A deep learning model for the differential diagnosis of benign and malignant salivary gland tumors based on ultrasound imaging and clinical data
Introduction
Parotid tumors (PTs) are widely recognized as a rare group of tumors with heterogeneous cellular and tissue features. The incidence rate of salivary malignancies is estimated to be 4–135 cases per million people annually (1). A considerable proportion of PTs are benign, ranging from 75% to 85% (2,3). The World Health Organization (WHO) in 2017 recognized 11 and 22 epithelial subtypes of benign parotid gland tumors (BPGTs) and malignant parotid gland tumors (MPGTs), respectively (4). Prognosis, tendency to metastasize, and therapeutic approach vary between these histological types. For most BPGTs, superficial parotidectomy (SP) is adequate (5). By comparison, a relatively aggressive surgical approach, such as total parotidectomy (TP) with radiotherapy, is needed for MPGTs to prevent malignant transformation (6,7). Therefore, a precise preoperative diagnosis that differentiates BPGTs from MPGTs is of great significance in determining the most appropriate surgical treatment.
Fine needle aspiration cytology (FNAC) is a widely used cytodiagnostic method because of its relatively high sensitivity and specificity. However, FNAC is not always conclusive due to sampling disqualification and the substantial heterogeneity of PTs (8,9). Ultrasonography, computed tomography (CT), and magnetic resonance imaging (MRI) have been increasingly applied in the preoperative evaluation of PTs, including identifying the stage of tumor based on the tumor-node-metastasis (TNM) classification. However, CTs may not provide sufficient anatomic details and the exact delineation of the tumor may remain unclear due to its low soft tissue resolution. For these reasons, MRIs have been universally recognized to be superior to CTs in soft tissue differentiation and neural involvement, but its clinical application is limited by high costs, susceptibility to motion artifacts, and radiation exposure (8-10). Owing to its convenience, speed, cost-effectiveness, real-time results, and dynamics, conventional ultrasound (CUS) has always been regarded as the first-line visualization method for PT imaging. Certainly, the accuracy of a CUS diagnosis largely depends on the experience of the operator, and poor coherence among characteristics and standardization errors can result in significant variability.
Accumulating evidence suggests that this limitation can be overcome using artificial intelligence (AI) algorithms, particularly deep learning (DL), which is based on neural networks (NN) that mimic the human brain to identify patterns in huge datasets (11,12). Different DL architectures have been developed for different tasks, but convolutional neural networks (CNNs) are presently the most widespread DL architecture typology in medical imaging. CNNs are composed of numerous layers, including an input layer, an output layer, and multiple hidden layers between. Each layer processes a representation of the observed patterns based on the input data it receives from the layer below (13-15). Consequently, DL shows remarkable capability to perform more particularized analyses and integrate massive amounts of data at high speeds but low cost without explicit feature definition. In recent years, DL-based medical image diagnosis has gained wide application across multiple medical domains. For example, DL algorithms have been developed for the diagnosis of Alzheimer’s disease using flourine-18 fluorodeoxyglucose positron emission tomography (18F-FDG PET) of the brain (16), differential diagnosis of breast US lesions and lung CT nodules (17), acquisition of enhanced spatial detail from cardiac MRI data (18), and early detection of diseases such as skin malignancy and diabetic retinopathy (19,20).
Moreover, DL methods have been extensively utilized in CT and MRI image analysis for PTs, including differential diagnosis of BPGTs and MPGTs, TNM classification of PTs, and evaluation of prognosis (21-24). However, to the best of our knowledge, there is a paucity of data exploring the application of DL-based ultrasound (US) imaging analysis to differentially diagnose BPGT and MPGT. Hence, in this investigation, we established a DL-based US imaging diagnostic model and evaluated its clinical value in the preoperative differentiation of BPGT and MPGT. The performance of the model was compared with the results obtained by 3 radiologists. We present the following article in accordance with the Standards for Reporting Diagnostic accuracy studies (STARD) reporting checklist (available at https://qims.amegroups.com/article/view/10.21037/qims-22-950/rc).
