Identification of ischemia-causing lesions using coronary plaque quantification and changes in fractional flow reserve derived from computed tomography across the lesion
Introduction
Coronary computed tomography angiography (CCTA) has been widely used as a first-line method to evaluate intermediate-risk patients with acute chest pain and no known coronary artery disease (CAD) (1). However, there is often a disconnect between the degree of coronary stenosis and lesion-specific ischemia. Only about half of the obstructive lesions diagnosed by CCTA or invasive coronary angiography (ICA) will result in ischemia (2,3), and non-obstructive lesions can also result in ischemia (4-6). Following advances in technology, non-invasive fractional flow reserve (FFR) and plaque composition analyses, which do not require additional imaging, radiation exposure, or drug administration, can now be performed using conventional CCTA data for the simulation calculation.
FFR derived from computed tomography (CT) (FFRCT) was recently given a class 2a recommendation with a B-nonrandomized (NR) level of evidence (i.e., a moderate level of evidence from 1 or more non-randomized studies) for the evaluation of intermediate-risk patients with acute chest pain and known CAD (1). Compared to the computational fluid dynamics method, machine-learning (ML)-based FFRCT requires less computation time and power and has been proven to have good diagnostic performance (7,8). Recently, studies have found that the change in FFRCT across the lesion (ΔFFRCT) has higher diagnostic performance than FFRCT distal to the lesion and can improve patient management (9-11). The assessment of plaque characteristics based on CCTA is comparable to that of intravascular ultrasonography (IVUS) (12), and a previous study (6) showed that the former is related to the presence of ischemia. However, studies on the quantitative indicators of plaque characteristics that might cause ischemia are inadequate. We hypothesized that quantitative plaque characteristics and ΔFFRCT would enhance the diagnostic accuracy of CCTA in detecting ischemia. Thus, this study sought to explore the connections between the degree of coronary artery stenosis, quantitative coronary plaque characteristics, ΔFFRCT, and ischemia determined by the FFR, which is used as a reference standard. We present the following article in accordance with the STARD reporting checklist (available at https://qims.amegroups.com/article/view/10.21037/qims-22-1049/rc).
Methods
Study population
This retrospective study comprised patients with suspected or known stable CAD who underwent CCTA and FFR measurement within 1 week between January and October 2019 at our institution. To be eligible for inclusion in the study, the patients had to have at least 1 lesion with CCTA stenosis of between 30% and 90%. Patients were excluded from the study if they met any of the following exclusion criteria: (I) were aged <18 years; (II) had a previous myocardial infarction or coronary revascularization; (III) had multiple lesions per vessel; and/or (IV) had CCTA images that could not be analyzed.
This study was conducted in accordance with the Declaration of Helsinki (as revised in 2013). The study was reviewed and approved by the Ethics Committee of Fuwai Hospital, National Center for Cardiovascular Diseases (No. 2018-1076), and the requirement for written informed consent was waived due to the retrospective nature of the study.
CCTA acquisition
A second- or third-generation dual-source CT scanner (Definition Flash, Siemens Healthineers, Forchheim, Germany) was used for all the CCTA scans, and the images were collected according to the cardiovascular CT protocol (13). Beta-blockers were administered if a patient had a heart rate ≥70 beats/min. The scan parameters were as follows: tube voltage, 100 or 120 kV; tube current, automatic tube current modulation; section thickness, 0.75 mm; reconstruction increment, 0.7 mm; medium soft-tissue convolution reconstruction kernel (I26f); and image acquisition prospectively triggered to the patients’ electrocardiogram (ECG) at 35% to 75% of the R-R interval. The raw CT data were transferred to the offline workstation for further postprocessing after reconstruction using an algorithm optimized for ECG-gated reconstruction.
The calcification score (CS) was assessed by local investigators using the Agatston method (14). Stenosis severity was categorized as mild stenosis (30–49%), moderate stenosis (50–69%), or severe stenosis (70–90%) in coronary segments ≥2 mm by 2 experienced investigators who were blind to the patients’ conditions. Coronary obstructive stenosis was defined as stenosis ≥50%.
Quantitative coronary plaque analysis
Coronary segments ≥2 mm with a plaque area >1 mm2 were analyzed using semi-automated postprocessing software (QAngio CT Research Edition v3.0; Medis Medical Imaging Systems BV, Leiden, The Netherlands). The analyses were performed by 2 readers who did not know the FFR results of the patients, and the average values were used in the analysis. The scan-specific thresholds for low-attenuation plaque (LAP) [attenuation <30 Hounsfield units (HU)], intermediate-attenuation plaque (IAP) (attenuation between 30 and 130 HU), and calcified plaque (CP) (attenuation >130 HU) were automatically generated. The quantitative plaque components were automatically generated within the manually designated area. The plaque length, total plaque volume (TPV), and percentage aggregate plaque volume (%APV) had been measured previously. The %APV was computed using to following formula: %APV = (TPV/vessel volume) ×100%.
FFRCT analysis
The FFRCT computation was performed using ML-based software (cFFR 3.0, Siemens Healthineers). Detailed information on the basic principles of the FFRCT calculation used in this method have been reported previously (15). The value of FFRCT was measured within 2 cm distal to the lesion plaque, which was colocalized with the invasive FFR. ΔFFRCT was defined as the change in the FFRCT across the lesion by computing the difference between the proximal and distal FFRCT values as follows (9-11): ΔFFRCT = proximal FFRCT − distal FFRCT. To assess the reproducibility between the observers, 2 experienced radiologists measured the ΔFFRCT of 30 consecutive vessels without knowledge of the patients’ conditions.
ICA and FFR measurements
The angiography was performed and the FFR was determined according to standard practice (16) by senior cardiovascular physicians. The FFR was defined as the ratio of the pressure in the distal coronary artery to the pressure in the aorta during maximum hyperemia. The FFR pressure wire (PressureWire, St. Jude Medical, St. Paul, Minnesota) was positioned at approximately 2 cm distal to the stenosis in vessel segments ≥2 mm. A FFR at a threshold of ≤0.80 indicated that the vessel stenosis was hemodynamically significant and causal of lesion-specific ischemia.
