Development and validation of a transformer-based CAD model for improving the consistency of BI-RADS category 3–5 nodule classification among radiologists: a multiple center study
Introduction
The incidence of breast cancer is the highest among all malignancies in women, and its early diagnosis and treatment significantly reduces mortality rates (1,2). Among several commonly used breast examination techniques, ultrasonography is the most convenient and most economical modality with no radiation and relatively low cost. However, the quality of ultrasonography directly depends on operator expertise and experience, especially as it relates to scanning techniques, ability to detect lesions, and description and interpretation of images (3). The Breast Imaging Reporting and Data Systems on ultrasonography (BI-RADS) represents an attempt to not only normalize and standardize the terminology used to describe a series of appearances in ultrasound images but also to classify breast nodules from category 1 through category 6 depending on the probability of malignancy (4). However, the probability for category 4 nodules varies widely (2–95%), and specific classification criteria for subcategories 4a, 4b, and 4c lack clear definitions. Furthermore, there is a lack of clear criteria of the classification in distinguishing categories between 3 and 4a and between 4c and 5 nodules (5,6). Subsequently, the BI-RADS classifications of categories 3–5 nodules are significantly different across various hospitals and radiologists (7). As a result, a given breast nodule may be over- or undertreated in response to a diagnosis. For example, a misclassification of benign nodules into category 4 or above increases psychological burden and medical expenses for a patient, whereas a misclassification of malignant nodules into category 3 can cause life-threatening delays in treatment.
Computer-aided diagnosis (CAD) models can bypass conventional subjective diagnoses by humans. In recent years, the expanded availability of breast imaging datasets has facilitated end-to-end deep learning, thereby achieving objective diagnosis of breast nodules. Although CAD models can be a highly effective aid to assisting radiologists in diagnosing diseases, their performance is closely related to the size of the training set, as larger sets composed of higher quality images may produce better diagnostic outcomes. Even though acquiring annotated images marked by experienced radiologists can be difficult, open databases have allowed the application of machine learning in a variety of fields. For example, convolutional neural networks (CNNs) have been satisfactorily applied to the segmentation of ultrasound images (8,9), the diagnosis of benign and malignant breast nodules (6,9-11), and BI-RADS classification (12,13). However, the local receptive field of CNNs in convolution operation limits the capturing of long-range pixel relationships. Furthermore, the convolutional filters have stationary weights that are not adapted for the given input image content at inference time (14). According to previous research, the self-attention-based mechanism of a transformer can not only outperform conventional CNNs for visual classification but also has relatively higher shape bias and is largely more consistent with human errors (15). Transformer was the first transduction model to rely entirely on self-attention to compute representations of its input and output. It was originally developed for machine learning in natural language processing (16) but has since been applied to medical imaging research (14,17-20). Gheflati and Rivaz compared the performance of vision transformer (ViT) against CNNs and showed that the ViT models had comparable or even superior efficacy to that of CNNs in the classification of breast ultrasound images (21). It has also been suggested that transformers focus more on shape recognition and exhibit higher computational efficacy and scalability than do CNNs, which are more inclined to texture recognition (22,23). The aim of this study was to develop and validate a transformer-based CAD model to be used in the classification of BI-RADS 3–5 nodules and thereby in the provision of classification references to radiologists in an attempt to improve their diagnostic consistency and accuracy. We present the following article in accordance with the TRIPOD reporting checklist (available at https://qims.amegroups.com/article/view/10.21037/qims-22-1091/rc).
Methods
A total of 21,332 images of 5,057 breast nodules were collected from 3,978 female patients from December 2006 to December 2019. The age of the patients ranged from 12 to 95 years, with a mean age of 47.81±14.55 years. All nodules were surgically removed from the patients, and pathological results were made available from 20 clinical centers in China, including Beijing Tongren Hospital affiliated with Capital Medical University, the Second Affiliated Hospital of Chongqing Medical University, the First Affiliated Hospital of Xi’an Jiaotong University, Southwest Hospital of Army Medical University, the Second Affiliated Hospital of Harbin Medical University, the First Hospital of Shanxi Medical University, Beijing Tiantan Hospital of Capital Medical University, Peking University First Hospital, Hunan Cancer Hospital, the Second Affiliated Hospital of Xi’an Jiaotong University, the Cancer Hospital at the Chinese Academy of Medical Sciences, the Third Affiliated Hospital of Guangxi Medical University, the First Affiliated Hospital of Hebei North University, Sichuan Provincial People’s Hospital, the First Affiliated Hospital of Zhengzhou University, the China-Japan Union Hospital of Jilin University, Qilu Hospital of Shandong University, the Second Xiangya Hospital of Central South University, the Second Hospital of Lanzhou University, and the First Affiliated Hospital of Nanchang University. We did not calculate the formal data size of this study, and all available data were used to maximize the power and generalizability of the results. This retrospective clinical study only involved the collection of age data, breast nodule images, imaging system models, and pathological results for patients. It did not interfere with individual treatment plans. The study was conducted in accordance with the Declaration of Helsinki (as revised in 2013). The study was approved by Ethics Committee of Beijing Tongren Hospital, Capital Medial University (No. TRECKY2019-139), and the requirement for individual consent for this retrospective analysis was waived.
The image inclusion criteria were as follows: (I) produced using a high-frequency probe (≥12 MHz), (II) containing only 1 nodule, and (III) exhibiting nodules with identifiable boundaries. The image exclusion criteria were as follows: (I) no nodules, (II) clear cysts, (III) more than 1 nodule, (IV) nodules too large to display a complete outline, and (V) poor quality or an unclear nodule margin. The open-source “cornerstonejs” and “cornerstone Tools” JavaScript frameworks were used to establish a breast nodule image annotation platform. The BI-RADS classification and labeling of each lesion on all images were performed independently by 5 radiologists with 8 (DR1), 11 (DR2), 12 (DR3), 15 (DR4), and 19 (DR5) years of experience in breast ultrasonography. Another senior radiologist with 21 years of experience served as the “referee” for final classification. Consistency was achieved to the degree possible as the 6 radiologists jointly discussed and formulated specific criteria for the classification of BI-RADS 3–5 nodules based on their experience and recent research (4,5,24,25) (Table 1).