Methods
Patients
A total of 266 patients, including 178 patients with BPGTs and 88 patients with MPGTs, were consecutively identified from the pathology database in The Third Affiliated Hospital of Kunming Medical University from January 2015 to March 2021. The inclusion criteria of the patients were as follows: (I) pathologically confirmed BPGT or MPGT; (II) having undergone preoperative US examination; (III) high-quality images without motion or artifacts were available and conducive to analysis. The exclusion criteria were as follows: (I) patients who had consented to medical treatment for the lesion before US examination, including surgery, transcatheter arterial chemoembolization, radiofrequency ablation, chemotherapy, radiotherapy, and targeted drug therapy; (II) inflammatory lesions; (III) incomplete medical records and laboratory tests related to the malignancy; and (IV) unsatisfactory image quality, including blurred images and incomplete lesion area.
Finally, the data for 173 patients were collated from the complete pathology database and used to randomly construct a training set (including 66 benign and 66 malignant samples) and a testing set (including 21 benign and 20 malignant samples). The recruitment pathway for patients is presented in Figure 1 and the distribution of tumors is detailed in Table 1.
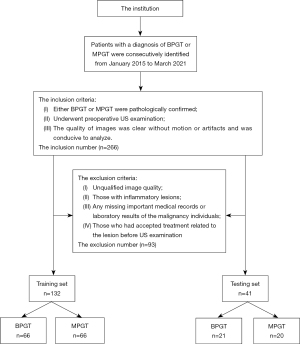
Table 1
Distribution of tumors | Number |
---|---|
Benign mass | |
Pleomorphic adenoma | 41 |
Warthin tumor | 26 |
Basal cell adenoma | 10 |
Hydatoncus | 10 |
Malignant mass | |
Mucoepidermoid carcinoma | 26 |
Adenocarcinoma | 25 |
Adenoid cystic carcinoma | 12 |
Acinic cell carcinoma | 15 |
Myoepithelial carcinoma | 4 |
Undifferentiated carcinoma | 4 |
US image acquisition and filtering
The US examinations were performed by radiologists with more than 5 years of experience in US diagnosis using various commercially available units, such as DC-8 (Mindray, Shenzhen, China), Logic E9 (GE), HD15 (Philips, Best, The Netherlands), and IU22 (Philips), equipped with a high-frequency linear array probe (6–14 MHz).
Image quality control
First, identifying patient information was removed from 2-dimensional (2D) US images of the parotid gland tumor obtained from the Picture Archiving and Communication Systems (PACS). Input images were then preprocessed by normalizing the grayscale of each image and reducing noise due to interference from the US machine. The same preprocessing steps were applied to the testing set. The 2D US grayscale images of the parotid gland tumor were embedded in the DL model for image processing and analysis, and the prediction results were recorded. Considering memory space and training time, we set the resolution of images to 224×224. This resolution was pre-trained in image net and could be performed by the default input resolution of network model.
DL model construction
In this paper, the stochastic gradient descent optimizer was used to update the parameters of the DL model, Binary cross-entropy loss was used as the loss function, and the learning rate was 0.01. We trained the model using a computer with a GeForce RTX 2060Ti (NVIDIA, Santa Clara, CA, USA) graphic processing unit and random-access memory of 16 GB.
To find the most suitable DL model for parotid data classification, we chose SqueezeNet as the model and compared the prediction performance of SqueezeNet with that of ResNet101, VGG16, and MobileNetV2. As can be seen in Table 2, both ResNet and VGG16 satisfied the needs of real-time diagnosis due to heavy calculation burden and long data prediction duration. In comparison, MobileNetV2, a lightweight network, has a shorter prediction time, but its prediction results were inferior to that of SqueezeNet (Table 2). Therefore, in this paper, SqueezeNet was selected as the eventual model to extract features from ultrasonic images and to identify BPGTs and MPGTs. SqueezeNet is a fully convolution network (FCN), without the full connection layer but with large parameters. Structurally, it is composed of convolutional layer, pooling layer, Fire module, and Softmax (Figure 2A). The Fire module adopts an idea similar to Inception and consists of 2 layers (Figure 2B): the squeeze layer that compresses the feature map through the convolutional layer of the 1×1 convolutional kernel and the expand layer that expands the feature map channel through the 1×1 and 3×3 convolutional layers and integrates them to generate the final feature map. The network involves many 1×1 convolution kernels, which speeds up the training rate of the model. Moreover, using a relevant model compression technology, a model 510 times smaller than AlexNet can be obtained without loss of accuracy.