Statistical analysis
The continuous variables are reported as either the mean ± standard deviation or the median with the interquartile range, while the categorical data are presented as the numbers and percentage. The intraclass correlation coefficient was used to evaluate the interobserver variability of ΔFFRCT to evaluate interobserver reproducibility. The Student’s t-test, Mann-Whitney U test, Kruskal-Wallis test, or chi-square test was used to compare the data as appropriate. To analyze the correlation between the FFR, plaque characteristics, and ΔFFRCT, a Spearman correlation analysis was used. The plaque variables and ΔFFRCT were dichotomized using an area under the curve (AUC) of the receiver operating characteristic (ROC) analysis and the Youden index, which was defined as the sum of the % sensitivity and % specificity minus 1, to determine the optimal thresholds for discriminating the FFR ≤0.80. Univariable and multivariable log-binomial regression analyses were conducted to estimate the relative risk factors of ischemia (FFR ≤0.80). Odds ratio (OR) estimates with 95% confidence intervals (CIs) were then used to evaluate the predictors of ischemia. To assess the incremental discrimination of ischemia, an AUC analysis was performed. Model 1 comprised CCTA stenosis ≥50%, Model 2 comprised Model 1 plus the independent risk factors for predicting ischemia, and Model 3 comprised Model 2 plus ΔFFRCT. The AUCs of the 3 models were compared using the method previously described by DeLong et al. (17), and the reclassification performance of each model was compared using relative integrated discrimination improvement (IDI) index and the category-free net reclassification index (NRI). All the statistical analyses were conducted using SPSS 25.0 (IBM Corp., Armonk, NY, USA), MedCalc 19.0.4 (MedCalc Software, Ostend, Belgium), and R 3.3.3 (R Foundation for Statistical Computing, Vienna, Austria) software. A two-tailed P value <0.05 was considered statistically significant.
Results
The selection process for the patients is shown in Figure 1. The study included a total of 144 patients, in whom 164 vessels were interrogated using the FFR [left anterior descending artery (LAD), 114 (69.5%); left circumflex artery (LCX), 26 (15.9%); and right coronary artery (RCA), 24 (14.6%)]. As Table 1 shows, the mean age of the patients included in the study was 56.8±9.1 years, and 111 (77.1%) of the patients were male. Lesion-specific ischemia was found in 49.4% (81/164) of the vessels belonging to 47.2% (68/144) of the patients, using the FFR as the reference, with a mean FFR value of 0.78±0.13. Of the 164 vessels measured by the FFR, 121 (73.8%) had obstructive stenosis on CCTA. A representative case is shown in Figure 2.
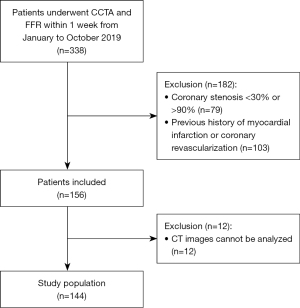
Table 1
Characteristics | Values |
---|---|
Number of patients, n | 144 |
Number of vessels, n | 164 |
Age, years | 56.8±9.1 |
Male | 111 (77.1) |
Body mass index, kg/m2 | 26.0 [23.9–28.4] |
Hypertension | 88 (61.1) |
Diabetes | 49 (34.0) |
Dyslipidemia | 120 (83.3) |
Current/past smoker | 78 (54.2) |
Family history of CAD | 18 (12.5) |
Vessel assessed | |
LAD | 114 (69.5) |
LCX | 26 (15.9) |
RCA | 24 (14.6) |
Vessel with CCTA maximum stenosis | |
Mild stenosis (30–49%) | 43 (26.2) |
Moderate stenosis (50–69%) | 54 (32.9) |
Severe stenosis (70–90%) | 67 (40.9) |
Invasive FFR | 0.78±0.13 |
Vessels with a FFR ≤0.80 | 81 (49.4) |
RCA with a FFR ≤0.80 | 7 (8.6) |
LAD with a FFR ≤0.80 | 66 (81.5) |
LCX with a FFR ≤0.80 | 8 (9.9) |
Heart rate, beats/min | 66 [59–75] |
DLP for CCTA, mGy·cm | 486.0 [371.0–665.5] |
Effective radiation dose for CCTA, mSv | 6.8 [5.2–9.3] |
The continuous variables are expressed as mean ± standard deviation or median [interquartile range], and the categorical variables are expressed as number (percentage). CAD, coronary artery disease; LAD, left anterior descending coronary artery; LCX, left circumflex coronary artery; RCA, right coronary artery; CCTA, coronary computed tomography angiography; FFR, fractional flow reserve; DLP, dose-length product.
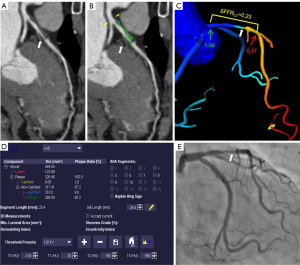
Relationship between the degree of CCTA stenosis and lesion-specific ischemia using FFR
Figure 3 shows the relationship between the degree of CCTA stenosis and the FFR. Among the 164 vessels interrogated using the FFR, 121 (73.8%) had obstructive stenosis on CCTA, with the LAD having the highest percentage of obstructive stenosis [81 (66.9%)], followed by the LCX [20 (16.5%)], and RCA [20 (16.5%)]. The FFR was found to be ≤0.80 in 72 (59.5%) vessels. Conversely, the FFR was ≤0.80 in only 9 (20.1%) vessels without obstructive stenosis, and the difference was statistically significant (P<0.001; Table 2). Among the 37 vessels with a FFR in the gray area (FFR, 0.71–0.80), 7 (18.9%), vessels did not have obstructive stenosis. As Table 3 shows, vessels with stenosis >50% showed a 5.6-fold increase in the probability of a FFR ≤0.80 compared to those with stenosis <50%.
