Diagnostic and prognostic performance of renal compartment volume and the apparent diffusion coefficient obtained from magnetic resonance imaging in mild, moderate and severe diabetic kidney disease
Introduction
With the increasing prevalence of type 2 diabetes mellitus (DM), diabetic kidney disease (DKD), i.e., DM-associated chronic kidney disease (CKD), is the leading cause of end-stage renal disease (ESRD), with high morbidity and mortality (1). More than 400 million adults are estimated to suffer from DM worldwide, with ~40% developing DKD. In the USA, DKD accounts for >50% of patients who commence renal replacement therapy (2-4). As the prevalence of DM increases, DKD is a public health issue (5). Early DKD identification is of paramount importance for disease interception or slowing disease progression (4). In accordance with Clinical Practice Guidelines (6), CKD is still largely diagnosed by serum creatinine (SCr) concentration and SCr-based metrics, i.e., estimated glomerular filtration rate (eGFR), and assessments of proteinuria/albuminuria, e.g., the urinary protein-to-creatinine ratio (UPCR) and albumin-to-creatinine ratio (UACR). The very low sensitivity of SCr-based metrics largely prevents early detection of CKD (7,8). Renal biopsy is the ‘gold standard’ for the diagnosis of DKD. Due to its invasiveness, it is unsuitable for DKD screening (9). Noninvasive diagnostic tools are warranted in clinical settings for monitoring disease progression and therapeutic DKD intervention.
Magnetic resonance imaging (MRI) offers noninvasive assessment of renal macromorphology (e.g., renal volume and cysts) and microstructure (e.g., fibrosis and edema) in health and disease (10-13). Total kidney volume has been demonstrated to be a viable diagnostic metric and a macromorphology marker for renal disease progression (14-16). Recent studies have suggested that changes in volume in the renal parenchyma and/or the renal sinus fat are indicators of renal disease progression (17,18). Whether volume changes in distinct renal compartments may constitute diagnostic and prognostic markers of DKD remains to be investigated.
Diffusion-weighted imaging (DWI) probes tissue microstructure (19,20) using the metric apparent diffusion coefficient (ADC) (12). (Pre)clinical studies have shown the diagnostic potential of ADC mapping for the evaluation of pathophysiological changes such as interstitial fibrosis in DKD (21,22). Notwithstanding this progress, DKD studies have only examined the diagnostic potential of ADC; there are no literature reports on the prognostic performance of ADC mapping in DKD patients. Recently, the predictive value of ADC regarding the evolution of GFR in CKD was explored, yet divergent conclusions were drawn. While one study concluded that renal cortical ADC predicts GFR decline, another report found that the difference in ADC (∆ADC) between the cortex (ADCcortex) and medulla (ADCmedulla) rather than ADCcortex alone predicts it (23,24). This divergence might be due to differences in the DWI acquisition protocols. Irrespective of these differences, reproducible and harmonized data postprocessing procedures for renal ADC quantification are essential for its translation into clinical practice. Manual placement of regions of interest (ROIs) is the common approach for renal ADC quantification. Target ROIs encompass the whole kidney parenchyma or the cortex and medulla using arbitrary regions in the upper and lower poles and the middle of the kidney (25). The twelve-layer concentric objects (TLCO) approach uses semiautomatic segmentation of the renal parenchyma into 12 layers with equal thickness and provides a more objective alternative (26). It addresses the spatial heterogeneity of clinically obtained ADC maps and facilitates layer-specific assessment of changes in renal microstructure.
Recognizing these opportunities, this study evaluates the diagnostic and prognostic performance of specific macromorphological and microstructural MR markers in DKD cohorts with mild, moderate, and severe CKD staging. For this purpose, changes in renal compartment volume, including (I) parenchyma volume, (II) pelvis volume, (III) whole-kidney volume and (IV) parenchyma volume percentage, were assessed together with the microstructural surrogate ADC. These MR metrics were correlated with conventional clinical markers. Their long-term prognostic performance was examined using Cox survival analysis. We present the following article in accordance with the STARD reporting checklist (available at https://qims.amegroups.com/article/view/10.21037/qims-23-149/rc).
Methods
Patients
This cross-sectional and longitudinal, single-center, prospective study was approved by the local institutional review board of Guangdong Provincial People’s Hospital (No. GDREC2017253H) and registered at the Chinese Clinical Trial Registry Center (registration No. ChiCTR-RRC-17012687). The study was conducted in accordance with the Declaration of Helsinki (as revised in 2013).
Between July 2014 and July 2016, 67 DKD patients who were screened in the Division of Nephrology and Endocrinology of Guangdong Provincial People’s Hospital were randomly enrolled. Written informed consent was obtained from all participants (Figure 1). Fifteen individuals were excluded due to renal cysts (diameter >4 cm, n=1), polycystic kidney disease (n=1), or poor image quality (n=13). Poor image quality included (I) patients who denied the MRI examination after enrollment into the study and after signing the written consent (n=1); (II) patients who underwent the MRI exam including anatomical scans using T2-weighted images (T2WIs) but excluding DWI (n=4); and (III) patients who underwent the MRI exam including DWI but with unexpected DWI protocols, such as unexpected b-value settings (e.g., b=0 s/mm2 and b=1,000 s/mm2) (n=3), different MR scanners (i.e., Philips scanner 1.5 T) (n=1), incomplete DWI dataset scanned (e.g., only single-slice DWI with b=0 s/mm2 were scanned and stored in the Picture Archiving and Communication System (PACS) of the Guangdong Provincial People’s Hospital) (n=2), and imperfect prescan adjustment of the B1+ field leading to an extremely low signal in the kidneys (n=2). For the remaining 52 MRI scans, DWI images with both low b values (b=0 s/mm2) and high b values (b=600 s/mm2) and T2WIs were visually checked to confirm the appropriate signal-to-noise ratio and to ensure the absence of severe artifacts for both kidneys. Ultimately, 52 DKD patients were involved in the cross-sectional analysis. Of the 52 patients who underwent clinical and MRI examinations, 14 were excluded due to either being lost to follow-up (n=12) or being diagnosed with ESRD at the first visit (n=2). The remaining 38 patients were included in the prognostic analysis (median follow-up =8.25 years; IQR, 7.1–8.25 years).
