Deep learning for the prediction of residual tumor after radiotherapy and treatment decision-making in patients with nasopharyngeal carcinoma based on magnetic resonance imaging
Introduction
Nasopharyngeal carcinoma (NPC) is a malignant tumor originating from the nasopharyngeal epithelium with obvious regional distribution characteristics, and it is most common in Southeast and East Asia. The incidence of NPC in China accounts for 46.9% of cases globally, and the standardized incidence and mortality in China are significantly higher than the world average, ranking 13th and 20th, respectively (1). Unfortunately, more than 70% of newly diagnosed patients have locoregionally advanced (stage III–IVA) NPC. Patients with early-stage (stage I and II) NPC have a better prognosis, with a 5-year overall survival (OS) rate of more than 90%, compared to those with locoregionally advanced disease, who have a 5-year OS of 71–85% (2). Therefore, the management of locally advanced NPC remains a challenge for clinicians. The main reasons for treatment failure include residual tumor, recurrent primary tumor, metastasis to cervical lymph nodes, and distant metastasis, all of which reduce OS (3). The long-term prognosis of patients with residual tumor after radiotherapy is poor, and the 5-year OS rate is only 76.6% (3-5), whereas the 5-year OS rate, local recurrence, and distant metastasis-free rate of patients without residual tumor are up to 90% (4,5). Thus, residual tumor after radiotherapy is an important adverse prognostic factor affecting the survival of patients with NPC. Accurate prediction of residual tumor before radiotherapy and a stricter intensive treatment strategy for high-risk patients would improve the treatment effect and survival rate of NPC patients.
In the 2020 European Society for Medical Oncology-European Reference Network for rare adult solid cancers (ESMO-EURACAN) clinical practice guidelines, concurrent chemoradiotherapy (CCRT) alone or induction chemotherapy (IC) plus CCRT (IC + CCRT) are currently recommended for patients with advanced NPC (6). Several prospective multicenter randomized controlled trials (RCTs) have shown that IC + CCRT significantly prolongs local recurrence-free survival (RFS), failure-free survival, and overall survival (OS) in patients with advanced disease compared with CCRT alone (7-10). However, compared with CCRT alone, IC has been found to cause a significantly higher incidence (up to 40%) of grade 3 or 4 adverse events, such as neutropenia, leukopenia, and stomatitis (8,11). Additionally, there are significant differences in the efficacy of IC in different patients. IC can effectively reduce tumor size in some patients yet not show significant efficacy in others (12,13). The limited benefit, apparent toxicity, and differential patient responses to IC suggest that patients who will benefit most should be identified prior to clinical decision-making. Zhao et al. extracted 19 radiomic features from pre-treatment magnetic resonance (MR) images to predict the efficacy of IC and found that the radiomic nomogram established by combining radiomic features and clinical data could effectively predict its efficacy (14).
However, despite the accurate prediction of a patients’ sensitivity to IC, whether an IC is the best option for an individual cannot be determined, as many patients in the advanced stage can achieve non-residual tumor after radiotherapy with CCRT. In other words, even if these patients respond well to IC, this therapy is redundant for them. Therefore, when deciding whether patients should receive IC, both their sensitivity to it and their prognosis after CCRT should be considered, and excessive treatment should be avoided wherever possible to achieve the best prognosis. However, in clinical practice, it is not possible to accurately select the most appropriate treatment for patients because the corresponding effect of different treatments cannot be predicted. Therefore, a prediction tool that can be utilized for individualized treatment, to obtain information on the prognosis of patients with various treatment regimens in advance, and to select the regimen with fewer side effects yet maximum efficacy should be developed.
This study aimed to establish a deep learning (DL) model based on pre-treatment MR images of the nasopharynx and neck of patients who then received CCRT and IC + CCRT to predict the risk of residual tumor after either treatment. We hoped to provide a reference for patients to select better treatment options, and to screen out high-risk patients who cannot achieve non-residual tumor with either treatment plan, so that they can progress to a more intensive treatment to improve their prognosis. In addition, this study compared the residual tumor status of patients treated with CCRT and IC + CCRT, and the model formed a recommendation regimen according to the treatment strategy and the corresponding residual status after radiotherapy. The model recommendation regimen was compared with a clinician-selected regimen to explore the feasibility of making treatment decisions based on DL (Figure 1). We present the following article in accordance with the TRIPOD reporting checklist (available at https://qims.amegroups.com/article/view/10.21037/qims-22-1226/rc).
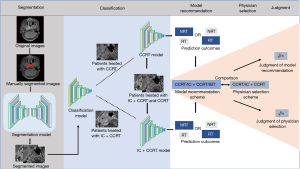
Methods
Patients
Data from 424 patients with locoregionally advanced NPC, diagnosed and treated with CCRT or IC + CCRT in Renmin Hospital of Wuhan University from June 2012 to June 2019, were collected. The sample size was determined based on practical considerations. The study was conducted in accordance with the Declaration of Helsinki (as revised in 2013), and the study protocol was approved by the Institutional Ethics Committee of the Renmin Hospital of Wuhan University. The requirement for informed consent was waived due to the retrospective nature of the study. The inclusion criteria were as follows: primary NPC pathologically diagnosed and treated at our hospital; treatment with CCRT or IC + CCRT; Magnetic resonance imaging (MRI) examination of the nasopharynx and neck taken within 1 month prior to treatment; locally advanced NPC; complete and uninterrupted chemoradiotherapy; MRI examination of the nasopharynx and neck performed 3–6 months after radiotherapy; no evidence of distant metastasis at the beginning of treatment; paclitaxel plus cisplatin or gemcitabine plus cisplatin use during IC; CCRT performed with intensity modulated radiotherapy (IMRT) plus cisplatin or nedaplatin. The exclusion criteria were as follows: lack of axial T1-weighted enhanced sequence and recurrent NPC after radiotherapy. The prescribed doses were 2.24 Gy × 33 fractions =73.92 Gy to the nasopharynx gross tumor volume (GTVnx), 2.12 Gy × 33 fractions =69.96 Gy to the lymph node gross tumor volume (GTVnd), and 1.8 Gy × 33 fractions =59.40 Gy and 1.64 Gy × 33 fractions =54.12 Gy to the clinical target volumes 1 and 2 (CTV1 and CTV2), respectively. Two experienced head and neck radiologists reviewed the MR images taken 3–6 months after the completion of radiotherapy to evaluate residual tumor. The treatment method, pre-treatment stage, and clinical diagnosis of the residual tumor of the patient were concealed during the evaluation. The diagnostic criteria for residual tumor were as follows (15): (I) residual tumor in the nasopharynx and soft tissue presented as hypointense on T1-weighted images, hyperintense on T2-weighted images, and enhancement following administration of Gd-DTPA; (II) lymph node residue diagnosed when the short-axis diameter of cervical lymph nodes was >10 mm or the short-axis diameter of retropharyngeal lymph nodes was >5 mm; (III) soft tissue invasion of the skull or no reduction or increase in skull base bone enhancement compared with pre-treatment images. A total of 16 experienced oncologists participated in treating patients with NPC in the study. As this was a retrospective study, we excluded patients with missing MR images and treatment information, and only included those with complete data.