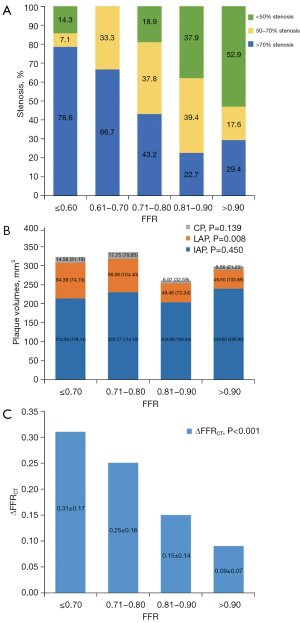
Table 2
Parameters | Overall (n=164) | CCTA ≥50% (n=121) | CCTA <50% (n=43) | ||||||||
---|---|---|---|---|---|---|---|---|---|---|---|
FFR ≤0.80 (n=81) | FFR >0.80 (n=83) | P | FFR ≤0.80 (n=72) | FFR >0.80 (n=49) | P | FFR ≤0.80 (n=9) | FFR >0.80 (n=34) | P | |||
Stenosis ≥50% | 72 (88.9) | 49 (59.0) | <0.001 | – | – | – | – | – | – | ||
LAP, mm3 | 89.3 (49.5–135.6) | 49.5 (25.3–104.0) | 0.001 | 89.5 (49.3–144.3) | 61.6 (35.5–125.5) | 0.083 | 89.3 (50.2–121.4) | 42.4 (20.29–65.2) | 0.012 | ||
IAP, mm3 | 225.2 (159.8–281.5) | 212.8 (142.3–272.7) | 0.474 | 222.0 (162.2–278.0) | 242.7 (164.8–294.0) | 0.334 | 246.7 (146.7–288.3) | 174.1 (125.8–224.6) | 0.118 | ||
CP, mm3 | 16.0 (3.2–45.2) | 7.7 (0.8–27.7) | 0.049 | 15.7 (2.2–45.7) | 5.9 (0.7–50.2) | 0.244 | 17.5 (8.2–53.0) | 8.5 (0.9–20.7) | 0.086 | ||
TPV, mm3 | 349.4 (275.0–441.0) | 294.6 (213.5–417.2) | 0.020 | 351.2 (259.9–446.7) | 339.8 (261.7–443.3) | 0.724 | 343.1 (316.4–406.4) | 236.8 (191.2–320.7) | 0.026 | ||
%APV, % | 33.2 (29.6–36.8) | 29.7 (26.5–34.0) | 0.001 | 33.4 (30.1–36.8) | 31.5 (28.1–36.6) | 0.164 | 30.3 (28.1–35.3) | 27.5 (24.6–31.0) | 0.057 | ||
Plaque length, mm | 30.5 (18.7–40.1) | 23.0 (13.9–28.7) | 0.001 | 29.3 (17.8–38.6) | 23.6 (15.5–33.8) | 0.092 | 36.6 (21.2–54.0) | 18.2 (10.6–27.7) | 0.007 | ||
CS | 43.3 (1.8–163.9) | 22.0 (0–104.0) | 0.111 | 41.7 (0.6–168.8) | 11.0 (0.0–123.7) | 0.258 | 98.0 (33.5–184.6) | 23.0 (3.0–66.8) | 0.053 | ||
ΔFFRCT | 0.28±0.17 | 0.14±0.13 | <0.001 | 0.29±0.17 | 0.16±0.14 | <0.001 | 0.25±0.16 | 0.10±0.09 | <0.001 |
The categorical variables are expressed as number (percentage), the continuous variables are expressed as median (interquartile range) or mean ± standard deviation. CCTA, coronary computed tomography angiography; FFRCT, fractional flow reserve derived from computed tomography; FFR, fractional flow reserve; LAP, low-attenuation plaque; IAP, intermediate-attenuation plaque; CP, calcified plaque; TPV, total plaque volume; %APV, percentage aggregate plaque volume; CS, calcification score.
Table 3
Predictors | Univariable analysis | Multivariable analysis | |||
---|---|---|---|---|---|
OR (95% CI) | P | OR (95% CI) | P | ||
Stenosis ≥50% | 5.551 (2.446–12.597) | <0.001 | 3.571 (1.437–8.878) | 0.006 | |
LAP ≥76.23 mm3 | 3.017 (1.594–5.711) | 0.001 | 2.347 (1.054–5.229) | 0.037 | |
IAP ≥175.4 mm3 | 1.582 (0.822–3.045) | 0.170 | – | – | |
CP ≥10.62 mm3 | 1.995 (1.072–3.714) | 0.029 | 1.242 (0.522–2.953) | 0.624 | |
TPV ≥282.57 mm3 | 2.703 (1.392–5.251) | 0.003 | 0.878 (0.366–2.106) | 0.770 | |
%APV ≥28.91% | 5.097 (2.409–10.783) | <0.001 | 2.893 (1.242–6.736) | 0.014 | |
Plaque length ≥28.69 mm | 3.138 (1.631–6.036) | 0.001 | 1.948 (0.875–4.338) | 0.103 | |
CS ≥135 | 2.346 (1.076–5.115) | 0.032 | 1.805 (0.651–5.010) | 0.257 | |
ΔFFRCT ≥0.14 | 12.859 (6.015–27.488) | <0.001 | – | – |
CCTA, coronary computed tomography angiography; FFR, fractional flow reserve; OR, odds ratio; CI, confidence interval; LAP, low-attenuation plaque; IAP, intermediate-attenuation plaque; CP, calcified plaque; TPV, total plaque volume; %APV, percentage aggregate plaque volume; CS, calcification score; FFRCT, fractional flow reserve derived from computed tomography.
Relationship between quantitative plaque characteristics using CCTA and lesion-specific ischemia using the FFR
As Figure 3 shows, the relationship between the volumes of LAP, IAP, and CP and the FFR was analyzed. The results showed that only the LAP volume differed significantly between the different FFR groups (P=0.008). However, there was no statistically significant difference in the IAP and CP volumes between the different FFR groups (P=0.450 and P=0.139, respectively). In this study, the volumes of LAP (R=−0.206 and P=0.008), %APV (R=−0.320, P<0.001), and plaque length (R=−0.284, P<0.001) were inversely related to the FFR; however, no significant correlations were found between the volumes of IAP (R=−0.022, P=0.777), CP (R=−0.142, P=0.071), CS (R=−0.092, P=0.239) and the FFR, and between the TPV (R=−0.108, P=0.168) and the FFR (Table 4). Table 2 shows the relationship between different quantitative plaque characteristics in relation to CCTA stenosis and FFR. In the overall group, the patients with a FFR ≤0.8 had higher volumes of LAP (89.3 vs. 49.5 mm3, respectively), CP (16.0 vs. 7.7 mm3, respectively) and TPV (349.4 vs. 294.6 mm3, respectively) than those with a FFR >0.8. The %APV was also higher in the patients with a FFR ≤0.8 compared to those with a FFR >0.8 (33.2 vs. 29.7, respectively). Additionally, the patients with a FFR ≤0.8 had a longer plaque length than those with a FFR >0.8 (30.5 vs. 23.0 mm, respectively). All of these differences were statistically significant (all P<0.05).
Table 4
Variables | R | P |
---|---|---|
FFR vs. LAP | −0.206 | 0.008 |
FFR vs. IAP | −0.022 | 0.777 |
FFR vs. CP | −0.142 | 0.071 |
FFR vs. TPV | −0.108 | 0.168 |
FFR vs. %APV | −0.320 | <0.001 |
FFR vs. plaque length | −0.284 | <0.001 |
FFR vs. CS | −0.092 | 0.239 |
FFR vs. ΔFFRCT | −0.524 | <0.001 |
FFR, fractional flow reserve; LAP, low-attenuation plaque; IAP, intermediate-attenuation plaque; CP, calcified plaque; TPV, total plaque volume; %APV, percentage aggregate plaque volume; CS, calcification score; FFRCT, fractional flow reserve derived from computed tomography.
