Artificial intelligence-aided ultrasound in renal diseases: a systematic review
Introduction
In clinical practice, ultrasound has been essential for the noninvasive diagnosis and management of renal diseases as it provides information on anatomy and its condition. The main clinical application of renal ultrasound imaging includes chronic kidney disease (CKD), acute kidney injury, kidney stones, benign or malignant renal tumors, and urinary obstruction (1,2). Although conventional ultrasound is one of the most used imaging tools for renal disease screening and diagnosis, it confronts the challenges of low efficiency in separating regions of interest from the surroundings, as a result of speckle noise, low image quality, insufficient contrast, and inconsistent intensity profile. In addition, the adjacency of organs with high scattering tissues may cause shadows to partially occlude the organs, leading to incorrect decisions. The majority of these limitations are associated with the advancement of equipment or techniques and do not diminish with increased operational experience. Furthermore, as the workloads of physicians have increased dramatically, errors are inevitable (3,4).
In recent years, artificial intelligence (AI) techniques have improved the management of patients by healthcare professionals. Several reports have demonstrated the excellent accuracy of AI in the diagnosis of multiple fields, such as oncology (5), skin diseases (6), and epidemic prevention and control (7). AI-aided medical imaging analysis is one of the fundamental issues in the field of AI in medicine (8). Based on the assessment of characteristics from ultrasound images, AI techniques increase efficiency, reduce subjective errors, and achieve objectives with minimal manual input by providing trained radiologists with prescreened images and identified features (9). AI technology in medical imaging can be roughly divided into “non-deep” machine learning algorithms, based on handcrafted engineered features, and deep learning algorithms, with fewer manual preprocessing steps. Their key difference lies in the existence of explicit feature predefinition or selection (Figure 1). For a computer-aided diagnosis system based on machine learning, three main stages are required: image preprocessing, feature extraction (such as texture analysis), and analysis aimed at solving a particular task or application using classifiers (such as an artificial neural network) (10). According to a recently published study, AI-aided technique is valid for improving the diagnosis accuracy of urolithiasis, pediatric urology, renal transplant, and urologic neoplasms by computer-based prediction and decision support models, providing quantitative diagnosis information, and overcoming substantial interobserver variability in ultrasound interpretation (10). However, according to search results, in the field of renal ultrasound, limited advances in AI have been made in the past 10 years. It is unclear whether AI-aided ultrasound is a reliable and valid technique in renal disease diagnosis in clinical practice. The application of AI-aided ultrasound to diagnose and predict renal diseases has not been reviewed previously. Therefore, this systematic review aimed to clarify the state of AI-aided ultrasound research associated with renal diseases, and further analyze the challenges that remain to be addressed in this area. We present the following article in accordance with the PRISMA reporting checklist (available at https://qims.amegroups.com/article/view/10.21037/qims-22-1428/rc).
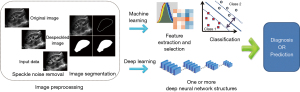
Methods
We searched the publication databases PubMed and Web of Science systematically in June 2022. In the search strategy, the following terms were used for the search syntax: (I) ultrasound: ultras*; (II) organs: renal, kidney; (III) artificial intelligence: artificial intelligent*, image segment*, texture analysis, machine learning, and deep learning. The complete search strategy was available from the authors. This study was conducted in accordance with the Preferred Reporting Items for Systematic Reviews and Meta-Analyses (PRISMA) guidelines (11). Two authors screened the titles and abstracts independently and deleted duplicate and irrelevant studies. Studies evaluating AI-aided ultrasound in renal disease diagnosis, detection, and prediction were eligible for inclusion in this study, and disagreements regarding the relevance of the studies were resolved by consensus. Animal studies, laboratory investigations, non-English studies, conference abstracts, and reviews were excluded. Because we mainly focused on the methods and efficiency of AI-aided ultrasound in disease diagnosis and prediction, the analyses of image registration and fusion (for ultrasound-guided kidney intervention) were excluded. Because of the heterogeneity of this review and the paucity of studies, a qualitative synthesis of the results used a narrative approach.
Checklists demonstrate the data obtained from the studies included in qualitative synthesis (Table 1 for image segmentation and Table 2 for AI-aided diagnosis). “Clinical parameters” included patient information and laboratory examination data. The performance considered in the studies was controversial depending on the study design. Therefore, we used accuracy/Dice similarity coefficient (DICE) as an evaluation parameter for image segmentation, and accuracy, area under the curve (AUC), and sensitivity/specificity as evaluation parameters for AI-aided diagnosis, because most studies addressed these metrics. Herein DICE is a measure of the overlap between two structures or methods.
Table 1
Author | Objective | Year | AI methods | Input | No. of patients | Data augmentation | No. of training set | No. of validation set | No. of testing set | Efficiency evaluation |
---|---|---|---|---|---|---|---|---|---|---|
Wang et al. (12) | Kidney segmentation (parenchymal area) | 2014 | 2-step level set (distance regularized level set, region-scalable fitting energy minimization method) | 2D image | 20 | – | 10 | – | – | SN score >0.9 |
Yin et al. (13) | Kidney segmentation | 2019 | Boundary distance regression deep neural network | 2D image | 100 | Transfer learning | 105 | 80 | – | DICE =94%, ACC =0.99 |
Yin et al. (14) | Kidney segmentation | 2020 | Boundary distance regression and pixel classification networks | 2D image | 152 | Transfer learning | 105 | 20 | 164 | DICE =93–94% |
Marsousi et al. (15) | Kidney detection | 2014 | Probabilistic kidney shape model, level set propagation | 3D image | Nm | – | 24 | – | Detection accuracy =92.86% | |
Marsousi et al. (3) | Kidney segmentation | 2017 | Shape-to-volume registration, combined prior knowledge of training shapes with anatomical knowledge; a level set method | 3D image | 8 | – | 16 | 30 | – | ACC =97.48%, Sensitivity/specificity =0.79/0.99 |
Chen et al. (16) | Kidney segmentation | 2021 | CNN (SDFNet) | 2D image | Nm | – | 450 | 50 | – | Sensitivity/specificity =0.94/0.99 |
Torres et al. (17) | Kidney segmentation | 2021 | A hybrid energy functional that combines localized region- and edge based terms | 3D image | Nm | – | 57 | – | – | DICE =81% |
Kang et al. (18) | Kidney-hydronephrosis evaluation (child) | 2013 | ASM with image acquisition priors, intensity correction and anatomical priors | 2D image | 34 | – | – | – | – | DICE =0.83–0.87 |
Cerrolaza et al. (19) | Kidney-hydronephrosis evaluation (child) | 2015 | Gabor-based appearance models, Graph-Cut Based method | 3D image | 19 | – | – | – | – | DICE =0.86 |
Cerrolaza et al. (20) | Kidney-hydronephrosis evaluation (child) | 2016 | Active shape model | 3D image | 39 | – | – | – | – | DICE =0.74–0.86 |
Nm, not mentioned; 2D, two-dimensional; 3D, three-dimensional; CNN, convolutional neural network; ASM, active shape models; ACC, accuracy; DICE, dice coefficient; SN, an author-defined index, the higher the SN score, the closer the segmentation result obtained by the method to the manual segmentation result.