Image acquisition and pre-processing
All patients underwent examination of the nasopharynx and neck with a 3.0-T MR scanner (GE Discovery MR750; GE Healthcare, Chicago, IL, USA) and were provided with pre-treatment axial T1-weighted enhanced images stored in DICOM format with a size of 512×512 pixels. Patients were placed in a supine position, and the scanning range was between 2 cm above the sella turcica and 2 cm below the lower clavicle margin. The contrast agent (15 mL gadolinium-labeled diethylenetriaminepentaacetic acid) was injected at 1.5 mL/s. The MRI parameters were as follows: repetition time, 2,699–4,480 ms; echo time, 67–117 ms; turnover angle, 111–142°; slice thickness, 4–6 mm; pixel size, 1.25 × 1.25 mm.
A total of 80 patients were randomly selected, and their images were imported into the ITK-SNAP software (http://www.itksnap.org/pmwiki/pmwiki.php). An experienced radiologist outlined the edges of the primary NPC lesion and cervical lymph nodes with a diameter greater than 1 cm layer-by-layer to mark their range, and a senior radiologist reviewed the marked results. The treatment method, evaluation, and clinical diagnosis of the residual tumor of the patient were concealed from the marking radiologists, and the original images and marked area of each image were saved correspondingly for the training and testing of the segmentation model. The trained segmentation model segmented the tumor regions on the MR images of the remaining patients, and the segmented images were used to train the classification model. A total of 210 patients (1,686 images) treated with CCRT were used to constitute the CCRT classification model dataset, and 214 patients (2,185 images) treated with IC + CCRT constituted the IC + CCRT classification model dataset. Patients in each category were randomly assigned to the training and test cohorts at a ratio of 4:1. Considering the heterogeneity of tumors and that the patient’s prognosis or risk of metastasis cannot be attributed to each lesion slice, we constructed a dataset using each patient as a unit, in addition to traditional datasets using each image as a unit. Each patient was first labeled, then the average probability value of all images obtained from each was set to the probability value of the patient and input into the model for learning.
Network architecture and model development
Our compiling platform was based on the Pytorch library (version 1.9.0; https://pytorch.org/) with CUDA (version 10.0; https://developer.nvidia.com/cuda-10.0) for GPU (NVIDIA T4) acceleration on a Windows operating system (Server 2019 data center version 64-bit). U-net (16) is the most used neural network segmentation framework for medical images. It adopts the encoder and decoder network structure and adds jump connections between feature maps of the same size as the encoder and decoder to achieve the fusion of high-dimensional and low-dimensional features of the image. DeepLabv3 (17) is one of the latest semantic segmentation networks using a multi-scale convolutional layer and encoder-decoder structure to improve segmentation accuracy. We manually segmented 700 images to construct a dataset for training the semantic segmentation model, which was built by transferring the U-Net and Deeplabv3 networks. The RMSprop Optimizer was used to train the models, with the initial learning rate and batch size set at 0.001 and 32, respectively. Each semantic segmentation model was trained for 20 epochs. The Dice similarity coefficient was used to evaluate the performance of the models, and the model with the highest coefficient was used to segment the tumor areas on the MR images with a rectangular segmentation method (Figure 2).
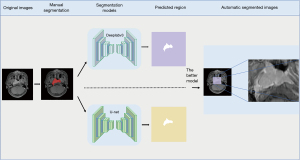
We transferred 4 common neural networks for classification model building [efficientnet_b0 (18), inception_resnet_v2 (19), resnet50 (20), and Xception] to avoid bias of the model caused by different networks having different data preferences, and CCRT and IC + CCRT datasets were used for training each model separately (Figure 3). These networks were trained using SGD optimizer with the initial learning rate and batch size set at 0.001 and 32, respectively, and each model was trained for 40 epochs. Full details of the model are available at https://github.com/yangzhu45623/lingongzi666.
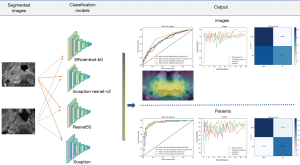
Formulation of model recommendations and comparison with physician decisions
We selected the trained CCRT and IC + CCRT models with the best performance among the 4 networks to predict the treatment effect of the 2 regimens on patients and to form recommendations based on the effect of treatment. After training the CCRT and IC + CCRT models, the test cohorts of the CCRT and IC + CCRT datasets were successively input into the models for testing, and the prediction results of the models for each patient were compared. The final model recommended appropriate treatment according to the prediction results of the 2 treatment regimens. The recommended principle was to select a treatment regimen with fewer side effects on the proviso that patients achieved non-residual tumor.