Table 2
Methods | Accuracy | F1 | Kappa | Sensitivity | Specificity | Time (s) | Size (M) |
---|---|---|---|---|---|---|---|
SqueezeNet 1_1 | 0.9250 | 0.9248 | 0.8500 | 0.9666 | 0.8833 | 665 | 2.79 |
VGG 16 | 0.8750 | 0.8747 | 0.7499 | 0.8611 | 0.8889 | 795 | 512 |
MobileNet V2 | 0.8028 | 0.8018 | 0.6055 | 0.7499 | 0.8556 | 559 | 2.5 |
Resnet101 | 0.8117 | 0.8115 | 0.6459 | 0.8012 | 0.8229 | 1,005 | 171 |
M, megabyte.
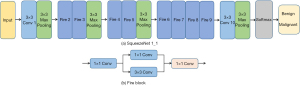
Annotation procedures
Among the 3 radiologists invited to annotate the images, there were 2 junior radiologists (doctor 1 and doctor 2) with 2 years of diagnosis experience in US and a senior doctor (doctor 3) with more than 5 years of diagnosis experience in US. All radiologists were blinded to the pathological results of the parotid gland tumor. They combined the American College of Radiology Thyroid Imaging Reporting and clinical data to discriminate benign from malignant parotid gland tumor. A detailed workflow of the study is shown in Figure 3.
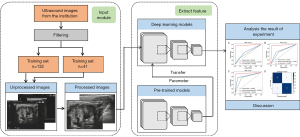
Statistical analysis
The receiver operator characteristic (ROC) curve was used to evaluate the discriminative power of the DL model for parotid gland tumor, as described by the sensitivity and specificity levels. Furthermore, the F1-score was calculated to measure the performance of the DL model. All statistical analyses were performed with SPSS 20.0 software (IBM Corp., Armonk, NY, USA), and a P value <0.05 was considered statistically significant.
The calculation formulas used were as follows:
where TP is true positive, indicating that the image is correctly classified by the classification algorithm; FN is false negative, indicating that the image is wrongly classified by the classification algorithm into other categories; TN is true negative, indicating that the classification algorithm correctly classifies non-category images into other categories; FP is false positive, indicating that the classification algorithm incorrectly classifies non-category images into such categories.
Ethical approval
This retrospective study was conducted in accordance with the Declaration of Helsinki (as revised in 2013) and approved by the Ethics Committee of Yunnan Cancer Hospital (No. KMMUIRB-282-21-03-R2). The data used in this research were collected as part of standard-of-care hospital routine. Written consent was provided by each patient before surgery or biopsy.
Results
Baseline characteristics
From January 2015 to March 2021, 508 US images were acquired from our institution for the training and testing sets, however, 50 images were removed from the 2 datasets based on the inclusion and exclusion criteria (Figure 1). The final dataset comprised 458 US images from 173 patients. The dataset for 41 patients was chosen as the testing set to evaluate our model. Baseline characteristics and clinicopathological information on tumor size and distribution are provided in Table 1.
A comparison of diagnostic accuracy between radiologists before and after combination with clinical data
Before combination with clinical data, the AUC of the senior radiologist (doctor 3, AUC =0.7500) was higher than that of the 2 junior radiologists (doctor 1, AUC =0.5750, doctor 2, AUC =0.6500; Figure 4). Furthermore, as can be seen in Table 3, the diagnostic accuracy of the senior radiologist was higher than that of the 2 junior radiologists (0.750 vs. 0.575 and 0.650, respectively).
