minimize the heterogeneity caused by scanners and other protocols, thus improving the general predictive performance of the classification model. The original images were processed using Statistical Parametric Mapping Software (SPM12) slice by slice to perform a full MRI scan first and then after slice-timing and motion correction. The other four sequences of MRI were then coregistered to the T2WI based on FLIRT, which is an available tool within the FMRIB Software Library (FSL). The image resolution was then adjusted by resampling all voxel sizes to 1×1×1 mm3.
A mask was generated based on the brain extraction tool (BET) in FSL to cover the brain area, and all the following postprocessing operations were performed under this mask. The bias field generated from the inhomogeneity of the magnetic field, which may be harmful to the radiogenomics-based classification, was corrected by the advanced normalization tool (ANT) with N4 bias field correction. Noise reduction and intensity normalization were then conducted on the marked area.
Feature extraction
Several derived images were calculated from the original image with a particular computational operation. Including the original images, 14 types of images were produced as follows: wavelet-filtered images (with the high- and low-spatial-frequency filters applied on each dimension, resulting in a total of 8 derived images in various combinations); Gaussian-filtered images (with a Laplacian of Gaussian filter applied on the original image, allowing an image with an enhanced edge to be produced); square images; square-root images; exponential images; and logarithmic images. The 18 first-order and 75 texture features, including 24 gray-level cooccurrence matrix features, 16 gray-level run-length matrix features, 16 gray-level size zone matrix features, 14 gray-level dependence matrix features, and 5 neighboring gray-tone difference matrix features, were then extracted from the original image and derived images. Additionally, 14 shape features were independently calculated from the original image. Finally, 1,316 MRI radiomics features (93 texture and first-order features in each of 14 types of images plus 14 shape features) were extracted from each sequence of the MRI scan. All the radiomic features were normalized before they were input into the selection pipeline. The calculations of MRI radiomics employed in the present study were performed using the Pyradiomics Python package (https://pyradiomics.readthedocs.io/). The magnetic resonance (MR) radiomics platform also supported all the calculations as a standalone tool.
Feature selection
In the present study, a two-stage feature selection pipeline was built, including univariate feature selection and dimension reduction. First, for the univariate feature selection, we analyzed the potential contribution of each feature to the classification model. A Mann-Whitney U test was performed, and features with a P value <0.05, representing a significant difference in groups by status, were selected. The least absolute shrinkage and selection operation was used to screen out the most predictive features. Second, redundant features were eliminated in the dimension reduction stage to further avoid overfitting. A collinearity test was performed. Pearson correlation coefficients between features were calculated, and highly correlated features were eliminated according to the matrix. Recursive feature elimination with cross-validation (RFECV) was performed, and the feature combination was determined. The classification algorithm in the present study was an estimator to adjust RFECV. An extra reliability analysis was conducted when the final feature combination was determined to confirm that all the features were valid with the ICC of both intrarater and interrater reproductivity analyses greater than 0.7. An overview of the customized pipeline is shown in Figure 3. All the source codes of this pipeline were uploaded for reproducibility purposes (https://github.com/LUKEDUDE/GliomasExperimentMRI).
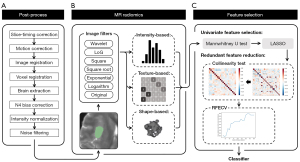
Classification algorithm
Three independent classifiers were built to predict three different characteristics, namely, histological phenotype, IDH mutation status, and Ki-67 expression level. Different MR sequence combinations were used to develop the best classifier for each characteristic. Feature selection was conducted separately for the classifiers with different MR sequence combinations. Stochastic gradient descent was used as an approach to fitting the linear classifier, and it was implemented under different convex loss functions, such as support vector machine (SVM) and logistic regression. In the present study, the best loss function was selected from the following nine different functions: hinge (known as soft-margin SVM), log, modified Huber, squared hinge, perceptron, squared loss, Huber, epsilon insensitive, and squared epsilon insensitive. Regularization was also conducted on the loss function to penalize complexity and avoid overfitting. The regularizer was built using the squared Euclidean norm L2 or the absolute norm L1 or a combination of both (Elastic Net) (15). All ML algorithms were implemented based on the Sklearn Python package (https://scikit-learn.org/stable/). The Statistics and Machine Learning Toolbox in the MATLAB environment (MathWorks, Inc. Natick, MA, USA), as a standalone tool, also supported the aforementioned functions, including feature selection.
Random grouping
The involved participants were grouped into a training-testing set, 80% of which was used for training and 20% for testing. All feature selection and training processes were performed independently every time according to the training set. We simulated a shifting distribution and repeatedly performed it to obtain an overview of the predictive performance of the pipeline. This random grouping test was repeated 30 times to minimize the randomness caused by the ML-based procedure and the heterogeneity of datasets.
