Diagnostic accuracy of dual-energy computed tomography-based nomogram for differentiating papillary thyroid microcarcinomas from micronodular goiters
Introduction
Thyroid cancer is the most common endocrine malignancy worldwide, with papillary thyroid cancer (PTC) being its most common subtype (1,2). The World Health Organization defines papillary thyroid microcarcinoma (PTMC) as a PTC with a maximum diameter of ≤1 cm (3,4). In recent years, the incidence of thyroid cancer has increased rapidly worldwide, with PTMC accounting for up to 50% of new cases (5,6). Thyroid micronodules refer to thyroid nodules with a maximum diameter <1 cm. Among the benign thyroid nodules, nodular goiters (NGs) are the most common. There are substantial differences in the treatment and management of PTMC and micronodular goiter (MNG). For MNG, the treatment mainly includes reassuring patients and follow-up observations instead of resection (7,8). Thus, it is crucial to accurately identify MNG from PTMC to avoid overtreatment, reduce both physical and psychological pain, and prevent the unnecessary medical expenditure of patients.
The cytology of samples obtained by fine-needle aspiration biopsy is the gold standard for the preoperative diagnosis of thyroid nodules. However, this procedure is invasive, and the most recent American Thyroid Association guidelines advise against biopsy for thyroid nodules <1 cm (9). Several imaging modalities, including ultrasound (US) and computed tomography (CT), have been widely used to diagnose thyroid diseases. US is the first-line imaging procedure because it is convenient and economical (10); however, the sensitivity and specificity of US vary widely, as this examination is strongly dependent on each individual operator’s experience and manipulation, and thus there is considerable interobserver variability (11,12). In addition, many risk stratification systems for thyroid nodules exist, and the risk level may vary for the same nodule under different risk stratification systems, which may increase patients’ confusion and fears. Contrast-enhanced CT is recommended as a complementary examination method for patients with clinically suspected advanced PTMC and has the advantages of being able to detect metastatic lymph nodes and extrathyroidal extensions (13,14). However, it should be noted that the imaging characteristics of PTMC and MNG overlap significantly. Thus, visual evaluations based on conventional radiological features, especially by inexperienced radiologists, cannot satisfactorily diagnose PTMC.
Recently, dual-energy CT (DECT), which extends the capabilities of conventional CT, has shown promising clinical application in tumor detection and characterization. This advanced technique can generate various parameters, such as iodine concentration (IC), slope of the spectral attenuation curves (λHU), effective atomic number (Zeff), and water content (WC), that can be used for quantitative analysis (15). Additionally, DECT not only reduces the radiation dose but also improves tumor visibility and the concurrent delineation of diseased tissues (16). Previous studies have shown that some DECT quantitative parameters, especially the IC value, have potential value in the differential diagnosis of malignant and benign thyroid nodules (17-21). However, to our knowledge, no study has investigated the application of DECT parameters in differentiating between PTMC and MNG. A nomogram is a visual tool of predictive statistical models that evaluates individual risk factors and has been used in the diagnosis of thyroid nodules (22); however, the existing nomogram only integrates US grayscale morphological features, still depends on the accuracy of operator-reported imaging features, and thus cannot reflect the thyroid function and angiogenesis levels of lesions.
In this study, we sought to develop and validate a nomogram that incorporated both the quantitative parameters and qualitative radiological features for the differential diagnosis of PTMC and MNG to aid clinical decision-making for individual patients. We present the following article in accordance with the STARD reporting checklist (available at https://qims.amegroups.com/article/view/10.21037/qims-22-698/rc).
Methods
Patient and thyroid micronodule selection
This retrospective study was conducted in accordance with the Declaration of Helsinki (as revised in 2013) and approved by the Ethics Committee of Chongqing General Hospital (No. KY S2022-025-01). The requirement of informed consent from the study participants was waived due to the retrospective nature of this study. The data of patients who underwent thyroidectomy with a postoperative pathologic examination between August 2021 and April 2022 at the Chongqing General Hospital were consecutively collected according to the inclusion and exclusion criteria (Figure 1). The inclusion criteria were as follows: (I) the target thyroid nodules were confirmed to be PTMC or NG in postoperative pathology reports; (II) the maximum diameters of the thyroid nodules were ≤1.0 cm; and (III) the patients underwent both noncontrast and contrast-enhanced DECT examinations within 1 week before surgery. The exclusion criteria were as follows: (I) therapy (e.g., radiotherapy, chemotherapy, or immunotherapy) had been performed before the DECT examination; (II) obvious artifacts or noise affected the image quality; (III) the thyroid micronodules were invisible or not clearly distinguished on the DECT images; and (IV) the images and pathology reports of the thyroid micronodules did not match. The clinical characteristic data, including age and gender, were obtained by reviewing the patients’ medical records.