One of the 4 following situations (Figure 4) was applicable to each patient: (I) When both the CCRT and IC + CCRT models predicted the patient had non-residual tumor, the model indicated that the patient could achieve non-residual tumor with CCRT only, so the patient was recommended to adopt CCRT. (II) When the CCRT model predicted non-residual tumor and the IC + CCRT model predicted residual tumor, the model indicated that CCRT could achieve non-residual tumor but IC + CCRT could not, so the patient was recommended to adopt CCRT. (III) When the IC + CCRT model predicted non-residual tumor and the CCRT model predicted residual tumor, the model indicated that the patient could only achieve non-residual tumor by IC + CCRT, so the patient was recommended to undergo IC + CCRT. (IV) When both the CCRT and IC + CCRT models predicted residual tumor, the model indicated that neither treatment plan could achieve non-residual tumor, and a more aggressive and individual-based treatment (IBT) was recommended.
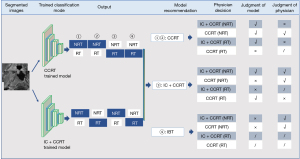
Finally, we compared the model-recommended regimen with the actual regimen selected by a physician and their corresponding effects. The model-recommended regimens and the corresponding treatment effect had 3 conditions: CCRT (non-residual tumor), IC + CCRT (non-residual tumor), and IBT (residual tumor). The physician-selected regimens and the corresponding treatment effect had 4 conditions: IC + CCRT (non-residual tumor), IC + CCRT (residual tumor), CCRT (non-residual tumor), and CCRT (residual tumor). Accordingly, patients could present with a total of 12 conditions for model recommendation and physician selection (Figure 3). The principle of judging whether the decisions made by the model and physician decisions were correct was to avoid excessive treatment while ensuring patients achieved non-residual tumor. For example, when the model predicted CCRT could achieve non-residual tumor, CCRT would be recommended. At this time, if the physician chooses IC + CCRT and the actual effect is non-residual tumor, the patient could, in fact, achieve non-residual tumor without additional IC, in which case the model is correct, and the physician is wrong. If the physician chooses IC + CCRT, and the actual effect is residual tumor, the model is correct, and the physician is wrong, whereas if the physician chooses CCRT and the actual effect is non-residual tumor, both the model and the physician are correct. If the physician chooses CCRT and the actual effect is residual tumor, the model prediction is wrong, and it is not yet capable of judging whether the physician’s decision is correct. When the model predicts the therapeutic effect of CCRT is residual tumor, but IC + CCRT can achieve non-residual tumor, IC + CCRT will be recommended, and if the physician chooses IC + CCRT and the actual effect is non-residual tumor, we believe both the model recommendation and the physician’s decision are correct. If the physician chooses IC + CCRT and the actual effect is residual tumor, the model prediction error will lead to a recommendation error and it is impossible to judge whether the physician’s decision is correct, and if the physician chooses CCRT and the actual effect is also non-residual tumor, the physician’s decision is judged to be accurate, and the model recommendation is wrong. If the physician chooses CCRT and the actual effect is residual tumor, the model recommendation is correct, and the physician’s decision is wrong. When the model believes neither of the 2 schemes can achieve non-residual tumor and recommends IBT, if the physician chooses IC + CCRT or CCRT to achieve non-residual tumor, then the model decision is wrong, and the physician’s decision is correct. Finally, if the effect of IC + CCRT or CCRT is residual tumor, we believe the patient needs IBT, and it is not yet possible to judge whether the model and physician’s decision are correct. In addition, only when the prediction results of the model are consistent with the actual results of patients can the model regimen be judged as correct.
Statistical analysis
Statistical analyses were performed using SPSS 22.0 (IBM Corp., Armonk, NY, USA) statistical software. Measurement data with normal distribution were expressed as () and analyzed by independent sample t-test, and counting data were presented as frequencies and analyzed using the chi-square test. Statistical significance was set at P<0.05. The Dice similarity coefficient was used to evaluate the performance of the segmentation model, whereas receiver operating characteristic (ROC) curve, accuracy, and confusion matrix were used to evaluate the classification model.
Results
Patient characteristics
After screening, we enrolled 210 patients undergoing CCRT and 214 patients undergoing IC + CCRT to construct the CCRT and IC + CCRT classification models. The residual tumor ratio was 34.52% (58/168) in the training cohort and 42.86% (18/42) in the test cohort of the CCRT model, and 23.98% (41/171) in the training cohort and 25.58% (11/43) in the test cohort of the IC + CCRT model. Five clinical factors associated with residual tumor: age, sex, American Joint Committee on Cancer (AJCC) stage, T stage, and N stage, were evenly distributed between the 2 cohorts of the CCRT and IC + CCRT models (Table 1).