Statistical analysis
The chi-squared test was performed to assess the difference in field strength in each population. The following indices were calculated to evaluate the classification model: area under the curve (AUC), accuracy (ACC; ACC values on both the training and testing sets were calculated), sensitivity (SE), specificity (SP), precision, F1 score, and P value. The mean and 95% confidence interval (CI) of each index within 30 random grouping tests were calculated. The P value was calculated from the permutation test (16), which was used to obtain an overview of the efficiency of the current model. With the shuffled status label, the training process was repeated with 1,000 epochs. The P value was then calculated, representing the ranking percentage of the testing ACC of the current model within 1,000 epochs. Bonferroni correction was used for adjustment. A P value <0.05 indicated a significant difference. All statistical analyses were performed based on Python and Statistical Product Service Solutions.
Advanced contrast analysis
First, an advanced contrast analysis was conducted to compare the performance of single-sequence-based classifiers. In the contrast analysis, the nonparametric test and pairwise comparison were employed to compare the testing ACCs of the random grouping tests of each single-sequence-based classifier.
Ultimate classifiers
According to the results of the contrast analysis, different sequence combinations were tested before the final combination with the best performance selected. The classifier under the final combination with the best performance was used as the ultimate classifier for each task. The Hosmer-Lemeshow (H-L) goodness of fit test was conducted for these three classifiers. A small fraction of participants whose MRI images were acquired from different types of scanners was used as a validation dataset.
Four-subtype classification
We integrated two independent classifiers for histological phenotype and IDH status to compensate for the unbalanced sampling distribution at the histological and IDH levels as well as to generate a complete four-subtype classification (LGG with IDH-mut, LGG with IDH-wild, GBM with IDH-mut, and GBM with IDH-wild).
Comparisons of LI thresholds
Additional classifiers were trained for Ki-67 expression with an LI threshold of 25%, and random grouping tests were conducted 30 times. The results were then compared with those of the classifiers with a threshold of 0.1. The classifiers were under the same-sequence combination. The Mann-Whitney U test was used to compare the testing ACC of Ki-67 classifiers under different thresholds.
Results
Characteristics of the study population
Table 1 lists the fundamental information of the included participants with certain status and produced by main scanners. No significant difference was found in field strength between the two groups in each population (histological phenotype, P=0.080; IDH status, P=0.825; Ki-67 expression, P=0.089). For the four-subtype classification, 118 participants with both histologic and IDH mutational labels were selected simultaneously. The classification contained 43 (36.44%) LGGs with IDH mutation, 22 (18.64%) LGGs with IDH wild-type, 6 (5.08%) GBMs with IDH mutation, and 47 (39.84%) GBMs with IDH wild-type. Furthermore, 20% of participants of each type were randomly selected to form the testing set.
Table 1
Characteristics | Histological phenotype (n=123) | IDH status (n=125) | Ki-67 expression (n=135) | |||||
---|---|---|---|---|---|---|---|---|
LGG, n=68 (55%) | GBM, n=55 (45%) | IDH mutant, n=51 (41%) | IDH wild-type, n=74 (59%) | Low expression, n=47 (35%) | High expression, n=88 (65%) | |||
Age (mean ± SD) (years) | 44.647±12.174 | 52.345±13.197 | 41.471±11.366 | 52.162±12.864 | 43.468±11.319 | 50.182±13.834 | ||
Gender (n) | ||||||||
Male | 42 | 27 | 32 | 39 | 26 | 49 | ||
Female | 26 | 28 | 19 | 35 | 21 | 39 | ||
Type of scanner (n) | ||||||||
A | 15 | 20 | 14 | 19 | 9 | 29 | ||
B | 31 | 23 | 21 | 35 | 22 | 39 | ||
C | 22 | 12 | 16 | 20 | 16 | 20 |
The participants were involved in the main training-testing procedure produced by the following scanners: A (1.5 T Signa HDxt), B (Discovery MR 750), and C (Discovery MR 750w). The remaining participants were examined with several other types of scanners, which represented a small fraction of the overall population. These participants formed an extra, independent validation set. LGG, lower-grade glioma; GBM, glioblastoma; IDH, isocitrate dehydrogenase; SD, standard deviation.
Advanced contrast analysis
In the present study, hinge was used as a loss function to build linear classifiers for histology and IDH status, which achieved a testing ACC of 0.84±0.05 for the histological phenotype and 0.75±0.06 for the IDH status using all five MRI modalities, indicating a more promising loss function compared to other loss functions. A squared hinge was also selected for Ki-67, and it achieved a testing ACC of 0.69±0.09. The elastic net was conducted on the loss function across all the tasks, and classifiers based on a single MRI sequence were built. The results of the contrast analysis are shown in Figure 4. The detailed results are shown in Supplementary Materials (Appendix 3).
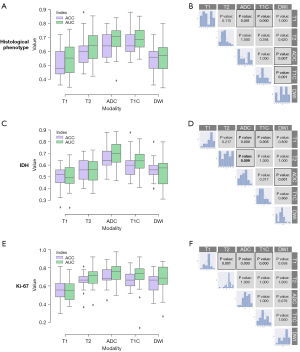
For the histological phenotype (Figure 4A,4B), the ADC- and T1C-based classifiers had better predictive performance and showed significant differences compared to the T1WI- and DWI-based classifiers (ADC vs. T1WI: 0.640 vs. 0.521, P=0.001; ADC vs. DWI: 0.640 vs. 0.543, P=0.007; T1C vs. T1WI: 0.655 vs. 0.521, P<0.001; T1C vs. DWI: 0.655 vs. 0.543, P=0.001); the T2WI-based classifiers showed a secondary performance (ACC =0.604).