The subgroup analysis revealed that in the stenosis <50% group, only the volumes of LAP and TPV and the plaque length were significantly higher in the patients with a FFR ≤0.8 compared to those with a FFR >0.8 (all P<0.05). Specifically, compared to those with a FFR >0.8, the patients with a FFR ≤0.8 had higher volumes of LAP (89.3 vs. 42.4 mm3, respectively) and TPV (343.1 vs. 236.8 mm3, respectively) and a longer plaque length (36.6 vs. 18.2 mm, respectively). However, in the patients with stenosis ≥50%, there were no statistically significant differences in any of the plaque characteristics between the 2 groups.
Table 3 sets out the optimal cut-off values for the different plaque characteristics to detect a FFR ≤0.80 as determined by the Youden index. The results of the univariate analysis indicated that stenosis ≥50% (OR, 5.551, 95% CI: 2.446–12.597, P<0.001), LAP ≥76.23 mm3 (OR, 3.017, 95% CI: 1.594–5.711, P=0.001), CP ≥10.62 mm3 (OR, 1.995, 95% CI: 1.072–3.714, P=0.029), TPV ≥282.57 mm3 (OR, 2.703, 95% CI: 1.392–5.251, P=0.003), the %APV ≥28.91% (OR, 5.097, 95% CI: 2.409–10.783, P<0.001), plaque length ≥28.69 mm (OR, 3.138, 95% CI: 1.631–6.036, P=0.001), and CS ≥135 (OR, 2.346, 95% CI: 1.076–5.115, P=0.032) were all related to FFR. After controlling for the degree of coronary stenosis, only LAP ≥76.23 mm3 (OR, 2.347, 95% CI: 1.054–5.229, P=0.037) and the %APV ≥28.91% (OR, 2.893, 95% CI: 1.242–6.736, P=0.014) remained significantly correlated with the FFR and predicted ischemia independently of the other plaque characteristics (Table 3).
Relationship between ΔFFRCT and lesion-specific ischemia using the FFR
The intraclass correlation coefficient of ΔFFRCT was 0.84 (95% CI: 0.69–0.92). Figure 3 displays the relationship between the ΔFFRCT and FFR values. As Table 4 shows, there was a significant negative correlation (R=−0.524, P<0.001) between the ΔFFRCT and FFR values. The mean ΔFFRCT was 0.21±0.17, and the optimal cut-off value for ΔFFRCT to detect FFR ≤0.80 was determined to be 0.14 based on the Youden index. An ΔFFRCT ≥0.14 was observed in 97 (59.1%) vessels. The mean ΔFFRCT according to the degree of coronary stenosis and FFR values is presented in Table 2. Notably, regardless of the degree of CCTA stenosis, patients with an invasive FFR ≤0.8 consistently had higher ΔFFRCT values than those with an invasive FFR >0.8.
Combined assessment of CCTA stenosis, plaque characteristics, and ΔFFRCT for diagnosing ischemia using the FFR
The AUC values (95% CI) for identifying ischemia (FFR ≤0.80) were 0.65 (0.57–0.72) for CCTA stenosis ≥50%, 0.63 (0.56–0.71) for LAP ≥76.23 mm3, 0.66 (0.58–0.73) for the %APV ≥28.91%, and 0.76 (0.68–0.82) for ΔFFRCT ≥0.14. Figure 4 displays the ROCs for the 3 models, and Table 5 presents the AUC, category-free NRI, and relative IDI values for the 3 models. Compared to Model 1, Model 2 had higher discriminant ability (AUC, 0.742 vs. 0.649, P=0.001) and higher reclassification ability (NRI, 0.339, P=0.027; relative IDI, 0.093, P<0.001) in the identification of ischemia. Model 3 had a higher discriminant ability (AUC, 0.828 vs. 0.742, P=0.004) and incremental reclassification ability (NRI, 1.029, P<0.001; relative IDI, 0.140, P<0.001) than Model 2.
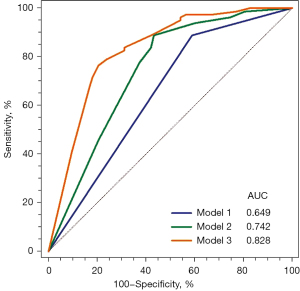
Table 5
Prediction model | AUC (95% CI) | Difference with previous model (95% CI) |
P | NRI (95% CI) | P | IDI (95% CI) | P |
---|---|---|---|---|---|---|---|
Model 1 | 0.649 (0.571–0.722) | – | – | – | – | – | – |
Model 2 | 0.742 (0.668–0.807) | 0.093 (0.036–0.150) | 0.001 | 0.339 (0.039–0.639) | 0.027 | 0.093 (0.048–0.138) | <0.001 |
Model 3 | 0.828 (0.762–0.883) | 0.086 (0.027–0.145) | 0.004 | 1.029 (0.773–1.285) | <0.001 | 0.140 (0.087–0.194) | <0.001 |
Model 1: CCTA stenosis ≥50%; Model 2: Model 1 + LAP ≥76.23 mm3 + %APV ≥28.91%; Model 3: Model 2 + ΔFFRCT ≥0.14. FFR, fractional flow reserve; AUC, area under the curve; CI, confidence interval; NRI, net reclassification index; IDI, integrated discrimination improvement; LAP, low-attenuation plaque; %APV, percentage aggregate plaque volume; FFRCT, fractional flow reserve derived from computed tomography; CCTA, coronary computed tomography angiography.
The discriminant and reclassification abilities of various combinations of LAP ≥76.23 mm3, %APV ≥28.91%, and ΔFFRCT ≥0.14 differed (Table 6). Among the 3 variables used in this study, ΔFFRCT ≥0.14 had the highest AUC and incremental reclassification ability when added to Model 1 as a single parameter. Compared to Model 1, the discriminant ability (AUC, 0.811 vs. 0.649, P<0.001) and reclassification ability (NRI, 1.101, P<0.001; relative IDI, 0.224, P<0.001) were improved when ΔFFRCT ≥0.14 was added to Model 1.