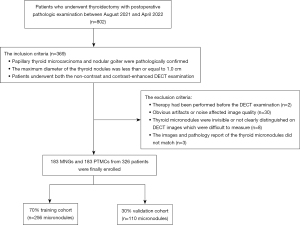
Ultimately, data for 366 focal thyroid micronodules (183 PTMCs and 183 MNGs) from 326 patients (51 men and 275 women; mean age 41.4±11.3 years; median age 41 years, range 19–72 years) were collected, and the cohort was further randomly divided into the training (n=256, 70%) and validation cohorts (n=110, 30%) based on a statistical calculation.
DECT image acquisition
The participants in our study underwent the noncontrast and contrast-enhanced neck scans with DECT devices (IQon Spectral CT, Philips Healthcare) and were scanned in the supine position from the oropharynx to the superior edge of the clavicle. The following identical acquisition protocol was used: tube voltage, 120 kV; smart milliampere-second (mAs); rotation time, 0.5 s; detector collimation, 64×0.625 mm; field of view, 350×350 mm; matrix, 512×512; layer thickness, 5 mm; and reconstruction thickness, 1.25 mm. After non-contrast CT scanning, contrast-enhanced CT scanning was performed. Nonionic contrast media (iohexol, 350 mgI/mL; Schering AG) was injected using an automatic injector at a dose of 1.5 mL/kg at 3.5 mL/s, which was followed by a 30-mL saline flush at the same rate. The scan delay times for the arterial phase (AP) and venous phase (VP) were 40 and 70 s, respectively (23). All the images were then transferred to a spectral CT-dedicated post-processing workstation (IntelliSpace Portal Version 10.1, Philips Healthcare) for further analysis.
Radiological features and DECT quantitative parameters
All the image analyses were independently performed by two radiologists with 6 and 14 years of experience in head and neck imaging, respectively, who were blinded to the pathological results to mitigate potential cognitive biases based on the noncontrast, AP, and VP images. The radiological features of the thyroid micronodules, including irregular shape, low density, punctate calcification, enhanced blurring, and thyroid edge interruption, were evaluated. Among these features, enhanced blurring and thyroid edge interruption were evaluated based on the noncontrast and contrast-enhanced images, and the other features were evaluated based on the noncontrast images. An irregular shape indicated that a micronodule was neither ovoid nor round. Thyroid micronodules were defined as low density if the density was less than that of the surrounding thyroid parenchyma. Punctate calcification was defined as microcalcification with a diameter of ≤2 mm in the nodules. Thyroid edge interruption referred to a partial defect in the thyroid capsule. Enhanced blurring referred to the tumor-thyroid junction being more obscure and the difference in the density between the nodules and normal thyroid parenchyma being smaller after enhancement. If any disagreements arose between the radiologists, the issue was discussed until a consensus was reached.
A quantitative analysis of the AP and VP images was performed. The regions of interests (ROIs) were manually placed within the thyroid nodules containing the largest possible lesion area, with apparent necrotic or cystic areas, calcification, and vessels being avoided. The ROIs were circular with an area ranging from 2.54 to 63.59 mm2. The size, shape, and location of the ROIs were kept constant in different phases using the copy-and-paste function. The measurements were performed twice independently by the 2 radiologists, and the average values were calculated to determine the final results for further analysis. The DECT post-processing workstation automatically calculated the IC and Zeff and generated the spectral attenuation curve. To minimize the influence of hemodynamic interpatient variations, the IC and Zeff values of the lesions were normalized to the carotid artery in the same section to calculate the normalized iodine concentration (NIC) and the normalized effective atomic number (NZeff). For energy levels <100 keV, the spectral curve showed larger changes and differences >100 keV, so a 40–100 keV energy range was selected to compute the λHU, which was calculated as follows: λHU = (CT value at 40 keV – CT value at 100 keV)/(100 – 40).