For the IDH status (Figure 4C,4D), the ADC-based classifiers showed a dominant predictive performance, which was significantly different from that of the T1WI-, T2WI-, and DWI-based classifiers (ADC vs. T1WI: 0.656 vs. 0.492, P<0.001; ADC vs. T2WI: 0.656 vs. 0.561, P=0.009; ADC vs. DWI: 0.656 vs. 0.549, P=0.001). The T1C-based classifiers also showed a difference compared to the T1WI-based classifiers (T1C vs. T1WI: 0.601 vs. 0.492, P=0.005), but they were not as competitive as the ADC-based classifiers.
For the Ki-67 LI (Figure 4E,4F), the T2WI-, ADC-, and T1C-based classifiers shared a similar predictive performance and showed significant differences compared to the T1WI-based classifiers (T2WI vs. T1WI: 0.657 vs. 0.556, P=0.001; ADC vs. T1WI: 0.699 vs. 0.556, P<0.001; T1C vs. T1WI: 0.670 vs. 0.556, P<0.001); the DWI-based classifiers showed a moderate performance (ACC =0.627).
The predictive performance of classifiers under several sequence combinations is demonstrated in Figure 5, and the detailed results are provided in Supplementary Materials (Appendix 3). Based on the results of the contrast analysis, we formulated three different sequence combinations for each task, with sequences gradually involving the main contributing sequences to the multisequence combinations (all five sequences). The ultimate classification model for each task was produced using the best combination that had the highest overall AUC and ACC as follows: a combination of all five sequences was employed for histological phenotype; a combination of ADC and T1C was employed for the IDH status; and a combination of T2WI, ADC, T1C, and DWI was employed for Ki-67 LI. The predictive performance of the final sequence combination is shown in Table 2.
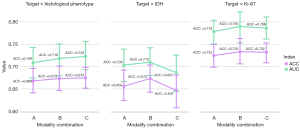
Table 2
Variables | Histological phenotype, mean (95% CI) | IDH status, mean (95% CI) | Ki-67 expression, mean (95% CI) |
---|---|---|---|
AUC | 0.7228 (0.6912–0.7543) | 0.7098 (0.6782–0.7413) | 0.7899 (0.7559–0.8239) |
ACC1 | 0.9755 (0.9622–0.9887) | 0.8966 (0.8729–0.9204) | 0.9888 (0.9831–0.9945) |
ACC2 | 0.6746 (0.6505–0.6988) | 0.6733 (0.6432–0.7033) | 0.7333 (0.7040–0.7626) |
SE | 0.6168 (0.5651–0.6685) | 0.7176 (0.6760–0.7592) | 0.8259 (0.7954–0.8586) |
SP | 0.7246 (0.6787–0.7705) | 0.6065 (0.5428–0.6701) | 0.5662 (0.5092–0.6232) |
P value | 0.0700 (0.0524–0.0877) | 0.1239 (0.0822–0.1657) | 0.0549 (0.0367–0.0732) |
CI, confidence interval; IDH, isocitrate dehydrogenase; AUC, area under the curve on the testing set; ACC1 represents accuracy on the training set, whereas ACC2 represents accuracy on the testing set; SE, sensitivity on the testing set; SP, specificity on the testing set; P, calculated from the permutation test.
Performance of the ultimate classifiers
The performance of the ultimate classifiers is shown in Table 3, and the receiver operating characteristic (ROC) curve is shown in Figure 6 for all three tasks (P values <0.05; within 1,000 epochs of testing, the testing ACC of the current classifier reached the top 50; P values >0.05 in H-L goodness of fit tests). In the independent validation of extra cases, the classifier for the histological phenotype correctly predicted 3 of 5 (60%) participants [3.0 T Signa HDxt (n=2) and uMR770 (n=3)], and the classifier for the IDH status correctly predicted 6 of 7 participants (85.7%) [3.0 T Signa HDxt (n=2), uMR770 (n=4), and 1.5 T Signa Excite (n=1)]. In addition, the Ki-67 classifier correctly predicted 9 of 13 subjects (69%) [3.0 T Signa HDxt (n=5), uMR770 (n=4), 1.5 T Signa Excite (n=2), uMR560 (n=1), and Skyra (n=1)]. Finally, 32, 21, and 13 selected MR features were used to build the ultimate classifiers for histological phenotype, IDH mutation, and Ki-67 LI. Detailed information on these radiomics features is listed in the Supplementary Materials (Appendix 4).