Table 1
Characteristics | CCRT | IC + CCRT | P | |||||||||||||||
---|---|---|---|---|---|---|---|---|---|---|---|---|---|---|---|---|---|---|
Training cohort | Test cohort | P | Training cohort | Test cohort | P | |||||||||||||
NRT | RT | P | NRT | RT | P | NRT | RT | P | NRT | RT | P | |||||||
Patients, n (%) | 110 (65.48) | 58 (34.52) | 24 (57.14) | 18 (42.86) | 0.315 | 130 (76.02) | 41 (23.98) | 32 (74.42) | 11 (25.58) | 0.826 | 0.008 | |||||||
Age (year), mean ± SD | 53.80±10.47 | 54.29±10.89 | 0.776 | 55.37±11.71 | 51.89±11.20 | 0.337 | 0.962 | 48.70±10.98 | 49.17±11.89 | 0.815 | 49.41±11.07 | 51.45±17.22 | 0.651 | 0.569 | 0.000 | |||
Sex, n (%) | ||||||||||||||||||
Male | 66 (39.29) | 43 (25.60) | 14 (33.33) | 12 (28.57) | 100 (58.48) | 32 (18.71) | 25 (58.14) | 8 (18.60) | ||||||||||
Female | 44 (26.19) | 15 (8.93) | 0.068 | 10 (23.81) | 6 (14.29) | 0.582 | 0.719 | 30 (17.54) | 9 (5.26) | 0.881 | 7 (16.28) | 3 (6.98) | 1.000 | 0.950 | 0.004 | |||
AJCC stage, n (%) | ||||||||||||||||||
III | 94 (55.95) | 22 (13.10) | 21 (50.00) | 5 (11.90) | 88 (51.46) | 8 (4.68) | 22 (51.16) | 2 (4.65) | ||||||||||
IVa | 16 (9.52) | 36 (21.43) | 0.000 | 3 (7.14) | 13 (30.95) | 0.000 | 0.376 | 42 (24.56) | 33 (19.30) | 0.000 | 10 (23.26) | 9 (20.93) | 0.000 | 0.969 | 0.014 | |||
T stage, n (%) | ||||||||||||||||||
T1 | 14 (8.33) | 0 (0.00) | 2 (4.76) | 0 (0.00) | 9 (5.26) | 0 (0.00) | 1 (2.33) | 0 (0.00) | ||||||||||
T2 | 30 (17.86) | 7 (4.17) | 9 (21.43) | 2 (4.76) | 33 (19.30) | 0 (0.00) | 11 (25.58) | 0 (0.00) | ||||||||||
T3 | 51 (30.36) | 16 (9.52) | 12 (28.57) | 4 (9.52) | 58 (33.92) | 11 (6.43) | 16 (37.21) | 2 (4.65) | ||||||||||
T4 | 15 (8.93) | 35 (20.83) | 0.000 | 1 (2.38) | 12 (28.57) | 0.000 | 0.821 | 30 (17.54) | 30 (17.54) | 0.000 | 4 (9.30) | 9 (20.93) | 0.000 | 0.652 | 0.503 | |||
N stage, n (%) | ||||||||||||||||||
N0 | 26 (15.48) | 4 (2.38) | 5 (11.90) | 0 (0.00) | 19 (11.11) | 2 (1.17) | 2 (4.65) | 0 (0.00) | ||||||||||
N1 | 26 (15.48) | 19 (11.31) | 6 (14.29) | 4 (9.52) | 50 (29.24) | 10 (5.85) | 9 (20.93) | 2 (4.65) | ||||||||||
N2 | 52 (30.95) | 30 (17.86) | 11 (26.19) | 11 (26.19) | 45 (26.32) | 19 (11.11) | 14 (32.56) | 7 (16.28) | ||||||||||
N3 | 6 (3.57) | 5 (2.98) | 0.032 | 2 (4.76) | 3 (7.14) | 0.083 | 0.551 | 16 (9.36) | 10 (5.85) | 0.040 | 7 (16.28) | 2 (4.65) | 0.531 | 0.175 | 0.003 |
The P value in the last column is the significance test value of the general data between patients treated with CCRT and those treated with IC + CCRT. CCRT, concurrent chemoradiotherapy; IC, induction chemotherapy; NRT, non-residual tumor; RT, residual tumor; AJCC, American Joint Committee on Cancer.
Results of the semantic segmentation models
After training for 20 epochs, the performances of Deeplabv3 and U-net gradually stabilized, and their Dice scores were 0.752 [95% confidence intervals (CI): 0.736–0.768] and 0.689 (95% CI: 0.675–0.703), respectively (Figure 5). Given the outperformance of Deeplabv3 over U-net, we used the Deeplabv3 network to perform rectangular segmentation of tumor regions in MR images.
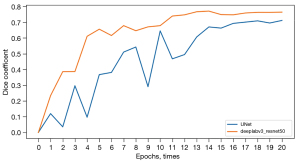
Performance of the classification models
The area under the curves (AUCs) of the efficientnet_b0, inception_resnet_v2, resnet50, and Xception networks trained with a single image as a unit were 0.713 (95% CI: 0.659–0.767), 0.720 (95% CI: 0.675–0.765), 0.778 (95% CI: 0.728–0.828), and 0.702 (95% CI: 0.640–0.764) (Figure 6A) and increased to 0.931 (95% CI: 0.884–0.978), 0.931 (95% CI: 0.879–0.983), 0.907 (95% CI: 0.857–0.957), and 0.938 (95% CI: 0.895–0.981) in the CCRT model (Figure 6B), respectively. The AUCs of the 4 neural networks trained with a single image as a unit were 0.806 (95% CI: 0.756–0.856), 0.834 (95% CI: 0.786–0.882), 0.833 (95% CI: 0.791–0.875), and 0.837 (95% CI: 0.792–0.882) (Figure 6C), and increased to 0.864 (95% CI: 0.818–0.910), 0.888 (95% CI: 0.835–0.941), 0.953 (95% CI: 0.911–0.995), and 0.955 (95% CI: 0.911–0.999) when trained with each patient as a unit in the IC + CCRT model (Figure 6D), respectively (Table 2). The overall performance of the Xception network was better than that of the other networks. The accuracy during the training process reflects the overall performance of the CCRT and IC + CCRT models (Figure 7).