Table 6
Prediction Models | AUC (95% CI) | P* | NRI | P* | IDI | P* |
---|---|---|---|---|---|---|
Model 1 (stenosis ≥50%) | 0.649 (0.571–0.722) | – | – | – | – | – |
Model 1 + LAP | 0.701 (0.625–0.770) | 0.036 | 0.535 (0.241–0.829) | <0.001 | 0.038 (0.008–0.069) | 0.015 |
Model 1 + %APV | 0.719 (0.644–0.787) | 0.001 | 0.644 (0.379–0.908) | <0.001 | 0.067 (0.029–0.105) | <0.001 |
Model 1 + ΔFFRCT | 0.811 (0.743–0.868) | <0.001 | 1.101 (0.849–1.353) | <0.001 | 0.224 (0.160–0.288) | <0.001 |
Model 1 + LAP + %APV | 0.742 (0.668–0.807) | 0.001 | 0.339 (0.039–0.639) | 0.027 | 0.093 (0.048–0.138) | <0.001 |
Model 1 + LAP + ΔFFRCT | 0.824 (0.757–0.879) | <0.001 | 1.101 (0.849–1.353) | <0.001 | 0.239 (0.173–0.305) | <0.001 |
Model 1 + %APV + ΔFFRCT | 0.835 (0.769–0.889) | <0.001 | 1.101 (0.849–1.353) | <0.001 | 0.249 (0.182–0.315) | <0.001 |
Model 1 + LAP + %APV + ΔFFRCT | 0.828 (0.762–0.883) | <0.001 | 1.124 (0.873–1.376) | <0.001 | 0.234 (0.168–0.299) | <0.001 |
*, the AUC, NRI, and IDI were compared with those of Model 1. FFRCT, fractional flow reserve derived from computed tomography; AUC, area under the curve; CI, confidence interval; NRI, net reclassification index; IDI, integrated discrimination improvement; LAP, low-attenuation plaque; %APV, percentage aggregate plaque volume.
Discussion
The main findings of this study are as follows. First, patients with a FFR ≤0.8 had more severe stenosis, a higher LAP volume, CP volume, TPV, ΔFFRCT and %APV and a longer plaque length than those with a FFR >0.8. Second, the degree of coronary stenosis, plaque characteristics, such as the LAP volume and %APV, and ΔFFRCT predicted lesion-specific ischemia in the patients with suspected or known CAD. Finally, the addition of the LAP volume and %APV assessment improved the discriminant and reclassification abilities of the assessments compared to the stenosis evaluation alone, and the addition of information about ΔFFRCT further increased the discriminant and reclassification abilities of the assessments.
Similar to previous studies (2,6,18), we found that only 59.5% of vessels with obstructive stenosis had ischemia, 7% of vessels had ischemia without obstructive stenosis, and 18.9% of vessels with FFRs of 0.71 to 0.80 had no obstructive stenosis. Previous research has shown that a diagnosis of ischemia using the FFR is associated with a future adverse prognosis, and that revascularization guided by the FFR improves event-free survival (19-21). Thus, it is important to improve the diagnosis of ischemia in addition to stenosis using CCTA. Moreover, other non-invasive methods are urgently needed to enhance the diagnosis of ischemia. By providing a more accurate diagnosis of ischemia, clinicians can better identify patients who would benefit from revascularization and improve their long-term outcomes.
Consistent with the findings of Takagi et al. (9), our previous study (11) also showed ΔFFRCT had a higher diagnostic performance than FFRCT (AUC, 0.743 vs. 0.803, P<0.001, respectively). As a non-invasive alternative to IVUS, CCTA-based coronary plaque characteristics had been shown to be associated with ischemia by several non-invasive imaging studies (6,18,22). Gaur et al. (18) found that the addition of plaque characteristics and FFRCT assessments improved the ability of models to identify ischemia of ischemia compared to CCTA alone. However, the association between ΔFFRCT, plaque characteristics, and ischemia remains unclear. Thus, we sought to show that the addition of plaque characteristics and ΔFFRCT assessment would improve the ability of models to identify ischemia compared to the stenosis evaluation alone.
Similar to our findings, several studies have reported an association between the LAP volume or %APV and ischemia. Specifically, Gaur et al. (18) and Nakazato et al. (22) reported an association between the LAP volume, %APV, and ischemia. In addition to LAP and %APV, Park et al. (6) also found that lesion length and positive remodeling were predictors of ischemia. However, one study (23) found that plaque length, plaque composition, or positive remodeling could not be used to identify ischemia. The differences in the study results might be related to the differences in the software used for the plaque analysis. Different software might use different algorithms and criteria for determining plaque characteristics, which could lead to variations in measurements of plaque volume or %APV. Our study showed that by identifying specific thresholds for the quantitative plaque characteristics (i.e., a LAP ≥76.23 mm3 and %APV ≥28.91%), it was possible to predict ischemia. This finding enhances the clinical applicability of quantitative plaque characteristic analyses and provides evidence for the clinical application of such analyses. However, there are some differences between our results and those of a previous study (18), and the relationship between the quantitative plaque characteristics and FFR was not continuous in the subgroup analysis of this study, which might be related to the small sample size of this study.
LAP is the non-invasive alternative of CCTA when a necrotic core is present. Plaques with necrotic cores can lead to local oxidative stress and inflammation, which can not only improve the level of vasoconstrictors, such as isoprostanes, but also reduce the production and bioavailability of vasodilators, such as nitric oxide (24,25). This can result in local endothelial dysfunction and focal “functional stenosis,” which may explain the mismatch between the degree of stenosis and ischemia that occurs in some cases (24,26). In addition, plaques containing necrotic cores are considered the primary cause of cardiovascular events (27-29). Thus, the presence of necrotic cores is associated with ischemia, and the findings of our study support this association.
The results of this study also suggest that a comprehensive approach that combines CCTA stenosis assessment with quantitative plaque characteristics (e.g., the LAP volume and %APV) and ΔFFRCT computation could be a valuable non-invasive strategy for evaluating patients with stable CAD. This approach could serve as a gatekeeper to ICA, as it could improve the ability of models to identify and reclassify ischemia compared to the stenosis evaluation alone.
A combination of the 3 parameters was used in the primary analysis of our study; however, it should be noted that the role of each parameter in terms of the discriminant and reclassification abilities of the models in the identification of ischemia might differ. In this study, a simpler combination of Model 1 and ΔFFRCT had a significantly higher AUC (0.811 vs. 0.649, respectively) and reclassification ability (NRI, 1.101; relative IDI, 0.224, respectively) than Model 1 alone, and a simpler combination of Model 1 and ΔFFRCT was as effective as the addition of all parameters (AUC, 0.811 vs. 0.828; NRI, 1.101 vs. 1.124; IDI, 0.224 vs. 0.234, respectively). These results imply that ΔFFRCT has a greater effect on lesion-specific ischemia than the LAP volume and %APV. This simple model with ΔFFRCT has the potential to be used immediately in real-world practice to identify ischemia, as ΔFFRCT can be easily calculated in current clinical practice. However, further studies need to be conducted to determine the best parameter or combination of parameters in diverse patients and lesion subsets. Takagi et al. (9,10) found that ΔFFRCT has a higher diagnostic performance than that of CCTA and improves the clinical management of patients. Lee et al. (30) found that ΔFFRCT improved the identification of plaques that subsequently causes acute coronary syndrome (ACS). At the phenotypic level, ΔFFRCT has been associated with reduced myocardial blood flow, and our study results also reinforce these findings.