Table 3
Variables | AUC | ACC1 | ACC2 | SE | SP | P value | EV |
---|---|---|---|---|---|---|---|
GBMs/LGGs | 0.933 | 0.969 | 0.800 | 0.800 | 0.800 | 0.024 | 3/5 |
IDH mutation/IDH wild-type | 0.881 | 0.950 | 0.840 | 0.812 | 0.888 | 0.015 | 6/7 |
Ki-67 high expression/low expression | 0.931 | 0.953 | 0.888 | 1.000 | 0.727 | 0.008 | 9/13 |
AUC, area under the curve on the testing set; ACC1 represents accuracy on the training set, whereas ACC2 represents accuracy on the testing set; SE, sensitivity on the testing set; SP, specificity on the testing set; P, calculated from the permutation test; EV, extra validation, the EV results are listed below (correct predictions/all predictions); GBMs, glioblastomas; LGGs, lower-grade gliomas; IDH, isocitrate dehydrogenase.
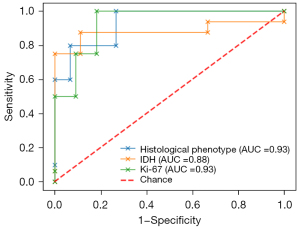
Four-subtype classification
The four-subtype classification based on two independent classifiers for the histological phenotype and IDH status achieved a testing ACC of 95.6%. Only one participant in the testing set was wrongly classified as follows: a GBM wild-type was wrongly regarded as a GBM mutation.
Different thresholds of Ki-67 LI
A significant difference was found between the predictive performance of Ki-67 classifiers (using ADC, T2WI, T1C, and DWI as the final sequence combinations) when 10% and 25% were considered as the thresholds (ACC: 0.733±0.08 vs. 0.597±0.08, P<0.001). The detailed results are provided in the Supplementary Materials (Appendix 3).
Discussion
In the present study, we developed three independent classifiers to differentiate glioblastomas from LGGs, IDH mutant from IDH wild-type, and high expression of Ki-67 from low expression of Ki-67. All three tasks achieved promising performance. In addition, a relatively precise prediction was made in the additional independent validation set, indicating that a satisfying performance was achieved using the present algorithm on heterogeneous data.
According to the advanced contrast analysis, the radiomics extracted from ADC maps had a major contribution to the construction of the classification model. The ADC-based classifiers significantly outperformed most of the other classifiers for all three tasks. ADC maps quantitatively reflect the density of tumor cells (16), which is critical for estimating the proliferation and growth of glioma. In the present study, it was presumed that ADC-related features support the prediction of IDH mutation and Ki-67 expression, both of which have a strong association with the proliferation and growth of gliomas (17-19). Many previous studies have suggested the contribution of ADC maps to the prediction of tumor grade (20), IDH status (21), and Ki-67 expression (22) for gliomas. Second, the T1C-related features also significantly contributed to the classification. T1C images have been recognized as the most reliable conventional head MRI sequences to visually estimate malignancy and proliferation in practice (23,24). These features can reflect a disruption of the blood-brain barrier and angiogenesis of neovascularity in the tumor region (25). Glioblastomas and IDH wild-type, which are commonly related to a more aggressive growth pattern, have an intense and irregular enhancement pattern; otherwise, other tumors have a mild enhancement pattern (26,27). Other studies have revealed an association between T1C imaging features and the development of glioma, including angiogenesis, cell proliferation, and cell migration (28). Although it was used in many previous studies (11,29,30), the present study suggested that T1WI did not largely contribute to developing a radiogenomics-based classifier.
Several other studies have investigated the contribution of radiomics derived from different MRI sequences to ML-based classification (31-33). These analyses were commonly performed using the ultimate multisequence-combined classifiers and lacked a real influence on clinical practice. Frequency analysis (31,34) or SE analysis (35), which focus on individual features, was used as a supplement. In the study, we conducted an advanced contrast analysis to compare the predictive performance of single-sequence-based classifiers before combining all the acquired sequences and attempted to identify the most contributing sequence. Several sequence combinations were tested. Considering that the decline occurred when all five MRI sequences were utilized for IDH and Ki-67 classification, the present findings suggested that it is not ideal to combine all the acquired MRI sequences to develop a radiogenomics-based classifier.