Table 1
Category 3 |
Solid nodules with clear and sharp margins in an oval shape, parallel to the skin |
Isolated complex cysts or clustered microcysts |
Category 4 |
Morphology |
Round in shape |
Irregular in shape* |
Nodular orientation |
Nodules not parallel to the skin. The anteroposterior diameter is greater than or equal to the transverse diameter* |
Nonsmooth margins |
Blurred—no clear margin between the mass and the surrounding tissues |
Angled—part of or all the margin at an acute angle |
Tiny lobes—with small bumps on the margin, crenated |
Burr-like—protruding sharp needles on the nodular margins* |
Internal echo |
Uneven echo, with anechoic zones or microcalcifications |
Posterior echo |
Attenuation* |
Changes in the surrounding tissue |
Disordered structure of the surrounding tissue, with normal anatomical layers being destroyed, thickening, or a rigid Cooper ligament |
Catheter changes (unusual pipe diameter or branch-like changes) |
>2-mm skin thickening or skin receding, sunken surface, or unclear margins showing tautly |
Edema, enhanced echo of the surrounding tissues, or tissue thickening |
Category 4a |
Satisfying 1 of the above requirements |
Category 4b |
Satisfying 2 of the above requirements or any 1 item marked with “*” |
Category 4c |
Satisfying 3 of the above requirements or any 2 items marked with “*” |
Category 5 |
Satisfying 4 or more requirements |
BI-RADS, Breast Imaging Reporting and Data Systems.
The abovementioned 5 radiologists responsible for annotating and conducting BI-RADS classification based on the specific criteria were blinded to patient ages, clinical symptoms, pathological results, and one other’s classification and final diagnosis. A rectangular box on the labeling platform was used to mark the nodule margin prior to classification and label selection (Figure 1). The following 2 strategies were adopted when the BI-RADS results of these 5 radiologists were inconsistent. (I) Images with a 4:1 consensus were classified according to the majority opinion. (II) All other disagreements were finalized by the referee, who was authorized to decide the final classification according to both the specific criteria and the pathological results so as to provide a more accurate database for the CAD model. All images were required to be annotated and refereed without being missed. A total of 21,332 images were randomly divided into development and test sets in a 7:3 ratio. The development set was subsequently and randomly subdivided into training and validation sets in an 8:2 ratio. The images for each set were not repeated to use in the other set. The detailed process of image selection and grouping is shown in Figure 2.
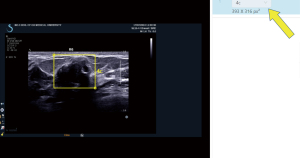
A CAD model was constructed by modifying the hierarchical ViT architecture for the localization of breast nodules and BI-RADS classification (26). We introduced a multiresolution feature extraction process to extract the lesion features and classify them through the attention mechanism. The model included 4 sets of block 1 and 1 set of block 2 networks used for feature extraction from input images at different resolutions. In the first 4 transformer structures, each set of blocks included a window-based multihead self-attention mechanism for feature extraction. The last group was composed of 2 transformer structures, the first of which was used to encode the input feature vector, and the second was used to obtain BI-RADS categories (e.g., 3, 4a, 4b, 4c, and 5) by decoding encoded feature vectors generated in the previous step. The input images 224×224×3 pixels in size were equally divided into 56×56 image blocks 4×4 in size. The images were input to the first block 1 set for feature extraction, producing 56×56×96 feature maps. The first output was then divided into 28×28 image blocks 8×8 pixels in size, which were input to the second block 1 set for feature extraction and generation of 28×28×192 feature maps. Similarly, the third and fourth block 1 sets produced 14×14×384 and 7×7×768 feature maps, respectively. The 7×7×768 feature maps were subsequently input to block 2 for BI-RADS category classification (e.g., 3, 4a, 4b, 4c, and 5). The input feature maps for block 1 sets 1–4 were first divided into image blocks of a specified size. The image blocks were then shifted, which modified feature information distributions for blocks of varying sizes, allowing the attention to be focused on a wider area. In block 2, position coding information was first added to the coded image block, which was then converted into a 1-dimensional vector and input to another transformer. BI-RADS categories were used as inputs to query detected nodular areas in each category. Final output results included the detected nodular areas and BI-RADS categories to which each nodule belonged (Figure 3). The accuracy of the entire calculation was determined as follows: Accuracyall = Accuracydetection × Accuracyclassification. In addition, the selection of nodules was based on the intersection over a union greater than 80% in the detection step between the predicted nodule and the ground truth. As mentioned earlier, the transformer structure was used to build a neural network, by means of which the breast nodules were detected; their features on ultrasound images were extracted; and their grades were classified. Considering the diversity of different sizes and distributions of breast nodules, along with almost the entire ultrasound image occupied by some lesions, we adopted the Swin transformer structure to extract ultrasound image features, which could merge multiple ultrasound image blocks of different scales and expand the neural network’s perception range. The use of a multiscale image block structure also allowed us to learn the features of different nodule regions, such as within nodules and on their boundaries, without directly segmenting out the nodule regions but through this network, and a weighted regression was performed to obtain nodule region and grade information. In the optimization process of the network, multiple image enhancement methods, such as horizontal flipping, mixup, and cutout, were used to enhance data in the training of the model, and the AdamW optimizer was applied to optimize the neural network, with an initial learning rate set to 0.001. The cosine annealing was used to adjust the learning rate, with a corresponding decay rate of 0.05. Our model codes are available online (https://github.com/oliverjiht/DVBCN).
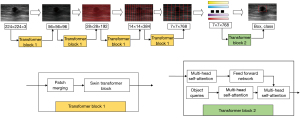
After the CAD model was established, a total of 500 breast nodule images with consistent diagnostic results between both the model and the radiologists, which consisted of 100 images randomly selected from each of the BI-RADS 3–5 categories, were uniformly composed into a sampling test set. Then, the abovementioned 5 radiologists reclassified the sampling test set by referencing BI-RADS classification results provided by the CAD model. Changes in diagnostic sensitivity (SEN), specificity (SPE), accuracy (ACC), classification consistency, and categorical adjustments for various nodule categories were observed.