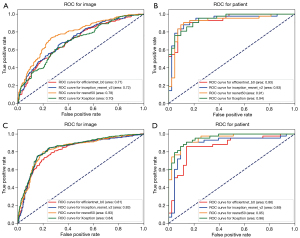
Table 2
Models | Datasets | Networks | ACC | Se | Sp | ROC | 95% CI |
---|---|---|---|---|---|---|---|
CCRT | Image | Efficientnet_b0 | 0.710 | 0.914 | 0.431 | 0.713 | 0.659–0.767 |
Inception_resnet_v2 | 0.714 | 0.948 | 0.456 | 0.720 | 0.675–0.765 | ||
Resnet50 | 0.735 | 0.871 | 0.638 | 0.778 | 0.728–0.828 | ||
Xception | 0.707 | 0.983 | 0.394 | 0.702 | 0.640–0.764 | ||
Average | 0.717 | 0.929 | 0.480 | 0.728 | |||
Patient | Efficientnet_b0 | 0.815 | 0.875 | 0.889 | 0.931 | 0.884–0.978 | |
Inception_resnet_v2 | 0.840 | 0.917 | 0.833 | 0.931 | 0.879–0.983 | ||
Resnet50 | 0.862 | 0.833 | 0.944 | 0.907 | 0.857–0.957 | ||
Xception | 0.788 | 0.792 | 0.944 | 0.938 | 0.895–0.981 | ||
Average | 0.826 | 0.854 | 0.903 | 0.928 | |||
IC + CCRT | Image | Efficientnet_b0 | 0.761 | 0.884 | 0.433 | 0.806 | 0.756–0.856 |
Inception_resnet_v2 | 0.745 | 0.873 | 0.510 | 0.834 | 0.786–0.882 | ||
Resnet50 | 0.736 | 0.931 | 0.471 | 0.833 | 0.791–0.875 | ||
Xception | 0.785 | 0.924 | 0.558 | 0.837 | 0.792–0.882 | ||
Average | 0.757 | 0.903 | 0.493 | 0.828 | |||
Patient | Efficientnet_b0 | 0.860 | 0.833 | 0.923 | 0.864 | 0.818–0.910 | |
Inception_resnet_v2 | 0.792 | 0.867 | 0.923 | 0.888 | 0.835–0.941 | ||
Resnet50 | 0.792 | 0.933 | 0.769 | 0.953 | 0.911–0.995 | ||
Xception | 0.838 | 0.867 | 0.923 | 0.955 | 0.911–0.999 | ||
Average | 0.821 | 0.875 | 0.885 | 0.915 |
ACC, accuracy; Se, sensitivity; Sp, specificity; ROC, receiver operating characteristic; CCRT, concurrent chemoradiotherapy; IC, induction chemotherapy; CI, confidence interval.
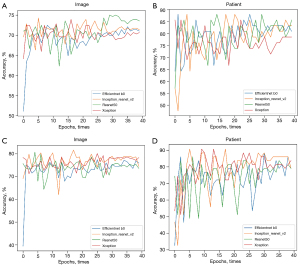
We added a confusion matrix to further evaluate whether the models could reliably classify objects and their performance in each category. As shown in Figure 8, the sensitivity of the 4 networks was slightly lower but the specificity was significantly higher when using each patient, rather than single images, as units.
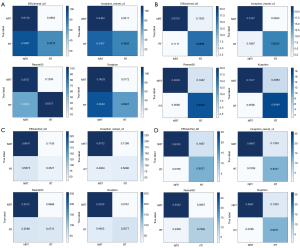
Grad-Cams clarifies how a network captures image features for prediction and removes doubts on whether the network is correct in its learning direction (Figure 9). Yellow areas shown in Grad-Cams have the strongest correlation with the classification. The Xception network was used as an example.
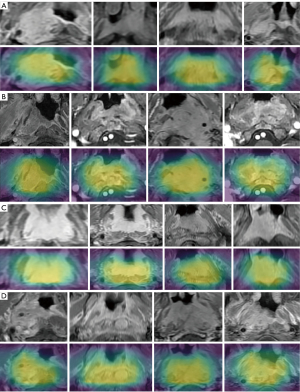
Comparison of model recommendations and physician decision
We statistically analyzed physician decisions and model recommendations for 85 patients in the test group, which revealed a total of 11 different situations (Table 3). We counted the correct and incorrect cases of physician decisions and model recommendations and removed the cases where the physician or the model could not be judged. Physician decision was correct in 39 cases, wrong in 26 cases, and unable to be judged right or wrong in 20 cases, resulting in a 60% “correct” rate of physician decisions. The model recommendation was correct in 58 cases, wrong in 11 cases, and could not be judged in 16 cases, resulting in an 84.06% “correct” rate of model recommendations, which was higher than that of the physician decisions (P=0.002).
Table 3
Situation | Model prediction | Model recommendation (predicted effect) | Physician decisions (actual effect) | Cases | |
---|---|---|---|---|---|
CCRT model | IC + CCRT model | ||||
1 | Residual tumor | Non-residual tumor | IC + CCRT (non-residual tumor) | IC + CCRT (non-residual tumor) | 9 |
2 | Non-residual tumor | Non-residual tumor | CCRT (non-residual tumor) | IC + CCRT (non-residual tumor) | 17 |
3 | Non-residual tumor | Residual tumor | CCRT (non-residual tumor) | IC + CCRT (non-residual tumor) | 2 |
4 | Residual tumor | Residual tumor | IBT (residual tumor) | IC + CCRT (non-residual tumor) | 4 |
5 | Residual tumor | Residual tumor | IBT (residual tumor) | IC + CCRT (residual tumor) | 11 |
6 | Non-residual tumor | Non-residual tumor | CCRT (non-residual tumor) | CCRT (non-residual tumor) | 21 |
7 | Non-residual tumor | Residual tumor | CCRT (non-residual tumor) | CCRT (non-residual tumor) | 2 |
8 | Residual tumor | Residual tumor | IBT (residual tumor) | CCRT (non-residual tumor) | 1 |
9 | Non-residual tumor | Non-residual tumor | CCRT (non- residual tumor) | CCRT (residual tumor) | 4 |
10 | Residual tumor | Non-residual tumor | IC + CCRT (non-residual tumor) | CCRT (residual tumor) | 9 |
11 | Residual tumor | Residual tumor | IBT (residual tumor) | CCRT (residual tumor) | 5 |
Situations 1, 6, 7: both the physician and the model made correct decisions; 2, 10: the model made the correct recommendation and the physician’s decision was wrong; 3, 4, 8: the physician made the correct decision and the model recommendation was wrong; 5, 11: unable to be determined; 9: the model recommendation was wrong and physician decision was unable to be determined. CCRT, concurrent chemoradiotherapy; IC, induction chemotherapy; IBT, individual-based treatment.