Many studies have focused on molecular prediction in glioma. For histological phenotype, radiologists tend to use postcontrast MRI to identify the tumor grade in clinical practice. A previous study has used the contrast-to-noise ratio of postcontrast images to differentiate high- and lower-grade glioma, resulting in an AUC, sensitivity, and SP of 0.88, 82.6%, and 91.7%, respectively (36). The present method achieved better performance. Logistic regression analysis and the deep learning method have been used, showing remarkable performance using radiomics features from conventional head MRIs (37,38). It has been demonstrated that when a large sample is available, a model based on T2WI achieves a high ACC (98.25%) (38). In addition, a previous study has found that the T1C-based classifier achieves the highest predictive performance in the histological phenotype among conventional MRI sequences, but this study did not recommend any optimal sequence combinations (11). IDH, as the most important molecular characteristic for glioma, has also been investigated, and several models have been built based on different sequences. A present study has demonstrated that models based on features from multiple conventional sequences achieve a good performance compared to those based on radiological characteristics with an ACC of 0.813, and this value can be increased when combined with age (39). Another study has shown that T1WI has the highest grading efficacy among single sequences (AUC =0.92), and combining all conventional MRIs with fraction anisotropy mapping from diffusion tensor imaging achieves a higher performance (AUC =0.97) (40). When study participants have LGGs, T2WI may be more important, resulting in an ACC of 84.9% (12). When arterial spin labeling (ASL) is combined with other sequences, the performance is higher (31,41). Combining advanced MRIs may improve the prediction performance, but they are not commonly used sequences in clinical practice. Hence, an optimal strategy to utilize conventional sequences is necessary. For predicting Ki-67 expression, a previous study has used features from T2WI to build a model in WHO II and WHO III grade gliomas, resulting in AUC and ACC values of 0.916 and 0.886, respectively (42). This previous study demonstrated that radiomics features can make a difference in the prediction of Ki-67 expression, but these researchers only used one sequence, and the contribution of different MRI sequences was not clear. Another study has used multiple conventional MRI sequences and ASL for Ki-67 expression prediction, demonstrating that the T2-fluid-attenuated inversion recovery (FLAIR)-based classifier has the highest predictive performance followed by ADC-based and T1WI-based classifiers. This previous study also demonstrated that the model based on multiple sequences has a higher predictive performance (AUC =0.881) than the models based on a single sequence (13), but this previous study did not investigate a combination with different sequences. In the present study, the optimal model had a higher AUC when three specific sequences were used after sequence selection instead of the model with all sequences.
Some previous studies focusing on the classification under the 2016 WHO classification of CNS tumors were confined to unbalanced sampling because GBM with IDH mutation is rare in clinical practice (1,43). For example, the study by Lu et al. (44) comprised only 8 participants with GBM IDH mutation, but they included 98 participants with GBM IDH wild-type in their training set for the classifier on the IDH status in GBMs, resulting in a deficient sample to differentiate the IDH mutation after classifying LGG and GBM even though the sample size was relatively large. The present method may solve this dilemma. In the present study, a four-subtype classification was proposed after integrating two independent classifiers for histological phenotype and IDH status. Instead of predicting IDH mutation using two separate classifiers under LGG and GBM, only one independent classifier was built in the present study. Moreover, the present classification only needed two binary classifiers instead of three to fulfill a four-subtype classification task, implying that few training samples were needed.
For identifying the Ki-67 expression level, the classifiers with a threshold of 10% significantly outperformed those with a threshold of 25%. Ki-67 LI is commonly used to estimate the proliferation of tumors, and a higher LI suggests poor progression. A previous study has suggested that 25% is close to the mean LI for a group of GBMs, and 10% of the Ki-67 LI is closely related to high-grade gliomas (WHO III and IV) (45). Another study has revealed that 10% may be a significant borderline to suggest a longer progression-free survival in LGGs (WHO II) (46). Considering this strong association between Ki-67 LI and WHO grade, we reasonably presumed that more significant differentiation between the participants occurred when the threshold was 10%.
The present study had several limitations. First, it was comprised of only 150 participants, and no independent validation dataset from different sources was employed. Restricted by the five MRI sequences required at the beginning, we were unable to collect a larger population or make use of any other publicly available dataset. Second, the IDH status was confirmed by the immunohistochemical method instead of sequence analysis, and only the IDH1 mutation was confirmed. IDH1 and IDH2 mutations, which are commonly tested in clinical practice, are the most common IDH mutations, and the IDH1 mutation accounts for 71% of IDH mutations in diffuse gliomas (2).
Conclusions
In conclusion, the present algorithm effectively and noninvasively predicts histological phenotype and IDH mutational status. In the present study, a precise four-subtype classification was generated. The present algorithm showed promising performance in predicting the Ki-67 expression level, with a significant differentiation based on a threshold of 10%. Contrast analysis suggested a dominant contribution made by ADC maps and T1C to the development of an effective classifier for all three tasks, but no substantial support was provided by T1WI and DWI. The present findings suggested that it is not always optimal to build an ML-based classifier by employing all acquired MRI sequences. Because the present study involved multiple scanners, the image quality was different among different scanners to some extent, which may have affected the assessment of the contribution of the MR sequences, especially DWI. However, some sequences may be sound options to develop robust classifiers when multiple scanners are involved.
Acknowledgments
Funding: None.
Footnote
Reporting Checklist: The authors have completed the TRIPOD reporting checklist. Available at https://qims.amegroups.com/article/view/10.21037/qims-22-887/rc
Conflicts of Interest: All authors have completed the ICMJE uniform disclosure form (available at https://qims.amegroups.com/article/view/10.21037/qims-22-887/coif). The authors have no conflicts of interest to declare.
Ethical Statement: The authors are accountable for all aspects of the work in ensuring that questions related to the accuracy or integrity of any part of the work are appropriately investigated and resolved. The study was conducted in accordance with the Declaration of Helsinki (as revised in 2013). The study was approved by the Ethics Committee of the First Medical Center of Chinese PLA General Hospital, and individual consent for this retrospective analysis was waived.
Open Access Statement: This is an Open Access article distributed in accordance with the Creative Commons Attribution-NonCommercial-NoDerivs 4.0 International License (CC BY-NC-ND 4.0), which permits the non-commercial replication and distribution of the article with the strict proviso that no changes or edits are made and the original work is properly cited (including links to both the formal publication through the relevant DOI and the license). See: https://creativecommons.org/licenses/by-nc-nd/4.0/.