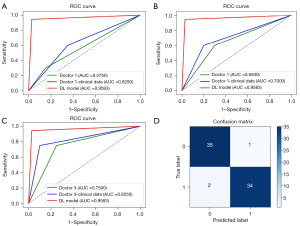
Table 3
Methods | Sensitivity | Specificity | Accuracy | F1-score |
---|---|---|---|---|
Doctor 1 | 0.850 | 0.300 | 0.575 | 0.540 |
Doctor 2 | 0.700 | 0.600 | 0.650 | 0.649 |
Doctor 3 | 0.750 | 0.750 | 0.750 | 0.750 |
DL model | 0.972 | 0.944 | 0.958 | 0.959 |
The detailed clinical data of the patients are shown in Table 4, including gender, age, body mass index (BMI), capsule, smoking history, drinking history, tumor location, distribution, shape, regularity, margin, density, cystic degeneration, and calcification. After combining the clinical data, the diagnostic accuracy of the senior radiologist was 0.825, and that of the 2 junior radiologists was 0.625 and 0.700, respectively (Table 5). These results demonstrated that effective combination with clinical data significantly improved the diagnostic results of radiologists (Figure 4).
Table 4
Clinical data | Training set (n=132) | Testing set (n=41) | |||||
---|---|---|---|---|---|---|---|
BPGT (n=66) | MPGT (n=66) | P1 | BPGT (n=21) | MPGT (n=20) | P2 | ||
Gender (M/F) | 41/25 | 35/31 | 0.907 | 9/11 | 11/9 | 0.943 | |
Age (year) | 46.17±23.83 | 46.62±28.38 | 0.848 | 44.86±22.86 | 64.35±26.65 | 0.019 | |
BMI | 23.48±6.97 | 22.80±8.34 | 0.507 | 23.75±9.4 | 22.84±8.39 | 0.510 | |
Smoking history (present/absent) | 18/48 | 19/47 | 0.682 | 7/14 | 5/15 | 0.883 | |
Drinking history (present/absent) | 12/54 | 17/49 | 0.848 | 3/18 | 3/17 | 0.203 | |
Tumor location (deep or shallow) | 64/2 | 58/8 | 0.201 | 19/2 | 15/5 | 0.010 | |
Distribution (single or bilateral) | 60/6 | 63/3 | 0.391 | 19/2 | 16/4 | 0.193 | |
Shape (round or not) | 64/2 | 31/35 | 0.050 | 21/0 | 6/14 | 0.036 | |
Capsule (present/absent) | 0/66 | 32/34 | 0.023 | 0/21 | 15/5 | 0.187 | |
Regularity(present/absent) | 60/6 | 27/39 | 0.890 | 17/4 | 5/15 | 0.368 | |
Margin (clear/unclear) | 65/1 | 35/31 | 0.972 | 21/0 | 6/14 | 0.752 | |
Density (low/middle/high/mixture/cystic) | 43/0/1/18/4 | 44/2/0/20/0 | 0.939 | 14/2/0/4/1 | 11/0/1/8/0 | 0.626 | |
Cystic degeneration (present/absent) | 40/20 | 47/19 | 0.911 | 16/5 | 11/9 | 0.908 | |
Calcification (present/absent) | 63/3 | 60/6 | 0.863 | 21/0 | 17/3 | 0.485 |
Categorical data are shown as numbers (n), numerical data are presented as mean ± standard deviation. M, male; F, female; BPGT, benign parotid gland tumor; MPGT, malignant parotid gland tumor; P1, the P value of comparison between benign and malignant parotid gland tumors in training set; P2, the P value of comparison between benign and malignant parotid gland tumors in testing set; BMI, body mass index.
