Comparative study of the quantitative accuracy of oncological PET imaging based on deep learning methods
Introduction
In terms of the detection, treatment, and follow-up of cancer, positron emission tomography (PET) is a sensitive, noninvasive imaging method that provides molecular-level metabolic information and fine anatomical morphological information of lesions (1-4). In clinical practice, the activity and acquisition time of injected radiopharmaceuticals are often limited by safety, patient tolerance, or compliance. Reducing acquisition time may positively impact patient comfort and increase patient throughput in the Division of Nuclear Medicine. Children, healthy volunteers, and cancer patients should receive lower doses of the tracer to reduce radiation exposure, as well as when multiple follow-up scans are performed and different tracers are used to monitor the progress of treatment. However, reducing the injection dose/acquisition time can increase image noise, reduce the signal-to-noise ratio (SNR), and increase potentially unnecessary artifacts, thus affecting diagnostic and quantitative accuracy.
In recent years, large datasets have been made available, optimization algorithms have advanced, and efficient network structures have emerged, which have been widely used to segment images (5), detect lesions (6), and restore image super-resolution in computer vision tasks (7). Medical imaging has recently benefited from deep learning-based reconstruction methods (8-11), such as using convolutional neural networks (CNNs) or generative adversarial networks (GANs) to denoise and reduce artifacts in images and improve the SNR of low-dose PET (LDPET) images. Deep learning has proven remarkably effective in predicting full-dose PET (FDPET) images from either LDPET or ultra-low-dose PET (ULDPET) images. Kaplan and Zhu suggested reconstructing FDPET images from 1/10th-dose PET images using residual CNN preserved image edges and metabolic parameters (12). By using LDPET sinograms as 3D Unet inputs, Sanaat reduced the bias of quantitative parameters in 83 brain regions using full-dose sinograms (13). Using 3D conditional generative adversarial networks (3D C-GANs), Wang et al. designed an end-to-end approach to predict high-dose PET images from the corresponding LDPET images (14). Ouyang et al. used GAN to generate PET images of amyloid plaques of high quality and simultaneously applied contrary learning, analyzing features, and perceptual impairments specific to particular tasks to considerably improve high-quality PET images (15). According to our research, PET image noise can be reduced, and image quality can be improved using deep learning. Different network structure frameworks have different absolute quantitative accuracy and visual image quality (16), but the impact on clinical diagnosis of tumors and quantitative accuracy of lesions remains to be explored.
According to our literature investigation, we found that the improved model based on CNN network is more used in PET image recovery for tumor lesions at present (17). Since PET images are gray images with relatively simple semantic meaning and fixed structure, high-level semantic information and low-level features are very important. CNN represented by Unet contains Skip connection and U-shaped structure, which can well retain low-level features and high-level features. The multi-layer continuous image input can provide enough information to distinguish the noise from the organization structure. In the literature of Lu et al. (16), it is confirmed that 3D Unet has the best quantitative performance compared with 2D and 2.5D Unet, so we choose 3D Unet to represent CNN. GAN network is an unsupervised deep learning Model. It generates satisfactory input results through the game learning of two types of Generative Model (generative model and Discriminative model) in the framework. In medical image reconstruction, segmentation, registration and other work, most work is based on Pixel2Pixel (P2P) GAN frame and Cycle GAN frame, P2P GAN has been applied in CT noise reduction, MR Reconstruction and PET noise reduction (18). Therefore, in this study, P2P was chosen to represent GAN.
In this study, we systematically compared two deep learning reconstruction models based on 3D Unet and Pix2Pix GAN to predict FDPET tumor images from LDPET images. The original LDPET (1/6) and (1/12) ULDPET images were used as input data to the 3D Unet and Pix2Pix GAN networks, respectively, to compare the effectiveness of noise reduction and accuracy in the quantitative measurements of the two deep learning models and explore their clinical applicability.
Methods
Patient clinical data collection
In total, 311 tumor patients (205 male and 106 female) who underwent PET/CT scanning in the Department of Nuclear Medicine at the First Hospital of Shanxi Medical University from March 2020 to December 2021 were included in this study. The tumor types of the included cases are shown in Figure 1. Their ages ranged between 20–90 years, with the mean patient age of 61±13 years. This study was conducted in accordance with the principles of the Declaration of Helsinki (as revised in 2013). The study protocol was approved by the Ethics Committee of First Hospital of Shanxi Medical University (No. K-GK001) and informed consent was taken from all individual participants.
PET/CT scanning protocol and reconstruction methods
The patients were scanned using the Discovery MI PET/CT apparatus (GE Healthcare, USA), and 18F-FDG was produced by the HM-10 cyclotron and 18F-FDG synthesis module of the Sumitomo Corporation of Japan (radiochemical purity >99%). The patients fasted for 4 to 6 hours before the examination, and their blood sugar levels were within the normal range. They were intravenously injected with 18F-FDG 3.7 MBq/kg according to their body weight, and then rested for 50–70 minutes after the injection. Scanning was performed from the base of the skull to the middle of the femur. The CT scanning parameters were as follows: tube voltage 120 kV, tube current 60–150 mA (automatic adjustment in x-y direction/z direction), noise index 18, pitch 0.984:1, slice thickness 2.75 mm, rotation time 0.5 s; PET scan in list mode, 3 min/bed, 5–7 beds. The patients were breathing with normal tidal airflow. Select PET list mode data for image reconstruction. The reconstruction algorithm was TOF (time of flight)-PSF (point spread function)-BSREM (block sequential regularized expectation maximization) with penalty factor β=350 (19). Images collected for 3 min per bed were regarded as full dose PET (FD-PET). Low-dose imaging was selected for the first 15 and 30 s of each bed collection (92% reduction and 87% reduction), which were denoted as 8%PET and 17%PET, respectively. At the same time, the pre-90s of each bed collection time was selected as the clinical Standard protocol collection, which was recorded as standard PET (s-PET).