The SPSS 28.0 software (IBM Corp., Armonk, NY, USA) and Python 3.8 (Python Software Foundation, Wilmington, DE, USA) were used for statistical analysis in this study. The classification results of the referee were adopted as the final diagnostic standard. The SEN, SPE, and ACC of CAD model for BI-RADS classification of categories 3–5 were calculated based on BI-RADS classification results decided by the referee radiologist. Taking 4a as a cutoff value of the benign and malignant distinction, a receiver operating characteristic (ROC) curve with a corresponding area under the curve (AUC) was calculated; meanwhile, a calibration curve was drawn in order to evaluate the performance of the CAD model. The chi-squared test was used to test the differences in the SEN, SPE, and ACC of the BI-RADS classification on the sampling test set before and after the CAD model category was referenced by each of the 5 radiologists. Kappa coefficients were compared before and after reference to CAD results by these radiologists to identify any improvements in the consistency among them. All statistical methods were considered significant at a P value below 0.05.
Results
Datasets
Of the 3,978 patients with breast nodules, 3,317 had 1 and the remaining 661 had 2 or more lesions. Of the 5,057 breast nodules, 2,390 were benign and 2,667 were malignant comprising 10,041 and 11,291 images, respectively (Table 2). There were 1–25 pieces of images in each nodule, with an average of 4.22±3.37. The maximum diameter of the nodule ranged from 0.30 to 7.74 cm, with an average of 2.05±1.20 cm. A total of 34 types of ultrasound machines were used in the study (Table 3, Figure 4). The breast nodules of categories 3–5 were distributed among the training, validation, and test sets according to the Python random function (Table 4). The distribution of variables between development and test sets is displayed in Table 5.
Table 2
Malignant/benign | Pathological result | Quantity |
---|---|---|
Malignant | Nonspecific invasive carcinoma | 8,690 |
Ductal carcinoma in situ | 1,072 | |
Mucinous carcinoma | 390 | |
Invasive lobular carcinoma | 387 | |
Intraductal papilloma | 179 | |
Medullary carcinoma | 146 | |
Other malignancy | 427 | |
Benign | Fibroadenomas | 5,434 |
Adenopathy | 2,491 | |
Intraductal papillary carcinoma | 999 | |
Breast abscess | 265 | |
Granulomatous inflammation | 328 | |
Other benign nodules | 524 |
Table 3
Characteristic | Benign nodules | Malignant nodules | P value |
---|---|---|---|
Nodules | 2,390 | 2,667 | |
Images | 10,041 | 11,291 | |
Age (years), mean ± standard deviation | 40.93±12.93 | 53.92±13.10 | <0.05 |
Nodule size (cm), mean ± standard deviation | 1.83±1.23 | 2.25±1.13 | <0.05 |
Types of ultrasound machines | 24 | 33 | <0.05 |
Table 4
Dataset | 3 | 4a | 4b | 4c | 5 | Total |
---|---|---|---|---|---|---|
Train | 2,512 | 1,370 | 1,759 | 4013 | 1,584 | 11,238 |
Val | 654 | 342 | 464 | 1,086 | 450 | 2,996 |
Test | 1,596 | 838 | 1,284 | 2,358 | 1,022 | 7,098 |
Total | 4,762 | 2,550 | 3,507 | 7,457 | 3,056 | 21,332 |
BI-RADS, Breast Imaging Reporting and Data Systems; Val, validation.
Table 5
Characteristics | Development set | Test set | P value |
---|---|---|---|
Benign images/malignant images | 6,684/7,550 | 3,357/3,741 | 0.642 |
Age (years), mean ± standard deviation | 48.01±14.50 | 47.27±14.62 | <0.05 |
Nodule size (cm), mean ± standard deviation | 2.06±1.21 | 2.04±1.18 | 0.265 |
Types of ultrasound machines | 34 | 33 | <0.05 |
CAD performance
The BI-RADS categories 3–5 classified by the CAD are shown in Figure 5. The SEN, SPE, and ACC of CAD for the various categories were 94.16% [95% confidence interval (CI): 0.929–0.952], 95.09% (95% CI: 0.945–0.956), and 94.89% (95% CI: 0.944–0.954), respectively, in category 3; 85.28% (95% CI: 0.827–0.875), 98.48% (95% CI: 0.982–0.988), and 96.90% (95% CI: 0.965–0.973), respectively, in category 4a; 87.04% (95% CI: 0.851–0.888), 97.38% (95% CI: 0.969–0.978), and 95.49% (95% CI: 0.950–0.960), respectively, in category 4b; 85.21% (95% CI: 0.838–0.865), 96.21% (95% CI: 0.956–0.967), and 92.28% (95% CI: 0.916–0.929), respectively, in category 4c, and 85.08% (95% CI: 0.825–0.873), 96.88% (95% CI: 0.964–0.973), and 95.45% (95% CI: 0.949–0.959), respectively, in category 5 (Table 6). Based on pathological results, the AUC of the CAD model was 0.924 (95% CI: 0.916–0.932; P<0.05) (Figure 6). The calibration curve showed that the predicted probability of CAD was a little higher than the actual probability (Figure 7). By means of the reference to the consequence of the CAD model, the average SEN (32.73–56.98%), SPE (82.46–89.26%), and ACC (72.41–82.65%) of the 5 radiologists who had independently read the ultrasound images of the nodule were markedly improved in the sampling test set (P<0.05) (Table 7).

Table 6
BI-RADS | SEN | 95% CI | SPE | 95% CI | ACC | 95% CI |
---|---|---|---|---|---|---|
3 | 94.16% | 0.929–0.952 | 95.09% | 0.945–0.956 | 94.89% | 0.944–0.954 |
4a | 85.28% | 0.827–0.875 | 98.48% | 0.982–0.988 | 96.90% | 0.965–0.973 |
4b | 87.04% | 0.851–0.888 | 97.38% | 0.969–0.978 | 95.49% | 0.950–0.960 |
4c | 85.21% | 0.838–0.865 | 96.21% | 0.956–0.967 | 92.28% | 0.916–0.929 |
5 | 85.08% | 0.825–0.873 | 96.88% | 0.964–0.973 | 95.45% | 0.949–0.959 |
CAD, computer-aided diagnosis; BI-RADS, Breast Imaging Reporting and Data Systems; SEN, sensitivity; CI, confidence interval; SPE, specificity; ACC, accuracy.