Table 5
Methods | Sensitivity | Specificity | Accuracy | F1-score |
---|---|---|---|---|
Doctor 1 | 0.650 | 0.600 | 0.625 | 0.634 |
Doctor 2 | 0.800 | 0.600 | 0.700 | 0.727 |
Doctor 3 | 0.900 | 0.750 | 0.825 | 0.824 |
DL model | 0.972 | 0.944 | 0.958 | 0.959 |
A comparison of diagnostic accuracy between radiologists and the DL model
However, whether with or without clinical data, diagnostic results of the 3 radiologists were significantly lower than that of the DL model. The model demonstrated a tremendously high diagnostic performance for parotid gland tumor, achieving specificity, sensitivity, accuracy, and F1-score of 94.4%, 97.2%, 95.8%, and 95.9% in the testing set, respectively. Furthermore, as can be seen in Table 5, the diagnostic accuracy of this model (accuracy =0.958) was higher than of the 3 radiologists (doctor 1, accuracy =0.625, doctor 2, accuracy =0.700, doctor 3, accuracy =0.825). These results demonstrated DL model can effective differentiate benign and malignant parotid gland tumor.
The ROC curve predicted by the DL model using the testing set is illustrated in Figure 4. The model demonstrated a tremendously high diagnostic performance for parotid gland tumor, achieving specificity, sensitivity, accuracy, and F1-score of 94.4%, 97.2%, 95.8%, and 95.9% in the testing set, respectively. The 2-class confusion matrix is illustrated in Figure 4D. No major mistakes were observed in the DL model, with MPGTs rarely predicted as BPGTs.
After training, all US images in the testing set were predicted using the DL model for binary classification. As can be seen in the training process presented in Figure 5, with increasing steps, the accuracy of the DL model increased, but its loss decreased, achieving an accuracy of 96.5%. In this experiment, overfitting was not observed, and the accuracy of the training set was similar to that of the testing set.
Interpretability of the DL model
Surprisingly, in the gray scale US images, we found that the DL model could predict the parotid gland tumor state from 2 locations: the border of the tumor and the low echo area in the tumor body (Figure 6). This capability significantly illustrates the effectiveness of the DL model. Heatmaps were generated using the weight file from the training process (Figure 6). The results in Figure 6 show that the regions concentrated with the highest predictive value are highlighted in red and yellow, whereas those with weaker predictive values are expressed as green and blue. This suggested that the DL model focuses on the most predictive image features of parotid gland tumors.
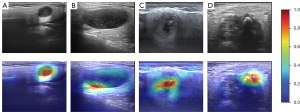
Discussion
The parotid gland is the largest salivary gland that is located in front of the ears on each side of the face. Most parotid gland neoplasms are benign but heterogeneous, and have the potential to recur and/or transform into malignant lesions. Therefore, accurate determination of the type of PT is critical for clinical diagnosis and subsequent treatment. In this study, we developed and validated a DL-based US imaging diagnostic model to provide a non-invasive tool for differentiating BPGT from MPGT. On testing, our transfer learning model exhibited good discriminative performance that exceeded the performance levels of 3 radiologists.
Our results showed that, after combining with clinical data, the DL model achieved an excellent result in the diagnosis of parotid gland tumor, with a significantly higher AUC value (AUC =0.9583) compared with that of doctor 1 + clinical data (AUC =0.6250), doctor 2 + clinical data (AUC =0.7250), and doctor 3 + clinical data (AUC =0.8250), respectively (all P<0.05). In addition, the sensitivity of this DL model was higher than that of US read by doctors in combination with clinical data (97.2% vs. 65%, 80%, and 90% for doctor 1 + clinical data, doctor 2 + clinical data, and doctor 3 + clinical data, respectively; all P<0.05). Similarly, the DL model showed higher specificity compared with that of doctors combined with clinical data (94.4% vs. 60%, 60%, and 75% for doctor 1 + clinical data, doctor 2 + clinical data, and doctor 3 + clinical data; all P<0.05). From these results, we concluded that the DL model can significantly improve a radiologist’s reading efficiency. Furthermore, the DL model, which can find lesions that are difficult to identify with the naked eye, can be a valuable tool to some radiologists with relatively little experience in diagnosis.