Development and validation of the nomogram and comparative models
The differences in the radiological features and DECT quantitative parameters between PTMC and MNG were first compared with univariate analysis. Subsequently, significant (P<0.05) parameters were entered into the forward stepwise-regression analysis to determine the independent predictors in the training cohort. Next, a DECT-radiological nomogram was built using the regression coefficients, which combined all the independent predictors. The radiological model was then constructed based on the independent radiological features, and the DECT model was constructed based on the independent DECT quantitative parameters. All 3 models were independently verified in the validation cohort.
Statistical analysis
The statistical analysis was conducted using R software (The R Foundation of Statistical Computing; http://www.R-project.org), MedCalc (version 18.2.1, MedCalc Software), SPSS (version 25.0, IBM Corp.), and empower (R; www.empowerstats.com, X&Y Solutions). The Kolmogorov-Smirnov test was used to check the normality assumption. The normally distributed continuous variables were compared using the independent samples t test, and the nonnormally distributed continuous variables were compared using the Mann-Whitney test. The categorical variables were compared using the chi-squared test. Variables with a P value <0.05 in the univariate analysis were candidates for the logistic regression model. Forward stepwise selection was applied using the likelihood ratio test with Akaike’ information criterion employed as the stopping rule to identify the independent indicators. The odds ratio (OR) and 95% confidence interval (CI) for each independent indicator was calculated. The diagnostic performances of the models were verified independently in the validation cohort and assessed using receiver operating characteristic (ROC) curves, and the areas under the ROC curve (AUCs) with the 95% CIs, sensitivity, and specificity were also calculated. The Delong test was used to compare the AUCs of the models, and decision curve analysis (DCA) was used to compare the clinical values by calculating the net benefits in the training and validation cohorts. A calibration curve was used to assess the calibration ability of the nomogram.
Results
Radiological features and DECT quantitative parameters
The radiological features and DECT quantitative parameters in the training and validation cohorts are summarized in Table 1. The univariate analysis in the training cohort revealed significant differences (P<0.05) in three qualitative features: punctate calcification, enhanced blurring, and thyroid edge interruption. Among the DECT variables, the mean λHU values in the AP, IC in the AP (ICAP), and Zeff in the AP (ZeffAP) were 3.45±0.88, 2.80±0.71, and 8.69±0.35, respectively, in the PTMC group; and 4.70±0.84, 3.79±0.65, and 9.09±0.24, respectively, in the MNG group. The median NIC values in the AP (NICAP) and NZeff in the AP (NZeffAP) were 0.30 (IQR, 0.23–0.34) and 0.80 (IQR, 0.77–0.82) respectively, in the PTMC group; and 0.37 (IQR, 0.32–0.43) and 0.82 (IQR, 0.80–0.85), respectively, in the MNG group. The above DECT parameters of PTMC were significantly lower than those of MNG in the training cohort (all P values <0.05). However, there were no statistical differences in any of the DECT parameters in the VP between the two groups (all P values >0.05) (Table 2). Representative images are shown in Figure 2.
Table 1
Variable | Training cohort (n=256) | Validation cohort (n=110) |
---|---|---|
DECT parameters | ||
λHUAP | 4.03±1.04 | 4.05±1.06 |
ICAP (mg/mL) | 3.24±0.84 | 3.26±0.84 |
NICAP | 0.33±0.08 | 0.34±0.09 |
ZeffAP | 8.89±0.34 | 8.88±0.36 |
NZeffAP | 0.81±0.04 | 0.81±0.06 |
λHUVP | 3.48±0.82 | 3.58±0.74 |
ICVP (mg/mL) | 2.81±0.66 | 2.89±0.60 |
NICVP | 0.68±0.13 | 0.70±0.13 |
ZeffVP | 8.69±0.32 | 8.74±0.25 |
NZeffVP | 0.94±0.03 | 0.94±0.03 |
Radiological feature, n (%) | ||
Punctate calcification | 95 (37.1) | 45 (40.9) |
Thyroid edge interruption | 171 (66.8) | 81 (73.6) |
Enhanced blurring | 118 (46.1) | 46 (41.8) |
Low density | 219 (85.5) | 93 (84.5) |
Irregular shape | 10 (3.9) | 6 (5.5) |
The data are represented as the mean ± standard deviation or n (%). DECT, dual-energy computed tomography; λHUAP, slope of the spectral Hounsfield unit curve in the arterial phase; ICAP, iodine concentration in the arterial phase; NICAP, normalized iodine concentration in the arterial phase; ZeffAP, effective atomic number in the arterial phase; NZeffAP, normalized effective atomic number in the arterial phase; λHUVP, slope of the spectral Hounsfield unit curve in the venous phase; ICVP, iodine concentration in the venous phase; NICVP, normalized iodine concentration in the venous phase; ZeffVP, effective atomic number in the venous phase; NZeffVP, normalized effective atomic number in the venous phase.