Deep learning reconstruction methods
3D Unet model
- Preprocessing: perform a normalization operation with a mean value of 0 and a variance of 1 on the PET image taken at a low dose and uniformly crop the image to a size of 256×256×64.
- Model structure: the entire model structure is shown in Figure 2. The 3D Unet was proposed based on the improvement in the 3D Unet (20). It includes an encoding structure for capturing semantics and a coding structure for an accurately positioned decoding structure. The entire model uses an LDPET image with a size of 256×256×64 as input, extracts image semantic features through an “encoding structure” constructed by three downsampling layers, uses ReLU as an activation function, and then constructs through three upsampling layers. The 3D Unet model is an improved version of the traditional 3D Unet model in the following three aspects:Figure 2 Process diagram of the 3D Unet model. Conv, convolution; BN, batch normalization; ReLU, rectified linear unit; 3D Unet, a deep network model based on CNN; CNN, convolutional neural network.
- In the encoding structure, the traditional 3D Unet model applies a max pooling layer to downsample the image, whereas the 3D Unet model uses a convolution operation with a 3×3×3 convolution kernel and stride of 2 to replace the max pooling layer.
- In the decoding structure, the 3D Unet model applies transposed convolution to upsample the image, whereas the 3D Unet uses bilinear interpolation instead of transposed convolution to remove potential checkerboard artifacts.
- In the traditional 3D Unet, the downsampled feature image and upsampled feature image are spliced through concatenation. In the 3D Unet, two feature images are directly added.
- Loss function: losses are calculated using the mean squared error (MSE), which is calculated as follows: where M represents the number of pixels, m represents the mth pixel, and X and Y represent the reconstructed and labeled images of the model, respectively.
- Post-processing: the image predicted by the model was stitched to restore the original image size, and the normalized pixel values were mapped back to the data range of the original image, according to the mean and variance.
P2P model
- Model structure: the overall model structure design is based on the P2P framework (21):
- The PET image generation network adopts an adjusted Unet network. The Unet encoder is downsampled three times, for a total of eight downsamplings. Furthermore, the decoder part is upsampled three times in accordance with the high-level semantic feature map of the encoder, which comprises more low-level and multi-dimensional picture features for multi-scale prediction, and skip connection is employed at the same stage.
- Obtain the actual collected image (3 min) scanned for a long time and the image discriminant model reconstructed by the model. Further, input the real image and generated image into the discrimination network in a different sequence, such as [real image generated image], the corresponding discrimination label is (1, 0). A discriminant network was constructed by stacking multilayer CNN convolution layers. After multiple convolution downsampling, the obtained features were straightened, and the discriminative probability of the real 3 min image and the generated 3 min image were predicted through two fully connected layers (Figure 3).
- Loss function.
- The loss function in the training stage of model generation is based on the L1 loss (regression loss function), as shown in the following formula: where yi_predictrepresents the ith layer image reconstructed by the generative model; yirepresents the real voxel value, corresponding to layer i, after normalization of the image; αi represents the weight of the layer-i image; n represents the number of layers in the image.
- Bidirectional cross-entropy loss functions were used in the training stage of the discriminant models, as shown in the formula: where P indicates the label (P=0 indicates that the image is generated by the model, P=1 indicates that the image is derived from the real image), and Q indicates the probability predicted by the discriminant model.
- Final loss function obtained from the generation and discriminant models: When the image output from the generated model is closer to the real image, the loss of the generated model G is increasingly smaller, whereas the loss of the discriminant model D is greater, thus realizing the optimization process of training.
- Image post-processing stage: all the slices predicted by the network are spliced together in order, and the resultant output in the range of 0–1 is mapped back to the data range of the PET image according to the maximum and minimum values of the original input data.
Training set and test set
In this study, 311 patients were randomly divided into training and test groups in the ratio of 7:3, with 218 patients used for training and 93 for testing; 8%PET and 17%PET raw data, respectively, were used as inputs to train the two models.
Visual assessment of PET image quality
The quality of PET images in the deep learning, 8%PET, 17%PET, and s-PET groups was evaluated by two experienced PET/CT physicians (with 5 and 8 years of experience in PET/CT diagnosis, respectively) without knowledge of image acquisition, reconstruction methods, and clinical information. Visual scoring was performed on a 1–5 scale (22): 1, unacceptable; 2, acceptable (subdiagnostic); 3, average; 4, good; and 5, excellent. When there was a difference between the two physicians, an additional score was assigned by a third physician.
Semi-quantitative analysis of PET images
AK software (Artificial Intelligent Kit, GE Healthcare, China) was used to delineate the spherical volume of interest (VOI) and measure the quantitative parameters of the liver background and tumor lesions in each group of images. On the CT tomographic images, measurements were conducted in the right liver lobe using a 3 cm VOI. The software automatically measured the maximum standardized uptake value (SUVmax), mean standardized uptake value (SUVmean), and standard deviation (SD) in the VOI and calculated the SNR in the liver [SNR = liver SUVmean/SD] (23). Similarly, an automated software program measured the SUVmax and SUVmean of 18F-FDG high-uptake tumor lesions by manually defining the VOIs on the CT images. The median diameter of the tumor lesions was 2.14 cm (1.65–3.94 cm), and the liver was used as the background to calculate the SNR in tumor lesions (SNR = SUVmax/liver SD) and the contrast-noise ratio (CNR) in tumor lesions [CNR = (lesion SUVmean − liver SUVmean)/liver SD] (24).
Statistical analysis
Data analysis was performed using IBM SPSS 22.0, and measurements with non-normal distributions were expressed as medians (P25, P75). The reconstruction data with 17%PET raw data as input were defined as 3D Unet-30s and P2P-30s groups, and the reconstruction data with 8%PET raw data as input were also defined as 3D Unet-15s and P2P-15s groups. The background of s-PET group images and quantitative parameters of tumor lesions were used as reference standards to evaluate the quality of the reconstructed image and its quantitative accuracy. The kappa test was used to analyze the agreement between the visual scores of the two physicians. The chi-square test was used to analyze the differences in the proportion of cases in the visual score of PET images among the groups. Multiple group comparisons were conducted using the Kruskal Wallis H test with Bonfferoni correction, whereas pairwise comparisons were conducted using the Wilcoxon signed-rank test. Statistical significance set at P<0.05 was regarded as consistent.