Based on the heatmaps generated from the DL model, we reasonably concluded that the DL model utilized tumor boundary information to efficiently discriminate benign from malignant tumors. However, the ability of the DL model to distinguish benign from malignant parotid gland tumors may be limited if the boundary information of the US image is blurred or disturbed. Meanwhile, other features of the US images may be ignored. In addition, although all the US images were collected by well-experienced doctors, there were still some differences in the quality of the images due to inconsistencies in diagnostic opinions and devices. Therefore, the prediction results obtained from the DL model should always be interpreted with caution.
The imaging representation of the tumors could present valuable additional information to support clinical diagnosis. However, the DL model may not entirely replace the evaluation of biopsies in the immediate future and much more data is needed before it can be adopted in clinical environments. The comparison of diagnostic performance between the doctors and the DL model showed that the DL model could provide a correct diagnosis, supporting its clinical application value.
As a novel non-invasive technique, AI exhibits superior pattern-recognition capabilities using imaging data and can provide a quantitative evaluation in an automated way. Several AI researchers have aimed to provide different AI-based strategies for obtaining valuable information to improve the diagnostic accuracy and early diagnosis of malignant tumors. Radiomics studies combined with DL techniques have been applied to differentiate benign and malignant parotid glands based on imaging data, such as MRI, CT, conventional US, sonoelastography, diffusion-weighted imaging, dynamic contrast-enhanced magnetic resonance imaging, and susceptibility-weighted imaging (23,25). Our results herein demonstrated that DL combined with US and clinical data could differentiate between BPGT and MPGT. In clinical practice, this technique may help decrease the incidence of false negative diagnoses, significantly improve the efficiency of film reading, and play an auxiliary role in diagnosis for some doctors with relatively limited experience.
However, there were some shortcomings in this study. First, for imaging diagnosis of parotid gland tumor, only a limited number of eligible patients was enrolled. With a total of only 176 patients and 458 US images from January 2015 to March 2021, this retrospective analysis may possess potential selection bias. Second, this was a single-center retrospective control study and the results should be verified in future large-scale multi-center trials. Lastly, DL analysis in this report was only developed with the US signature. Other image processing techniques, including gray-scale US features, pulsed doppler sonographic, contrast enhanced US, and sonoelastography features may improve the image quality and further improve the diagnosis results. Therefore, future studies should enroll more patients and image data to train the model for diagnosing different classes of parotid gland cancer and identify different imaging characteristics.
Conclusions
In spite of its intrinsic limitations and disadvantages, the DL model accurately identified and discriminated BPGT from MPGT. In practice, this US AI-based predictive DL model demonstrated superior diagnostic performance compared to specialized radiologists for the differential diagnosis of BPGT and MPGT. Interestingly, the results showed that the DL model had higher sensitivity and specificity in the diagnosis of BPGT and MPGT. Therefore, this model provides an ingenious method for highly accurate diagnosis of parotid tumors. However, a larger multi-center investigation is warranted to further evaluate the diagnostic ability of our DL model.
Acknowledgments
Funding: This study was supported by funding from the National Natural Science Foundation of China (No. 82160125 to ZCH and No. 82160347 to JFH); the Joint Special Funds for the Department of Science and Technology of Yunnan Province Kunming Medical University (No. 202201AY070001-168,170,136,041,160.202001AY070001-195); the Yunnan Provincial Department of Education Science Research Fund Project (Nos. 2020J0197, 2022J0235, 2021J0263, 2023Y0654 and 2019EF001 [-236]); the Science and Technology Innovation Team of Diagnosis and Treatment for Glucolipid Metabolic Diseases in Kunming Medical University (No. CXTD202106); and Yunnan Fundamental Research Projects (No. 202101AY070001-[171]).