Table 2
Variable | MNG group (n=122) | PTMC group (n=134) | F/Z/χ2 | P value |
---|---|---|---|---|
λHUAP | 4.70±0.84 | 3.45±0.88 | 0.102 | <0.001 |
ICAP (mg/mL) | 3.79±0.65 | 2.80±0.71 | 0.429 | <0.001 |
NICAP | 0.37 (0.32, 0.43) | 0.30 (0.23, 0.34) | –8.235 | <0.001 |
ZeffAP | 9.09±0.24 | 8.69±0.35 | 5.072 | <0.001 |
NZeffAP | 0.82 (0.80, 0.85) | 0.80 (0.77, 0.82) | –5.711 | <0.001 |
λHUVP | 3.53 (3.07, 4.11) | 3.40 (2.94, 4.05) | –1.024 | 0.306 |
ICVP (mg/mL) | 2.86 (2.49, 3.32) | 2.75 (2.37, 3.27) | –1.055 | 0.291 |
NICVP | 0.68±0.11 | 0.72±0.14 | 13.741 | 0.065 |
ZeffVP | 8.73 (8.57, 8.93) | 8.70 (8.52, 8.92) | –0.823 | 0.410 |
NZeffVP | 0.94±0.02 | 0.95±0.03 | 6.672 | 0.054 |
Punctate calcification | 31 (25.4) | 64 (47.8) | 13.670 | <0.001 |
Thyroid edge interruption | 68 (55.7) | 103 (76.9) | 12.853 | <0.001 |
Enhanced blurring | 43 (35.2) | 75 (56.0) | 11.038 | 0.001 |
Low density | 104 (85.2) | 124 (95.2) | 3.485 | 0.062 |
Irregular shape | 3 (2.5) | 7 (5.2) | 1.031 | 0.254 |
The data are presented as the mean ± standard deviations, median and IQR, or n (%). DECT, dual-energy computed tomography; PTMC, papillary thyroid microcarcinoma; MNG, micronodular goiter; λHUAP, slope of the spectral Hounsfield unit curve in the arterial phase; ICAP, iodine concentration in the arterial phase; NICAP, normalized iodine concentration in the arterial phase; ZeffAP, effective atomic number in the arterial phase; NZeffAP, normalized effective atomic number in the arterial phase; λHUVP, slope of the spectral Hounsfield unit curve in the venous phase; ICVP, iodine concentration in the venous phase; NICVP, normalized iodine concentration in the venous phase; ZeffVP, effective atomic number in the venous phase; NZeffVP, normalized effective atomic number in the venous phase.
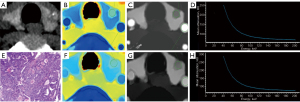
Development of prediction models for differentiating between PTMC and MNG
All the variables with P values <0.05 were included in the multivariate logistic regression analysis (Table 3). The forward stepwise logistic regression analysis revealed that punctate calcification (OR =2.163; 95% CI: 1.091–4.289; P=0.027), enhanced blurring (OR =3.188; 95% CI: 1.624–6.260; P=0.001), ICAP (OR =0.172; 95% CI: 0.090–0.329; P<0.001), and NICAP (OR =0.003; 95% CI: 0.001–0.775; P=0.041) showed statistically significant differences and were incorporated as independent risk factors to build the DECT-radiological nomogram for predicting the PTMC probability individually (Figure 3). Additionally, the radiological model was constructed based on the radiological features, including punctate calcification and enhanced blurring, while the DECT model was constructed based on the DECT quantitative parameters, including the ICAP and NICAP.