Results
Visual assessment of PET image quality
The visual scores of the two physicians for the 3D Unet-15s/3D Unet-30s and P2P-15s/-30s groups and between groups (8%PET, 17%PET, and s-PET) were highly consistent, with a kappa value of 0.719 (95% CI: 0.697–0.741), P<0.001). Image visual scores of 236 subjects in the 3D Unet-15s group were ≥3 points, compared with 303 subjects in the 3D Unet-30s group. A total of 280 patients in the P2P-15s and P2P-30s groups had visual scores ≥3, while 1,130,303 patients in the 8%PET, 16%PET and s-PET groups had visual scores ≥3, respectively. Statistically significant differences were found in the composition ratio of visual scores between groups (χ2=1,252.81 and 1,286.98, respectively, P<0.001 for both groups) (Figure 4), and the images of typical cases are shown in Figure 5 and Figure 6.
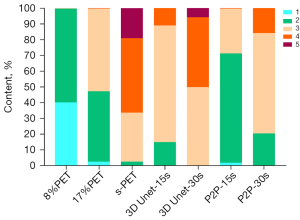
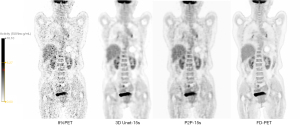
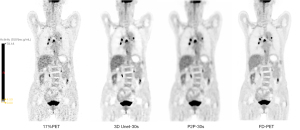
3D Unet deep learning model based on CNN
The ability to suppress background tissue noise
In this study, we selected liver, lung, aorta and lumbar spine as background tissues, and measured the noise levels of four background tissues in 8%PET, 17%PET and two deep learning model reconstruction image groups.
With 8%PET as the input of the model, 3D Unet can reduce the SD of the background tissue and significantly increase the SNR. Compared with standard dose images, SD and SNR in liver and aorta of the 3D Unet-15s group were lower than those of the s-PET group.
With 17%PET as the input of the model, 3D Unet also reduced the SD of background tissue and increased the SNR. Noise levels in lung and lumbar tissues were not statistically different from those in the s-PET group (Tables 1-4).
Table 1
Group | Liver | Lung | Aorta | Lumbar spine |
---|---|---|---|---|
3D Unet-15s | 0.20 (0.14, 0.28)* | 0.06 (0.05, 0.10) | 0.12 (0.09, 0.15)* | 0.27 (0.16, 0.37) |
P2P-15s | 0.31 (0.23, 0.37)* | 0.11 (0.09, 0.13)* | 0.16 (0.15, 0.23) | 0.27 (0.24, 0.34) |
8%PET | 0.86 (0.64, 1.06) | 0.22 (0.16, 0.25) | 0.55 (0.46, 0.77) | 1.20 (0.75, 1.57) |
s-PET | 0.25 (0.19, 0.29) | 0.08 (0.06, 0.09) | 0.19 (0.16, 0.20) | 0.27 (0.23, 0.41) |
H | 32.13 | 35.22 | 35.11 | 28.65 |
P | <0.0001 | <0.0001 | <0.0001 | <0.0001 |
The italic font represents a statistical difference between this value and that of the 8%PET group. And * indicates that the value is statistically significant compared with the s-PET group. SD, standard deviation; PET, positron emission tomography; s-PET, standard positron emission tomography (per bed time: 90 s); 3D Unet, a deep network model based on CNN; P2P, Pixel2Pixel deep network model based on GAN; H, H value for the Kruskal-Wallis method; CNN, convolutional neural network; GAN, generative adversarial network.
Table 2
Group | Liver | Lung | Aorta | Lumbar spine |
---|---|---|---|---|
3D Unet-15s | 13.42 (10.04, 19.71)* | 7.83 (6.10, 8.84)* | 15.73 (12.15, 22.36)* | 7.69 (6.15, 10.82) |
P2P-15s | 7.49 (6.66, 10.34)* | 3.58 (2.73, 4.36)* | 8.14 (7.30, 10.48) | 6.29 (5.73, 7.31) |
8%PET | 2.69 (1.95, 3.28) | 2.00 (1.18, 2.14) | 2.34 (1.55, 3.01) | 1.51 (1.20, 2.26) |
s-PET | 8.29 (7.77, 10.02) | 5.00 (4.23, 5.64) | 7.95 (6.22, 9.32) | 5.95 (4.77, 8.28) |
H | 36.86 | 40.84 | 37.06 | 31.10 |
P | <0.0001 | <0.0001 | <0.0001 | <0.0001 |
The italic font represents a statistical difference between this value and that of the 8%PET group. And * indicates that the value is statistically significant compared with the s-PET group. SNR, signal-to-noise ratio; PET, positron emission tomography; s-PET, standard positron emission tomography (per bed time: 90 s); 3D Unet, a deep network model based on CNN; P2P, Pixel2Pixel deep network model based on GAN; H, H value for the Kruskal-Wallis method; CNN, convolutional neural network; GAN, generative adversarial network.