Footnote
Reporting Checklist: The authors have completed the STARD reporting checklist. Available at https://qims.amegroups.com/article/view/10.21037/qims-22-950/rc
Conflicts of Interest: All authors have completed the ICMJE uniform disclosure form (available at https://qims.amegroups.com/article/view/10.21037/qims-22-950/coif). The authors report that this study received funding from the National Natural Science Foundation of China (No. 82160125 to ZCH and No. 82160347 to JFH); the Joint Special Funds for the Department of Science and Technology of Yunnan Province Kunming Medical University (No. 202201AY070001-168,170,136,041,160.202001AY070001-195); the Yunnan Provincial Department of Education Science Research Fund Project (Nos. 2020J0197, 2022J0235, 2021J0263, 2023Y0654 and 2019EF001 [-236]); the Science and Technology Innovation Team of Diagnosis and Treatment for Glucolipid Metabolic Diseases in Kunming Medical University (No. CXTD202106); and Yunnan Fundamental Research Projects (No. 202101AY070001-[171]). The authors have no other conflicts of interest to declare.
Ethical Statement: The authors are accountable for all aspects of the work in ensuring that questions related to the accuracy or integrity of any part of the work are appropriately investigated and resolved. The study was conducted in accordance with the Declaration of Helsinki (as revised in 2013). This retrospective research was approved by the Ethics Committee of Yunnan Cancer Hospital (No. KMMUIRB-282-21-03-R2). The data used in this research were collected as part of standard-of-care hospital routine. Written consent was provided by each patient before surgery or biopsy.
Open Access Statement: This is an Open Access article distributed in accordance with the Creative Commons Attribution-NonCommercial-NoDerivs 4.0 International License (CC BY-NC-ND 4.0), which permits the non-commercial replication and distribution of the article with the strict proviso that no changes or edits are made and the original work is properly cited (including links to both the formal publication through the relevant DOI and the license). See: https://creativecommons.org/licenses/by-nc-nd/4.0/.
References
- Hay AJ, Migliacci J, Karassawa Zanoni D, McGill M, Patel S, Ganly I. Minor salivary gland tumors of the head and neck-Memorial Sloan Kettering experience: Incidence and outcomes by site and histological type. Cancer 2019;125:3354-66. [Crossref] [PubMed]
- Bradley PJ, McGurk M. Incidence of salivary gland neoplasms in a defined UK population. Br J Oral Maxillofac Surg 2013;51:399-403. [Crossref] [PubMed]
- Tian Z, Li L, Wang L, Hu Y, Li J. Salivary gland neoplasms in oral and maxillofacial regions: a 23-year retrospective study of 6982 cases in an eastern Chinese population. Int J Oral Maxillofac Surg 2010;39:235-42. [Crossref] [PubMed]
- Slootweg PJ, El-Naggar AK. World Health Organization 4th edition of head and neck tumor classification: insight into the consequential modifications. Virchows Arch 2018;472:311-3.
- Kadletz L, Grasl S, Grasl MC, Perisanidis C, Erovic BM. Extracapsular dissection versus superficial parotidectomy in benign parotid gland tumors: The Vienna Medical School experience. Head Neck 2017;39:356-60. [Crossref] [PubMed]
- Grégoire V, Jeraj R, Lee JA, O'Sullivan B. Radiotherapy for head and neck tumours in 2012 and beyond: conformal, tailored, and adaptive? Lancet Oncol 2012;13:e292-300. [Crossref] [PubMed]
- Lewis AG, Tong T, Maghami E. Diagnosis and Management of Malignant Salivary Gland Tumors of the Parotid Gland. Otolaryngol Clin North Am 2016;49:343-80. [Crossref] [PubMed]