References
- Louis DN, Perry A, Reifenberger G, von Deimling A, Figarella-Branger D, Cavenee WK, Ohgaki H, Wiestler OD, Kleihues P, Ellison DW. The 2016 World Health Organization Classification of Tumors of the Central Nervous System: a summary. Acta Neuropathol 2016;131:803-20. [Crossref] [PubMed]
- Hartmann C, Meyer J, Balss J, Capper D, Mueller W, Christians A, Felsberg J, Wolter M, Mawrin C, Wick W, Weller M, Herold-Mende C, Unterberg A, Jeuken JW, Wesseling P, Reifenberger G, von Deimling A. Type and frequency of IDH1 and IDH2 mutations are related to astrocytic and oligodendroglial differentiation and age: a study of 1,010 diffuse gliomas. Acta Neuropathol 2009;118:469-74. [Crossref] [PubMed]
- Yan H, Parsons DW, Jin G, McLendon R, Rasheed BA, Yuan W, Kos I, Batinic-Haberle I, Jones S, Riggins GJ, Friedman H, Friedman A, Reardon D, Herndon J, Kinzler KW, Velculescu VE, Vogelstein B, Bigner DD. IDH1 and IDH2 mutations in gliomas. N Engl J Med 2009;360:765-73. [Crossref] [PubMed]
- Houillier C, Wang X, Kaloshi G, Mokhtari K, Guillevin R, Laffaire J, Paris S, Boisselier B, Idbaih A, Laigle-Donadey F, Hoang-Xuan K, Sanson M, Delattre JY. IDH1 or IDH2 mutations predict longer survival and response to temozolomide in low-grade gliomas. Neurology 2010;75:1560-6. [Crossref] [PubMed]
- Kizilbash SH, Giannini C, Voss JS, Decker PA, Jenkins RB, Hardie J, Laack NN, Parney IF, Uhm JH, Buckner JC. The impact of concurrent temozolomide with adjuvant radiation and IDH mutation status among patients with anaplastic astrocytoma. J Neurooncol 2014;120:85-93. [Crossref] [PubMed]
- Yuan X, Liu D, Wang Y, Li X. Significance of nuclear magnetic resonance combined with Ki-67 and VEGF detection in the diagnosis and prognosis evaluation of brain glioma. J BUON 2018;23:410-5. [PubMed]
- Pouget C, Hergalant S, Lardenois E, Lacomme S, Houlgatte R, Carpentier C, Dehais C, Rech F, Taillandier L, Sanson M, Appay R, Colin C, Figarella-Branger D, Battaglia-Hsu SF, Gauchotte G. Ki-67 and MCM6 labeling indices are correlated with overall survival in anaplastic oligodendroglioma, IDH1-mutant and 1p/19q-codeleted: a multicenter study from the French POLA network. Brain Pathol 2020;30:465-78. [Crossref] [PubMed]
- Yuan Y, Xiang W, Yanhui L, Ruofei L, Shuang L, Yingjun F, Qiao Z, Yanwu Y, Qing M. Ki-67 overexpression in WHO grade II gliomas is associated with poor postoperative seizure control. Seizure 2013;22:877-81. [Crossref] [PubMed]
- Liu Z, Zhang T, Jiang H, Xu W, Zhang J. Conventional MR-based Preoperative Nomograms for Prediction of IDH/1p19q Subtype in Low-Grade Glioma. Acad Radiol 2019;26:1062-70. [Crossref] [PubMed]
- Xu J, Ren Y, Zhao X, Wang X, Yu X, Yao Z, Zhou Y, Feng X, Zhou XJ, Wang H. Incorporating multiple magnetic resonance diffusion models to differentiate low- and high-grade adult gliomas: a machine learning approach. Quant Imaging Med Surg 2022;12:5171-83. [Crossref] [PubMed]
- Wang Q, Li Q, Mi R, Ye H, Zhang H, Chen B, Li Y, Huang G, Xia J. Radiomics Nomogram Building From Multiparametric MRI to Predict Grade in Patients With Glioma: A Cohort Study. J Magn Reson Imaging 2019;49:825-33. [Crossref] [PubMed]
- Zhang X, Tian Q, Wang L, Liu Y, Li B, Liang Z, Gao P, Zheng K, Zhao B, Lu H. Radiomics Strategy for Molecular Subtype Stratification of Lower-Grade Glioma: Detecting IDH and TP53 Mutations Based on Multimodal MRI. J Magn Reson Imaging 2018;48:916-26. [Crossref] [PubMed]
- Su C, Jiang J, Zhang S, Shi J, Xu K, Shen N, Zhang J, Li L, Zhao L, Zhang J, Qin Y, Liu Y, Zhu W. Radiomics based on multicontrast MRI can precisely differentiate among glioma subtypes and predict tumour-proliferative behaviour. Eur Radiol 2019;29:1986-96. [Crossref] [PubMed]
- Guo J, Ren J, Shen J, Cheng R, He Y. Do the combination of multiparametric MRI-based radiomics and selected blood inflammatory markers predict the grade and proliferation in glioma patients? Diagn Interv Radiol 2021;27:440-9. [Crossref] [PubMed]