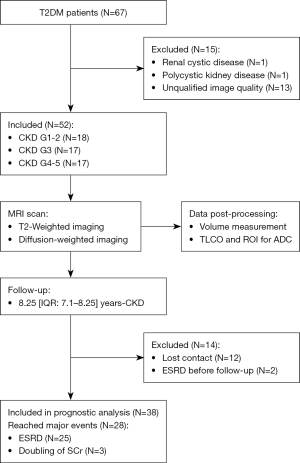
The initial diagnosis of DKD was based on the treating physician’s evaluation of the medical history of diabetes, hypertension, symptoms of diabetic retinopathy, etc. This evaluation also included laboratory assessments of renal biopsy, eGFR <60 mL/min/1.73 m2, or albuminuria over 3 months (24-hour albuminuria >30 mg or UACR >30 mg/g) according to the Kidney Disease Outcomes Quality Initiative (KDOQI) Clinical Practice Guideline for Diabetes and CKD (27). The diagnostic information and medical history of all patients were recorded and are available in the hospital information system (HIS). Patients were stratified into 3 stages according to eGFR (grade groups: G) (25): mild (CKD G1–2, eGFR ≥60 mL/min/1.73 m2), moderate (CKD G3, eGFR >30–59 mL/min/1.73 m2), and severe DKD (CKD G4–5, eGFR ≤29 mL/min/1.73 m2). The baseline characteristics of the patients are summarized in Table 1.
Table 1
Variables | CKD G1–2 | CKD G3 | CKD G4–5 | P value |
---|---|---|---|---|
N | 18 | 17 | 17 | n.a. |
Age (years) | 54.7±10.0 | 57.3±11.2 | 56.4±7.5 | 0.718 |
Gender (male), n (%) | 10 (55.5) | 12 (70.6) | 11 (64.8) | 0.662 |
DM duration (year) | 8.5 (3.5–12.0) | 12.0 (6.0–12.5) | 10.0 (8.0–12.5) | 0.184 |
BMI (kg/m2) | 23.7±3.5 | 24.7±3.0 | 24.9±3.1 | 0.513 |
BSA (m2) | 1.62±0.15 | 1.70±0.19 | 1.68±0.14 | 0.319 |
Hemoglobin (g/L) | 123.7±23.8 | 111.6±19.3 | 93.5±12.6 | <0.001 |
SCr (μmol/L) | 72.5 (57.8–84.1) | 136 (122.0–178.9) | 286.0 (236.9–374.3) | <0.001 |
BUN (mmol/L) | 5.7±2.5 | 9.3±3.2 | 14.5±5.3 | <0.001 |
eGFR (mL/min/1.73 m2) | 94.4±18.7 | 43.2±8.3 | 17.3±6.4 | <0.001 |
mGFR (mL/min/1.73 m2); n | 87.0±20.3; 15 | 42.1±10.3; 15 | 22.3±6.1; 15 | <0.001 |
HbA1c (%) | 9.7±3.0 | 7.6±1.6 | 8.0±1.1 | <0.05 |
UACR (mg/g) | 76.5 (49.9–1,179.9) | 1,302.9 (244.7–1,912.5) | 2,538.0 (1,802.9–3,852.5) | <0.001 |
UPCR (mg/g) | 198.3 (126.5–1,440.4) | 1,776.8 (359.9–2,656.9) | 3,745.1 (2,772.7–6,027.7) | <0.001 |
Data are presented as the mean ± SD or median with interquartile range unless stated otherwise. CKD stages were stratified by eGFR. DKD, diabetic kidney disease; CKD, chronic kidney disease; DM, diabetic mellitus; BMI, body mass index; BSA, body surface area; SCr, serum creatinine; BUN, blood urea nitrogen; eGFR, estimated glomerular filtration rate; mGFR, measured glomerular filtration rate; UACR, urine albumin-creatinine ratio; UPCR, urine protein-creatinine ratio; HbA1c, hemoglobin A1c; n.a., not available.
Outcomes
The end-time of follow-up was November 2022. The primary outcome was defined as the composite of doubling of SCr or ESRD (28). During the follow-up period, all patients received standard medical care according to guidelines with the goal of slowing or preventing disease progression. This included but was not limited to angiotensin-converting enzyme inhibitors/angiotensin receptor blockers (ACEIs/ARBs) for controlling blood pressure and insulin for controlling blood glucose. All patients were advised to ensure a healthy lifestyle involving sport activities and low-salt, low-protein, and low-sugar diets and were regularly visited every 6 months (25).
Clinical examination
Clinical examination followed the KDOQI guidelines. Participants were instructed to stop diuretics, water and food intake at least 6 hours before examination (27). Data routinely obtained included age, sex, DM duration, body mass index (BMI) and body surface area (BSA). A blood sample and a first-morning urine sample were collected to quantify hemoglobin (Hb) concentration, HbA1c, blood urea nitrogen (BUN), and SCr [based on which eGFR was calculated according to the CKD Epidemiology Collaboration (CKD-EPI) equation (29)] as well as the urinary concentrations of creatinine, total protein, and albumin, from which UPCR and UACR were derived. Finally, GFR was directly measured (mGFR) by clearance of 99mTc-diethylenetriaminepentaacetic acid utilizing emission computed tomography. As 7 patients denied this measurement due to concerns regarding radiation, mGFR was obtained in a total of 45 patients.
MRI examination
MRI was performed within one week after clinical assessment. Patients were instructed to stop taking diuretics, water and food at least 6 hours before MRI. MRI was conducted on a whole-body 3-T scanner (Signa Excite, GE Healthcare, Milwaukee, WI, USA) using a built-in body radiofrequency (RF) coil for excitation and an 8-channel RF torso surface array for reception. Respiratory triggering was used to eliminate the influence of motion artifacts on renal volume estimation using T2WI and on ADC quantification derived from DWI.
For anatomical imaging, two-dimensional (2D) coronal fat-suppressed T2-weighted (T2WI), multislice fast spin echo imaging was implemented: echo time (TE) =215 ms, repetition time (TR) =15,789 ms, number of signal average (NSA) =2, field of view (FOV) =320×320 mm2, acquisition matrix size =320×192, Generalized Autocalibrating Partial Parallel Acquisition (GRAPPA) factor =2, reconstructed in-plane resolution =0.625×0.625 mm2 including zero filling, slice thickness =3 mm, slice gap =0.6 mm, number of slices =24, receiver bandwidth =195 Hz/pixel, and fat suppression mode = Spectral Presaturation with Inversion Recovery (SPIR).
For DWI, 2D coronal fat-suppressed multislice single-shot spin-echo echo-planner imaging was implemented: TE =60 ms, TR =3,000 ms, NSA =4, FOV =320×320 mm2, acquisition matrix size =96×96, GRAPPA factor =2, reconstructed in-plane resolution =1.25×1.25 mm2 including zero filling, slice thickness =3 mm, slice gap =0.6 mm, number of slices =14, receiver bandwidth =1,953 Hz/pixel, diffusion weighting: b=0 and 600 s/mm2, and fat suppression mode = SPIR.
Data analysis
Figure 2 illustrates the postprocessing of T2WI and ADC maps analyzed by the TLCO approach. Morphological assessments concerned the renal volumes: (I) the parenchyma, (II) the pelvis, (III) the whole kidney, and (IV) the percentage of the parenchyma (i.e., the parenchyma volume divided by the whole-kidney volume). The parenchyma and pelvis were manually labeled by an experienced radiologist (6 years of experience in abdominal MRI) on T2WI, and the volumes were measured using ITK-SNAP (30). Representative labeling of the parenchyma and pelvis and the reconstructed three-dimensional (3D) surface mesh are shown in Figure 2A. Following segmentation, the calculated volumes were corrected by BSA using Stevenson’s formula, which is widely used for the Chinese population (31).