Table 2
Author | Year | Input | Output (diagnosis/prediction) | Image preprocessing | Feature extraction | Classification/statistic method | Size of patient | Data augmentation | Size of Dataset | ACC (%) | AUC | Sensitivity/specificity | |
---|---|---|---|---|---|---|---|---|---|---|---|---|---|
Training set | Testing set | ||||||||||||
Bommanna et al. (21) | 2008 | 2D image | Normal, medical renal diseases and cortical cyst | Segmentation and rotation | Texture analysis | Hybrid fuzzy-neural system | Nm | – | 150 | 78 | 80–85 | – | – |
Subramanya et al. (22) | 2015 | 2D image | Normal, medical renal disease, cyst | De-speckling | KNN | SVM | 35 | – | 35 | Internal validation | 86.3 | – | – |
Bama et al. (23) | 2016 | 2D image | Hydronephrosis, nephrocalcinosis, normal and multicystic dysplasia | Speckle noise removal and segmentation | Texture analysis | SVM | Nm | Multiple ROIs obtained from one image | 40 | Internal validation | 88–92 | – | 0.88–0.92/– |
Yu et al. (24)* | 2020 | 2D image | Abnormal and normal cases of the kidney | Automatic recognization and location | – | DCNN | 1460 | – | 2,922 | 400 | 94.67 | 0.96 | 0.98/– |
Nithya et al. (25) | 2019 | 2D image | Normal, kidney stone and tumor | Speckle noise removal and segmentation (K-Means) | Texture analysis-GLCM | ANN | Nm | – | 80 | 20 | 93.45 | – | 1.00/0.90 |
Sudharson et al. (26) | 2020 | 2D image | Normal, cyst, stone, and tumor | Speckle noise removal | Deep neural networks | SVM | Nm | Transfer learning | 4,940 | 520 | 95–97 | – | – |
Sagreiya et al. (27) | 2019 | SWV, clinical information | Renal cell carcinoma, angiomyolipoma | – | – | SVM | 51 | – | 52 | Internal validation | 94 | 0.98 | – |
Shin et al. (28) | 2019 | 2D image | Wilms tumor, clear cell sarcoma and rhabdoid tumor of the kidney | Normalization | Texture analysis-GLCM, GLRLM | Subgroup analysis with post hoc analysis | 32 | – | 32 | Internal validation | >76 | >0.89 | >0.7/1.0 |
Selvarani et al. (29) | 2019 | 2D image | Kidney stone | Speckle noise removal and segmentation (K-Means) | Texture analysis-GLCM | SVM | Nm | – | 100 | Internal validation | 98.8 | – | – |
Muller et al. (30) | 2021 | Video (2D image) | ESWL hit rate | Image Annotation | – | U-net | 11 | – | 57 | Internal validation | 63.9 | – | 0.56/0.75 |
Kuo et al. (31) | 2019 | 2D image | CKD | Image normalization | – | CNN (ResNet, XGBoost) | 1299 | Transfer learning, image shift, rotation, horizontal flip | 4505 | Internal validation | 85.6 | 0.904 | 0.61/0.92 |
Hao et al. (32) | 2020 | 2D image | CKD (classification and screening) | – | Texture analysis | CNN (ResNet) | 226 | Transfer learning, image rotation, shifting, random gray level transformation of pixels | 226 | Internal validation | 96 | 0.97 | 0.99/0.82 |
Li et al. (33) | 2021 | 2D, CDFI and SWE parameters | CKD-abnormal and normal cases | – | KNN | MLP-SVM | 203 | – | 142 | 61 | 81 | 0.91 | 0.81/0.81 |
Zhang et al. (34)* | 2021 | 2D image | CKD-Membranous nephropathy and IgA nephropathy | Segmentation | LASSO logistic regression | Random forest, logistic regression | 68 | – | 470 | 153 | 76.5 | 0.76 | 0.75/0.89 |
Athavale et al. (35)* | 2021 | 2D image | CKD | Speckle noise removal, segmentation (U-net) | Pretrained CNN | XGBoost | 352 | Transfer learning | 5523 | 612 | 86.8 | – | 0.80/– |
Chen et al. (36) | 2019 | 2D image | CKD | Inpainting, median filter | Texture, standard deviation, area, and brightness analysis | SVM | 205 | – | 798 | Internal validation | 77.9–83.7 | – | – |
Zhu et al. (37) | 2022 | 2D, CDFI, SWE, clinical information | CKD (kidney fibrosis) | – | – | SVM | 117 | – | 117 | Internal validation | – | 0.833 | 0.77/0.72 |
Ahmad et al. (38) | 2021 | 2D image | CKD | De-speckling, cropping | Texture analysis-GLCM | LDA | Nm | – | 308 | Internal validation | 96–100 | – | – |
Kim et al. (39) | 2021 | 2D image | CKD | Histogram equalization, range filter preprocessing | Texture analysis-GLCM | ANN | Nm | – | 741 | Internal validation | 95.4 | – | – |
Abbasian Ardakani et al. (8) | 2017 | 2D image | Rejected and unrejected allografts | Segmentation | Texture analysis, LDA | Nearest neighbor classifier | 61 | – | 61 | – | 98.36 | 0.975 | 0.91/1.00 |
Abbasian et al. (40) | 2020 | 2D image | Increased or decreased serum creatinine (sCr) | – | Texture analysis-LDA | KNN | 40 | – | 40 | – | 93.5 | 0.974 | 0.95/0.91 |
Lin et al. (41) | 2021 | 2D image | Hydronephrosis in children | Gray processing, normalization, segmentation (U-net) | – | Fluid-to-kidney area ratio, linear regression | 699 | – | 1414 | 394 | – | 0.99 | 0.90/0.80 |
Smail et al. (42) | 2020 | 2D image | Hydronephrosis in children | Speckle noise removal, cropping, normalization | – | CNN | 673 | Image rotation and shifting | 2420 | Internal validation | 51–78 | – | – |
Cerrolaza et al. (43) | 2016 | 2D image | Hydronephrosis in children | – | Quantitative image analysis algorithms | SVM, logistic regression | 50 | – | 50 | Internal validation | – | 0.94–0.98 | 1.00/0.74–0.90 |
Zheng et al. (44) | 2019 | 2D image | CAKUT | Segmentation (graph-cuts), normalization | Transfer learning (CNN), texture analysis | SVM | 100 | – | 100 | Internal validation | 81–87 | 0.88–0.92 | 0.85–0.88/0.74–0.88 |
Yin et al. (45) | 2019 | 2D image | CAKUT | – | – | CNN (GCN) | 225 | – | 4933 | Internal validation | 85 | – | 0.86/0.84 |
Yin et al. (46) | 2020 | 2D image | CAKUT (posterior urethral valves) | Normalization | Transfer learning (CNN) | Deep learning (VGG 16) | 157 | Image rotation, left-right flipping | 157 | Internal validation | 92.5 | 0.96 | 0.87/0.98 |