Table 3
Variables | Odds ratio | 95% CI | P value | Odds ratio | 95% CI | P value |
---|---|---|---|---|---|---|
λHUAP | 0.743 | 0.047–11.679 | 0.832 | |||
ICAP | 0.178 | 0.093–0.342 | <0.001 | 0.172 | 0.090–0.329 | <0.001 |
NICAP | 0.003 | 0.001–0.792 | 0.041 | 0.003 | 0.001–0.775 | 0.041 |
ZeffAP | 0.710 | 0.016–31.621 | 0.860 | |||
NZeffAP | 0.199 | 0.001–110.245 | 0.830 | |||
Punctate calcification | 2.129 | 1.071–4.233 | 0.031 | 2.163 | 1.091–4.289 | 0.027 |
Thyroid edge interruption | 1.658 | 0.822–3.343 | 0.158 | |||
Enhanced blurring | 2.973 | 1.505–5.874 | 0.002 | 3.188 | 1.624–6.260 | 0.001 |
DECT, dual-energy computed tomography; PTMC, papillary thyroid microcarcinoma; MNG, micronodular goiter; λHUAP, slope of the spectral Hounsfield unit curve in the arterial phase; ICAP, iodine concentration in the arterial phase; NICAP, normalized iodine concentration in the arterial phase; ZeffAP, effective atomic number in the arterial phase; NZeffAP, normalized effective atomic number in the arterial phase.
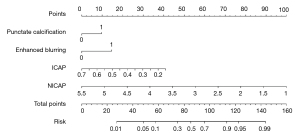
Diagnostic performances of the 3 models
Table 4 shows the predictive results of the 3 models, and Figure 4 shows the ROC analysis of the 3 models for identifying PTMC and MNG in the training and validation cohorts. The radiological model had a diagnostic AUC of 0.661 (95% CI: 0.595–0.728), a sensitivity of 0.754, and a specificity of 0.516 in the training cohort; and an AUC of 0.701 (95% CI: 0.601–0.800), a sensitivity of 0.592, and a specificity of 0.738 in the validation cohort. The DECT model had a diagnostic AUC of 0.856 (95% CI: 0.810–0.902), a sensitivity of 0.769, and a specificity of 0.770 in the training cohort; and an AUC of 0.791 (95% CI: 0.704–0.877), a sensitivity of 0.653, and a specificity of 0.803 in the validation cohort. The DECT–radiological nomogram for identifying PTMC had a diagnostic AUC of 0.880 (95% CI: 0.839–0.921), a sensitivity of 0.799, and a specificity of 0.803 in the training cohort; and an AUC of 0.836 (95% CI: 0.760–0.911), a sensitivity of 0.633, and a specificity of 0.820 in the validation cohort. The calibration curve of the nomogram showed that the prediction results were in good agreement with the pathological findings in both the training and validation cohorts (Figure 5).
Table 4
Pathological results | Training cohort (n=256) | Validation cohort (n=110) | |||||||||||||||
---|---|---|---|---|---|---|---|---|---|---|---|---|---|---|---|---|---|
Radiological model | DECT model | DECT-radiological nomogram | Radiological model | DECT model | DECT-radiological nomogram | ||||||||||||
True | False | True | False | True | False | True | False | True | False | True | False | ||||||
True | 101 | 33 | 103 | 31 | 107 | 27 | 29 | 20 | 32 | 17 | 31 | 18 | |||||
False | 59 | 63 | 28 | 94 | 24 | 98 | 16 | 45 | 12 | 49 | 11 | 50 |
DECT, dual-energy computed tomography; PTMC, papillary thyroid microcarcinoma; MNG, micronodular goiter.
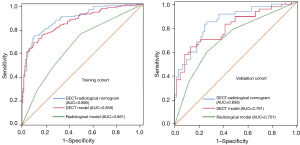
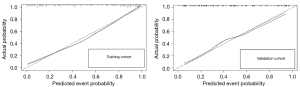
The DeLong test revealed that the DECT-radiological nomogram outperformed the radiological model in the training (P<0.001) and validation cohorts (P=0.026). The DECT model had better a diagnostic performance than did the radiological model (P<0.001) in the training cohort, but there was no significant difference in the validation cohort (P=0.169). On the basis of the DCA, as the threshold probability fell within a range of 0.04–0.98 in the training cohort and 0.1–0.85 in the validation cohort, the DECT-radiological nomogram was considered to have a greater net benefit than the all or no-intervention strategy. Additionally, the DECT-radiological nomogram and DECT model were better able to distinguish between MNG and PTMC than was the radiological model (Figure 6).
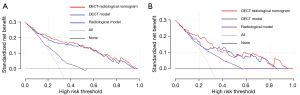
Discussion
In this retrospective study, we developed and validated a DECT-radiological nomogram to differentiate between PTMC and MNG that combined two quantitative parameters (ICAP and NICAP) and two radiological features (punctate calcification and enhanced blurring). The nomogram performed better than did the DECT model and the radiological model in the training and validation cohorts, and had ideal identification and calibration abilities. Thus, the DECT quantitative parameters complemented the conventional radiological features in the differential diagnosis of PTMC and MNG.