This study had some limitations. The FFR, which is a test of pressure loss that is poorly related to coronary flow, might be an imperfect reference standard for myocardial ischemia, and it does not have the necessary diagnostic or clinical characteristics to be used as a gold-standard reference test (31-34). The use of FFR might have affected the reliability of the results. The coronary flow reserve (CFR), which is the ratio of coronary blood during maximal vasodilation divided by that during resting conditions, appears to be a better reference standard for myocardial ischemia at an individual level (34). However, at present, the FFR is still widely used as a reference standard for many non-invasive imaging examinations (6,9,11,18,22,35). Accordingly, further studies urgently need to be conducted to investigate the associations between ΔFFRCT and lesion-specific ischemia using CFR as a reference standard. Similar to a previous study (36), the correlation between FFR and ΔFFRCT was moderate in our study, which suggests that further studies need to be conducted to identify more appropriate non-invasive alternative indicators in the future. Findings from previous studies of FFRCT and clinical outcomes have been inconsistent (37,38), and this study lacked clinical outcome data, and as such further clinical outcome studies still need to be conducted to analyze the effectiveness of these methods. This study was a retrospective, small-sample study. Thus, there may be potential selection bias in this study. Further, the relationship between stenosis severity and plaque characteristics was dose-dependent, which might have resulted in collinearity. The thresholds for plaque characteristics and ΔFFRCT were generated from the data of this study, and as such, optimal thresholds may differ in diverse populations. Finally, there are other indicators based on CCTA that can be used to identify high-risk plaque and predict ischemia, such as the fat attenuation index (39,40). Thus, further studies need to be conducted to determine the best parameter combination for identifying ischemia.
Conclusions
CCTA stenosis, quantitative plaque characteristics, and ΔFFRCT can be used to predict lesion-specific ischemia in patients with suspected or known CAD. The incorporation of quantitative plaque characteristics and ΔFFRCT into the assessment models improved the identification of ischemia compared to the stenosis assessment alone.
Acknowledgments
Funding: This study was supported by the Beijing Municipal Commission of Science and Technology (No. Z201100005620013, to Yang Gao and Bin Lu); the Clinical and Translational Medicine Research Foundation of the Chinese Academy of Medical Sciences (No. 2019XK320065, to Yang Gao and Bin Lu); the Ministry of Science and Technology of China, National Key Research and Development Project (No. 2016YFC1300402, to Yang Gao and Bin Lu); and the Subproject of ‘Double first-class’ Clinical Medicine Discipline Construction (No. 2019E-XK04-02, to Yang Gao and Bin Lu).
Footnote
Reporting Checklist: The authors have completed the STARD reporting checklist. Available at https://qims.amegroups.com/article/view/10.21037/qims-22-1049/rc
Conflicts of Interest: All authors have completed the ICMJE uniform disclosure form (available at https://qims.amegroups.com/article/view/10.21037/qims-22-1049/coif). YG and BL report grant support from the Beijing Municipal Commission of Science and Technology (No. Z201100005620013), the Clinical and Translational Medicine Research Foundation of the Chinese Academy of Medical Sciences (No. 2019XK320065), the Ministry of Science and Technology of China, National Key Research and Development Project (No. 2016YFC1300402), and the Subproject of the ‘Double First-Class’ Clinical Medicine Discipline Construction (No. 2019E-XK04-02). XY is an employee of Siemens Healthcare. The other authors have no conflicts of interest to declare.
Ethical Statement: The authors are accountable for all aspects of the work, including ensuring that any questions related to the accuracy or integrity of any part of the work have been appropriately investigated and resolved. This study was conducted in accordance with the Declaration of Helsinki (as revised in 2013). The study was reviewed and approved by the Ethics Committee of Fuwai Hospital, National Center for Cardiovascular Diseases (No. 2018-1076), and the requirement for written informed consent was waived due to the study’s retrospective nature.
Open Access Statement: This is an Open Access article distributed in accordance with the Creative Commons Attribution-NonCommercial-NoDerivs 4.0 International License (CC BY-NC-ND 4.0), which permits the non-commercial replication and distribution of the article with the strict proviso that no changes or edits are made and the original work is properly cited (including links to both the formal publication through the relevant DOI and the license). See: https://creativecommons.org/licenses/by-nc-nd/4.0/.
References
- Gulati M, Levy PD, Mukherjee D, Amsterdam E, Bhatt DL, Birtcher KK, et al. 2021 AHA/ACC/ASE/CHEST/SAEM/SCCT/SCMR Guideline for the Evaluation and Diagnosis of Chest Pain: A Report of the American College of Cardiology/American Heart Association Joint Committee on Clinical Practice Guidelines. Circulation 2021;144:e368-454.