- Friedman J, Hastie T, Tibshirani R. Regularization Paths for Generalized Linear Models via Coordinate Descent. J Stat Softw 2010;33:1-22. [Crossref] [PubMed]
- Humphries PD, Sebire NJ, Siegel MJ, Olsen ØE. Tumors in pediatric patients at diffusion-weighted MR imaging: apparent diffusion coefficient and tumor cellularity. Radiology 2007;245:848-54. [Crossref] [PubMed]
- Beesley MF, McLaren KM. Cytokeratin 19 and galectin-3 immunohistochemistry in the differential diagnosis of solitary thyroid nodules. Histopathology 2002;41:236-43. [Crossref] [PubMed]
- Jin Q, Zhang W, Qiu XG, Yan W, You G, Liu YW, Jiang T, Wang L. Gene expression profiling reveals Ki-67 associated proliferation signature in human glioblastoma. Chin Med J (Engl) 2011;124:2584-8. [PubMed]
- Ichimura K. Molecular pathogenesis of IDH mutations in gliomas. Brain Tumor Pathol 2012;29:131-9. [Crossref] [PubMed]
- Lee EJ, Lee SK, Agid R, Bae JM, Keller A, Terbrugge K. Preoperative grading of presumptive low-grade astrocytomas on MR imaging: diagnostic value of minimum apparent diffusion coefficient. AJNR Am J Neuroradiol 2008;29:1872-7. [Crossref] [PubMed]
- Lee S, Choi SH, Ryoo I, Yoon TJ, Kim TM, Lee SH, Park CK, Kim JH, Sohn CH, Park SH, Kim IH. Evaluation of the microenvironmental heterogeneity in high-grade gliomas with IDH1/2 gene mutation using histogram analysis of diffusion-weighted imaging and dynamic-susceptibility contrast perfusion imaging. J Neurooncol 2015;121:141-50. [Crossref] [PubMed]
- Calvar JA, Meli FJ, Romero C, Calcagno ML, Yánez P, Martinez AR, Lambre H, Taratuto AL, Sevlever G. Characterization of brain tumors by MRS, DWI and Ki-67 labeling index. J Neurooncol 2005;72:273-80. [Crossref] [PubMed]
- Pope WB, Sayre J, Perlina A, Villablanca JP, Mischel PS, Cloughesy TF. MR imaging correlates of survival in patients with high-grade gliomas. AJNR Am J Neuroradiol 2005;26:2466-74. [PubMed]
- Chaichana KL, Kosztowski T, Niranjan A, Olivi A, Weingart JD, Laterra J, Brem H, Quiñones-Hinojosa A. Prognostic significance of contrast-enhancing anaplastic astrocytomas in adults. J Neurosurg 2010;113:286-92. [Crossref] [PubMed]
- Watanabe M, Tanaka R, Takeda N. Magnetic resonance imaging and histopathology of cerebral gliomas. Neuroradiology 1992;34:463-9. [Crossref] [PubMed]
- Park YW, Han K, Ahn SS, Bae S, Choi YS, Chang JH, Kim SH, Kang SG, Lee SK. Prediction of IDH1-Mutation and 1p/19q-Codeletion Status Using Preoperative MR Imaging Phenotypes in Lower Grade Gliomas. AJNR Am J Neuroradiol 2018;39:37-42. [Crossref] [PubMed]
- Tervonen O, Forbes G, Scheithauer BW, Dietz MJ. Diffuse "fibrillary" astrocytomas: correlation of MRI features with histopathologic parameters and tumor grade. Neuroradiology 1992;34:173-8. [Crossref] [PubMed]
- Liu X, Li Y, Sun Z, Li S, Wang K, Fan X, Liu Y, Wang L, Wang Y, Jiang T. Molecular profiles of tumor contrast enhancement: A radiogenomic analysis in anaplastic gliomas. Cancer Med 2018;7:4273-83. [Crossref] [PubMed]
- Maynard J, Okuchi S, Wastling S, Busaidi AA, Almossawi O, Mbatha W, Brandner S, Jaunmuktane Z, Koc AM, Mancini L, Jäger R, Thust S. World Health Organization Grade II/III Glioma Molecular Status: Prediction by MRI Morphologic Features and Apparent Diffusion Coefficient. Radiology 2020;296:111-21. [Crossref] [PubMed]
- Wu S, Meng J, Yu Q, Li P, Fu S. Radiomics-based machine learning methods for isocitrate dehydrogenase genotype prediction of diffuse gliomas. J Cancer Res Clin Oncol 2019;145:543-50. [Crossref] [PubMed]
- Ren Y, Zhang X, Rui W, Pang H, Qiu T, Wang J, Xie Q, Jin T, Zhang H, Chen H, Zhang Y, Lu H, Yao Z, Zhang J, Feng X. Noninvasive Prediction of IDH1 Mutation and ATRX Expression Loss in Low-Grade Gliomas Using Multiparametric MR Radiomic Features. J Magn Reson Imaging 2019;49:808-17. [Crossref] [PubMed]