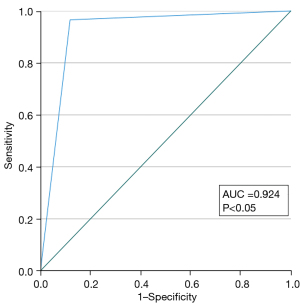
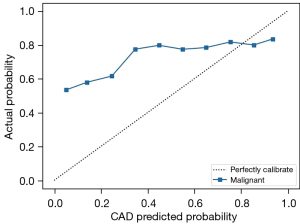
Table 7
Radiologist | BI-RADS | SEN | SPE | ACC | ||||||||
---|---|---|---|---|---|---|---|---|---|---|---|---|
Before | After | P value | Before | After | P value | Before | After | P value | ||||
DR1 | 3 | 51.00% | 62.00% | <0.05 | 91.00% | 91.00% | 0.441 | 83.00% | 85.20% | 0.341 | ||
4a | 27.72% | 59.41% | <0.05 | 81.45% | 90.48% | <0.05 | 70.60% | 84.20% | <0.05 | |||
4b | 24.00% | 53.00% | <0.05 | 88.50% | 88.25% | <0.05 | 73.20% | 81.20% | <0.05 | |||
4c | 43.43% | 66.67% | <0.05 | 70.32% | 88.53% | <0.05 | 65.00% | 84.20% | <0.05 | |||
5 | 13.00% | 57.00% | <0.05 | 86.50% | 91.25% | <0.05 | 71.80% | 84.40% | <0.05 | |||
DR2 | 3 | 58.00% | 74.00% | <0.05 | 67.75% | 86.25% | 1.000 | 65.80% | 83.80% | <0.05 | ||
4a | 31.68% | 58.42% | <0.05 | 86.47% | 90.98% | <0.05 | 75.40% | 84.40% | <0.05 | |||
4b | 23.00% | 47.00% | <0.05 | 91.50% | 91.25% | 0.520 | 77.80% | 82.40% | 0.068 | |||
4c | 61.62% | 74.75% | 0.481 | 60.10% | 95.01% | 0.393 | 60.40% | 91.00% | <0.05 | |||
5 | 6.00% | 72.00% | <0.05 | 93.25% | 93.00% | <0.05 | 75.80% | 88.80% | <0.05 | |||
DR3 | 3 | 71.00% | 67.00% | <0.05 | 81.75% | 80.25% | 1.000 | 79.60% | 77.60% | 0.441 | ||
4a | 18.81% | 46.53% | <0.05 | 93.98% | 85.71% | <0.05 | 78.80% | 77.80% | <0.05 | |||
4b | 13.00% | 36.00% | <0.05 | 90.00% | 89.50% | 0.262 | 74.60% | 78.80% | 0.116 | |||
4c | 32.32% | 21.21% | <0.05 | 75.31% | 96.26% | <0.05 | 66.80% | 81.40% | <0.05 | |||
5 | 36.00% | 73.00% | <0.05 | 76.75% | 84.25% | <0.05 | 68.60% | 82.00% | <0.05 | |||
DR4 | 3 | 1.00% | 74.00% | 1.000 | 100.00% | 93.00% | <0.05 | 80.20% | 89.20% | <0.05 | ||
4a | 16.83% | 79.21% | 0.682 | 87.97% | 92.23% | <0.05 | 73.75% | 90.75% | <0.05 | |||
4b | 39.00% | 58.00% | <0.05 | 73.75% | 90.75% | 0.301 | 66.80% | 84.20% | <0.05 | |||
4c | 66.67% | 75.00% | 0.725 | 54.61% | 91.00% | <0.05 | 57.00% | 87.80% | <0.05 | |||
5 | 11.00% | 58.00% | 0.064 | 92.25% | 94.50% | 1.000 | 76.00% | 87.20% | <0.05 | |||
DR5 | 3 | 43.00% | 29.00% | 0.761 | 93.25% | 93.50% | 1.000 | 83.20% | 80.60% | 0.552 | ||
4a | 23.76% | 33.66% | <0.05 | 85.46% | 86.97% | <0.05 | 73.00% | 76.20% | 0.245 | |||
4b | 32.00% | 35.00% | <0.05 | 82.25% | 78.00% | 1.000 | 72.20% | 69.40% | 0.330 | |||
4c | 39.39% | 59.60% | <0.05 | 78.30% | 81.05% | <0.05 | 70.60% | 76.80% | <0.05 | |||
5 | 35.00% | 55.00% | 0.132 | 79.00% | 88.50% | 0.690 | 70.20% | 81.80% | <0.05 |
SEN, sensitivity; SPE, specificity; ACC, accuracy; CAD, computer-aided diagnosis; BI-RADS, Breast Imaging Reporting and Data Systems; DR, doctor.
As a result, a total of 1,583 classifications were changed. Of them, 905 classifications were adjusted to a lower category with an average of 181 modifications by the 5 radiologists (Table 8), and 272 out of 905 were downgraded from category 4a or above to 3 with an average of 54.4 modifications among the radiologists, with 226 (83.09%) of these being pathologically benign. Of the 5 radiologists, 4 had 46 (16.91%) error downgrades on 25 images in 6 different pathological types (Table 9). The remaining 678 classifications were adjusted to a higher category with an average of 135.6 modifications in these radiologists, and 147 of these 678 were upgraded from category 3 to 4a or above with an average of 29.4 modifications by these radiologists; of these 147 classifications, 68 (46.26%) represented malignant pathological changes in nodules. In contrast, the other 917 classifications were not adjusted. Of these, 657 were still classified as category 4a or above and 411 (62.56%) were diagnosed as malignant lesions pathologically. In addition, 260 were category 3, of which 244 (93.8%) were found to be benign tumors in pathological examinations of nodules.