*, low risk studies. Nm, not mentioned; 2D, two-dimensional; ACC, accuracy; AUC, area under the curve; KNN, k-nearest neighbor; SVM, support vector machine; ROI, region of interest; SWV, shear-wave velocity; SWE, shear-wave elastography; GLCM, grey level co-occurrence matrix; DCNN, deep convolutional neural network; CNN, convolutional neural network; GCN, graph convolutional network; VGG, visual geometry Group; ANN, artificial neural network; CDFI, color doppler flow imaging; CKD, chronic kidney disease; LASSO, least absolute shrinkage and selection operator; LDA, linear discriminant analysis; ESWL, extracorporeal shock wave lithotripsy; CAKUT, congenital abnormalities of the kidney and urinary tract.
The Prediction Model Risk of Bias Assessment Tool (PROBAST) (47) was used to assess the risk of bias for all the AI-aided diagnosis studies. It includes 20 signaling questions across four domains including participants, predictors, outcome, and analysis. The risk of bias in the studies was classified as low, moderate, or high in each domain and overall. The applicability concerns were not considered in this study because of the uncertain subject.
Results
The number of publications on AI-aided ultrasound in renal diseases has increased rapidly from 2016 onwards (Figure 2). Database searches identified 364 studies, which included 168 from the Web of Science and 196 from PubMed. After excluding duplicates, 240 studies were screened by title and abstract, of which 167 studies were irrelevant or non-English articles. Following full-text access, a total of 38 studies were considered eligible for review, including 10 for ultrasound image segmentation and 28 for AI-aided ultrasound diagnosis or prediction. Figure 3 summarizes the search strategy of this study.
Owing to the uncertain subjects and evaluation index, the studies included in the qualitative synthesis were divided into two parts: AI-aided diagnosis or prediction studies and image segmentation. For ultrasound image segmentation, the following indexes were summarized: author, year of publication, AI method, input, and number of patients, data augmentation methods, number of training sets, validation and testing sets, and efficiency evaluation. For AI-aided diagnosis or prediction, author, year of publication, input, output, image preprocessing, feature extraction, classification/statistics methods, size of patients, data augmentation, and size of training and testing set were summarized.
In AI-aided diagnosis or prediction studies, most of the input data were two-dimensional (2D) ultrasound images (25/28), and three studies used value-based data from multiple ultrasound techniques such as Color Doppler flow imaging (CDFI) and elastography (3/28). Output (targeted diagnosis or prediction) was indicated as the aim of studies, including automatic differential diagnosis of kidney lesions, kidney stone detection, CKD diagnosis/grading, quantitative analysis of hydronephrosis in children, and automatic diagnosis of congenital abnormalities of the kidney and urinary tract (CAKUT). To improve the accuracy, image segmentation, speckle noise removal/de-speckling, and normalization were the most frequently used image preprocessing methods. Six out of 19 machine learning studies were published before 2019. The most frequently used machine learning algorithm for classification was the support vector machine (SVM), and other algorithms contained logistic regression, linear regression, artificial neural network (ANN), k-Nearest Neighbor (KNN), random forest, linear discriminant analysis (LDA), and fuzzy-neural network. Feature extraction was an imperative procedure of AI-aided ultrasound studies and most of the machine learning studies used texture analysis. Deep learning algorithm used studies (9/28) were all published after 2018, and the commonly used algorithm methods were convolutional neural network (CNN) and its optimization algorithms. Although conventional deep learning methods did not need feature predefinition or selection, four of the recent studies combined traditional (like texture analysis) or cutting-edge feature extraction methods (like transfer learning) with deep learning algorithms to further improve the evaluation efficiency.
The size of the dataset was generally small in these published studies. The largest dataset was 5,523 cases. Only seven studies contained more than 1,000 images and over a third of the datasets (11/28) contained less than 100 images. With regards to the image source, the number of patients was not mentioned in nearly 25% of the studies (7/28). Nearly one in four studies used data augmentation methods to enlarge the dataset, and four of these used transfer learning. Regarding the evaluation performance, most of the studies used internal validation, only eight studies had performed external tests using independent datasets. The median values of accuracy and AUC of these studies were approximately 0.88 and 0.96, respectively.
The risk of bias evaluated by using PROBAST demonstrated that 86% of AI-aided diagnosis or prediction models were classified into a high-risk group (Figure 4). Most of the studies were classified as low risk in domains of participants, predictors, and outcome (62%, 83%, and 90%, respectively). However, in domains of analysis, high risk accounted for a higher ratio (62%). High risk factors included the lack of appropriate data sources (1.1) and a reasonable number of participants (4.1), inappropriate handling method for missing data (4.4), using univariable analysis for predictor selection (4.5), ignorance of the complexities in data (4.6), inappropriate evaluation of relevant model performance measures (4.7), and model overfitting, underfitting, and optimism (4.8).