Discussion
Residual tumor has a very important impact on the prognosis of NPC patients. Xu et al. assessed the prediction of residual tumor based on a nomogram to facilitate high-risk patients to receive more intensive treatment and improve the prognosis (21). However, there may be differences in the efficacy of different treatment modalities in different patients. As these previous studies did not explore the status of residual tumor in patients who received different treatment regimens, they could not provide constructive suggestions for clinical treatment selection. Moreover, clinicians cannot identify high-risk patients because of the failure of one treatment. At present, IC + CCRT and CCRT are the preferred regimens for patients with locally advanced NPC, and although IC + CCRT has a better prognosis than CCRT alone (7,10), some patients with advanced-stage disease who receive CCRT can achieve non-residual tumor without additional IC. The goal of clinical treatment is to simplify the treatment plan as far as possible on the premise that patients can achieve non-residual tumor to reduce the adverse reactions caused by additional chemoradiotherapy. However, we could not effectively judge the effects of the 2 treatment methods beforehand. Therefore, we introduced semantic segmentation and classification networks to learn the pre-treatment MR features of patients with NPC who achieved non-residual tumor and residual tumor after CCRT or IC + CCRT to enable accurate prediction of residual tumor based on preoperative MR images and to form a model recommendation scheme according to the prediction results of the CCRT and IC + CCRT models. Although the overall prognosis of patients who receive IC + CCRT is better than that of those who receive the CCRT regimen, we found that statistically, about 2/3 of patients who could achieve non-residual tumor after IC + CCRT were predicted to be non-residual tumor by the CCRT model, whereas 1/2 of patients who could not achieve non-residual tumor after CCRT were predicted to be non-residual tumor by the IC + CCRT model. This suggests that many patients who could have achieved non-residual tumor with CCRT have instead received additional IC because of the inability to predict the prognosis of patients. At the same time, some patients could have achieved non-residual tumor with IC + CCRT but chose the CCRT regimen, and likely failed to achieve the best prognosis. In addition, the predicted curative effect of some patients in the test cohort was residual tumor regardless of whether they were input into the CCRT model or IC + CCRT model, suggesting that these patients could not achieve non-residual tumor with the 2 conventional regimens and required a stricter intensive treatment strategy. It can be seen that predicting the therapeutic effect of patients has a crucial impact on avoiding excessive treatment. Therefore, we constructed the CCRT and IC + CCRT models based on DL to predict the efficacy of the 2 treatment plans in advance and formed a model recommendation plan according to the treatment regimen and the corresponding efficacy to assist clinicians in selecting an appropriate regimen before treatment.
To further evaluate the model-recommended regimen, in addition to comparing the treatment plan and outcome with the actual physician decision, it is also necessary to determine whether the model prediction is correct. For instance, in situation 2, the selected IC + CCRT regimen obtained non-residual tumor as the model correctly predicted, and the model-recommended plan was better than the physician plan, indicating that the model was correct. Conversely, in situation 3, although the plan recommended by the model was better than the physician-determined plan, the model prediction was not consistent with the actual situation, and was judged as a model error. The obvious difference between the model- and physician-selected regimes suggests that the application of the model-recommended scheme in clinical practice will effectively improve the prognosis of patients, reduce the application of excessive treatment for some patients, and promote precise treatment in patients with NPC. The low accuracy of physician decision-making is mainly due to the inability to effectively predict the treatment efficacy for patients, and the accuracy of the model scheme mainly depends on the accuracy of the classification model prediction. Although the model prediction results in accordance with the actual situation is the first condition to judge the correctness of the model scheme, each patient chose only 1 treatment scheme, it is not clear whether the model prediction of the other scheme is correct. Further, although the prediction accuracy of our model is high, prediction errors may still exist for some patients. Therefore, follow-up studies with large sample sizes are still needed to improve the accuracy of the model, minimize the risk of prediction errors, and achieve more accurate model recommendation schemes.
We introduced a semantic segmentation model that can automatically segment tumor regions from complex nasopharyngeal and neck MR images for classification model learning. Different from classical cat and dog classification learning, pixels representing classification features can appear anywhere in the image, whereas disease is usually distributed in the corresponding anatomical area. Delineating the corresponding area in advance can eliminate surrounding interference factors and prevent failure caused by a lack of prior anatomical knowledge. Our previous study confirmed that the anatomic partition-based training method can effectively improve model performance when the dataset is reduced (22). Compared with tedious manual segmentation, the semantic segmentation model is more convenient for automatic image segmentation and more suitable for clinical practice. In addition, we used rectangular segmentation as the segmentation method to retain the surrounding structure of the tumor, which was not only more consistent with the real situation but, as shown by previous studies, the surrounding area of the tumor provided information on the prognosis, metastasis status, and other situations (23,24).
Although previous studies on applying DL in medicine have mostly reported on models trained using a single-image unit, defining the patient as a unit is obviously more consistent with clinical thinking and medical images (25,26). Medical images are unique, and not all image slices of patients with certain classification characteristics contain classification information. On the contrary, the classification features of patients often exist in only a few slices. We previously confirmed that when a model is trained using a single image as a unit, the images of other slices promote erroneous learning for the model (27). Moreover, tumors are heterogeneous, and the prognosis or metastasis risk of patients cannot be attributed to each tumor slice. Therefore, a learning method that involves labeling each slice is not reasonable for tumor images, and the purpose of the training model is to classify patients. The classification results of a single image cannot represent the classification of patients, and the classification results of multiple images of the same patient are likely to be different, which affects the final classification. In addition to traditional single image labeling, we labeled each patient to achieve a model trained using each patient as a unit. The results showed that the model trained using each patient as a unit had better performance than the model trained using individual images as a unit, which not only confirms that not all tumor layers of a patient have information about the patient's prognosis but also demonstrates the correctness and reliability of considering each patient as a unit.