We found that punctate calcification and enhanced blurring were the most important radiological features for distinguishing between MNG and PTMC. Punctate calcification, of which the pathological basis is the psammoma body, has been shown to be a characteristic feature of PTC in several previous studies (24-26). Similarly, we also found that punctate calcification was more prevalent in the PTMC group than the MNG group. Previous studies have reported that new blood vessels are more dense in the tumor-thyroid junction area than the central area in PTMC (27,28), which may lead to the centripetal enhancement and the smaller density difference between PTMC and normal thyroid parenchyma after enhancement. Based on the two independent indicators, we constructed the radiological model, which had a moderate diagnostic ability with an AUC of 0.661 in the training cohort and an AUC of 0.701 in the validation cohort. However, unlike the findings of some previous studies (26,29), we found no significant differences in terms of the indicators of irregular shape, lower density, and thyroid edge interruption. Thus, PTMC and MNG may not be able to be accurately identified by the assessment of radiological features based on naked-eye observations, and such assessment may also be affected by subjectivity and consequent instability issues.
To address this issue, quantitative parameters calculated from DECT have been used to diagnose thyroid lesions, and positive results have been obtained (17-21). Our results indicated that the ICAP and NICAP of PTMC were significantly lower than those of MNG, which is consistent with the findings of Lee et al. (18). However, another study found no significant difference in the IC and NIC in the AP (19). The inconsistencies in these results may be due to the different enhanced scan delay times. The IC on contrast-enhanced CT is closely related to the intrinsic iodine uptake of thyroid micronodules and hemodynamics. In theory, normal follicular cells responsible for iodine uptake exist in MNGs, but they are replaced by cancer cells and fiber connective tissues in PTMCs (30), which decrease the iodine-uptake ability of PTMCs. In addition, MNGs lack fibrous capsules and show the same vascularization as does normal thyroid parenchyma (31). However, PTMCs fail to form a neovascular bed, and arteriovenous fistulas have a deficient blood supply (27,32), which may explain why the enhancement degree of PTMCs is significantly lower than that of MNGs in the AP. Thus, the ICAP of PTMC was significantly lower than that of MNG. The ICs were then normalized using the carotid artery to minimize the individual circulation variation between the patients, and we found that the NICAP was an independent risk factor for differentiating between MNG and PTMC. The DECT model composed of the ICAP and NICAP had better diagnostic performance with an AUC of 0.856 in the training cohort and an AUC 0.791 in the validation cohort as compared with the radiological model.
By incorporating the radiological model and the DECT model, we established the DECT-radiological nomogram, which had the highest AUC (training cohort: AUC 0.880; validation cohort: AUC 0.836). The DECT-radiological nomogram and the DECT model had better diagnostic sensitivity and specificity in the differential diagnosis of PTMC and MNG in the training and validation cohorts than did the radiological model. Further, the DCA showed more patients would benefit from the DECT-radiological nomogram and DECT model than from the radiological model, which indicated that the DECT quantitative parameters added incremental value to the radiological features in terms of their clinical usefulness.
To the best of the authors’ knowledge, this was the first study to construct a nomogram based on DECT images to differentiate between PTMC and MNG, and this nomogram could serve as an easy-to-use, repeatable, and visual method for assessing individual risk scores in clinic. Some researchers have analyzed the diagnostic ability of high-resolution US (HRUS) using different classification and evaluation systems to diagnose thyroid malignant micronodules, and the AUCs of these systems have ranged from 0.68 to 0.738 (28,33). Conversely, the AUC and specificity of our prediction model was higher than those of HRUS. Moreover, DECT imaging is more objective and can facilitate the preoperative evaluation of lymph node status and extrathyroidal extensions. Thus, efforts should be made to increase the use of DECT in preoperative examinations in clinical settings in the future.
This study had some limitations. First, the analysis was retrospective and conducted at a single institution. More prospective multicenter studies with large sample sizes need to be conducted to validate our results. Second, the different enhanced scan delay times might have affected the IC values; thus, a future optimal contrast-enhanced CT examination protocol for tumor conspicuity is needed. Third, the clinical indicators that radiologists use to determine the overall condition of the thyroid in daily practice to support their diagnoses were not included in our study, and thus further research in this area needs to be conducted.
Conclusions
Our study showed that the DECT quantitative analysis has the potential to differentiate between PTMC and MNG. The DECT-radiological nomogram performed well in the training and validation cohorts and may be used in clinic to guide individualized therapeutic and management decision making.