- Son E, Panwar A, Mosher CH, Lydiatt D. Cancers of the Major Salivary Gland. J Oncol Pract 2018;14:99-108. [Crossref] [PubMed]
- Lee YY, Wong KT, King AD, Ahuja AT. Imaging of salivary gland tumours. Eur J Radiol 2008;66:419-36. [Crossref] [PubMed]
- Maraghelli D, Pietragalla M, Cordopatri C, Nardi C, Peired AJ, Maggiore G, Colagrande S. Magnetic resonance imaging of salivary gland tumours: Key findings for imaging characterisation. Eur J Radiol 2021;139:109716. [Crossref] [PubMed]
- Li M, Wan C. The use of deep learning technology for the detection of optic neuropathy. Quant Imaging Med Surg 2022;12:2129-43. [Crossref] [PubMed]
- Lin H, Xiao H, Dong L, Teo KB, Zou W, Cai J, Li T. Deep learning for automatic target volume segmentation in radiation therapy: a review. Quant Imaging Med Surg 2021;11:4847-58. [Crossref] [PubMed]
- Hosny A, Parmar C, Quackenbush J, Schwartz LH, Aerts HJWL. Artificial intelligence in radiology. Nat Rev Cancer 2018;18:500-10. [Crossref] [PubMed]
- Esteva A, Robicquet A, Ramsundar B, Kuleshov V, DePristo M, Chou K, Cui C, Corrado G, Thrun S, Dean J. A guide to deep learning in healthcare. Nat Med 2019;25:24-9. [Crossref] [PubMed]
- Miotto R, Wang F, Wang S, Jiang X, Dudley JT. Deep learning for healthcare: review, opportunities and challenges. Brief Bioinform 2018;19:1236-46. [Crossref] [PubMed]
- Ding Y, Sohn JH, Kawczynski MG, Trivedi H, Harnish R, Jenkins NW, Lituiev D, Copeland TP, Aboian MS, Mari Aparici C, Behr SC, Flavell RR, Huang SY, Zalocusky KA, Nardo L, Seo Y, Hawkins RA, Hernandez Pampaloni M, Hadley D, Franc BL. A Deep Learning Model to Predict a Diagnosis of Alzheimer Disease by Using (18)F-FDG PET of the Brain. Radiology 2019;290:456-64. [Crossref] [PubMed]
- Cheng JZ, Ni D, Chou YH, Qin J, Tiu CM, Chang YC, Huang CS, Shen D, Chen CM. Computer-Aided Diagnosis with Deep Learning Architecture: Applications to Breast Lesions in US Images and Pulmonary Nodules in CT Scans. Sci Rep 2016;6:24454. [Crossref] [PubMed]
- Masutani EM, Bahrami N, Hsiao A. Deep Learning Single-Frame and Multiframe Super-Resolution for Cardiac MRI. Radiology 2020;295:552-61. [Crossref] [PubMed]
- Schmidt-Erfurth U, Sadeghipour A, Gerendas BS, Waldstein SM, Bogunović H. Artificial intelligence in retina. Prog Retin Eye Res 2018;67:1-29. [Crossref] [PubMed]
- Yu KH, Beam AL, Kohane IS. Artificial intelligence in healthcare. Nat Biomed Eng 2018;2:719-31. [Crossref] [PubMed]
- Hague C, McPartlin A, Lee LW, Hughes C, Mullan D, Beasley W, Green A, Price G, Whitehurst P, Slevin N, van Herk M, West C, Chuter R. An evaluation of MR based deep learning auto-contouring for planning head and neck radiotherapy. Radiother Oncol 2021;158:112-7. [Crossref] [PubMed]
- Xia X, Feng B, Wang J, Hua Q, Yang Y, Sheng L, Mou Y, Hu W. Deep Learning for Differentiating Benign From Malignant Parotid Lesions on MR Images. Front Oncol 2021;11:632104. [Crossref] [PubMed]
- Park J, Lee JS, Oh D, Ryoo HG, Han JH, Lee WW. Quantitative salivary gland SPECT/CT using deep convolutional neural networks. Sci Rep 2021;11:7842. [Crossref] [PubMed]
- Chang YJ, Huang TY, Liu YJ, Chung HW, Juan CJ. Classification of parotid gland tumors by using multimodal MRI and deep learning. NMR Biomed 2021;34:e4408. [Crossref] [PubMed]
- Matsuo H, Nishio M, Kanda T, Kojita Y, Kono AK, Hori M, Teshima M, Otsuki N, Nibu KI, Murakami T. Diagnostic accuracy of deep-learning with anomaly detection for a small amount of imbalanced data: discriminating malignant parotid tumors in MRI. Sci Rep 2020;10:19388. [Crossref] [PubMed]