Previous studies on artificial intelligence in the field of medicine have generally predicted the prognosis of patients and the risk of distant metastasis, and the outcomes of patients with different treatment methods have not been explored. However, there are differences in the prognosis of patients with different treatment methods. This study is the first to explore residual tumor in different patients who received 1 of the 2 conventional clinical treatment methods, which not only provides valuable advice for the selection of a clinical treatment plan but also lays a foundation for subsequent research on the application of artificial intelligence in the field of precision medical treatment.
Limitations
First, after strict inclusion and exclusion screening, we included only 424 patients who received either the CCRT or IC + CCRT regimen. Although the number of patients was balanced between the 2 treatment regimens, the sample size was still small. For this reason, we did not classify patients who received paclitaxel plus cisplatin versus gemcitabine plus cisplatin during IC, nor did we classify patients who received cisplatin versus nedaplatin during CCRT. Moreover, although there was statistical significance between the accuracy of model recommendation and doctors' decisions in the test cohort, the number of the test dataset was small. Second, we did not perform external validation to verify the generalizability of the model. Finally, the patient’s age, Epstein-Barr virus DNA level, and other factors were not included in the learning process. Since these factors could affect the accuracy of the classification model, we will investigate them in future studies.
Conclusions
Our results show that the combination of a semantic segmentation and classification network can effectively predict residual tumor in NPC after radiotherapy. The model recommendation based on the prediction results of CCRT and IC + CCRT is superior to a physician’s determination, and can protect certain patients from receiving additional IC, while also improving the prognosis of patients.
Acknowledgments
We thank all those who contributed to this research.
Funding: This study was supported by the General Project of National Natural Science Foundation of China (Nos. 81970860 and 81870705), Project (No. 81870705) funded the study before its closure in December 2022.
Footnote
Reporting Checklist: The authors have completed the TRIPOD reporting checklist. Available at https://qims.amegroups.com/article/view/10.21037/qims-22-1226/rc
Conflicts of Interest: All authors have completed the ICMJE uniform disclosure form (available at https://qims.amegroups.com/article/view/10.21037/qims-22-1226/coif). The authors have no conflicts of interest to declare.
Ethical Statement: The authors are accountable for all aspects of the work in ensuring that questions related to the accuracy or integrity of any part of the work are appropriately investigated and resolved. The study was conducted in accordance with the Declaration of Helsinki (as revised in 2013). The study protocol was approved by the Institutional Ethics Committee of the Renmin Hospital of Wuhan University, and the requirement for informed consent was waived due to the retrospective nature of the study.
Open Access Statement: This is an Open Access article distributed in accordance with the Creative Commons Attribution-NonCommercial-NoDerivs 4.0 International License (CC BY-NC-ND 4.0), which permits the non-commercial replication and distribution of the article with the strict proviso that no changes or edits are made and the original work is properly cited (including links to both the formal publication through the relevant DOI and the license). See: https://creativecommons.org/licenses/by-nc-nd/4.0/.
References
- Bray F, Ferlay J, Soerjomataram I, Siegel RL, Torre LA, Jemal A. Global cancer statistics 2018: GLOBOCAN estimates of incidence and mortality worldwide for 36 cancers in 185 countries. CA Cancer J Clin 2018;68:394-424. [Crossref] [PubMed]
- Tang LL, Chen YP, Mao YP, Wang ZX, Guo R, Chen L, Tian L, Lin AH, Li L, Sun Y, Ma J. Validation of the 8th Edition of the UICC/AJCC Staging System for Nasopharyngeal Carcinoma From Endemic Areas in the Intensity-Modulated Radiotherapy Era. J Natl Compr Canc Netw 2017;15:913-9.
- Wang M, Xu Y, Chen X, Chen H, Gong H, Chen S. Prognostic significance of residual or recurrent lymph nodes in the neck for patients with nasopharyngeal carcinoma after radiotherapy. J Cancer Res Ther 2016;12:909-14. [Crossref] [PubMed]
- Lv JW, Zhou GQ, Li JX, Tang LL, Mao YP, Lin AH, Ma J, Sun Y. Magnetic Resonance Imaging-Detected Tumor Residue after Intensity-Modulated Radiation Therapy and its Association with Post-Radiation Plasma Epstein-Barr Virus Deoxyribonucleic Acid in Nasopharyngeal Carcinoma. J Cancer 2017;8:861-9. [Crossref] [PubMed]
- He Y, Zhou Q, Shen L, Zhao Y, Lei M, Wei R, Shen L, Cao S. A retrospective study of the prognostic value of MRI-derived residual tumors at the end of intensity-modulated radiotherapy in 358 patients with locally-advanced nasopharyngeal carcinoma. Radiat Oncol 2015;10:89. [Crossref] [PubMed]
- Bossi P, Chan AT, Licitra L, Trama A, Orlandi E, Hui EP, Halámková J, Mattheis S, Baujat B, Hardillo J, Smeele L, van Herpen C, Castro A, Machiels JPESMO Guidelines Committee. Nasopharyngeal carcinoma: ESMO-EURACAN Clinical Practice Guidelines for diagnosis, treatment and follow-up Ann Oncol 2021;32:452-65. [Crossref] [PubMed]