Acknowledgments
We would like to thank all the volunteers who participated in the study as well as the staff at the Department of Radiology, Chongqing General Hospital, China, for their selfless and valuable assistance.
Funding: This study received funding from the Medical Research Key Program of the Combination of Chongqing National Health Commission and Chongqing Science and Technology Bureau, China (No. 2019ZDXM010) and the Medical Research Program of the Combination of Chongqing National Health Commission and Chongqing Science and Technology Bureau, China (No. 2020FYYX151).
Footnote
Reporting Checklist: The authors have completed the STARD reporting checklist. Available at https://qims.amegroups.com/article/view/10.21037/qims-22-698/rc
Conflicts of Interest: All authors have completed the ICMJE uniform disclosure form (available at https://qims.amegroups.com/article/view/10.21037/qims-22-698/coif). XZ is a staff member at the Department of Clinical Science of Philips Healthcare and assisted with the scientific research for data processing. The other authors have no conflicts of interest to declare.
Ethical Statement: The authors are accountable for all aspects of the work in ensuring that questions related to the accuracy or integrity of any part of the work are appropriately investigated and resolved. This retrospective study was conducted in accordance with the Declaration of Helsinki (as revised in 2013) and was approved by the Ethics Committee of Chongqing General Hospital (No. KY S2022-025-01). The requirement of informed consent from the study participants was waived due to the retrospective nature of this study.
Open Access Statement: This is an Open Access article distributed in accordance with the Creative Commons Attribution-NonCommercial-NoDerivs 4.0 International License (CC BY-NC-ND 4.0), which permits the non-commercial replication and distribution of the article with the strict proviso that no changes or edits are made and the original work is properly cited (including links to both the formal publication through the relevant DOI and the license). See: https://creativecommons.org/licenses/by-nc-nd/4.0/.
References
- Dideban S, Abdollahi A, Meysamie A, Sedghi S, Shahriari M. Thyroid Papillary Microcarcinoma: Etiology, Clinical Manifestations,Diagnosis, Follow-up, Histopathology and Prognosis. Iran J Pathol 2016;11:1-19. [PubMed]
- Haugen BR. 2015 American Thyroid Association Management Guidelines for Adult Patients with Thyroid Nodules and Differentiated Thyroid Cancer: What is new and what has changed? Cancer 2017;123:372-81. [Crossref] [PubMed]
- Du L, Wang Y, Sun X, Li H, Geng X, Ge M, Zhu Y. Thyroid cancer: trends in incidence, mortality and clinical-pathological patterns in Zhejiang Province, Southeast China. BMC Cancer 2018;18:291. [Crossref] [PubMed]
- Hedinger C, Williams ED, Sobin LH. The WHO histological classification of thyroid tumors: a commentary on the second edition. Cancer 1989;63:908-11.
- Zhi J, Zhao J, Gao M, Pan Y, Wu J, Li Y, Li D, Yu Y, Zheng X. Impact of major different variants of papillary thyroid microcarcinoma on the clinicopathological characteristics: the study of 1041 cases. Int J Clin Oncol 2018;23:59-65. [Crossref] [PubMed]
- Malandrino P, Pellegriti G, Attard M, Violi MA, Giordano C, Sciacca L, Regalbuto C, Squatrito S, Vigneri R. Papillary thyroid microcarcinomas: a comparative study of the characteristics and risk factors at presentation in two cancer registries. J Clin Endocrinol Metab 2013;98:1427-34. [Crossref] [PubMed]
- Fisher SB, Perrier ND. The incidental thyroid nodule. CA Cancer J Clin 2018;68:97-105. [Crossref] [PubMed]
- Cappola AR, Mandel SJ. Improving the long-term management of benign thyroid nodules. JAMA 2015;313:903-4. [Crossref] [PubMed]