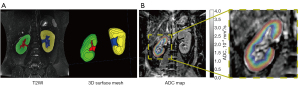
The middle coronal slice with the largest renal parenchyma area was selected and analyzed with TLCO for ADC quantification using homemade MATLAB scripts (MATLAB 2018b, MathWorks, Natick, MA).
The ADC map was calculated pixelwise using:
where ln() denotes the natural logarithm, b1 and b2 denote the diffusion weighting (b-values of b1=0 and b2=600 s/mm2), and S(b1) and S(b2) denote the signal intensity of DWI images at b1 and b2, respectively.
TLCO was applied to the ADC maps. The mean ADC values derived from the outer 6 layers were assigned to the renal cortex (ADCcortex), and those derived from the inner 6 layers were assigned to the medulla (ADCmedulla). ΔADC represents ADCcortex − ADCmedulla.
TLCO was compared with conventional ROI placement. For this purpose, three circular masks (radius =6 pixel) were manually placed at the upper, middle, and lower renal poles to obtain the mean ADCcortex and ADCmedulla. To compare the interobserver variability of TLCO and conventional ROI placement, two experienced double-blinded radiologists (6 and 5 years of experience in abdominal MRI) measured ADC by both methods in random order. Renal cysts and vessels were discarded from the TLCO and ROI analysis.
Statistical analysis
Statistical data analysis was conducted using SPSS (SPSS 26, SPSS Inc., Chicago, IL) and R v4.2.2 (https://www.r-project.org, Vienna, Austria) (32). Data are presented as the mean ± standard deviation or median with interquartile range, according to data distribution (assessed by the Shapiro-Wilk test). Differences in baseline characteristics among DKD groups were assessed by the F test. MR markers of renal compartment volumes, ADCcortex, ADCmedulla, ΔADC and ADC profiles along the cortico-medullary axis were averaged over the left and right kidney and were compared among DKD groups using one-way analysis of variance (ANOVA) with a post-hoc LSD test or Kruskal-Wallis one-way ANOVA with a post-hoc Bonferroni test. Interobserver reproducibility of ADC was assessed by Bland-Altman analysis, coefficient of variance (CoV) and intraclass correlation coefficient (ICC) type (2.1) (33). Pearson’s and Spearman’s analyses were used to assess correlations between MRI and clinical biomarkers. Receiver-operating characteristic (ROC) analysis and area under the curve (AUC) statistics were applied to evaluate the diagnostic performance of MR markers, and the optimal cutoff values were calculated at the maximized Youden index (34).
For the prognostic analysis, the median period of follow-up and its interquartile range were calculated according to the reverse Kaplan-Meier method (35). A Cox proportional hazard model was used to evaluate the association between MR markers and renal outcomes; eGFR and UACR were selected as covariates in the Cox model based on prior knowledge. Model performance was assessed using Harrell’s C-statistics and Akaike information criterion (AIC) (36). Model performance was compared using the likelihood ratio test. The influence of TLCO and ROI on the diagnostic and prognostic performance of ADC was assessed.
The correlation coefficients were classified as follows: |r|<0.2, no correlation, 0.2≤|r|<0.4, weak correlation, 0.4≤|r|<0.6, moderate correlation, 0.6≤|r|<0.8, strong correlation, and |r|≥0.8, extremely strong correlation, where operator |*| denotes the absolute value. P<0.05 was considered statistically significant.
Results
Baseline characteristics of DKD patients
Patients were stratified into 3 severity groups: mild (n=18), moderate (n=17), and severe DKD (n=17). No significant differences in age, sex, DM duration, BMI, or BSA were observed for the three groups. With increasing CKD stages, significant decreases in Hb, eGFR and mGFR (in line with SCr and BUN increase) and increases in UPCR and UACR were observed. HbA1c was ≥6.5% for all DKD groups, confirming DM for all enrolled patients.
Differences in MR markers among DKD groups
Representative postprocessing results of T2WI and ADC mapping for one exemplary DKD patient (45-year-old female with CKD G1) are shown in Figure 2. Representative masks generated by TLCO were superimposed on the calculated ADC map of the right kidney, and 12 layers were labeled with different colors.
ADCs and renal compartment volumes are provided in Table 2. For CKD stages G3 and G4–5, ADCcortex, ADCmedulla, and ΔADC were significantly lower than for G1–2. The parenchyma volume and whole-kidney volume were significantly lower for G3 and G4–5 than for G1–2. There were no significant differences among the stages for pelvis volume and the percentage of parenchyma volume.
Table 2
Variables | CKD G1–2 | CKD G3 | CKD G4–5 |
---|---|---|---|
ADCcortex (10−3 mm2/s) | 2.20±0.16 | 1.95±0.18*** | 1.87±0.14*** |
ADCmedulla (10−3 mm2/s) | 2.08±0.16 | 1.90±0.18** | 1.87±0.16*** |
ΔADC (10−3 mm2/s) | 0.11±0.08 | 0.04±0.08* | 0.00±0.08*** |
Parenchyma volume (mL/m2) | 100.5±22.2 | 83.5±23.3* | 75.6±19.6** |
Pelvis volume (mL/m2) | 16.6±3.8 | 15.5±5.6 | 15.4±3.2 |
Parenchyma percentage (%) | 85.9 (84.8–88.1) | 85.4 (81.9–87.5) | 82.4 (78.4–87.2) |
Kidney volume (mL/m2) | 117.2±23.7 | 99.0±25.7* | 90.9±19.1** |
Data are presented as the mean ± SD or median with interquartile range, as appropriate. P<0.05 was considered significant. *, post-hoc paired comparisons with CKD G1–2, P<0.05; **, post-hoc paired comparisons with CKD G1–2, P<0.01; ***, post-hoc paired comparisons with CKD G1–2, P<0.001. MR, magnetic resonance; DKD, diabetic kidney disease; CKD, chronic kidney disease; ADC, apparent diffusion coefficient; ADCcortex, ADC in the cortex; ADCmedulla, ADC in the medulla.
Comparison of ADC profiles among DKD groups
Figure 3 shows ADC profiles along the cortico-medullary axis. Significantly higher ADCs were observed for all 12 layers for the mild versus moderate (P<0.05) and severe DKD (P<0.01) groups, with the largest ADC difference observed in the cortex.