- Yang Q, Cao SM, Guo L, Hua YJ, Huang PY, Zhang XL, et al. Induction chemotherapy followed by concurrent chemoradiotherapy versus concurrent chemoradiotherapy alone in locoregionally advanced nasopharyngeal carcinoma: long-term results of a phase III multicentre randomised controlled trial. Eur J Cancer 2019;119:87-96. [Crossref] [PubMed]
- Sun Y, Li WF, Chen NY, Zhang N, Hu GQ, Xie FY, et al. Induction chemotherapy plus concurrent chemoradiotherapy versus concurrent chemoradiotherapy alone in locoregionally advanced nasopharyngeal carcinoma: a phase 3, multicentre, randomised controlled trial. Lancet Oncol 2016;17:1509-20. [Crossref] [PubMed]
- Li WF, Chen NY, Zhang N, Hu GQ, Xie FY, Sun Y, et al. Concurrent chemoradiotherapy with/without induction chemotherapy in locoregionally advanced nasopharyngeal carcinoma: Long-term results of phase 3 randomized controlled trial. Int J Cancer 2019;145:295-305. [Crossref] [PubMed]
- Bongiovanni A, Vagheggini A, Fausti V, Mercatali L, Calpona S, Di Menna G, Miserocchi G, Ibrahim T. Induction chemotherapy plus concomitant chemoradiotherapy in nasopharyngeal carcinoma: An updated network meta-analysis. Crit Rev Oncol Hematol 2021;160:103244. [Crossref] [PubMed]
- Zhang Y, Chen L, Hu GQ, Zhang N, Zhu XD, Yang KY, et al. Gemcitabine and Cisplatin Induction Chemotherapy in Nasopharyngeal Carcinoma. N Engl J Med 2019;381:1124-35. [Crossref] [PubMed]
- Liu SL, Sun XS, Yan JJ, Chen QY, Lin HX, Wen YF, Guo SS, Liu LT, Xie HJ, Tang QN, Liang YJ, Li XY, Lin C, Du YY, Yang ZC, Xiao BB, Yang JH, Tang LQ, Guo L, Mai HQ. Optimal cumulative cisplatin dose in nasopharyngeal carcinoma patients based on induction chemotherapy response. Radiother Oncol 2019;137:83-94. [Crossref] [PubMed]
- Peng H, Chen L, Zhang Y, Li WF, Mao YP, Liu X, Zhang F, Guo R, Liu LZ, Tian L, Lin AH, Sun Y, Ma J. The Tumour Response to Induction Chemotherapy has Prognostic Value for Long-Term Survival Outcomes after Intensity-Modulated Radiation Therapy in Nasopharyngeal Carcinoma. Sci Rep 2016;6:24835. [Crossref] [PubMed]
- Zhao L, Gong J, Xi Y, Xu M, Li C, Kang X, Yin Y, Qin W, Yin H, Shi M. MRI-based radiomics nomogram may predict the response to induction chemotherapy and survival in locally advanced nasopharyngeal carcinoma. Eur Radiol 2020;30:537-46. [Crossref] [PubMed]
- Ng SH, Chan SC, Yen TC, Liao CT, Chang JT, Ko SF, Wang HM, Lin CY, Chang KP, Lin YC. Comprehensive imaging of residual/ recurrent nasopharyngeal carcinoma using whole-body MRI at 3 T compared with FDG-PET-CT. Eur Radiol 2010;20:2229-40. [Crossref] [PubMed]
- Ronneberger O, Fischer P, Brox T. U-Net: Convolutional Networks for Biomedical Image Segmentation. In: Navab N, Hornegger J, Wells W, Frangi A. (eds) Medical Image Computing and Computer-Assisted Intervention – MICCAI 2015. MICCAI 2015. Lecture Notes in Computer Science, vol 9351. Springer, Cham.
-
.Chen LC Papandreou G Schroff F Adam H Rethinking Atrous Convolution for Semantic Image Segmentation 2017 :arXiv:1706.05587. - Tan MX, Le QV. EfficientNet: Rethinking Model Scaling for Convolutional Neural Networks. International Conference on Machine Learning, PMLR 2019;97:6105-14.
- Szegedy C, Ioffe S, Vanhoucke V, Alemi AA. Inception-v4, Inception-ResNet and the Impact of Residual Connections on Learning. Thirty-First Aaai Conference on Artificial Intelligence 2017. p. 4278-84.
- He KM, Zhang XY, Ren SQ, Sun J. Deep Residual Learning for Image Recognition. 2016 IEEE Conference on Computer Vision And Pattern Recognition (CVPR) 2016. p. 770-8.
- Xu M, Liu C, Mi JL, Wang RS. A Nomogram for the Prognosis of Nasopharyngeal Carcinoma with MR Imaging-Detected Tumor Residue at the End of Intensity-Modulated Radiotherapy. Cancer Manag Res 2020;12:3835-44. [Crossref] [PubMed]
- Li S, Hua HL, Li F, Kong YG, Zhu ZL, Li SL, Chen XX, Deng YQ, Tao ZZ. Anatomical Partition-Based Deep Learning: An Automatic Nasopharyngeal MRI Recognition Scheme. J Magn Reson Imaging 2022;56:1220-9. [Crossref] [PubMed]
- Wu Q, Wang S, Zhang S, Wang M, Ding Y, Fang J, Wu Q, Qian W, Liu Z, Sun K, Jin Y, Ma H, Tian J. Development of a Deep Learning Model to Identify Lymph Node Metastasis on Magnetic Resonance Imaging in Patients With Cervical Cancer. JAMA Netw Open 2020;3:e2011625. [Crossref] [PubMed]
- Wu X, Dong D, Zhang L, Fang M, Zhu Y, He B, Ye Z, Zhang M, Zhang S, Tian J. Exploring the predictive value of additional peritumoral regions based on deep learning and radiomics: A multicenter study. Med Phys 2021;48:2374-85. [Crossref] [PubMed]
- Zhu M, Pi Y, Jiang Z, Wu Y, Bu H, Bao J, Chen Y, Zhao L, Peng Y. Application of deep learning to identify ductal carcinoma in situ and microinvasion of the breast using ultrasound imaging. Quant Imaging Med Surg 2022;12:4633-46. [Crossref] [PubMed]
- Li J, Zhou Y, Wang P, Zhao H, Wang X, Tang N, Luan K. Deep transfer learning based on magnetic resonance imaging can improve the diagnosis of lymph node metastasis in patients with rectal cancer. Quant Imaging Med Surg 2021;11:2477-85. [Crossref] [PubMed]
- Hua HL, Li S, Xu Y, Chen SM, Kong YG, Yang R, Deng YQ, Tao ZZ. Differentiation of eosinophilic and non-eosinophilic chronic rhinosinusitis on preoperative computed tomography using deep learning. Clin Otolaryngol 2023;48:330-8. [Crossref] [PubMed]