Table 3
Group | Liver | Lung | Aorta | Lumbar spine |
---|---|---|---|---|
3D Unet-30s | 0.19 (0.15, 0.24)* | 0.06 (0.05, 0.09) | 0.12 (0.10, 0.14)* | 0.26 (0.20, 0.43) |
P2P-30s | 0.28 (0.25, 0.33)* | 0.10 (0.07, 0.13)* | 0.16 (0.13, 0.20) | 0.29 (0.22, 0.37) |
17%PET | 0.45 (0.38, 0.67) | 0.12 (0.11, 0.16) | 0.35 (0.25, 0.42) | 0.66 (0.45, 0.90) |
s-PET | 0.25 (0.19, 0.29) | 0.08 (0.06, 0.09) | 0.19 (0.16, 0.20) | 0.27 (0.23, 0.41) |
H | 29.46 | 25.18 | 32.96 | 19.93 |
P | <0.0001 | <0.0001 | <0.0001 | <0.0001 |
The italic font represents a statistical difference between this value and that of the 17%PET group. And * indicates that the value is statistically significant compared with the s-PET group. SD, standard deviation; PET, positron emission tomography; s-PET, standard positron emission tomography (per bed time: 90 s); 3D Unet, a deep network model based on CNN; P2P, Pixel2Pixel deep network model based on GAN; H, H value for the Kruskal-Wallis method; CNN, convolutional neural network; GAN, generative adversarial network.
Table 4
Group | Liver | Lung | Aorta | Lumbar spine |
---|---|---|---|---|
3D Unet-30s | 11.29 (10.11, 12.95)* | 7.40 (5.00, 9.01)* | 13.85 (9.15, 17.47)* | 7.19 (5.34, 11.07)* |
P2P-30s | 11.32 (9.46, 14.59)* | 5.88 (4.43, 7.75)* | 13.31 (10.05, 15.87)* | 8.55 (6.78, 10.74)* |
17%PET | 4.70 (3.49, 5.19) | 2.64 (2.03, 3.17) | 3.93 (2.84, 5.30) | 3.15 (2.05, 3.81) |
s-PET | 8.29 (7.77, 10.02) | 5.00 (4.23, 5.64) | 7.95 (6.22, 9.32) | 5.95 (4.77, 8.28) |
H | 31.06 | 27.54 | 35.80 | 27.63 |
P | <0.0001 | <0.0001 | <0.0001 | <0.0001 |
The italic font represents a statistical difference between this value and that of the 17%PET group. And * indicates that the value is statistically significant compared with the s-PET group. SNR, signal-to-noise ratio; PET, positron emission tomography; s-PET, standard positron emission tomography (per bed time: 90 s); 3D Unet, a deep network model based on CNN; P2P, Pixel2Pixel deep network model based on GAN; H, H value for the Kruskal-Wallis method; CNN, convolutional neural network; GAN, generative adversarial network.
Effect on noise and SNR of tumor tissue
Regardless of whether 8%PET or 17%PET was used as input to the model, SNRs of tumor lesions in 3D Unet-15s group were higher than those in the original image, but there was no statistical difference in SNR compared with the standard dose group.
NR is an important indicator to measure image contrast. The larger CNR, the larger the difference between the focal signal and the background area, and correspondingly, the better the image contrast. Therefore, we further compared the influence of the deep learning model on the CNR of tumor lesions, when 8%PET or 17%PET was used as the model input, 3D Unet could increase the CNR of tumor lesions to a level similar to that of the standard dose group (Tables 5,6, Figures 7,8).
Table 5
Group | PET quantitative parameters of tumor lesions | |||
---|---|---|---|---|
SNR | CNR | SUVmean | SUVmax | |
3D Unet-15s | 33.81 (24.79, 45.52) | 9.94 (5.63, 13.72) | 3.78 (3.06, 4.86) | 6.57 (4.54, 9.61)* |
P2P-15s | 20.45 (15.08, 29.24)* | 3.97 (1.06, 6.91)* | 3.03 (2.62, 3.99)* | 4.72 (3.70, 6.27)* |
8%PET | 16.38 (10.78, 19.90) | 3.19 (2.18, 4.82) | 4.25 (3.62, 5.34) | 10.81 (7.44, 16.41) |
s-PET | 34.82 (24.92, 51.82) | 9.68 (5.90, 13.95) | 3.93 (3.23, 4.86) | 7.29( 5.78, 10.59) |
H | 54.90 | 27.25 | 16.81 | 37.28 |
P | <0.0001 | <0.0001 | 0.001 | <0.0001 |
The italic font represents a statistical difference between this value and that of the 8%PET group. And * indicates that the value is statistically significant compared with the s-PET group. SNR, signal-to-noise ratio; CNR, contrast-noise ratio; SUVmean, mean standardized uptake values for all voxels in the ROI; SUVmax, maximum standardized uptake values for all voxels in the ROI; PET, positron emission tomography; s-PET, standard positron emission tomography (per bed time: 90 s); 3D Unet, a deep network model based on CNN; P2P, Pixel2Pixel deep network model based on GAN; H, H value for the Kruskal-Wallis method; ROI, region of interest; CNN, convolutional neural network; GAN, generative adversarial network.
Table 6
Groups | PET quantitative parameters of tumor lesions | |||
---|---|---|---|---|
SNR | CNR | SUVmean | SUVmax | |
3D Unet-30s | 39.29 (29.10, 50.56) | 9.40 (4.66, 12.77) | 3.55 (3.27, 4.60)* | 7.45 (5.42, 9.73) |
P2P-30s | 25.31 (19.83, 39.49)* | 4.88 (1.18, 8.96)* | 3.78 (3.32, 5.09) | 5.47 (4.47, 7.51)* |
17%PET | 17.61 (13.26, 24.68) | 4.39 (3.12, 7.10) | 4.17 (3.47, 5.30) | 8.37 (6.38, 11.29) |
s-PET | 34.82 (24.92, 51.82) | 9.68 (5.90, 13.95) | 3.93 (3.23, 4.86) | 7.29 (5.78, 10.59) |
H | 38.19 | 17.67 | 2.63 | 12.20 |
P | <0.0001 | 0.001 | 0.452 | 0.007 |
The italic font represents a statistical difference between this value and that of the 17%PET group. And * indicates that the value is statistically significant compared with the s-PET group. SNR, signal-to-noise ratio; CNR, contrast-noise ratio; SUVmean, mean standardized uptake values for all voxels in the ROI; SUVmax, maximum standardized uptake values for all voxels in the ROI; PET, positron emission tomography; s-PET, standard positron emission tomography (per bed time: 90 s); 3D Unet, a deep network model based on CNN; P2P, Pixel2Pixel deep network model based on GAN; H, H value for the Kruskal-Wallis method; ROI, region of interest; CNN, convolutional neural network; GAN, generative adversarial network.