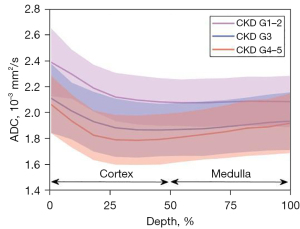
Correlations between MR markers and clinical biomarkers
The results of the correlation analysis are provided in Table 3. ADCcortex and ΔADC were significantly correlated with all clinical biomarkers, with moderate to strong correlations with eGFR and mGFR and moderate correlations with Hb and UACR. ADCmedulla showed a weak correlation with eGFR, a moderate correlation with mGFR, and a weak correlation with UACR. There was no significant correlation between ADCmedulla and Hb. The kidney and parenchyma volumes only show weak to moderate correlations with eGFR and mGFR.
Table 3
Variables | eGFR (n=52) | mGFR (n=45) | Hemoglobin (n=52) | UACR (n=52) | |||||||
---|---|---|---|---|---|---|---|---|---|---|---|
r | P value | r | P value | R | P value | r | P value | ||||
ADCcortex (10−3 mm2/s) | 0.581 | <0.001*** | 0.618 | <0.001*** | 0.415 | 0.002** | –0.511 | <0.001*** | |||
ADCmedulla (10−3 mm2/s) | 0.387 | 0.005** | 0.445 | 0.002** | 0.234 | 0.096 | –0.312 | 0.006** | |||
ΔADC (10−3 mm2/s) | 0.556 | <0.001*** | 0.538 | <0.001*** | 0.485 | <0.001*** | –0.529 | <0.001*** | |||
Parenchyma volume (mL/m2) | 0.405 | 0.003** | 0.370 | 0.012* | 0.010 | 0.942 | –0.006 | 0.968 | |||
Pelvis volume (mL/m2) | 0.119 | 0.401 | 0.180 | 0.235 | 0.146 | 0.301 | 0.009 | 0.952 | |||
Parenchyma percentage (%) | 0.269 | 0.053 | 0.213 | 0.160 | -0.065 | 0.647 | –0.063 | 0.685 | |||
Kidney volume (mL/m2) | 0.399 | 0.003** | 0.375 | 0.011* | 0.034 | 0.811 | –0.008 | 0.957 |
*, P<0.05; **, P<0.01; ***, P<0.001. eGFR, estimated glomerular filtration rate; mGFR, measured glomerular filtration rate; UACR, urine albumin-creatinine ratio; MR, magnetic resonance; ADC, apparent diffusion coefficient; ADCcortex, ADC in the cortex; ADCmedulla, ADC in the medulla.
Interobserver reproducibility of TLCO-based ADC assessment
Interobserver reproducibility of TLCO was benchmarked against conventional manual ROI placement. The Bland-Altman analysis demonstrated smaller 95% limits of agreement intervals for ADCcortex, ADCmedulla and ΔADC obtained from TLCO versus manually placed ROIs (Figure 4). This is in line with the higher ICC for TLCO versus manual ROIs (Table S1).
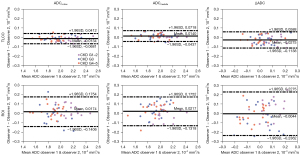
Diagnostic performance of MR markers
The mean AUC values were 0.904, 0.807 and 0.789 for ADCcortex, ADCmedulla, and ΔADC, respectively, as measured by TLCO and were higher than those measured by ROI (Table 4). The mean AUC values of the parenchyma and kidney volume were 0.760 and 0.768, respectively. Optimal cutoff values of MR markers were obtained at the maximum Youden index. Excellent discrimination performance was observed for ADCcortex with a sensitivity of 83.3% and specificity of 91.2%.
Table 4
Variables | AUC (95% CI) | Threshold | Sensitivity (%) | Specificity (%) | P value |
---|---|---|---|---|---|
ADCcortex (10−3 mm2/s) | |||||
TLCO | 0.904 (0.821–0.986) | 2.061 | 83.3 | 91.2 | <0.001*** |
ROI | 0.881 (0.785–0.976) | 2.002 | 83.3 | 79.4 | <0.001*** |
ADCmedulla (10−3 mm2/s) | |||||
TLCO | 0.807 (0.691–0.923) | 1.947 | 88.9 | 64.7 | <0.001*** |
ROI | 0.663 (0.512–0.815) | 1.931 | 83.3 | 47.1 | 0.054 |
ΔADC (10−3 mm2/s) | |||||
TLCO | 0.789 (0.658–0.920) | 0.053 | 83.3 | 70.6 | <0.001*** |
ROI | 0.786 (0.658–0.914) | 0.019 | 83.3 | 61.8 | <0.001*** |
Parenchyma volume (mL/m2) | 0.760 (0.622–0.897) | 77.41 | 94.0 | 50.0 | 0.002** |
Pelvis volume (mL/m2) | 0.601 (0.437–0.766) | 18.60 | 38.9 | 85.3 | 0.233 |
Parenchyma percentage (%) | 0.637 (0.484–0.790) | 85.01 | 77.8 | 58.8 | 0.106 |
Kidney volume (mL/m2) | 0.768 (0.632–0.904) | 110.1 | 66.7 | 79.4 | 0.002** |
**, P<0.01; ***, P<0.001. ROC, receiver operating characteristic; MR, magnetic resonance; DKD, diabetic kidney disease; eGFR, estimated glomerular filtration rate; AUC, area under the curve; CI, confidence interval; ADC, apparent diffusion coefficient; ADCcortex, ADC in the cortex; ADCmedulla, ADC in the medulla; TLCO, twelve-layer concentric objects; ROI, region of interest.
Prognostic performance of MR markers for predicting renal outcomes
Thirty-eight DKD patients were included in the prognostic analysis. After a median follow-up period of 8.25 years, the primary renal outcome occurred in 28 patients. The Cox survival analysis of the association between the renal outcome and the MR markers is summarized in Tables 5,6 and Table S2. Clinical predictors of age, sex, eGFR and UACR, which are associated with renal function decline, were integrated in the analysis. The univariate analysis demonstrated that eGFR, UACR, ADCcortex (as measured by TLCO), ΔADC, parenchyma volume and pelvis volume were significantly correlated with the outcome. The multivariate analysis revealed that ADCcortex as measured by TLCO independently predicted renal outcomes, while ΔADC and the parenchyma and pelvis volumes did not.