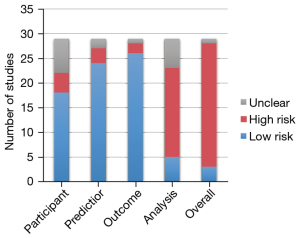
Segmentation and detection
Image segmentation is often a first and essential stage for image analysis and further evaluation. In this qualitative synthesis, 10 studies of renal ultrasound image segmentation were identified. Five studies used 2D ultrasound images and five studies used three-dimensional (3D) ultrasound images. Only one of the studies evaluated the performance of the model using an individual testing set.
Seven studies focused on the innovation and efficiency of kidney segmentation algorithms (3,12-17). Wang et al. proposed a two-step level set method for segmentation of both the kidney boundary (distance regularized level set evolution) and kidney collecting system (region-scalable fitting energy minimization) to determine the renal parenchymal area, and the SN score evaluated using ten cases was >0.9 (12). Yin et al. developed a boundary distance regression deep neural network for kidney segmentation, which extracted high-level image features using transfer learning (13). To improve the stability of kidney ultrasound image segmentation, Yin et al. found that the boundary detection strategy worked better than pixelwise classification techniques for segmenting clinical ultrasound images (14). The DICE score for these deep learning models achieved 0.93–0.94. Marsousi et al. combined prior knowledge of training shapes (shape-to-volume registration) and anatomical knowledge, to propose a detecting and segmenting method in 3D kidney ultrasound image segmentation. The detection accuracy was 92.86% (3,15).
Three studies focused on the segmentation of the kidney collecting (KS) system and quantitative kidney-hydronephrosis evaluation in children (18-20). Hydronephrosis index (HI) is a quantitative measurement of hydronephrosis severity, which was defined as the ratio of the collecting system area to the total area of the kidney collecting system and renal parenchyma (43). Kang et al. used a combination of improvements made to the active shape model framework and minimal user intervention to segment the KS system and computed the HI semi-automatically. The DICE score was >0.8 (18). Cerrolaza et al. developed a variant of the popular active shape model considering brightness and contrast normalization, and positional prior information in 3D kidney image segmentation with a DICE score of 0.86 (19).
AI-aided renal ultrasound diagnosis and prediction
Differential diagnosis or identification of kidney diseases
In early studies, classifiers were trained for simple differential diagnosis among different lesions, or between normal and abnormal ultrasound performances. In this systematic review, 10 studies were identified for differential diagnosis or identification of kidney diseases (21-30). After image rotation, speckle noise removal, and normalization, AI methods could distinguish normal, cyst, kidney stone, tumor, hydronephrosis, nephrocalcinosis, and multicystic dysplasia rapidly with an accuracy range from 80% to 97% (21-25). Sudharson et al. introduced an ensemble of pretrained deep neural networks (DNNs) like ResNet-101, ShuffleNet, and MobileNet-v2 using transfer learning, and final predictions were done by using the majority voting technique. With an accuracy of 95–97%, it showed better classification performance than the individual models in the early and automatic diagnosis of kidney disorders (26). Sagreiya et al. used value-based SVM analysis to improve the ability of shear wave elastography in differentiating renal cell carcinoma from angiomyolipoma (27). The results of a study by Shin et al. indicated that texture analysis using features from the second-order statistics achieved an AUC greater than 0.89 for differentiating Wilms tumor from clear cell sarcoma and rhabdoid tumor (28).
CKD
CKD is defined as a chronic condition that causes kidney damage for more than three months or continuous kidney dysfunction. In clinical applications, CKD is usually identified through the glomerular filtration rate (GFR) test, especially when GFR is lower than 60 mL/min/1.73 m2 for 3 months or more. Early and noninvasive detection is crucial to preventing or delaying the progression of CKD. In this systematic review, 12 studies were identified in this section, of which 10 referred to CKD and related diseases classification or screening (31-39,48) and two referred to complications after allograft renal transplantation (8,40). Iqbal et al. showed that texture feature obtained from the cortex region in ultrasound images was more significant than those obtained from the entire kidney or renal medulla in distinguishing between normal and CKD patients (48). Kuo et al. combined a deep neural network with a transfer learning technique to identify CKD status based on 4,505 kidney ultrasound images labeled using GFR. This AI-GFR estimation had reached a classification accuracy of 85.6% (31). Hao et al. proposed a novel approach named texture branch network containing both traditional texture features and deep features for CKD image screening. This scheme of fusing texture features and deep features combined with transfer learning was found to be suitable for an unbalanced small-sample dataset with an accuracy of 96% and a sensitivity of 99% (32). Li et al. used machine learning classifiers for the diagnosis of CKD based on value-based data of 2D, CDFI, and shear wave elastography (SWE). It showed that the elastic hardness parameter of the renal cortex was the most important, and the highest diagnostic accuracy of SVM was 80.98% (33). In a study by Zhang et al., quantitative radiomics features based on kidney ultrasound images were associated with the histological classification of glomerulopathy, which could distinguish IgA nephropathy from membranous nephropathy (34). The ability of ultrasound-based prediction of kidney interstitial fibrosis and tubular atrophy using a deep learning framework was also demonstrated in a diagnostic evaluation of 6,135 images in a study by Athavale et al., and the accuracy at the patient level was approximately 90% (35). In an assessment of kidney function after allograft transplantation, Abbasian et al. used 16 texture features extracted from 61 cases classified by the nearest neighbor classifier to identify the occurrence of transplant rejection, the AUC was 0.975 (8).
Pediatric application
The application of AI-aided renal ultrasound diagnosis in the pediatric domain mainly consisted of hydronephrosis in children (three studies) and CAKUT (three studies) (41-45,49,50). Lin et al. and Smail et al. used a deep learning approach to grade hydronephrosis ultrasound images and quantify the fluid and kidney areas automatically (41,42). Cerrolaza et al. introduced a semi-automated means which computed 131 morphological parameters (including size, geometric shape, and curvature) to define sonographic biomarkers for hydronephrotic renal units. As a consequence of a good performance (AUC 0.94–0.98), this method could potentially decrease the number of diuretic renograms in up to 62% of children (43).