Table 8
Adjustments | DR1 | DR2 | DR3 | DR4 | DR5 | Total | Average |
---|---|---|---|---|---|---|---|
Downgraded | |||||||
Total | 153 | 164 | 145 | 273 | 170 | 905 | 181 |
From 4a or above to 3 | 42 | 54 | 40 | 101 | 35 | 272 | 54.4 |
Benign in pathology | 31 | 43 | 24 | 93 | 35 | 226 | 45.2 |
Accuracy (%) | 73.81 | 79.63 | 60.00 | 92.08 | 100 | 83.09 | |
Upgraded | |||||||
Total | 163 | 145 | 100 | 84 | 186 | 678 | 135.6 |
From 3 to ≥4a | 31 | 28 | 38 | 0 | 50 | 147 | 29.4 |
Malignant in pathology | 13 | 17 | 21 | 0 | 17 | 68 | 13.6 |
Accuracy (%) | 41.94 | 60.71 | 55.26 | 0.00 | 34.00 | 46.26 | |
Unadjusted | |||||||
Category 3 | 56 | 75 | 106 | 1 | 20 | 260 | 52 |
Benign in pathology | 55 | 74 | 92 | 1 | 20 | 242 | 48.4 |
Accuracy (%) | 98.21 | 98.67 | 86.79 | 100 | 100 | 93.08 | |
Category ≥4a | 128 | 116 | 147 | 142 | 144 | 657 | 131.4 |
Malignant in pathology | 68 | 74 | 102 | 92 | 75 | 411 | 82.2 |
Accuracy (%) | 53.13 | 63.79 | 69.39 | 64.79 | 60.48 | 62.56 |
CAD, computer-aided diagnosis; DR, doctor.
Table 9
Pathology type | Downgrade number | Image number | Image features |
---|---|---|---|
Carcinoma with neuroendocrine features | 4 | 1 | Cystic-solid and parallel to the skin |
Ductal carcinoma in situ | 16 | 7 | Parallel to skin and/or intraductal nodule |
Invasive carcinoma of no special type | 22 | 13 | Parallel to skin or small nodule with curvilinear calcification |
Invasive solid papillary carcinoma | 1 | 1 | Parallel to skin |
Mucinous carcinoma | 2 | 2 | Parallel to skin or cystic-solid |
Myeloid sarcoma | 1 | 1 | Parallel to skin |
Total | 46 | 25 |
Among the 21,332 classified images, the same annotation was exhibited by all 5 radiologists on 1,416 (6.64%), and a 4:1 inconsistency was seen on 5,620 (26.35%). A weighted k test through 10 paired comparisons in these 5 radiologists indicated that all the k values for all the images were less than 0.6 (0.33–0.57), indicating normal or moderate consistency, and 7 of 10 k values for the sampling test set were under 0.6 (0.30–0.62) indicating normal or moderate levels in consistency, with the remaining 3 k values being slightly higher than 0.6. After the radiologists referred to the categories of CAD model, almost all the k values for the sampling test set were increased to above 0.60 (0.54–0.87) because of up- or downgrade readjustments (Figure 8).
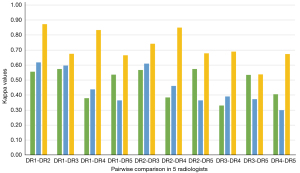
Discussion
This study focused on the difficult problem of classification of BI-RADS category 3–5 nodules. With a large number of cases in a multiple center study, a large dataset of patients with breast nodules was established and then used to construct a CAD model based on transformer in an attempt to improve the classification efficacy of the BI-RADS category 3–5 lesions and the consistency of the classification among radiologists.
BI-RADS defines and explains the image feature of breast nodules to describe them in a more unified standard across different radiologists. However, it does not set a clearly specific standard of the image features for every category of BI-RADS classification due likely to the great individual differences in evaluation of nodular categories of the different physicians. Such physician-to-physician discrepancies are the most common in category 3–5 breast nodules in affecting the diagnosis and treatment of the disease (27). In the first part of the study, 5 radiologists jointly agreed on the ultrasonic classification criteria for BI-RADS 3–5 nodules. However, the k values across 21,332 images reviewed by various radiologists were 0.57 at best, which is similar to the findings of Jales et al. and Berg et al. (27,28). This indicates that even if the BI-RADS classification standard jointly developed by doctors with extensive experience in breast diagnostic imaging is followed, the results of classification will still vary across different doctors due to individual differences in the recognition of specific features of the image. To reduce the difference, Berg et al. suggested that immediate feedback is helpful for correcting classifications, regardless of the radiologist experience, because when they provided feedback to correct the result in error, k values were found to increase from 0.53 to 0.59 (28). For this reason, our study employed another radiologist who was both experienced and had access to the pathological diagnosis after surgery to act as the referee to decide the results of the disputes among the 5 radiologists. There were 14,296 (67.01%) images in which the final result could not be determined by following the majority opinion at a ratio of 5:0 or 4:1. The referee radiologist finalized classification by referencing both the classification criteria of ultrasound images and the pathological results. Under a condition of the dual insurance of pathological results and classification imaging criteria, the obvious “bias” of labeling benign nodules as category 4b or above or malignant nodules as category 3 could be reduced, thus providing a more accurate dataset for CAD model construction and subsequently producing a model with higher ACC in the identification of category 3–5 breast nodules. The calibration curve demonstrated that the prediction probability was slightly higher than the actual probability, which might be related to a portion of benign nodules being predicted as category of 4b or above (323/2,733) and a few of the malignant lesions being predicted as 4a or below (149/4,365). In our dataset, there were no negative images with no nodules. Although all the images enrolled in the training set had a nodule, the other area outside the nodule on the image, which was equivalent to the negative image without any nodular lesion, was also used in the whole feature extraction process and queried and learnt by the network. As a result, the model could process those images with or without nodules.