Table 5
Variables | Hazard ratio (95% CI) | P value |
---|---|---|
Age, per 10 years | 0.77 (0.54–1.10) | 0.149 |
Gender | ||
Male | Reference | |
Female | 1.03 (0.46–2.33) | 0.945 |
eGFR | ||
≥60 mL/min/1.73 m2 | Reference | |
<60 mL/min/1.73 m2 | 6.08 (2.26–16.40) | <0.001*** |
UACR | ||
<300 mg/g | Reference | |
≥300 mg/g | 9.19 (2.13–39.76) | 0.003** |
ADCcortex | ||
TLCO | ||
≥2.061×10−3 mm2/s | Reference | |
<2.061×10−3 mm2/s | 4.59 (1.73–12.21) | 0.002** |
ROI | ||
≥2.002×10−3 mm2/s | Reference | |
<2.002×10−3 mm2/s | 1.96 (0.92–4.17) | 0.081 |
ADCmedulla | ||
TLCO | ||
≥1.947×10−3 mm2/s | Reference | |
<1.947×10−3 mm2/s | 1.93 (0.92–4.08) | 0.084 |
ROI | ||
≥1.931×10−3 mm2/s | Reference | |
<1.931×10−3 mm2/s | 1.01 (0.48–2.13) | 0.971 |
ΔADC | ||
TLCO | ||
≥0.053×10−3 mm2/s | Reference | |
<0.053×10−3 mm2/s | 2.44 (1.14–5.22) | 0.022** |
ROI | ||
≥0.019×10−3 mm2/s | Reference | |
<0.019×10−3 mm2/s | 2.44 (1.16–5.13) | 0.018** |
Parenchyma volume | ||
≥77.41 mL/m2 | Reference | |
<77.41 mL/m2 | 2.33 (1.09–4.97) | 0.029** |
Pelvis volume | ||
≥18.60 mL/m2 | Reference | |
<18.60 mL/m2 | 3.05 (1.06–8.83) | 0.040** |
Parenchyma percentage | ||
≥85.01% | Reference | |
<85.01% | 1.26 (0.61–2.64) | 0.533 |
Kidney volume | ||
≥110.1 mL/m2 | Reference | |
<110.1 mL/m2 | 1.78 (0.81–3.92) | 0.153 |
**, P<0.01; ***, P<0.001. MR, magnetic resonance; CI, confidence interval; eGFR, estimated glomerular filtration rate; UACR, urine albumin–creatinine ratio; ADC, apparent diffusion coefficient; ADCcortex, ADC in the cortex; ADCmedulla, ADC in the medulla; TLCO, twelve-layer concentric objects; ROI, region of interest.
Table 6
Variables | Model 1a | Model 2b | Model 3c | Model 4d | Model 5e | Model 6f | |||||||||||
---|---|---|---|---|---|---|---|---|---|---|---|---|---|---|---|---|---|
HR (95% CI) | P value | HR (95% CI) | P value | HR (95% CI) | P value | HR (95% CI) | P value | HR (95% CI) | P value | HR (95% CI) | P value | ||||||
eGFR | 4.98 (1.80–13.79) | 0.002** | 3.69 (1.26–10.81) | 0.017* | 5.29 (1.87–14.94) | 0.002** | 4.56 (1.59–13.05) | 0.005** | 4.85 (1.61–14.64) | 0.005** | 4.29 (1.35–13.58) | 0.013* | |||||
UACR | 7.05 (1.61–30.97) | 0.010** | 10.07 (2.11–41.95) | 0.004** | 8.08 (1.73–37.86) | 0.008* | 6.74 (1.51–30.05) | 0.012** | 7.06 (1.61–30.97) | 0.010** | 7.19 (1.63–31.59) | 0.009** | |||||
ADCcortex | – | – | 3.40 (1.13–10.24) | 0.030* | – | – | – | – | – | – | – | – | |||||
ΔADC | |||||||||||||||||
TLCO | – | – | – | – | 0.78 (0.35–1.76) | 0.553 | – | – | – | – | – | – | |||||
ROI | – | – | – | – | – | – | 2.02 (0.92–4.48) | 0.082 | – | – | – | – | |||||
Parenchyma volume | – | – | – | – | – | – | – | – | 1.05 (0.46–2.39) | 0.906 | – | – | |||||
Pelvis volume | – | – | – | – | – | – | – | – | – | 1.36 (0.41–4.56) | 0.616 |
a, adjusted for eGFR and UACR; b, adjusted for eGFR, UACR and ADCCortex; c, adjusted for eGFR, UACR and ΔADC_TLCO; d, adjusted for eGFR, UACR and ΔADC_ROI; e, adjusted for eGFR, UACR and parenchyma volume; f, adjusted for eGFR, UACR and pelvis volume; *, P<0.05; **, P<0.01. MR, magnetic resonance; HR, hazard ratio; CI, confidence interval; eGFR, estimated glomerular filtration rate; UACR, urine albumin-creatinine ratio; ADC, apparent diffusion coefficient; ADCcortex, ADC in the cortex; TLCO, twelve-layer concentric objects; ROI, region of interest.
The prognostic performance of the Cox model using ADC only for the prediction of renal outcome was evaluated and compared against the clinical model that uses eGFR and UACR. For this purpose, a likelihood ratio test was applied using Harrell’s C-statistics. The results (Table S2) demonstrate that the Cox models using ADC only show significantly inferior C-Index than the clinical model using eGFR and UACR (P<0.001). This finding suggests that Cox models using only ADC as an input exhibit poorer model performance for the prediction of renal outcomes than the clinical model. The comparison of model performance of the multivariate Cox models (Table 7) revealed that adding ADCcortex as a covariant significantly improved the performance when included in the baseline model that relied on eGFR and UACR only (P=0.017). Adding ΔADC does not (P>0.05) improve the prediction.
Table 7
Variables | C-Index (95% CI) | AIC | P valuea |
---|---|---|---|
Model 1 | 0.748 (0.666–0.830) | 152.8 | – |
Model 2 | 0.780 (0.701–0.860) | 149.1 | 0.017* |
Model 3 | 0.761 (0.670–0.851) | 154.5 | 0.998 |
Model 4 | 0.783 (0.689–0.876) | 151.9 | 0.085 |
Model 5 | 0.745 (0.648–0.841) | 154.8 | 0.915 |
Model 6 | 0.757 (0.668–0.846) | 154.6 | 0.641 |
a, compared with Model 1; *, P<0.05. CI, confidence interval; AIC, Akaike information criterion.
Discussion
This study evaluated the diagnostic and prognostic performance of MR markers of macromorphological renal compartment volume changes and of the microstructural MR surrogate ADC in DKD cohorts with G1–G5 CKD stages. We revealed moderate correlations between ADCcortex and GFR, UACR, and Hb and demonstrated the best diagnostic performance of ADCcortex in discriminating DKD patients with normal versus declined eGFR. The Cox survival analysis demonstrated the prognostic value of ADCcortex as a predictor of renal outcomes independent of the clinical biomarkers eGFR and UACR.
To facilitate our study, the TLCO segmentation approach was adapted for ADC quantification. A previous work used three outer layers and 8 to 10 inner layers for the assessment of the MR metric R2* (a surrogate for blood oxygenation) (26). Our approach uses the outer 6 layers and the inner 6 layers for ADC quantification in the cortex and medulla, respectively. Computed tomography (CT) and ultrasound studies have confirmed that the cortex occupies one-third to half of the renal parenchyma width. In DM, cortical thickness is even expected to be increased (37-39). Therefore, a layer model that uses the outer 50% and the inner 50% of the renal parenchyma for ADC quantification in the cortex and medulla is advantageous.