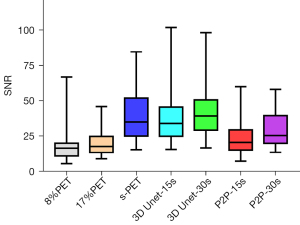
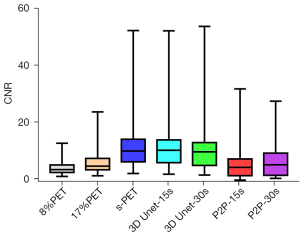
Effect on semi-quantitative parameters of tumor tissue
The metabolic parameters of tumor have strong correlation with the evaluation of benign and malignant tumor, the evaluation of tumor efficacy, and the evaluation of tumor prognosis. Therefore, we further observed the influence of deep learning model on semi-quantitative parameters of tumor lesions. When 8%PET was used as model input, SUVmean and SUVmax of tumor lesions in the 3D Unet-15s group were lower than those in the original image group. Compared with standard dose images, SUVmax of tumor lesions in the 3D Unet-15s group was significantly decreased, while SUVmean had no significant difference. When 17%PET was used as model input, SUVmean and SUVmax of tumor lesions in the 3D Unet-30s group were lower than those in the original image group, and only SUVmax was not significantly different in the 3D Unet-30s group compared with the standard dose group (Tables 5,6, Figures 9,10).
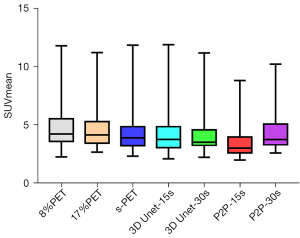
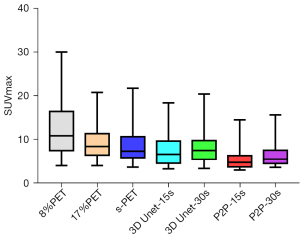
P2P deep learning model based on GAN
The ability to suppress background tissue noise
When 8%PET was used as model input, P2P significantly decreased SD and increased SNR in four background tissues. Compared with standard dose images, SD and SNR in liver and lungs were higher and lower in the P2P-15s group than in the s-PET group, and SD and SNR in the aorta and lumbar spine were not different from those in the s-PET group.
When 17%PET was used as model input, the SD of background tissue was decreased and SNR was increased in P2P-30s group. Compared with standard dose images, there were statistical differences in SD and SNR in lung and liver in the P2P-30s group, but no statistical differences in aortic and lumbar values (Tables 1-4).
Effect on noise and SNR of tumor tissue
When 8%PET or 17%PET was used as model input, SNR of P2P-15s group was higher than that of original image group, but still significantly lower than that of standard dose group (s-PET group).
Then, the influence of P2P deep learning model on CNR of tumor lesions was observed. When 8%PET was used as model input, CNR of P2P-15s group was higher than that of original image group, but significantly lower than that of s-PET group. When 17%PET was used as model input, CNR of tumor lesions in the P2P-30s group showed no statistical difference compared with the original image group, and was significantly lower than that in the s-PET group (Tables 5,6, Figures 7,8).
Effect on semi-quantitative parameters of tumor tissue
When 8%PET was used as model input, the SUVmean and SUVmax of tumor lesions in the P2P-15s group were lower than those in the original image group and the standard dose group. When 17%PET was used as model input, SUVmax of tumor lesions in P2P-30s group was significantly lower than that in standard dose group, while SUVmean had no statistical difference (Tables 5,6, Figures 9,10).
Discussion
A systematic comparison of the effectiveness of deep learning reconstruction models, based on CNN and GAN, for improving LDPET images was conducted in this study to determine their impact on tumor clinical diagnosis. The results showed that both the 3D Unet and P2P models improved the quality of the LDPET tumor image and improved the objective quantitative parameter SNR of image quality, both in the background tissue and tumor lesions. However, the 3D Unet model was significantly better than the P2P model in improving the quality of the clinical images of the PET tumor; it could meet the accuracy of tumor lesion quantification and reach the level of tumor diagnosis.
Deep learning methods using GANs and CNNs have shown promise in medical image denoising (25). Wang et al. (14), Islam and Zhang (26), and Sajjad et al. (27) used a GAN synthesis model to generate PET images of the brain at different stages of progression of Alzheimer’s disease. Ouyang et al. also used a GAN model to generate images of a PET scan with a standard dose of amyloid from intensively low-dosage (1%) images (15). Xie et al. achieved a trade-off between lesion contrast recovery and background noise using an improved GAN model (28). A GAN-based deep learning model was used to restore LDPET images of the brain and body (29-32). The results show that the GAN-based model can retain more details in the image while reducing SUVmean and SUVmax errors. However, for the recovery of LDPET images of tumor lesions, an improved reconstruction model based on a CNN was adopted. Using CNN, Kaplan and Zhu obtained better image edge, structure, and texture details and refined the quantitative parameters using 1/10 LDPET images as inputs (12). Lu et al. used a 3D Unet deep learning reconstruction model to significantly reduce the dose of 18F-FDG in patients with tumors and ensure diagnostic accuracy (16). Furthermore, 2D and 2.5D Unets were found to have inferior quantitative performance when compared to 3D Unet, and when using 10% low counts to predict standard-dose PET images. 3D Unet was able to reduce image noise and SUVmean deviation even for subcentimeter lung nodules, consistent with the results of this study. There are also studies (13,33-35) using fewer doses of PET images, and CNN also shows an advantage in tumor quantification. A 3D Unet model was developed by Sanaat et al. to predict FDPET images from 5% LDPET images (13), thereby improving both image quality and SUV accuracy. Wang et al. used 6.25% 18F-FDG ULDPET images and MRI images of the same machine from 33 children and young adults and input them into the CNN network for image quality recovery (35). The results showed a significant improvement in SUVmax values of tumor lesions and reference tissues and achieved similar parameter values as the standard PET clinical scan.