The emergence of artificial intelligence (AI) has provided an alternative solution to avoiding the subjective influence on the diagnosis and classification of a disease, including that involved with the BI-RADS classification of breast nodules (12,29). Through deep learning of large data sets, AI forms its own unique algorithm to provide referable objective information for doctors’ diagnosis and treatment (30). Jiang et al., having adopted a deep convolutional neural network (DCNN), identified the 4 molecular subtypes of breast cancer with the ACC ranging from 80.07% to 97.02% in 1 test set and 87.94% to 98.83% in the other test set (31). Ciritsis et al. demonstrated DCNN could mimic human decision-making in the evaluation of breast lesions according to the BI-RADS category, obtaining AUCs of 83.8 and 96.7 on the internal and external datasets, respectively, for a DCNN equivalent of 84.6 and 90.9 for the human operators (32). In testing the proposed 2-stage grading system against 2,238 cases with breast tumor in ultrasound images, Huang et al. found that the 2-stage framework achieved an ACC of 99.8% on BI-RADS category 3, 94% on 4a, 73.4% on 4b, 92.2% on 4c, and 87.6% on 5 (13). In this multicenter study, more than 20,000 images of nearly 4,000 patients with over 5,000 breast nodules from 20 clinical centers in China were collected, and pathological results involving a variety of patterns of breast nodular lesions were obtained in all of them; furthermore, more than 30 types of ultrasound machines were used. A CAD model that was trained with a training set comprising an abundance of pathologically confirmed images and taken from a multitude of ultrasound devices from different clinical centers would have higher generalization and better robustness in clinical application. Unlike the previous reported studies (6,10,29,33,34), in this study, the imaging features of the nodules, such as shape, orientation, margin, internal echo, posterior echo, and variability in surrounding tissue, were routinely extracted. In our research, the task-specific feature extraction and long-range feature capture capabilities of self-attention and multihead mechanisms used in transformer technology were adopted in order to minimize the influence of human-selected rules in the machine learning algorithm, which is the mode of end-to-end autonomous learning (35).
Lee et al. also demonstrated that the distinction ACC between benign and malignant breast nodules was significantly higher with the aid of a CAD model and that the AUC of the inexperienced radiologists evidently increased from 0.65 to 0.71, whereas the AUC of the experienced group changed marginally from 0.83 to 0.84 (36). Similar studies have shown that radiologists lacking experience in breast ultrasound have benefited significantly from the adoption of a CAD model, especially regarding category 4a breast nodules, thereby minimizing unnecessary biopsies (37,38). However, although the radiologists participating in this study had at least 8 years of experience with breast ultrasound, their classification consistency was markedly improved with almost all the k values increasing to greater than 0.6; moreover, diagnostic efficacy was also increased by approximately 24% (32.73–56.98%) and 7% (82.46–89.26%) for SEN and SPE, respectively, for the total classification on average, after they evaluated the nodule in combination with the CAD model diagnosis. Additionally, further accurate downgrading and upgrading of the lesions occurred in most (1,583/2,500, 63.32%) of the recategorizations performed by all radiologists. There was a high agreement rate (226/272, 83.09%) with pathological diagnoses in those reductions from category 4a or above to 3. These accurate category decreases assisted by the CAD model avoid unnecessary biopsies and reduce the medical costs and psychological burden of patients (24). Xiao et al. demonstrated that CAD could improve the diagnostic performance of experienced radiologist and subsequently prevent the unnecessary biopsy of 54.76% of benign lesions (39); Choi et al. also found that CAD could assist radiologists in correctly reclassifying BI-RADS 3 or 4a nodules (40). Conversely, an agreement rate (68/147, 46.26%) with pathology was obtained in those increases from category 3 to 4a or above. The lower rate of less than 50% might be related to the larger range of positive predictive values between category 4a and 5 (malignant possibility 2–95%). These increased categorizations provide patients and surgeons with confidence for biopsy or surgery, thereby preventing delays in the diagnosis and treatment of breast cancer. In addition, the lack of typical and obvious appearances of the malignant nodule caused 46 incorrect recategorizations of the 25 images. Appearances including cystic-solid change, parallel growth, curvilinear calcification, or intraductal nodule on images can easily lead to a lack of confidence or hesitation in determining the classification. Although the occurrence rate was low (46/272, 16.91%), it is worth noting that CAD might also mislead doctors in making erroneous downgrades.
This study had two main limitations. First, the optimal number of images used in the CAD training set should be the number in which all the sonographic features of the lesion can be contained but balanced with the appropriate image reviewing workload. In our study, about 4 pieces of the image per nodule on average were collected for the training set with a small number of errors. Therefore, it is warranted to determine whether a few of the images should be added to have a higher diagnostic sensitivity of the CAD system so as to further reduce its error of degradation. Second, color Doppler ultrasound was not included in our dataset, with which the combination of grayscale ultrasound images in establishing an AI model could prove superior in the differentiation of benign from malignant breast nodules (10). In addition, spectral Doppler, elastography, and contrast-enhanced ultrasound are also expected to be studied in this field.
The value of AI in the field of breast ultrasound has gradually been confirmed. Although this study is one among many, it may help serve as a cornerstone toward establishing mature and applicable products in the future even if the full integration of AI into clinical practice remains a somewhat distant reality. There remain many avenues for improvement, such as open-source sharing of models, large-scale public datasets, dependence on region of interest annotation, formulation of research and development guidelines applicable to different medical institutions and manufacturers, and supervision of laws and integrity (41-43). Thus, the aim to construct an AI product with a high efficacy in diagnosis and treatment is realizable and was the primary motivation of this research.
Conclusions
Through our multicenter study, a CAD model based on transformer technology was established, achieving a high ACC, a SEN of more than 85%, and a SPE of at least 95% in the classification of BI-RADS category 3–5 nodules, showing a similar capacity to the veteran models. Even radiologists who were experienced with breast ultrasound could significantly improve their own diagnostic efficacy and consistency with others in the classification through the assistance of the CAD model.
Acknowledgments
Funding: This project was supported by the National Key Research and Development Plan of China (No. 2016YFC0104803).
Footnote
Reporting Checklist: The authors have completed the TRIPOD reporting checklist. Available at https://qims.amegroups.com/article/view/10.21037/qims-22-1091/rc
Conflicts of Interest: All authors have completed the ICMJE uniform disclosure form (available at https://qims.amegroups.com/article/view/10.21037/qims-22-1091/coif). The authors have no conflicts of interest to declare.
Ethical Statement: The authors are accountable for all aspects of the work in ensuring that questions related to the accuracy or integrity of any part of the work are appropriately investigated and resolved. The study was conducted in accordance with the Declaration of Helsinki (as revised in 2013). The study was approved by Ethics Committee of Beijing Tongren Hospital, Capital Medial University (No. TRECKY2019-139), and the requirement for individual consent for this retrospective analysis was waived.
Open Access Statement: This is an Open Access article distributed in accordance with the Creative Commons Attribution-NonCommercial-NoDerivs 4.0 International License (CC BY-NC-ND 4.0), which permits the non-commercial replication and distribution of the article with the strict proviso that no changes or edits are made and the original work is properly cited (including links to both the formal publication through the relevant DOI and the license). See: https://creativecommons.org/licenses/by-nc-nd/4.0/.