Three studies of AI-aided CAKUT ultrasound diagnosis were identified, which were performed in the same single research center. CAKUT, including posterior urethral valves and kidney dysplasia, is the most common cause of end-stage renal disease in children (49). The diagnosis of CAKUT is usually based on kidney size, hydronephrosis, abnormal kidney position, echogenicity of the kidney parenchyma, and ureter and bladder abnormalities (50). Zheng et al. developed a new model that combined pretrained deep features based on transfer learning with conventional imaging features to distinguish CAKUT from controls. The AUC of this approach achieved 0.92, which performed better than classifiers built on either the transfer learning features or the conventional features alone (44). Yin et al. introduced a multi-instance deep learning method for fast diagnosis of CAKUT with an AUC of 0.96, which was found to be better than classifiers built on either single sagittal images or single transverse images (45).
Discussion
In the clinical practice of renal ultrasound imaging, there are still some key problems that have not been resolved, such as quantitative analysis of CKD severity. In addition to improving the diagnosis efficiency of ultrasound, it is also significant to explore more image features, which could reflect the pathological type and features (46). In recent years, AI technology in medical imaging has involved the diagnosis of various diseases (51), and the use of AI technology in renal ultrasound has shown potential in addressing the problems above (52,53). In this systematic review, we have illustrated and analyzed the current status of AI-aided renal ultrasound from two important aspects: image segmentation and AI-aided diagnosis or prediction.
Image segmentation, which extracts regions of interest from the original image, helps characterize the tissue and further improves the efficiency of diagnosis; it is a reliable and essential procedure for ultrasound images (16,54,55). Speckle noise, acoustic shadow, and low contrast between kidneys and other tissues are problems in renal segmentation (12). Regardless of 2D or 3D images, because the kidney has a known shape and localization, the combination of prior knowledge of training shapes and anatomical knowledge proved superior in improving the accuracy of kidney detection and segmentation on ultrasound images (3). In kidney segmentation studies enrolled in this systematic review listed in Table 1, we found the studies using prior knowledge had great segmentation performance (3,19,20). In addition, image preprocessing using Gaussian filtering, wavelet-based filtering, etc. was reported to be effective in addressing the problems of speckle noises (15).
For AI-aided diagnosis or prediction studies, a serious problem is whether the studies of AI-aided methods can be explainable and reliable for clinical application (56). The latest guidance on AI released by the World Health Organization in 2021 also defined “Ensure transparency, explainability, and intelligibility” as one of the six core principles (57). The systematic review shows that the focus of the research is switching to multicenter, big data studies, especially after 2019. Clinical problem-oriented research has become the focus of research involving AI technology in renal ultrasound. Nevertheless, based on the evaluation of PROBAST in the results, the unclear source of data, inadequate sample size, inappropriate analysis methods, and lack of rigorous external validation are found to be the most frequent and critical risk factors in AI-aided renal ultrasound studies. As known, overfitting is a common problem in studies with a small number of samples, which is caused by not only the small size of the dataset but also the small number of patients (58). However, in the period covered by the search, the number of patients was not mentioned in nearly a quarter of AI-aided renal ultrasound studies. From the aspects of data collected, the size and quality of sample data are both important in this kind of research. However, what sample size would be adequate for AI-aided ultrasound studies is still unclear and controversial. Some of the research followed the Widrow-Hoff learning rule, which suggested 10 data or patients for every image feature that would be used in the model, but this rule is very rough (59). In different clinical scenarios, the sample size estimate should take suitable crowd, the source of the data, data acquired equipment, and the type of sample into consideration, as well as data from the results of previous clinical studies. Furthermore, it suggested that prospective randomized data collected in hospital is preferred, rather than open-source data, which could help ensure generalization and repeatability of AI methods. Similarly, to avoid serious bias and overfitting, an effort should be made to perform AI-related clinical trials according to the requirement of the latest guidelines (60).
According to Table 2, we found only eight of 28 studies performed external tests using an independent dataset, and 17 of 28 studies performed an internal test. Although heat maps, feature visualization, prototypical comparisons, and other approaches or indicators have been used to illustrate the performance of AI techniques in medicine, it is difficult to avoid the influence of subjectivity (61). Based on highly validated performance requirements, rigorous internal and external validations are recommended for AI-aided studies (62).
Abnormal and normal ultrasound image identification that can distinguish one lesion from another (such as computer-aided ultrasound diagnosis of normal, medical renal disease, and kidney cyst) automatically were popular in earlier studies (21-25). In general, the performance of the classification algorithms in these studies was impressive. However, because their ultrasound features were significantly different, these tasks were void of applicable clinical value. Since 2019, the evaluation of CKD using AI-aided ultrasound imaging has gradually become a hot topic. Although timely and effective treatment (like hemodialysis and kidney transplant) is the primary focus of CKD, of equal importance is the achievement of early detection (63). Still, it is difficult to make a quantitative diagnosis of CKD at an early stage; a GFR test based on blood serum creatine level (sCr) always changes significantly in CKD stages 3−5. In addition, the gold standard of CKD diagnosis is a biopsy, which is invasive with the risk of complications that hamper its general application (64). Texture features of renal ultrasound images, especially for the renal cortex, were various in different stages of CKD based on the changes in microstructure including fibrosis composition and lipid fraction (65). Thus, texture analysis has been widely reported as an effective approach to reducing interobserver variability. Unlike renal tumors, kidney stones, or other renal diseases, good performances of CKD evaluation using AI-aided renal ultrasound were demonstrated by studies that used different datasets. As for the future research trend in this field, according to the results of this systematic review, multicenter studies with big data should be considered for the evaluation of CKD using AI-aided renal ultrasound. For renal diseases with few reports, basic research on small and medium-sized samples should be performed to assess algorithm fitness and effectiveness.
There were also some limitations in this systematic review. First, the included studies in this review had high heterogeneity, which was caused by various clinical themes, AI methods, and the evaluation methods involved. Therefore, this systematic review only reflected the recent progress of the application of AI methods in renal disease, rather than any definite quantitative conclusion. Second, acute kidney injury was excluded in this review because the research involving the application of ultrasound in this disease is rarely reported. Last, although PubMed and Web of Science were selected as the databases in this review, a small number of AI studies published at preprint sites and other databases were not included.
Conclusions
AI provides a novel efficient strategy for the evaluation and diagnosis of renal diseases. In this study, we conducted a systematic review to evaluate and analyze the trend in the application of AI techniques in renal ultrasound. The use of AI-based ultrasound systems in CKD and quantitative hydronephrosis diagnosis will be a promising possibility in the near future. It should be noted that to improve the stability and reliability, the sample size and image quality, rigorous external validation, and adherence to guidelines and standards should be carefully considered in future studies.