We found that the 3D Unet model was significantly better than the P2P model for denoising the PET images. 3D Unet builds the entire model based on a 3D convolution. With 3D convolution, we can better acquire the three-dimensional spatial information of an image, and the network model can perform more reliably and robustly if the data contain more dimensions; therefore, reconstructed images are of better quality. Simultaneously, compared with the workflow of P2P stitching to obtain the final image layer by layer, the 3D Unet stitching has fewer layers and uses bilinear interpolation to replace the upsampling layer, which can effectively eliminate the checkerboard artifacts in the reconstructed image. The P2P model takes 2D data as the training target; therefore, the processing speed is high and hardware requirement is low. In this study, high-count reconstructions were used as gold standard labels to represent the unknown PET images to be reconstructed. In addition, instead of feeding noisy images directly into the CNN, a feasible set of PET images was determined using the CNN, thus the entire network was more data-driven. The most desirable feature of this model is that it can include more extensive prior information in the image representation, such as interpatient scanning information. In addition, when the prior information comes from multiple resources, such as time and anatomical information, we can use multiple input channels to aggregate the information and let the network decide the best combination during the training phase. In this study, 3D Unet and P2P models had essentially the same ability to reduce noise, restore image quality, and improve SNR in (1/6 and 1/12) LDPET, both of which can meet the image quality requirements of clinical tumor diagnosis. However, the two models had different recovery effects on the accuracy of the quantitative tumor parameters. A significant reduction was observed in the quantitative parameters SUVmean and SUVmax for cancerous lesions for both models. 3D Unet-30s significantly reduced SUVmean (P=0.016) compared with 90 s, whereas SUVmax did not differ significantly (P=0.313). In the 3D Unet-15s group, SUVmax was significantly reduced (P=0.034), whereas SUVmean was not significantly different (P=0.315). SUVmean may have been affected by differences in the metabolic volume delineated by the tumor. Among P2P-15s/-30s participants, SUVmax was significantly lower than that of the 90 s participants (all P<0.0001). Thus, with the 3D Unet model, the quantitative parameters of the tumors were maintained more accurately.
In addition, we found that the 3D Unet model had obvious advantages in improving the CNR of foci with high FDG uptake. By constraining the measured data, 3D Unet can help recover some small features that have been removed by image denoising methods, facilitating the recovery of CNR (36). This study confirmed the recovery effect of the 3D Unet model on the CNR index of LDPET images, which could allow the CNR to reach the level of 90s-PET images with lower dose (1/12 dose) PET data input and clearly display and effectively detect small lesions (37). However, the size of lesions in this study ranged from 1.65 to 3.94 cm, and stratified analysis was not performed according to the size of lesions. As a result, it is uncertain whether this model would perform well in other tasks (such as detecting small lesions). Moreover, the robustness of the 3D Unet model should be determined by further testing via quantification of other tasks.
For the optimization of model performance, this study also used 1/6 and 1/12 noise level data to train the model. da Costa-Luis and Reader suggested that a 3D Unet network design is robust when only a limited number of training data are available while accepting multiple inputs (including competitive denoising methods) (38). When the test data dose levels exceed the training dose levels, a new training session is required to achieve an optimal neural network performance. In this study, data with two noise levels were used to train the model separately, instead of superimposing the two types of data. At the same time, some studies have proposed that training models with anatomic morphological imaging information and LDPET images can further improve the image details and quantitative accuracy of lesions. In addition, a combination of different PET reconstruction algorithms, such as the Bayesian algorithm (BSREM) with a CNN network, has also been used to optimize model performance (39).
The applicability of this model is limited, mainly because of the SiPM digital PET/CT equipment and data obtained based on the BSREM reconstruction algorithm. However, the equipment used in most clinical trials is not uniform and PET data with the same acquisition reconstruction parameters cannot be used. Moreover, the study was limited in sample size, which made it impossible to apply fair judgment to the performance of the deep learning model. To make the model more robust, the training dataset must be increased. In addition to cross-validating the generalizability of the model, we hope to conduct more multicenter studies. Furthermore, a variety of tumors were included in this study, and deep-learning model research focusing on a single tumor may be more conducive to the clinical application of the model, such as the application of LDPET in the screening of pulmonary nodules.
Conclusions
In this study, the advantages of two different models in the clinical tumor diagnosis environment were compared. Both GAN and CNN can suppress image noise to varying degrees and improve the overall quality of the image. However, when CNN represented by 3D Unet reduces the noise of tumor lesions, it can improve the CNR of tumor lesions and correspondingly improve the salience of tumor lesions. Moreover, the SUVmean and SUVmax of tumor lesions are similar to those under the collection conditions of clinical standard protocols, which can meet the needs of clinical diagnosis.
Acknowledgments
The research guider, Rui Chai, was instrumental in defining the path of this research. For this, all authors are extremely grateful.
Funding: This research was funded by the National Natural Science Foundation of China (Nos. 81971655, 82027804 and U22A6008). The funder had no role in the design of the study; in the collection, analyses, or interpretation of data; in the writing of the manuscript; or in the decision to publish the results.
Footnote
Conflicts of Interest: All authors have completed the ICMJE uniform disclosure form (available at https://qims.amegroups.com/article/view/10.21037/qims-22-1181/coif). The authors report that this research was funded by the National Natural Science Foundation of China (Nos. 81971655, 82027804 and U22A6008). The authors have no other conflicts of interest to declare.
Ethical Statement: The authors are accountable for all aspects of the work in ensuring that questions related to the accuracy or integrity of any part of the work are appropriately investigated and resolved. This study was conducted in accordance with the Declaration of Helsinki (as revised in 2013). The study protocol was approved by the Ethics Committee of First Hospital of Shanxi Medical University (No. K-GK001) and informed consent was taken from all individual participants.