Application of TLCO segmentation revealed that the ADC depends on the parenchyma depth. ADC was significantly higher for the cortex versus the medulla. This is in agreement with previous studies that reported a cortico-medullary ADC gradient using the conventional ROI approach (25). Our Bland-Altman and ICC analyses indicate that TLCO segmentation presents a highly reproducible alternative to the conventional approach. It is suitable for harmonization of and integration into standard data postprocessing pipelines tailored for renal ADC quantification in clinical studies.
In this work, we examined the diagnostic performance of MR markers of renal compartment volumes and ADCs. The best diagnostic performance was found for ADCcortex (AUC >0.9). Renal ADCs and the volumes of the parenchyma and whole kidney were significantly decreased in advanced CKD stages, which is consistent with recent reports (15). The decreases in ADCs and in renal volume can be attributed to advanced fibrosis and kidney atrophy (21,22). This is in line with the histological findings we obtained for 8 patients (Figure S1). We further explored the correlation between MR and clinical markers and demonstrated a moderate correlation between ADCcortex and ΔADC and clinical markers of GFR, Hb, and UACR. Only weak to moderate correlations between parenchyma and whole-kidney volumes and the clinical markers of GFR were observed. This is in line with the ADC results of a recent CKD study (40). Our findings suggest that the microstructural MR metric ADC-based marker ADCcortex is a more sensitive marker for the assessment of pathophysiological changes in DKD than the macromorphological renal compartment volumes obtained from T2-weighted MRI.
This is the first study that examines the prognostic performance of MR markers in DKD cohorts, including a long follow-up time of >8 years. Our univariate Cox analysis shows that ADCcortex and ΔADC are predictors of renal outcomes. However, ADC alone does not have better predictive performance than the clinical biomarkers eGFR and UACR. The multivariate Cox analysis including clinical biomarkers of eGFR and UACR revealed that ADCcortex rather than ΔADC significantly predicts renal outcomes. Our findings are consistent with a recent report on functional MRI and changes in eGFR in patients with CKD (23). Our results do not affirm another recent CKD study that concluded that “ΔADC can be a predictor of kidney function decline … in patients with native kidney disease or kidney allograft, independent of baseline kidney function and proteinuria” (24). Differences in the DWI methodology, postprocessing pipeline, disease etiology and stage might explain these divergent observations. The authors used a dedicated, read-out segmented DWI approach to reduce image distortion (24). As this approach substantially increases the imaging time (>5 times), it amplifies the sensitivity to bulk motion, which, by its interference with the diffusion-driven displacement of water, may compromise ADC quantification. Additionally, ROI-based ADC quantification is subject to observer experience, especially in the case of advanced CKD, where the discrimination between the cortex and medulla presents a challenge. This can be addressed with the semiautomatic TLCO approach, which facilitates minimum observer interaction for the discrimination of the outer and inner borders of the renal parenchyma (41).
There are some recognized limitations in the present study. First, although all three DKD groups were age- and sex-matched, the patient cohort was relatively small and warrants a larger sample size for future validation and reproducibility of our results. Second, DKD was confirmed with biopsy in a small subgroup of our patients, and the patients involved can be considered CKD plus type 2 DM in general, which is more common in clinical practice. Third, DWI was acquired at b=0 and 600 s/mm2 with limited diffusion direction, and only ADC was measured and analyzed. More advanced DWI techniques, such as intravoxel incoherent motion (IVIM) and diffusion tensor imaging (DTI) (42), are expected to provide further insights. It is an acknowledged caveat of our study that the effect of kurtosis using high b-values (b>1,000 s/mm2) was not considered. At high b-values, the probability distribution of the diffusion displacement deviates from a Gaussian distribution. This is considered to be a consequence of the restrictions on water molecule displacement imposed by microstructures (40,43). Finally, single-shot spin-echo EPI-based renal DWI still suffers from image blurring and artifacts; thus, future studies should involve more advanced DWI methodologies, such as accelerated read-out segmented EPI (44), in conjunction with semiautomated TLCO renal layer segmentation for robust and reproducible ADC quantification. Accelerated fast spin-echo MRI and multiband Rapid Acquisition with Relaxation Enhancement (RARE)-EPI hybrids constitute viable alternatives for improving the anatomic integrity of renal DWI (45).
Conclusions
This study evaluated the diagnostic and prognostic performance of renal compartment volumes and ADCs as measured by TLCO in DKD cohorts and demonstrated the diagnostic potential of ADCcortex in discriminating DKD patients with decreased renal function. The survival analysis demonstrated significant predictive value of ADCcortex for renal outcomes independently of clinical biomarkers. Therefore, we propose DWI in conjunction with TLCO for robust, reproducible, and comprehensive evaluation of ADC for DKD diagnosis and outcome prediction in future studies.
Acknowledgments
Funding: This work was supported by grants from the Key Area Research and Development Program of Guangdong Province (No. 2021B0101420006 to Z Liu), Regional Innovation and Development Joint Fund of National Natural Science Foundation of China (No. U22A20345 to Z Liu), Guangdong Provincial Key Laboratory of Artificial Intelligence in Medical Image Analysis and Application (No. 2022B1212010011 to Z Liu), National Natural Science Foundation of China (No. 82170731 & No. 81470974 to W Wang), High-level Hospital Construction Project of Guangdong Province (No. DFJH201908 to W Wang; No. DFJHBF202105 to Z Liu), and German Research Foundation (Deutsche Forschungsgemeinschaft) Collaborative Research Center (1365 “Renoprotection” Project B04 to E Seeliger).
Footnote
Reporting Checklist: The authors have completed the STARD reporting checklist. Available at https://qims.amegroups.com/article/view/10.21037/qims-23-149/rc
Conflicts of Interest: All authors have completed the ICMJE uniform disclosure form (available at https://qims.amegroups.com/article/view/10.21037/qims-23-149/coif). WW was supported by grants from the National Natural Science Foundation of China (Nos. 82170731 and 81470974) and the High-level Hospital Construction Project of Guangdong Province (No. DFJH201908). ZL was supported by grants from the Key Area Research and Development Program of Guangdong Province (No. 2021B0101420006), Regional Innovation and Development Joint Fund of National Natural Science Foundation of China (No. U22A20345), Guangdong Provincial Key Laboratory of Artificial Intelligence in Medical Image Analysis and Application (No. 2022B1212010011), and High-level Hospital Construction Project of Guangdong Province (No. DFJHBF202105). Erdmann Seeliger was supported by grants from the German Research Foundation (Deutsche Forschungsgemeinschaft) Collaborative Research Center (1365 “Renoprotection” Project B04). ZW reports being a full-time employee of Philips (2020-now) during the conduct of the study. The other authors have no conflicts of interest to declare.
Ethical Statement: The authors are accountable for all aspects of the work in ensuring that questions related to the accuracy or integrity of any part of the work are appropriately investigated and resolved. This cross-sectional and longitudinal, single-center, prospective study was approved by the local institutional review board of Guangdong Provincial People’s Hospital (No. GDREC2017253H) and registered at the Chinese Clinical Trial Registry Center (registration number: ChiCTR-RRC-17012687). The study was conducted in accordance with the Declaration of Helsinki (as revised in 2013). Written informed consent was obtained from all participants.