Acknowledgments
Funding: This work was supported in part by the National Natural Science Foundation of China (No. 82102054), Clinical Research 4310 Program of the First Affiliated Hospital of The University of South China (No. 4310-2021-K06).
Footnote
Reporting Checklist: The authors have completed the PRISMA reporting checklist. Available at https://qims.amegroups.com/article/view/10.21037/qims-22-1428/rc
Conflicts of Interest: All authors have completed the ICMJE uniform disclosure form (available at https://qims.amegroups.com/article/view/10.21037/qims-22-1428/coif). Zhiyi Chen serves as an unpaid editorial board member of Quantitative Imaging in Medicine and Surgery. The other authors have no conflicts of interest to declare.
Ethical Statement: The authors are accountable for all aspects of the work in ensuring that questions related to the accuracy or integrity of any part of the work are appropriately investigated and resolved.
Open Access Statement: This is an Open Access article distributed in accordance with the Creative Commons Attribution-NonCommercial-NoDerivs 4.0 International License (CC BY-NC-ND 4.0), which permits the non-commercial replication and distribution of the article with the strict proviso that no changes or edits are made and the original work is properly cited (including links to both the formal publication through the relevant DOI and the license). See: https://creativecommons.org/licenses/by-nc-nd/4.0/.
References
- Romero-González G, Manrique J, Slon-Roblero MF, Husain-Syed F, De la Espriella R, Ferrari F, Bover J, Ortiz A, Ronco C. PoCUS in nephrology: a new tool to improve our diagnostic skills. Clin Kidney J 2022;16:218-29. [Crossref] [PubMed]
- Ronco C, Bellomo R, Kellum JA. Acute kidney injury. Lancet 2019;394:1949-64. [Crossref] [PubMed]
- Marsousi M, Plataniotis KN, Stergiopoulos S. An Automated Approach for Kidney Segmentation in Three-Dimensional Ultrasound Images. IEEE J Biomed Health Inform 2017;21:1079-94. [Crossref] [PubMed]
- Chen J, Remulla D, Nguyen JH, Dua A, Liu Y, Dasgupta P, Hung AJ. Current status of artificial intelligence applications in urology and their potential to influence clinical practice. BJU Int 2019;124:567-77. [Crossref] [PubMed]
- Huynh E, Hosny A, Guthier C, Bitterman DS, Petit SF, Haas-Kogan DA, Kann B, Aerts HJWL, Mak RH. Artificial intelligence in radiation oncology. Nat Rev Clin Oncol 2020;17:771-81. [Crossref] [PubMed]
- Esteva A, Kuprel B, Novoa RA, Ko J, Swetter SM, Blau HM, Thrun S. Dermatologist-level classification of skin cancer with deep neural networks. Nature 2017;542:115-8. [Crossref] [PubMed]
- Jin C, Chen W, Cao Y, Xu Z, Tan Z, Zhang X, Deng L, Zheng C, Zhou J, Shi H, Feng J. Development and evaluation of an artificial intelligence system for COVID-19 diagnosis. Nat Commun 2020;11:5088. [Crossref] [PubMed]
- Abbasian Ardakani A, Mohammadi A, Khalili Najafabad B, Abolghasemi J. Assessment of Kidney Function After Allograft Transplantation by Texture Analysis. Iran J Kidney Dis 2017;11:157-64. [PubMed]
- Hosny A, Parmar C, Quackenbush J, Schwartz LH, Aerts HJWL. Artificial intelligence in radiology. Nat Rev Cancer 2018;18:500-10. [Crossref] [PubMed]
- Shah M, Naik N, Somani BK, Hameed BMZ. Artificial intelligence (AI) in urology-Current use and future directions: An iTRUE study. Turk J Urol 2020;46:S27-39. [Crossref] [PubMed]
- Page MJ, McKenzie JE, Bossuyt PM, Boutron I, Hoffmann TC, Mulrow CD, et al. The PRISMA 2020 statement: an updated guideline for reporting systematic reviews. BMJ 2021;372: [PubMed]
- Wang H, Pulido JE, Song Y, Furth SL, Tu C, Zhang C, Li C, Tasian GE. Segmentation of renal parenchymal area from ultrasound images using level set evolution. Annu Int Conf IEEE Eng Med Biol Soc 2014;2014:4703-6. [PubMed]
- Yin S, Zhang Z, Li H, Peng Q, You X, Furth SL, Tasian GE, Fan Y. Fully-automatic segmentation of kidneys in clinical ultrasound images using a boundary distance regression network. Proc IEEE Int Symp Biomed Imaging 2019;2019:1741-4. [Crossref] [PubMed]
- Yin S, Peng Q, Li H, Zhang Z, You X, Fischer K, Furth SL, Tasian GE, Fan Y. Automatic kidney segmentation in ultrasound images using subsequent boundary distance regression and pixelwise classification networks. Med Image Anal 2020;60:101602. [Crossref] [PubMed]
- Marsousi M, Plataniotis KN, Stergiopoulos S. Shape-based kidney detection and segmentation in three-dimensional abdominal ultrasound images. Annu Int Conf IEEE Eng Med Biol Soc 2014;2014:2890-4. [Crossref] [PubMed]
- Chen G, Dai Y, Li R, Zhao Y, Cui L, Yin X. SDFNet: Automatic segmentation of kidney ultrasound images using multi-scale low-level structural feature. Expert Systems with Application 2021;185:115619. [Crossref]
- Torres HR, Queiros S, Morais P, Oliveira B, Gomes-Fonseca J, Mota P, Lima E, D'Hooge J, Fonseca JC, Vilaca JL. Kidney Segmentation in 3-D Ultrasound Images Using a Fast Phase-Based Approach. IEEE Trans Ultrason Ferroelectr Freq Control 2021;68:1521-31. [Crossref] [PubMed]
- Kang X, Safdar N, Myers E, Martin AD, Grisan E, Peters CA, Linguraru MG. Automatic analysis of pediatric renal ultrasound using shape, anatomical and image acquisition priors. Med Image Comput Comput Assist Interv 2013;16:259-66.