Open Access Statement: This is an Open Access article distributed in accordance with the Creative Commons Attribution-NonCommercial-NoDerivs 4.0 International License (CC BY-NC-ND 4.0), which permits the non-commercial replication and distribution of the article with the strict proviso that no changes or edits are made and the original work is properly cited (including links to both the formal publication through the relevant DOI and the license). See: https://creativecommons.org/licenses/by-nc-nd/4.0/.
References
- Cheson BD. PET/CT in Lymphoma: Current Overview and Future Directions. Semin Nucl Med 2018;48:76-81. [Crossref] [PubMed]
- Delgado Bolton RC, Calapaquí-Terán AK, Giammarile F, Rubello D. Role of (18)F-FDG PET/CT in establishing new clinical and therapeutic modalities in lung cancer. A short review. Rev Esp Med Nucl Imagen Mol 2019;38:229-33. (Engl Ed). [Crossref] [PubMed]
- El-Galaly TC, Gormsen LC, Hutchings M. PET/CT for Staging; Past, Present, and Future. Semin Nucl Med 2018;48:4-16. [Crossref] [PubMed]
- Paydary K, Seraj SM, Zadeh MZ, Emamzadehfard S, Shamchi SP, Gholami S, Werner TJ, Alavi A. The Evolving Role of FDG-PET/CT in the Diagnosis, Staging, and Treatment of Breast Cancer. Mol Imaging Biol 2019;21:1-10. [Crossref] [PubMed]
- Alom MZ, Yakopcic C, Hasan M, Taha TM, Asari VK. Recurrent residual U-Net for medical image segmentation. J Med Imaging (Bellingham) 2019;6:014006. [Crossref] [PubMed]
- Amiri M, Brooks R, Behboodi B, Rivaz H. Two-stage ultrasound image segmentation using U-Net and test time augmentation. Int J Comput Assist Radiol Surg 2020;15:981-8. [Crossref] [PubMed]
- Park J, Hwang D, Kim KY, Kang SK, Kim YK, Lee JS. Computed tomography super-resolution using deep convolutional neural network. Phys Med Biol 2018;63:145011. [Crossref] [PubMed]
- Chen KT, Schürer M, Ouyang J, Koran MEI, Davidzon G, Mormino E, Tiepolt S, Hoffmann KT, Sabri O, Zaharchuk G, Barthel H. Generalization of deep learning models for ultra-low-count amyloid PET/MRI using transfer learning. Eur J Nucl Med Mol Imaging 2020;47:2998-3007. [Crossref] [PubMed]
- Eun DI, Jang R, Ha WS, Lee H, Jung SC, Kim N. Deep-learning-based image quality enhancement of compressed sensing magnetic resonance imaging of vessel wall: comparison of self-supervised and unsupervised approaches. Sci Rep 2020;10:13950. [Crossref] [PubMed]
- Higaki T, Nakamura Y, Tatsugami F, Nakaura T, Awai K. Improvement of image quality at CT and MRI using deep learning. Jpn J Radiol 2019;37:73-80. [Crossref] [PubMed]
- Weyts K, Lasnon C, Ciappuccini R, Lequesne J, Corroyer-Dulmont A, Quak E, Clarisse B, Roussel L, Bardet S, Jaudet C. Artificial intelligence-based PET denoising could allow a two-fold reduction in [18F]FDG PET acquisition time in digital PET/CT. Eur J Nucl Med Mol Imaging 2022;49:3750-60. [Crossref] [PubMed]
- Kaplan S, Zhu YM. Full-Dose PET Image Estimation from Low-Dose PET Image Using Deep Learning: a Pilot Study. J Digit Imaging 2019;32:773-8. [Crossref] [PubMed]
- Sanaat A, Arabi H, Mainta I, Garibotto V, Zaidi H. Projection Space Implementation of Deep Learning-Guided Low-Dose Brain PET Imaging Improves Performance over Implementation in Image Space. J Nucl Med 2020;61:1388-96. [Crossref] [PubMed]
- Wang Y, Yu B, Wang L, Zu C, Lalush DS, Lin W, Wu X, Zhou J, Shen D, Zhou L. 3D conditional generative adversarial networks for high-quality PET image estimation at low dose. Neuroimage 2018;174:550-62. [Crossref] [PubMed]
- Ouyang J, Chen KT, Gong E, Pauly J, Zaharchuk G. Ultra-low-dose PET reconstruction using generative adversarial network with feature matching and task-specific perceptual loss. Med Phys 2019;46:3555-64. [Crossref] [PubMed]
- Lu W, Onofrey JA, Lu Y, Shi L, Ma T, Liu Y, Liu C. An investigation of quantitative accuracy for deep learning based denoising in oncological PET. Phys Med Biol 2019;64:165019. [Crossref] [PubMed]
- Pain CD, Egan GF, Chen Z. Deep learning-based image reconstruction and post-processing methods in positron emission tomography for low-dose imaging and resolution enhancement. Eur J Nucl Med Mol Imaging 2022;49:3098-118. [Crossref] [PubMed]
- Yi X, Walia E, Babyn P. Generative adversarial network in medical imaging: A review. Med Image Anal 2019;58:101552. [Crossref] [PubMed]
- Wu Z, Guo B, Huang B, Hao X, Wu P, Zhao B, Qin Z, Xie J, Li S. Phantom and clinical assessment of small pulmonary nodules using Q.Clear reconstruction on a silicon-photomultiplier-based time-of-flight PET/CT system. Sci Rep 2021;11:10328. [Crossref] [PubMed]