- Alis D, Bagcilar O, Senli YD, Yergin M, Isler C, Kocer N, Islak C, Kizilkilic O. Machine learning-based quantitative texture analysis of conventional MRI combined with ADC maps for assessment of IDH1 mutation in high-grade gliomas. Jpn J Radiol 2020;38:135-43. [Crossref] [PubMed]
- Sakai Y, Yang C, Kihira S, Tsankova N, Khan F, Hormigo A, Lai A, Cloughesy T, Nael K. MRI Radiomic Features to Predict IDH1 Mutation Status in Gliomas: A Machine Learning Approach using Gradient Tree Boosting. Int J Mol Sci 2020;21:8004. [Crossref] [PubMed]
- Kocak B, Durmaz ES, Ates E, Sel I, Turgut Gunes S, Kaya OK, Zeynalova A, Kilickesmez O. Radiogenomics of lower-grade gliomas: machine learning-based MRI texture analysis for predicting 1p/19q codeletion status. Eur Radiol 2020;30:877-86. [Crossref] [PubMed]
- van der Voort SR, Incekara F, Wijnenga MMJ, Kapas G, Gardeniers M, Schouten JW, Starmans MPA, Nandoe Tewarie R, Lycklama GJ, French PJ, Dubbink HJ, van den Bent MJ, Vincent AJPE, Niessen WJ, Klein S, Smits M. Predicting the 1p/19q Codeletion Status of Presumed Low-Grade Glioma with an Externally Validated Machine Learning Algorithm. Clin Cancer Res 2019;25:7455-62. [Crossref] [PubMed]
- Fayed N, Morales H, Modrego PJ, Pina MA. Contrast/Noise ratio on conventional MRI and choline/creatine ratio on proton MRI spectroscopy accurately discriminate low-grade from high-grade cerebral gliomas. Acad Radiol 2006;13:728-37. [Crossref] [PubMed]
- Hwan-Ho Cho. Hyunjin Park. Classification of low-grade and high-grade glioma using multi-modal image radiomics features. Annu Int Conf IEEE Eng Med Biol Soc 2017;2017:3081-4. [PubMed]
- Karabacak M, Ozkara BB, Mordag S, Bisdas S. Deep learning for prediction of isocitrate dehydrogenase mutation in gliomas: a critical approach, systematic review and meta-analysis of the diagnostic test performance using a Bayesian approach. Quant Imaging Med Surg 2022;12:4033-46. [Crossref] [PubMed]
- Tan Y, Zhang ST, Wei JW, Dong D, Wang XC, Yang GQ, Tian J, Zhang H. A radiomics nomogram may improve the prediction of IDH genotype for astrocytoma before surgery. Eur Radiol 2019;29:3325-37. [Crossref] [PubMed]
- Lin CJ, Chang FC, Lin CJ, Liaw YC, Tu PC, Wang PN, Saver JL, Lee IH. Long-term cognitive and multimodal imaging outcomes after carotid artery stenting vs intensive medication alone for severe asymptomatic carotid stenosis. J Formos Med Assoc 2022;121:134-43. [Crossref] [PubMed]
- Wu S, Zhang X, Rui W, Sheng Y, Yu Y, Zhang Y, Yao Z, Qiu T, Ren Y. A nomogram strategy for identifying the subclassification of IDH mutation and ATRX expression loss in lower-grade gliomas. Eur Radiol 2022;32:3187-98. [Crossref] [PubMed]
- Wang T, Xiao F, Wu G, Fang J, Sun Z, Feng H, Zhang J, Xu H. Impairments in Brain Perfusion, Metabolites, Functional Connectivity, and Cognition in Severe Asymptomatic Carotid Stenosis Patients: An Integrated MRI Study. Neural Plast 2017;2017:8738714. [Crossref] [PubMed]
- Parsons DW, Jones S, Zhang X, Lin JC, Leary RJ, Angenendt P, et al. An integrated genomic analysis of human glioblastoma multiforme. Science 2008;321:1807-12. [Crossref] [PubMed]
- Lu CF, Hsu FT, Hsieh KL, Kao YJ, Cheng SJ, Hsu JB, Tsai PH, Chen RJ, Huang CC, Yen Y, Chen CY. Machine Learning-Based Radiomics for Molecular Subtyping of Gliomas. Clin Cancer Res 2018;24:4429-36. [Crossref] [PubMed]
- Eneström S, Vavruch L, Frånlund B, Nordenskjöld B. Ki-67 antigen expression as a prognostic factor in primary and recurrent astrocytomas. Neurochirurgie 1998;44:25-30. [PubMed]
- Fisher BJ, Naumova E, Leighton CC, Naumov GN, Kerklviet N, Fortin D, Macdonald DR, Cairncross JG, Bauman GS, Stitt L. Ki-67: a prognostic factor for low-grade glioma? Int J Radiat Oncol Biol Phys 2002;52:996-1001. [Crossref] [PubMed]