- Cerrolaza JJ, Grisan E, Safdar N, Myers E, Jago J, Peters CA, Linguraru MG. Quantification of kidneys from 3D ultrasound in pediatric hydronephrosis. Annu Int Conf IEEE Eng Med Biol Soc 2015;2015:157-60. [Crossref] [PubMed]
- Cerrolaza JJ, Safdar N, Biggs E, Jago J, Peters CA, Linguraru MG. Renal Segmentation From 3D Ultrasound via Fuzzy Appearance Models and Patient-Specific Alpha Shapes. IEEE Trans Med Imaging 2016;35:2393-402. [Crossref] [PubMed]
- Bommanna Raja K, Madheswaran M, Thyagarajah K. A hybrid fuzzy-neural system for computer-aided diagnosis of ultrasound kidney images using prominent features. J Med Syst 2008;32:65-83. [Crossref] [PubMed]
- Subramanya MB, Kumar V, Mukherjee S, Saini M. SVM-Based CAC System for B-Mode Kidney Ultrasound Images. J Digit Imaging 2015;28:448-58. [Crossref] [PubMed]
- Bama S, Selvathi D. Discrete Tchebichef moment based machine learning method for the classification of disorder with ultrasound kidney images. Biomed Res 2016;2016:S223-9.
- Yu W, Zhang Y. Automated detection of kidney abnormalities using multi-feature fusion convolutional neural networks. Knowl Based Syst 2020;200:105873. [Crossref]
- Nithya A, Appathurai A, Venkatadri N, Ramji D, Palagan C. Kidney disease detection and segmentation using artificial neural network and multi-kernel k-means clustering for ultrasound images. Measurement 2019;149:106952. [Crossref]
- Sudharson S, Kokil P. An ensemble of deep neural networks for kidney ultrasound image classification. Comput Methods Programs Biomed 2020;197:105709. [Crossref] [PubMed]
- Sagreiya H, Akhbardeh A, Li D, Sigrist R, Chung BI, Sonn GA, Tian L, Rubin DL, Willmann JK. Point Shear Wave Elastography Using Machine Learning to Differentiate Renal Cell Carcinoma and Angiomyolipoma. Ultrasound Med Biol 2019;45:1944-54. [Crossref] [PubMed]
- Shin HJ, Kwak JY, Lee E, Lee MJ, Yoon H, Han K, Kim MJ. Texture Analysis to Differentiate Malignant Renal Tumors in Children Using Gray-Scale Ultrasonography Images. Ultrasound Med Biol 2019;45:2205-12. [Crossref] [PubMed]
- Selvarani S, Rajendran P. Detection of Renal Calculi in Ultrasound Image Using Meta-Heuristic Support Vector Machine. J Med Syst 2019;43:300. [Crossref] [PubMed]
- Muller S, Abildsnes H, Østvik A, Kragset O, Gangås I, Birke H, Langø T, Arum CJ. Can a Dinosaur Think? Implementation of Artificial Intelligence in Extracorporeal Shock Wave Lithotripsy. Eur Urol Open Sci 2021;27:33-42. [Crossref] [PubMed]
- Kuo CC, Chang CM, Liu KT, Lin WK, Chiang HY, Chung CW, Ho MR, Sun PR, Yang RL, Chen KT. Automation of the kidney function prediction and classification through ultrasound-based kidney imaging using deep learning. NPJ Digit Med 2019;2:29. [Crossref] [PubMed]
- Hao P, Xu Z, Tian S, Wu F, Chen W, Wu J, Luo X. Texture branch network for chronic kidney disease screening based on ultrasound images. Front Inform Technol Electron Eng 2020;21:1161-70. [Crossref]
- Li G, Liu J, Wu J, Tian Y, Ma L, Liu Y, Zhang B, Mou S, Zheng M. Diagnosis of Renal Diseases Based on Machine Learning Methods Using Ultrasound Images. Curr Med Imaging 2021;17:425-32. [Crossref] [PubMed]
- Zhang L, Chen Z, Feng L, Guo L, Liu D, Hai J, Qiao K, Chen J, Yan B, Cheng G. Preliminary study on the application of renal ultrasonography radiomics in the classification of glomerulopathy. BMC Med Imaging 2021;21:115. [Crossref] [PubMed]
- Athavale AM, Hart PD, Itteera M, Cimbaluk D, Patel T, Alabkaa A, Arruda J, Singh A, Rosenberg A, Kulkarni H. Development and Validation of a Deep Learning Model to Quantify Interstitial Fibrosis and Tubular Atrophy From Kidney Ultrasonography Images. JAMA Netw Open 2021;4:e2111176. [Crossref] [PubMed]
- Chen C, Pai T, Hsu H, Lee C, Chen K, Chen Y. Prediction of chronic kidney disease stages by renal ultrasound imaging. Enterp Inf Syst 2019;14:178-95. [Crossref]
- Zhu M, Ma L, Yang W, Tang L, Li H, Zheng M, Mou S. Elastography ultrasound with machine learning improves the diagnostic performance of traditional ultrasound in predicting kidney fibrosis. J Formos Med Assoc 2022;121:1062-72. [Crossref] [PubMed]
- Ahmad R, Mohanty B. Chronic kidney disease stage identification using texture analysis of ultrasound images. Biomed Signal Process Control 2021;69:102695. [Crossref]
- Kim DH, Ye SY. Classification of Chronic Kidney Disease in Sonography Using the GLCM and Artificial Neural Network. Diagnostics (Basel) 2021;11:864. [Crossref] [PubMed]
- Abbasian AA, Sattar AR, Abolghasemi J, Mohammadi A. Correlation between Kidney Function and Sonographic Texture Features after Allograft Transplantation with Corresponding to Serum Creatinine: A Long Term Follow-Up Study. J Biomed Phys Eng 2020;10:713-26. [PubMed]
- Lin Y, Khong PL, Zou Z, Cao P. Evaluation of pediatric hydronephrosis using deep learning quantification of fluid-to-kidney-area ratio by ultrasonography. Abdom Radiol (NY) 2021;46:5229-39. [Crossref] [PubMed]
- Smail LC, Dhindsa K, Braga LH, Becker S, Sonnadara RR. Using Deep Learning Algorithms to Grade Hydronephrosis Severity: Toward a Clinical Adjunct. Front Pediatr 2020;8:1. [Crossref] [PubMed]
- Cerrolaza JJ, Peters CA, Martin AD, Myers E, Safdar N, Linguraru MG. Quantitative Ultrasound for Measuring Obstructive Severity in Children with Hydronephrosis. J Urol 2016;195:1093-9. [Crossref] [PubMed]
- Zheng Q, Furth SL, Tasian GE, Fan Y. Computer-aided diagnosis of congenital abnormalities of the kidney and urinary tract in children based on ultrasound imaging data by integrating texture image features and deep transfer learning image features. J Pediatr Urol 2019;15:75.e1-7. [Crossref] [PubMed]
- Yin S, Peng Q, Li H, Zhang Z, You X, Liu H, Fischer K, Furth SL, Tasian GE, Fan Y. Multi-instance Deep Learning with Graph Convolutional Neural Networks for Diagnosis of Kidney Diseases Using Ultrasound Imaging. Uncertain Safe Util Machine Learn Med Imaging Clin Image Based Proced (2019) 2019;11840:146-54. [Crossref] [PubMed]
- Yin S, Peng Q, Li H, Zhang Z, You X, Fischer K, Furth SL, Fan Y, Tasian GE. Multi-instance Deep Learning of Ultrasound Imaging Data for Pattern Classification of Congenital Abnormalities of the Kidney and Urinary Tract in Children. Urology 2020;142:183-9. [Crossref] [PubMed]
- Wolff RF, Moons KGM, Riley RD, Whiting PF, Westwood M, Collins GS, Reitsma JB, Kleijnen J, Mallett S. PROBAST Group. PROBAST: A Tool to Assess the Risk of Bias and Applicability of Prediction Model Studies. Ann Intern Med 2019;170:51-8. [Crossref] [PubMed]
- Iqbal F, Pallewatte A, Wansapura J. Texture analysis of ultrasound images of chronic kidney disease. Colombo, Sri Lanka: 2017 Seventeenth International Conference on Advances in ICT for Emerging Regions (ICTer), 2017:1-5.