- Gong Kuang, Guan Jiahui. Kyungsang Kim, Xuezhu Zhang, Jaewon Yang, Youngho Seo, El Fakhri G, Jinyi Qi, Quanzheng Li. Iterative PET Image Reconstruction Using Convolutional Neural Network Representation. IEEE Trans Med Imaging 2019;38:675-85. [Crossref]
- Park YJ, Choi D, Choi JY, Hyun SH. Performance Evaluation of a Deep Learning System for Differential Diagnosis of Lung Cancer With Conventional CT and FDG PET/CT Using Transfer Learning and Metadata. Clin Nucl Med 2021;46:635-40. [Crossref] [PubMed]
- Messerli M, Stolzmann P, Egger-Sigg M, Trinckauf J, D'Aguanno S, Burger IA, von Schulthess GK, Kaufmann PA, Huellner MW. Impact of a Bayesian penalized likelihood reconstruction algorithm on image quality in novel digital PET/CT: clinical implications for the assessment of lung tumors. EJNMMI Phys 2018;5:27. [Crossref] [PubMed]
- Yan J, Schaefferkoette J, Conti M, Townsend D. A method to assess image quality for Low-dose PET: analysis of SNR, CNR, bias and image noise. Cancer Imaging 2016;16:26. [Crossref] [PubMed]
- Wu Z, Guo B, Huang B, Zhao B, Qin Z, Hao X, Liang M, Xie J, Li S. Does the beta regularization parameter of bayesian penalized likelihood reconstruction always affect the quantification accuracy and image quality of positron emission tomography computed tomography? J Appl Clin Med Phys 2021;22:224-33. [Crossref] [PubMed]
- Lei Y, Dong X, Wang T, Higgins K, Liu T, Curran WJ, Mao H, Nye JA, Yang X. Whole-body PET estimation from low count statistics using cycle-consistent generative adversarial networks. Phys Med Biol 2019;64:215017. [Crossref] [PubMed]
- Islam J, Zhang Y. GAN-based synthetic brain PET image generation. Brain Inform 2020;7:3. [Crossref] [PubMed]
- Sajjad M, Ramzan F, Khan MUG, Rehman A, Kolivand M, Fati SM, Bahaj SA. Deep convolutional generative adversarial network for Alzheimer's disease classification using positron emission tomography (PET) and synthetic data augmentation. Microsc Res Tech 2021;84:3023-34. [Crossref] [PubMed]
- Xie Z, Baikejiang R, Li T, Zhang X, Gong K, Zhang M, Qi W, Asma E, Qi J. Generative adversarial network based regularized image reconstruction for PET. Phys Med Biol 2020;65:125016. [Crossref] [PubMed]
- Sanaat A, Shiri I, Arabi H, Mainta I, Nkoulou R, Zaidi H. Deep learning-assisted ultra-fast/low-dose whole-body PET/CT imaging. Eur J Nucl Med Mol Imaging 2021;48:2405-15. [Crossref] [PubMed]
- Wang Y, Zhou L, Yu B, Wang L, Zu C, Lalush DS, Lin W, Wu X, Zhou J, Shen D. 3D Auto-Context-Based Locality Adaptive Multi-Modality GANs for PET Synthesis. IEEE Trans Med Imaging 2019;38:1328-39. [Crossref] [PubMed]
- Xue H, Zhang Q, Zou S, Zhang W, Zhou C, Tie C, Wan Q, Teng Y, Li Y, Liang D, Liu X, Yang Y, Zheng H, Zhu X, Hu Z. LCPR-Net: low-count PET image reconstruction using the domain transform and cycle-consistent generative adversarial networks. Quant Imaging Med Surg 2021;11:749-62. [Crossref] [PubMed]
- Zhao K, Zhou L, Gao S, Wang X, Wang Y, Zhao X, Wang H, Liu K, Zhu Y, Ye H. Study of low-dose PET image recovery using supervised learning with CycleGAN. PLoS One 2020;15:e0238455. [Crossref] [PubMed]
- Chaudhari AS, Mittra E, Davidzon GA, Gulaka P, Gandhi H, Brown A, Zhang T, Srinivas S, Gong E, Zaharchuk G, Jadvar H. Low-count whole-body PET with deep learning in a multicenter and externally validated study. NPJ Digit Med 2021;4:127. [Crossref] [PubMed]
- Spuhler K, Serrano-Sosa M, Cattell R, DeLorenzo C, Huang C. Full-count PET recovery from low-count image using a dilated convolutional neural network. Med Phys 2020;47:4928-38. [Crossref] [PubMed]
- Wang YJ, Baratto L, Hawk KE, Theruvath AJ, Pribnow A, Thakor AS, Gatidis S, Lu R, Gummidipundi SE, Garcia-Diaz J, Rubin D, Daldrup-Link HE. Artificial intelligence enables whole-body positron emission tomography scans with minimal radiation exposure. Eur J Nucl Med Mol Imaging 2021;48:2771-81. [Crossref] [PubMed]
- Cui J, Gong K, Guo N, Wu C, Meng X, Kim K, Zheng K, Wu Z, Fu L, Xu B, Zhu Z, Tian J, Liu H, Li Q. PET image denoising using unsupervised deep learning. Eur J Nucl Med Mol Imaging 2019;46:2780-9. [Crossref] [PubMed]
- Schaefferkoetter J, Yan J, Ortega C, Sertic A, Lechtman E, Eshet Y, Metser U, Veit-Haibach P. Convolutional neural networks for improving image quality with noisy PET data. EJNMMI Res 2020;10:105. [Crossref] [PubMed]
- da Costa-Luis CO, Reader AJ. Micro-Networks for Robust MR-Guided Low Count PET Imaging. IEEE Trans Radiat Plasma Med Sci 2020;5:202-12. [Crossref] [PubMed]
- Mehranian A, Wollenweber SD, Walker MD, Bradley KM, Fielding PA, Su KH, Johnsen R, Kotasidis F, Jansen FP, McGowan DR. Image enhancement of whole-body oncology [18F]-FDG PET scans using deep neural networks to reduce noise. Eur J Nucl Med Mol Imaging 2022;49:539-49. [Crossref] [PubMed]