- Meijboom WB, Van Mieghem CA, van Pelt N, Weustink A, Pugliese F, Mollet NR, Boersma E, Regar E, van Geuns RJ, de Jaegere PJ, Serruys PW, Krestin GP, de Feyter PJ. Comprehensive assessment of coronary artery stenoses: computed tomography coronary angiography versus conventional coronary angiography and correlation with fractional flow reserve in patients with stable angina. J Am Coll Cardiol 2008;52:636-43. [Crossref] [PubMed]
- Shaw LJ, Berman DS, Maron DJ, Mancini GB, Hayes SW, Hartigan PM, et al. Optimal medical therapy with or without percutaneous coronary intervention to reduce ischemic burden: results from the Clinical Outcomes Utilizing Revascularization and Aggressive Drug Evaluation (COURAGE) trial nuclear substudy. Circulation 2008;117:1283-91. [Crossref] [PubMed]
- Curzen N, Rana O, Nicholas Z, Golledge P, Zaman A, Oldroyd K, Hanratty C, Banning A, Wheatcroft S, Hobson A, Chitkara K, Hildick-Smith D, McKenzie D, Calver A, Dimitrov BD, Corbett S. Does routine pressure wire assessment influence management strategy at coronary angiography for diagnosis of chest pain?: the RIPCORD study. Circ Cardiovasc Interv 2014;7:248-55. [Crossref] [PubMed]
- Schuijf JD, Wijns W, Jukema JW, Atsma DE, de Roos A, Lamb HJ, Stokkel MP, Dibbets-Schneider P, Decramer I, De Bondt P, van der Wall EE, Vanhoenacker PK, Bax JJ. Relationship between noninvasive coronary angiography with multi-slice computed tomography and myocardial perfusion imaging. J Am Coll Cardiol 2006;48:2508-14. [Crossref] [PubMed]
- Park HB, Heo R, Ó Hartaigh B, Cho I, Gransar H, Nakazato R, Leipsic J, Mancini GBJ, Koo BK, Otake H, Budoff MJ, Berman DS, Erglis A, Chang HJ, Min JK. Atherosclerotic plaque characteristics by CT angiography identify coronary lesions that cause ischemia: a direct comparison to fractional flow reserve. JACC Cardiovasc Imaging 2015;8:1-10. [Crossref] [PubMed]
- Tesche C, De Cecco CN, Baumann S, Renker M, McLaurin TW, Duguay TM, Bayer RR 2nd, Steinberg DH, Grant KL, Canstein C, Schwemmer C, Schoebinger M, Itu LM, Rapaka S, Sharma P, Schoepf UJ, Coronary CT. Angiography-derived Fractional Flow Reserve: Machine Learning Algorithm versus Computational Fluid Dynamics Modeling. Radiology 2018;288:64-72. [Crossref] [PubMed]
- Coenen A, Kim YH, Kruk M, Tesche C, De Geer J, Kurata A, Lubbers ML, Daemen J, Itu L, Rapaka S, Sharma P, Schwemmer C, Persson A, Schoepf UJ, Kepka C, Hyun Yang D, Nieman K. Diagnostic Accuracy of a Machine-Learning Approach to Coronary Computed Tomographic Angiography-Based Fractional Flow Reserve: Result From the MACHINE Consortium. Circ Cardiovasc Imaging 2018;11:e007217. [Crossref] [PubMed]
- Takagi H, Ishikawa Y, Orii M, Ota H, Niiyama M, Tanaka R, Morino Y, Yoshioka K. Optimized interpretation of fractional flow reserve derived from computed tomography: Comparison of three interpretation methods. J Cardiovasc Comput Tomogr 2019;13:134-41. [Crossref] [PubMed]
- Takagi H, Leipsic JA, McNamara N, Martin I, Fairbairn TA, Akasaka T, et al. Trans-lesional fractional flow reserve gradient as derived from coronary CT improves patient management: ADVANCE registry. J Cardiovasc Comput Tomogr 2022;16:19-26. [Crossref] [PubMed]
- Yan H, Gao Y, Zhao N, Geng W, Hou Z, An Y, Zhang J, Lu B. Change in Computed Tomography-Derived Fractional Flow Reserve Across the Lesion Improve the Diagnostic Performance of Functional Coronary Stenosis. Front Cardiovasc Med 2022;8:788703. [Crossref] [PubMed]
- Dey D, Schepis T, Marwan M, Slomka PJ, Berman DS, Achenbach S. Automated three-dimensional quantification of noncalcified coronary plaque from coronary CT angiography: comparison with intravascular US. Radiology 2010;257:516-22. [Crossref] [PubMed]
- Abbara S, Blanke P, Maroules CD, Cheezum M, Choi AD, Han BK, Marwan M, Naoum C, Norgaard BL, Rubinshtein R, Schoenhagen P, Villines T, Leipsic J. SCCT guidelines for the performance and acquisition of coronary computed tomographic angiography: A report of the society of Cardiovascular Computed Tomography Guidelines Committee: Endorsed by the North American Society for Cardiovascular Imaging (NASCI). J Cardiovasc Comput Tomogr 2016;10:435-49. [Crossref] [PubMed]
- Agatston AS, Janowitz WR, Hildner FJ, Zusmer NR, Viamonte M Jr, Detrano R. Quantification of coronary artery calcium using ultrafast computed tomography. J Am Coll Cardiol 1990;15:827-32. [Crossref] [PubMed]
- Itu L, Rapaka S, Passerini T, Georgescu B, Schwemmer C, Schoebinger M, Flohr T, Sharma P, Comaniciu D. A machine-learning approach for computation of fractional flow reserve from coronary computed tomography. J Appl Physiol (1985) 2016;121:42-52. [PubMed]
- Pijls NH, van Schaardenburgh P, Manoharan G, Boersma E, Bech JW, van't Veer M, Bär F, Hoorntje J, Koolen J, Wijns W, de Bruyne B. Percutaneous coronary intervention of functionally nonsignificant stenosis: 5-year follow-up of the DEFER Study. J Am Coll Cardiol 2007;49:2105-11. [Crossref] [PubMed]
- DeLong ER, DeLong DM, Clarke-Pearson DL. Comparing the areas under two or more correlated receiver operating characteristic curves: a nonparametric approach. Biometrics 1988;44:837-45. [Crossref] [PubMed]
- Gaur S, Øvrehus KA, Dey D, Leipsic J, Bøtker HE, Jensen JM, Narula J, Ahmadi A, Achenbach S, Ko BS, Christiansen EH, Kaltoft AK, Berman DS, Bezerra H, Lassen JF, Nørgaard BL. Coronary plaque quantification and fractional flow reserve by coronary computed tomography angiography identify ischaemia-causing lesions. Eur Heart J 2016;37:1220-7. [Crossref] [PubMed]
- Bech GJ, De Bruyne B, Pijls NH, de Muinck ED, Hoorntje JC, Escaned J, Stella PR, Boersma E, Bartunek J, Koolen JJ, Wijns W. Fractional flow reserve to determine the appropriateness of angioplasty in moderate coronary stenosis: a randomized trial. Circulation 2001;103:2928-34. [Crossref] [PubMed]