References
- Shieh Y, Eklund M, Sawaya GF, Black WC, Kramer BS, Esserman LJ. Population-based screening for cancer: hope and hype. Nat Rev Clin Oncol 2016;13:550-65. [Crossref] [PubMed]
- Zheng RS, Sun KX, Zhang SW, Zeng HM, Zou XN, Chen R, Gu XY, Wei WW, He J. Report of cancer epidemiology in China, 2015. Zhonghua Zhong Liu Za Zhi 2019;41:19-28. [PubMed]
- Li J, Bu Y, Lu S, Pang H, Luo C, Liu Y, Qian L. Development of a Deep Learning-Based Model for Diagnosing Breast Nodules With Ultrasound. J Ultrasound Med 2021;40:513-20. [Crossref] [PubMed]
- Mendelson EB, Böhm-Vélez M, Berg WA, et al. ACR BI-RADS Ultrasound. In: ACR BI-RADS Atlas, Breast Imaging Reporting and Data System. 5th edition. Reston, VA, USA: American College of Radiology, 2013.
- Stavros AT, Freitas AG, deMello GGN, Barke L, McDonald D, Kaske T, Wolverton D, Honick A, Stanzani D, Padovan AH, Moura APC, de Campos MCV. Ultrasound positive predictive values by BI-RADS categories 3-5 for solid masses: An independent reader study. Eur Radiol 2017;27:4307-15. [Crossref] [PubMed]
- Niu S, Huang J, Li J, Liu X, Wang D, Zhang R, Wang Y, Shen H, Qi M, Xiao Y, Guan M, Liu H, Li D, Liu F, Wang X, Xiong Y, Gao S, Wang X, Zhu J. Application of ultrasound artificial intelligence in the differential diagnosis between benign and malignant breast lesions of BI-RADS 4A. BMC Cancer 2020;20:959. [Crossref] [PubMed]
- Tang Y, Liang M, Tao L, Deng M, Li T. Machine learning-based diagnostic evaluation of shear-wave elastography in BI-RADS category 4 breast cancer screening: a multicenter, retrospective study. Quant Imaging Med Surg 2022;12:1223-34. [Crossref] [PubMed]
- Xu Y, Wang Y, Yuan J, Cheng Q, Wang X, Carson PL. Medical breast ultrasound image segmentation by machine learning. Ultrasonics 2019;91:1-9. [Crossref] [PubMed]
- Wan KW, Wong CH, Ip HF, Fan D, Yuen PL, Fong HY, Ying M. Evaluation of the performance of traditional machine learning algorithms, convolutional neural network and AutoML Vision in ultrasound breast lesions classification: a comparative study. Quant Imaging Med Surg 2021;11:1381-93. [Crossref] [PubMed]
- Qian X, Zhang B, Liu S, Wang Y, Chen X, Liu J, Yang Y, Chen X, Wei Y, Xiao Q, Ma J, Shung KK, Zhou Q, Liu L, Chen Z. A combined ultrasonic B-mode and color Doppler system for the classification of breast masses using neural network. Eur Radiol 2020;30:3023-33. [Crossref] [PubMed]
- Shia WC, Chen DR. Classification of malignant tumors in breast ultrasound using a pretrained deep residual network model and support vector machine. Comput Med Imaging Graph 2021;87:101829. [Crossref] [PubMed]
- Destrempes F, Trop I, Allard L, Chayer B, Garcia-Duitama J, El Khoury M, Lalonde L, Cloutier G. Added Value of Quantitative Ultrasound and Machine Learning in BI-RADS 4-5 Assessment of Solid Breast Lesions. Ultrasound Med Biol 2020;46:436-44. [Crossref] [PubMed]
- Huang Y, Han L, Dou H, Luo H, Yuan Z, Liu Q, Zhang J, Yin G. Two-stage CNNs for computerized BI-RADS categorization in breast ultrasound images. Biomed Eng Online 2019;18:8. [Crossref] [PubMed]
- Shamshad F, Khan S, Waqas Zamir S, Haris Khan M, Hayat M, Shahbaz Khan F, Fu H. Transformers in Medical Imaging: A Survey. arXiv 2022. arXiv:2201.09873.
- Tuli S, Dasgupta I, Grant E, Griffiths TL. Are Convolutional Neural Networks or Transformers more like human vision? arXiv 2021. arXiv:2105.07197.
- Vaswani A, Shazeer N, Parmar N, Uszkoreit J, Jones L, Gomez AN, Kaiser L, Polosukhin I. Attention Is All You Need. arXiv 2017. arXiv:1706.03762.
- Olthof AW, Shouche P, Fennema EM, IJpma FFA, Koolstra RHC, Stirler VMA, van Ooijen PMA, Cornelissen LJ. Machine learning based natural language processing of radiology reports in orthopaedic trauma. Comput Methods Programs Biomed 2021;208:106304. [Crossref] [PubMed]
- Zhang H, Hu D, Duan H, Li S, Wu N, Lu X. A novel deep learning approach to extract Chinese clinical entities for lung cancer screening and staging. BMC Med Inform Decis Mak 2021;21:214. [Crossref] [PubMed]
- He K, Gan C, Li Z, Rekik I, Yin Z, Ji W, Gao Y, Wang Q, Zhang J, Shen D. Transformers in Medical Image Analysis: A Review. arXiv 2022. arXiv:2202.12165.
- Liu Z, Lv Q, Lee CH, Shen L. Medical image analysis based on transformer: A Review. arXiv 2022. arXiv:2208.06643.
- Gheflati B, Rivaz H. Vision Transformer for Classification of Breast Ultrasound Images. arXiv 2021. arXiv:2110.14731.
- Baker N, Lu H, Erlikhman G, Kellman PJ. Deep convolutional networks do not classify based on global object shape. PLoS Comput Biol 2018;14:e1006613. [Crossref] [PubMed]
- Dosovitskiy A, Beyer L, Kolesnikov A, Weissenborn D, Zhai X, Unterthiner T, Dehghani M, Minderer M, Heigold G, Gelly S, Uszkoreit J, Houlsby N. An Image is Worth 16x16 Words: Transformers for Image Recognition at Scale. arXiv 2020. arXiv:2010.11929.