Open Access Statement: This is an Open Access article distributed in accordance with the Creative Commons Attribution-NonCommercial-NoDerivs 4.0 International License (CC BY-NC-ND 4.0), which permits the non-commercial replication and distribution of the article with the strict proviso that no changes or edits are made and the original work is properly cited (including links to both the formal publication through the relevant DOI and the license). See: https://creativecommons.org/licenses/by-nc-nd/4.0/.
References
- Thomas MC, Brownlee M, Susztak K, Sharma K, Jandeleit-Dahm KA, Zoungas S, Rossing P, Groop PH, Cooper ME. Diabetic kidney disease. Nat Rev Dis Primers 2015;1:15018. [Crossref] [PubMed]
- Zheng Y, Ley SH, Hu FB. Global aetiology and epidemiology of type 2 diabetes mellitus and its complications. Nat Rev Endocrinol 2018;14:88-98. [Crossref] [PubMed]
- DeFronzo RA, Reeves WB, Awad AS. Pathophysiology of diabetic kidney disease: impact of SGLT2 inhibitors. Nat Rev Nephrol 2021;17:319-34. [Crossref] [PubMed]
- Alicic RZ, Rooney MT, Tuttle KR. Diabetic Kidney Disease: Challenges, Progress, and Possibilities. Clin J Am Soc Nephrol 2017;12:2032-45. [Crossref] [PubMed]
- Molitch ME, Adler AI, Flyvbjerg A, Nelson RG, So WY, Wanner C, Kasiske BL, Wheeler DC, de Zeeuw D, Mogensen CE. Diabetic kidney disease: a clinical update from Kidney Disease: Improving Global Outcomes. Kidney Int 2015;87:20-30. [Crossref] [PubMed]
- KDIGO 2022 Clinical Practice Guideline for Diabetes Management in Chronic Kidney Disease. Kidney Int 2022;102:S1-S127. [Crossref] [PubMed]
- Jufar AH, Lankadeva YR, May CN, Cochrane AD, Bellomo R, Evans RG. Renal functional reserve: from physiological phenomenon to clinical biomarker and beyond. Am J Physiol Regul Integr Comp Physiol 2020;319:R690-702. [Crossref] [PubMed]
- Porrini E, Ruggenenti P, Luis-Lima S, Carrara F, Jiménez A, de Vries APJ, Torres A, Gaspari F, Remuzzi G. Estimated GFR: time for a critical appraisal. Nat Rev Nephrol 2019;15:177-90. [Crossref] [PubMed]
- Anders HJ, Huber TB, Isermann B, Schiffer M. CKD in diabetes: diabetic kidney disease versus nondiabetic kidney disease. Nat Rev Nephrol 2018;14:361-77. [Crossref] [PubMed]
- Selby NM, Blankestijn PJ, Boor P, Combe C, Eckardt KU, Eikefjord E, et al. Magnetic resonance imaging biomarkers for chronic kidney disease: a position paper from the European Cooperation in Science and Technology Action PARENCHIMA. Nephrol Dial Transplant 2018;33:ii4-ii14. [Crossref] [PubMed]
- Mahmoud H, Buchanan C, Francis ST, Selby NM. Imaging the kidney using magnetic resonance techniques: structure to function. Curr Opin Nephrol Hypertens 2016;25:487-93. [Crossref] [PubMed]
- Caroli A, Schneider M, Friedli I, Ljimani A, De Seigneux S, Boor P, Gullapudi L, Kazmi I, Mendichovszky IA, Notohamiprodjo M, Selby NM, Thoeny HC, Grenier N, Vallée JP. Diffusion-weighted magnetic resonance imaging to assess diffuse renal pathology: a systematic review and statement paper. Nephrol Dial Transplant 2018;33:ii29-40. [Crossref] [PubMed]
- Tao Q, Yi P, Cai Z, Chen Z, Deng Z, Liu R, Feng Y. Ratiometric chemical exchange saturation transfer pH mapping using two iodinated agents with nonequivalent amide protons and a single low saturation power. Quant Imaging Med Surg 2022;12:3889-902. [Crossref] [PubMed]
- Gladytz T, Millward JM, Cantow K, Hummel L, Zhao K, Flemming B, Periquito JS, Pohlmann A, Waiczies S, Seeliger E, Niendorf T. Reliable kidney size determination by magnetic resonance imaging in pathophysiological settings. Acta Physiol (Oxf) 2021;233:e13701. [Crossref] [PubMed]
- Makvandi K, Hockings PD, Jensen G, Unnerstall T, Leonhardt H, Jarl LV, Englund C, Francis S, Sundgren AK, Hulthe J, Baid-Agrawal S. Multiparametric magnetic resonance imaging allows non-invasive functional and structural evaluation of diabetic kidney disease. Clin Kidney J 2022;15:1387-402. [Crossref] [PubMed]
- Cantow K, Gladytz T, Millward JM, Waiczies S, Niendorf T, Seeliger E. Monitoring kidney size to interpret MRI-based assessment of renal oxygenation in acute pathophysiological scenarios. Acta Physiol (Oxf) 2023;237:e13868. [Crossref] [PubMed]
- Li Q, Wang D, Zhu X, Shen K, Xu F, Chen Y. Combination of renal apparent diffusion coefficient and renal parenchymal volume for better assessment of split renal function in chronic kidney disease. Eur J Radiol 2018;108:194-200. [Crossref] [PubMed]
- Spit KA, Muskiet MHA, Tonneijck L, Smits MM, Kramer MHH, Joles JA, de Boer A, van Raalte DH. Renal sinus fat and renal hemodynamics: a cross-sectional analysis. MAGMA 2020;33:73-80. [Crossref] [PubMed]
- Norris DG, Niendorf T, Leibfritz D. Health and infarcted brain tissues studied at short diffusion times: the origins of apparent restriction and the reduction in apparent diffusion coefficient. NMR Biomed 1994;7:304-10. [Crossref] [PubMed]
- Periquito JS, Gladytz T, Millward JM, Delgado PR, Cantow K, Grosenick D, Hummel L, Anger A, Zhao K, Seeliger E, Pohlmann A, Waiczies S, Niendorf T. Continuous diffusion spectrum computation for diffusion-weighted magnetic resonance imaging of the kidney tubule system. Quant Imaging Med Surg 2021;11:3098-119. [Crossref] [PubMed]
- Inoue T, Kozawa E, Okada H, Inukai K, Watanabe S, Kikuta T, Watanabe Y, Takenaka T, Katayama S, Tanaka J, Suzuki H. Noninvasive evaluation of kidney hypoxia and fibrosis using magnetic resonance imaging. J Am Soc Nephrol 2011;22:1429-34. [Crossref] [PubMed]