- Tonino PA, De Bruyne B, Pijls NH, Siebert U, Ikeno F. van' t Veer M, Klauss V, Manoharan G, Engstrøm T, Oldroyd KG, Ver Lee PN, MacCarthy PA, Fearon WF; FAME Study Investigators. Fractional flow reserve versus angiography for guiding percutaneous coronary intervention. N Engl J Med 2009;360:213-24. [Crossref] [PubMed]
- De Bruyne B, Pijls NH, Kalesan B, Barbato E, Tonino PA, Piroth Z, et al. Fractional flow reserve-guided PCI versus medical therapy in stable coronary disease. N Engl J Med 2012;367:991-1001. [Crossref] [PubMed]
- Nakazato R, Shalev A, Doh JH, Koo BK, Gransar H, Gomez MJ, Leipsic J, Park HB, Berman DS, Min JK. Aggregate plaque volume by coronary computed tomography angiography is superior and incremental to luminal narrowing for diagnosis of ischemic lesions of intermediate stenosis severity. J Am Coll Cardiol 2013;62:460-7. [Crossref] [PubMed]
- Naya M, Murthy VL, Blankstein R, Sitek A, Hainer J, Foster C, Gaber M, Fantony JM, Dorbala S, Di Carli MF. Quantitative relationship between the extent and morphology of coronary atherosclerotic plaque and downstream myocardial perfusion. J Am Coll Cardiol 2011;58:1807-16. [Crossref] [PubMed]
- Ahmadi A, Kini A, Narula J. Discordance between ischemia and stenosis, or PINSS and NIPSS: are we ready for new vocabulary? JACC Cardiovasc Imaging 2015;8:111-4. [Crossref] [PubMed]
- Lavi S, Yang EH, Prasad A, Mathew V, Barsness GW, Rihal CS, Lerman LO, Lerman A. The interaction between coronary endothelial dysfunction, local oxidative stress, and endogenous nitric oxide in humans. Hypertension 2008;51:127-33. [Crossref] [PubMed]
- Ahmadi N, Ruiz-Garcia J, Hajsadeghi F, Azen S, Mack W, Hodis H, Lerman A. Impaired coronary artery distensibility is an endothelium-dependent process and is associated with vulnerable plaque composition. Clin Physiol Funct Imaging 2016;36:261-8. [Crossref] [PubMed]
- Stone GW, Maehara A, Lansky AJ, de Bruyne B, Cristea E, Mintz GS, Mehran R, McPherson J, Farhat N, Marso SP, Parise H, Templin B, White R, Zhang Z, Serruys PW. PROSPECT Investigators. A prospective natural-history study of coronary atherosclerosis. N Engl J Med 2011;364:226-35. [Crossref] [PubMed]
- Narula J, Nakano M, Virmani R, Kolodgie FD, Petersen R, Newcomb R, Malik S, Fuster V, Finn AV. Histopathologic characteristics of atherosclerotic coronary disease and implications of the findings for the invasive and noninvasive detection of vulnerable plaques. J Am Coll Cardiol 2013;61:1041-51. [Crossref] [PubMed]
- Motoyama S, Sarai M, Harigaya H, Anno H, Inoue K, Hara T, Naruse H, Ishii J, Hishida H, Wong ND, Virmani R, Kondo T, Ozaki Y, Narula J. Computed tomographic angiography characteristics of atherosclerotic plaques subsequently resulting in acute coronary syndrome. J Am Coll Cardiol 2009;54:49-57. [Crossref] [PubMed]
- Lee JM, Choi G, Koo BK, Hwang D, Park J, Zhang J, et al. Identification of High-Risk Plaques Destined to Cause Acute Coronary Syndrome Using Coronary Computed Tomographic Angiography and Computational Fluid Dynamics. JACC Cardiovasc Imaging 2019;12:1032-43. [Crossref] [PubMed]
- De Bruyne B, Bartunek J, Sys SU, Heyndrickx GR. Relation between myocardial fractional flow reserve calculated from coronary pressure measurements and exercise-induced myocardial ischemia. Circulation 1995;92:39-46. [Crossref] [PubMed]
- Pijls NH, De Bruyne B, Peels K, Van Der Voort PH, Bonnier HJ, Bartunek J, Koolen JJ, Koolen JJ. Measurement of fractional flow reserve to assess the functional severity of coronary-artery stenoses. N Engl J Med 1996;334:1703-8. [Crossref] [PubMed]
- van Nunen LX, Zimmermann FM, Tonino PA, Barbato E, Baumbach A, Engstrøm T, Klauss V, MacCarthy PA, Manoharan G, Oldroyd KG, Ver Lee PN, Van't Veer M, Fearon WF, De Bruyne B, Pijls NHFAME Study Investigators. Fractional flow reserve versus angiography for guidance of PCI in patients with multivessel coronary artery disease (FAME): 5-year follow-up of a randomised controlled trial. Lancet 2015;386:1853-60. [Crossref] [PubMed]
- Stegehuis VE, Wijntjens GW, Piek JJ, van de Hoef TP. Fractional Flow Reserve or Coronary Flow Reserve for the Assessment of Myocardial Perfusion: Implications of FFR as an Imperfect Reference Standard for Myocardial Ischemia. Curr Cardiol Rep 2018;20:77. [Crossref] [PubMed]
- Nous FMA, Geisler T, Kruk MBP, Alkadhi H, Kitagawa K, Vliegenthart R, et al. Dynamic Myocardial Perfusion CT for the Detection of Hemodynamically Significant Coronary Artery Disease. JACC Cardiovasc Imaging 2022;15:75-87. [Crossref] [PubMed]
- Celeng C, Leiner T, Maurovich-Horvat P, Merkely B, de Jong P, Dankbaar JW, van Es HW, Ghoshhajra BB, Hoffmann U, Takx RAP. Anatomical and Functional Computed Tomography for Diagnosing Hemodynamically Significant Coronary Artery Disease: A Meta-Analysis. JACC Cardiovasc Imaging 2019;12:1316-25. [Crossref] [PubMed]
- Patel MR, Nørgaard BL, Fairbairn TA, Nieman K, Akasaka T, Berman DS, et al. 1-Year Impact on Medical Practice and Clinical Outcomes of FFR(CT): The ADVANCE Registry. JACC Cardiovasc Imaging 2020;13:97-105. [Crossref] [PubMed]
- Hamilton MCK, Charters PFP, Lyen S, Harries IB, Armstrong L, Richards GHC, Strange JW, Johnson T, Manghat NE. Computed tomography-derived fractional flow reserve (FFR(CT)) has no additional clinical impact over the anatomical Coronary Artery Disease - Reporting and Data System (CAD-RADS) in real-world elective healthcare of coronary artery disease. Clin Radiol 2022;77:883-90. [Crossref] [PubMed]
- Chen X, Dang Y, Hu H, Ma S, Ma Y, Wang K, Liu T, Lu X, Hou Y. Pericoronary adipose tissue attenuation assessed by dual-layer spectral detector computed tomography is a sensitive imaging marker of high-risk plaques. Quant Imaging Med Surg 2021;11:2093-103. [Crossref] [PubMed]
- Dai X, Hou Y, Tang C, Lu Z, Shen C, Zhang L, Zhang J. Long-term prognostic value of the serial changes of CT-derived fractional flow reserve and perivascular fat attenuation index. Quant Imaging Med Surg 2022;12:752-65. [Crossref] [PubMed]