- Daniel M, Szymanik-Grzelak H, Sierdziński J, Podsiadły E, Kowalewska-Młot M, Pańczyk-Tomaszewska M. Epidemiology and Risk Factors of UTIs in Children-A Single-Center Observation. J Pers Med 2023;13:138. [Crossref] [PubMed]
- Damasio MB, Bodria M, Dolores M, Durand E, Sertorio F, Wong MCY, Dacher JN, Hassani A, Pistorio A, Mattioli G, Magnano G, Vivier PH. Comparative Study Between Functional MR Urography and Renal Scintigraphy to Evaluate Drainage Curves and Split Renal Function in Children With Congenital Anomalies of Kidney and Urinary Tract (CAKUT). Front Pediatr 2020;7:527. [Crossref] [PubMed]
- Rajpurkar P, Chen E, Banerjee O, Topol EJ. AI in health and medicine. Nat Med 2022;28:31-8. [Crossref] [PubMed]
- Kuppili V, Biswas M, Sreekumar A, Suri HS, Saba L, Edla DR, Marinho RT, Sanches JM, Suri JS. Extreme Learning Machine Framework for Risk Stratification of Fatty Liver Disease Using Ultrasound Tissue Characterization. J Med Syst 2017;41:152. [Crossref] [PubMed]
- Hwang YN, Lee JH, Kim GY, Jiang YY, Kim SM. Classification of focal liver lesions on ultrasound images by extracting hybrid textural features and using an artificial neural network. Biomed Mater Eng 2015;26:S1599-611. [Crossref] [PubMed]
- Torres HR, Queirós S, Morais P, Oliveira B, Fonseca JC, Vilaça JL. Kidney segmentation in ultrasound, magnetic resonance and computed tomography images: A systematic review. Comput Methods Programs Biomed 2018;157:49-67. [Crossref] [PubMed]
- Yang X, Li H, Liu L, Ni D. Scale-aware Auto-context-guided Fetal US Segmentation with Structured Random Forests. BIO Integration 2020;1:118-29. [Crossref]
- Reyes M, Meier R, Pereira S, Silva CA, Dahlweid FM, von Tengg-Kobligk H, Summers RM, Wiest R. On the Interpretability of Artificial Intelligence in Radiology: Challenges and Opportunities. Radiol Artif Intell 2020;2:e190043. [Crossref] [PubMed]
- World Health Organization. Geneva: Ethics and governance of artificial intelligence for health: WHO guidance; 2021 [cited 2022 Dec 27]. Available online: https://www.who.int/publications/i/item/9789240029200
- Wu Y, Guo Y, Ma J, Sa Y, Li Q, Zhang N. Research Progress of Gliomas in Machine Learning. Cells 2021; [Crossref] [PubMed]
- Castiglioni I, Rundo L, Codari M, Di Leo G, Salvatore C, Interlenghi M, Gallivanone F, Cozzi A, D'Amico NC, Sardanelli F. AI applications to medical images: From machine learning to deep learning. Phys Med 2021;83:9-24. [Crossref] [PubMed]
- Rivera SC, Liu X, Chan AW, Denniston AK, Calvert MJSPIRIT-AI and CONSORT-AI Working Group. Guidelines for clinical trial protocols for interventions involving artificial intelligence: the SPIRIT-AI Extension. BMJ 2020;370:m3210. [Crossref] [PubMed]
- Selbst AD, Barocas S. The intuitive appeal of explainable machines. Fordham Law Rev 2018;87:1085-139.
- Ghassemi M, Oakden-Rayner L, Beam AL. The false hope of current approaches to explainable artificial intelligence in health care. Lancet Digit Health 2021;3:e745-50. [Crossref] [PubMed]
- Saha A, Saha A, Mittra T. Performance measurements of machine learning approaches for prediction and diagnosis of chronic kidney disease (CKD). Bangkok, Thailand: Proceedings of the 2019 7th International Conference on Computer and Communications Management, 2019. ACM Digital Library, 2019:200-4.
- Alnazer I, Bourdon P, Urruty T, Falou O, Khalil M, Shahin A, Fernandez-Maloigne C. Recent advances in medical image processing for the evaluation of chronic kidney disease. Med Image Anal 2021;69:101960. [Crossref] [PubMed]
- Notohamiprodjo M, Dietrich O, Horger W, Horng A, Helck AD, Herrmann KA, Reiser MF, Glaser C. Diffusion tensor imaging (DTI) of the kidney at 3 tesla-feasibility, protocol evaluation and comparison to 1.5 Tesla. Invest Radiol 2010;45:245-54. [Crossref] [PubMed]