- Hueper K, Hartung D, Gutberlet M, Gueler F, Sann H, Husen B, Wacker F, Reiche D. Magnetic resonance diffusion tensor imaging for evaluation of histopathological changes in a rat model of diabetic nephropathy. Invest Radiol 2012;47:430-7. [Crossref] [PubMed]
- Srivastava A, Cai X, Lee J, Li W, Larive B, Kendrick C, Gassman JJ, Middleton JP, Carr J, Raphael KL, Cheung AK, Raj DS, Chonchol MB, Fried LF, Block GA, Sprague SM, Wolf M, Ix JH, Prasad PV, Isakova T. Kidney Functional Magnetic Resonance Imaging and Change in eGFR in Individuals with CKD. Clin J Am Soc Nephrol 2020;15:776-83. [Crossref] [PubMed]
- Berchtold L, Crowe LA, Combescure C, Kassaï M, Aslam I, Legouis D, Moll S, Martin PY, de Seigneux S, Vallée JP. Diffusion-magnetic resonance imaging predicts decline of kidney function in chronic kidney disease and in patients with a kidney allograft. Kidney Int 2022;101:804-13. [Crossref] [PubMed]
- Mrđanin T, Nikolić O, Molnar U, Mitrović M, Till V. Diffusion-weighted imaging in the assessment of renal function in patients with diabetes mellitus type 2. MAGMA 2021;34:273-83. [Crossref] [PubMed]
- Pruijm M, Milani B, Pivin E, Podhajska A, Vogt B, Stuber M, Burnier M. Reduced cortical oxygenation predicts a progressive decline of renal function in patients with chronic kidney disease. Kidney Int 2018;93:932-40. [Crossref] [PubMed]
- KDOQI Clinical Practice Guideline for Diabetes and CKD. 2012 Update. Am J Kidney Dis 2012;60:850-86. [Crossref] [PubMed]
- Jung HH. Evaluation of Serum Glucose and Kidney Disease Progression Among Patients With Diabetes. JAMA Netw Open 2021;4:e2127387. [Crossref] [PubMed]
- Levey AS, Stevens LA, Schmid CH, Zhang YL, Castro AF 3rd, Feldman HI, Kusek JW, Eggers P, Van Lente F, Greene T, Coresh J. CKD-EPI (Chronic Kidney Disease Epidemiology Collaboration). A new equation to estimate glomerular filtration rate. Ann Intern Med 2009;150:604-12. [Crossref] [PubMed]
- Yushkevich PA, Piven J, Hazlett HC, Smith RG, Ho S, Gee JC, Gerig G. User-guided 3D active contour segmentation of anatomical structures: significantly improved efficiency and reliability. Neuroimage 2006;31:1116-28. [Crossref] [PubMed]
- Stevenson P. Height. weight. surface formula for the estimation of surface area in Chinese subjects. Chinese Journal of Physiology 1937;12:327-30.
- The R Core Team. R: A Language and Environment for Statistical Computing. R Foundation for Statistical Computing, 2014.
- Shrout PE, Fleiss JL. Intraclass correlations: uses in assessing rater reliability. Psychol Bull 1979;86:420-8. [Crossref] [PubMed]
- Youden WJ. Index for rating diagnostic tests. Cancer 1950;3:32-5. [Crossref] [PubMed]
- Tiseo M, Boni L, Ambrosio F, Camerini A, Baldini E, Cinieri S, Brighenti M, Zanelli F, Defraia E, Chiari R, Dazzi C, Tibaldi C, Turolla GM, D'Alessandro V, Zilembo N, Trolese AR, Grossi F, Riccardi F, Ardizzoni A. Italian, Multicenter, Phase III, Randomized Study of Cisplatin Plus Etoposide With or Without Bevacizumab as First-Line Treatment in Extensive-Disease Small-Cell Lung Cancer: The GOIRC-AIFA FARM6PMFJM Trial. J Clin Oncol 2017;35:1281-7. [Crossref] [PubMed]
- Akaike H. A new look at the statistical model identification. IEEE Transactions on Automatic Control 1974;19:716-23. [Crossref]
- Koc AS, Sumbul HE. Renal cortical stiffness obtained by shear wave elastography imaging is increased in patients with type 2 diabetes mellitus without diabetic nephropathy. J Ultrasound 2018;21:279-85. [Crossref] [PubMed]
- Glodny B, Unterholzner V, Taferner B, Hofmann KJ, Rehder P, Strasak A, Petersen J. Normal kidney size and its influencing factors - a 64-slice MDCT study of 1.040 asymptomatic patients. BMC Urol 2009;9:19. [Crossref] [PubMed]
- Takata T, Koda M, Sugihara T, Sugihara S, Okamoto T, Miyoshi K, Hodotsuka M, Fujise Y, Matono T, Okano J, Hosho K, Iyama T, Fukui T, Fukuda S, Munemura C, Isomoto H. Left Renal Cortical Thickness Measured by Ultrasound Can Predict Early Progression of Chronic Kidney Disease. Nephron 2016;132:25-32. [Crossref] [PubMed]
- Liu Y, Zhang GM, Peng X, Li X, Sun H, Chen L. Diffusion kurtosis imaging as an imaging biomarker for predicting prognosis in chronic kidney disease patients. Nephrol Dial Transplant 2022;37:1451-60. [Crossref] [PubMed]
- Piskunowicz M, Hofmann L, Zuercher E, Bassi I, Milani B, Stuber M, Narkiewicz K, Vogt B, Burnier M, Pruijm M. A new technique with high reproducibility to estimate renal oxygenation using BOLD-MRI in chronic kidney disease. Magn Reson Imaging 2015;33:253-61. [Crossref] [PubMed]
- Zhang H, Wang P, Shi D, Yao X, Li Y, Liu X, Sun Y, Ding J, Wang S, Wang G, Ren K. Capability of intravoxel incoherent motion and diffusion tensor imaging to detect early kidney injury in type 2 diabetes. Eur Radiol 2022;32:2988-97. [Crossref] [PubMed]
- Jensen JH, Helpern JA, Ramani A, Lu H, Kaczynski K. Diffusional kurtosis imaging: the quantification of non-gaussian water diffusion by means of magnetic resonance imaging. Magn Reson Med 2005;53:1432-40. [Crossref] [PubMed]
- Friedli I, Crowe LA, Viallon M, Porter DA, Martin PY, de Seigneux S, Vallée JP. Improvement of renal diffusion-weighted magnetic resonance imaging with readout-segmented echo-planar imaging at 3T. Magn Reson Imaging 2015;33:701-8. [Crossref] [PubMed]
- Paul K, Huelnhagen T, Oberacker E, Wenz D, Kuehne A, Waiczies H, Schmitter S, Stachs O, Niendorf T. Multiband diffusion-weighted MRI of the eye and orbit free of geometric distortions using a RARE-EPI hybrid. NMR Biomed 2018; [Crossref] [PubMed]