- Chae EY, Cha JH, Shin HJ, Choi WJ, Kim HH. Reassessment and Follow-Up Results of BI-RADS Category 3 Lesions Detected on Screening Breast Ultrasound. AJR Am J Roentgenol 2016;206:666-72. [Crossref] [PubMed]
- Adarsh AD, Kumar KR, Kodumur V, Bora MK. Role of breast ultrasound in evaluation of BIRADS 3 and BIRADS 4 breast masses. J Evolution Med Dent Sci 2017;6:3524-7. [Crossref]
- Liu Z, Lin Y, Cao Y, Hu H, Wei Y, Zhang Z, Lin S, Guo B. Swin Transformer: Hierarchical Vision Transformer using Shifted Windows. arXiv 2021. arXiv:2103.14030.
10.1109/ICCV48922.2021.00986 10.1109/ICCV48922.2021.00986 - Jales RM, Sarian LO, Torresan R, Marussi EF, Alvares BR, Derchain S. Simple rules for ultrasonographic subcategorization of BI-RADS®-US 4 breast masses. Eur J Radiol 2013;82:1231-5. [Crossref] [PubMed]
- Berg WA, Blume JD, Cormack JB, Mendelson EB. Training the ACRIN 6666 Investigators and effects of feedback on breast ultrasound interpretive performance and agreement in BI-RADS ultrasound feature analysis. AJR Am J Roentgenol 2012;199:224-35. [Crossref] [PubMed]
- Zhang E, Seiler S, Chen M, Lu W, Gu X. BIRADS features-oriented semi-supervised deep learning for breast ultrasound computer-aided diagnosis. Phys Med Biol 2020;65:125005. [Crossref] [PubMed]
- Fujioka T, Mori M, Kubota K, Oyama J, Yamaga E, Yashima Y, Katsuta L, Nomura K, Nara M, Oda G, Nakagawa T, Kitazume Y, Tateishi U. The Utility of Deep Learning in Breast Ultrasonic Imaging: A Review. Diagnostics (Basel) 2020;10:1055. [Crossref] [PubMed]
- Jiang M, Zhang D, Tang SC, Luo XM, Chuan ZR, Lv WZ, Jiang F, Ni XJ, Cui XW, Dietrich CF. Deep learning with convolutional neural network in the assessment of breast cancer molecular subtypes based on US images: a multicenter retrospective study. Eur Radiol 2021;31:3673-82. [Crossref] [PubMed]
- Ciritsis A, Rossi C, Eberhard M, Marcon M, Becker AS, Boss A. Automatic classification of ultrasound breast lesions using a deep convolutional neural network mimicking human decision-making. Eur Radiol 2019;29:5458-68. [Crossref] [PubMed]
- Moon WK, Lo CM, Cho N, Chang JM, Huang CS, Chen JH, Chang RF. Computer-aided diagnosis of breast masses using quantified BI-RADS findings. Comput Methods Programs Biomed 2013;111:84-92. [Crossref] [PubMed]
- Shan J, Alam SK, Garra B, Zhang Y, Ahmed T. Computer-Aided Diagnosis for Breast Ultrasound Using Computerized BI-RADS Features and Machine Learning Methods. Ultrasound Med Biol 2016;42:980-8. [Crossref] [PubMed]
- Valanarasu JMJ, Oza P, Hacihaliloglu I, Patel VM. Medical Transformer: Gated Axial-Attention for Medical Image Segmentation. arXiv 2021. arXiv:2102.10662.
10.1007/978-3-030-87193-2_4 10.1007/978-3-030-87193-2_4 - Lee J, Kim S, Kang BJ, Kim SH, Park GE. Evaluation of the effect of computer aided diagnosis system on breast ultrasound for inexperienced radiologists in describing and determining breast lesions. Med Ultrason 2019;21:239-45. [Crossref] [PubMed]
- Zhao C, Xiao M, Liu H, Wang M, Wang H, Zhang J, Jiang Y, Zhu Q. Reducing the number of unnecessary biopsies of US-BI-RADS 4a lesions through a deep learning method for residents-in-training: a cross-sectional study. BMJ Open 2020;10:e035757. [Crossref] [PubMed]
- Wang XY, Cui LG, Feng J, Chen W. Artificial intelligence for breast ultrasound: An adjunct tool to reduce excessive lesion biopsy. Eur J Radiol 2021;138:109624. [Crossref] [PubMed]
- Xiao M, Zhao C, Li J, Zhang J, Liu H, Wang M, Ouyang Y, Zhang Y, Jiang Y, Zhu Q. Diagnostic Value of Breast Lesions Between Deep Learning-Based Computer-Aided Diagnosis System and Experienced Radiologists: Comparison the Performance Between Symptomatic and Asymptomatic Patients. Front Oncol 2020;10:1070. [Crossref] [PubMed]
- Choi JS, Han BK, Ko ES, Bae JM, Ko EY, Song SH, Kwon MR, Shin JH, Hahn SY. Effect of a Deep Learning Framework-Based Computer-Aided Diagnosis System on the Diagnostic Performance of Radiologists in Differentiating between Malignant and Benign Masses on Breast Ultrasonography. Korean J Radiol 2019;20:749-58. [Crossref] [PubMed]
- Lei YM, Yin M, Yu MH, Yu J, Zeng SE, Lv WZ, Li J, Ye HR, Cui XW, Dietrich CF. Artificial Intelligence in Medical Imaging of the Breast. Front Oncol 2021;11:600557. [Crossref] [PubMed]
- Davis A, Billick K, Horton K, Jankowski M, Knoll P, Marshall JE, Paloma A, Palma R, Adams DB. Artificial Intelligence and Echocardiography: A Primer for Cardiac Sonographers. J Am Soc Echocardiogr 2020;33:1061-6. [Crossref] [PubMed]
- Moawad AW, Fuentes DT, ElBanan MG, Shalaby AS, Guccione J, Kamel S, Jensen CT, Elsayes KM. Artificial Intelligence in Diagnostic Radiology: Where Do We Stand, Challenges, and Opportunities. J Comput Assist Tomogr 2022;46:78-90. [Crossref] [PubMed]