- Luster M, Aktolun C, Amendoeira I, Barczyński M, Bible KC, Duntas LH, et al. European Perspective on 2015 American Thyroid Association Management Guidelines for Adult Patients with Thyroid Nodules and Differentiated Thyroid Cancer: Proceedings of an Interactive International Symposium. Thyroid 2019;29:7-26. [Crossref] [PubMed]
- Bernet VJ, Chindris AM. Update on the Evaluation of Thyroid Nodules. J Nucl Med 2021;62:13S-9S. [Crossref] [PubMed]
- Persichetti A, Di Stasio E, Coccaro C, Graziano F, Bianchini A, Di Donna V, Corsello S, Valle D, Bizzarri G, Frasoldati A, Pontecorvi A, Papini E, Guglielmi R. Inter- and Intraobserver Agreement in the Assessment of Thyroid Nodule Ultrasound Features and Classification Systems: A Blinded Multicenter Study. Thyroid 2020;30:237-42. [Crossref] [PubMed]
- Lee HJ, Yoon DY, Seo YL, Kim JH, Baek S, Lim KJ, Cho YK, Yun EJ. Intraobserver and Interobserver Variability in Ultrasound Measurements of Thyroid Nodules. J Ultrasound Med 2018;37:173-8. [Crossref] [PubMed]
- Haugen BR, Alexander EK, Bible KC, Doherty GM, Mandel SJ, Nikiforov YE, Pacini F, Randolph GW, Sawka AM, Schlumberger M, Schuff KG, Sherman SI, Sosa JA, Steward DL, Tuttle RM, Wartofsky L. 2015 American Thyroid Association Management Guidelines for Adult Patients with Thyroid Nodules and Differentiated Thyroid Cancer: The American Thyroid Association Guidelines Task Force on Thyroid Nodules and Differentiated Thyroid Cancer. Thyroid 2016;26:1-133. [Crossref] [PubMed]
- Gao M, Ge M, Ji Q, Cheng R, Lu H, Guan H, et al. 2016 Chinese expert consensus and guidelines for the diagnosis and treatment of papillary thyroid microcarcinoma. Cancer Biol Med 2017;14:203-11. [Crossref] [PubMed]
- Simons D, Kachelriess M, Schlemmer HP. Recent developments of dual-energy CT in oncology. Eur Radiol 2014;24:930-9. [Crossref] [PubMed]
- Forghani R. An update on advanced dual-energy CT for head and neck cancer imaging. Expert Rev Anticancer Ther 2019;19:633-44. [Crossref] [PubMed]
- Li M, Zheng X, Li J, Yang Y, Lu C, Xu H, Yu B, Xiao L, Zhang G, Hua Y. Dual-energy computed tomography imaging of thyroid nodule specimens: comparison with pathologic findings. Invest Radiol 2012;47:58-64. [Crossref] [PubMed]
- Lee DH, Lee YH, Seo HS, Lee KY, Suh SI, Ryoo I, You SH, Kim B, Yang KS. Dual-energy CT iodine quantification for characterizing focal thyroid lesions. Head Neck 2019;41:1024-31. [Crossref] [PubMed]
- Li HW, Wu XW, Liu B, Liu WD, Gao N. Clinical values of of gemstone spectral CT in diagnosing thyroid disease. J Xray Sci Technol 2015;23:45-56. [Crossref] [PubMed]
- Jiang L, Liu D, Long L, Chen J, Lan X, Zhang J. Dual-source dual-energy computed tomography-derived quantitative parameters combined with machine learning for the differential diagnosis of benign and malignant thyroid nodules. Quant Imaging Med Surg 2022;12:967-78. [Crossref] [PubMed]
- Gao SY, Zhang XY, Wei W, Li XT, Li YL, Xu M, Sun YS, Zhang XP. Identification of benign and malignant thyroid nodules by in vivo iodine concentration measurement using single-source dual energy CT: A retrospective diagnostic accuracy study. Medicine (Baltimore) 2016;95:e4816. [Crossref] [PubMed]
- Guo BL, Ouyang FS, Ouyang LZ, Liu ZW, Lin SJ, Meng W, Huang XY, Chen HX, Yang SM, Hu QG. Development and validation of an ultrasound-based nomogram to improve the diagnostic accuracy for malignant thyroid nodules. Eur Radiol 2019;29:1518-26. [Crossref] [PubMed]
- Lee YH, Seo HS, Suh SI, Ryoo I, You SH, Son KR, Kwon SY, Son GS, Yang KS. Feasibility Study of a Contrast-Enhanced Multi-Detector CT (64 Channels) Protocol for Papillary Thyroid Carcinoma: The Influence of Different Scan Delays on Tumor Conspicuity. Thyroid 2016;26:726-33. [Crossref] [PubMed]
- Kim BK, Choi YS, Kwon HJ, Lee JS, Heo JJ, Han YJ, Park YH, Kim JH. Relationship between patterns of calcification in thyroid nodules and histopathologic findings. Endocr J 2013;60:155-60. [Crossref] [PubMed]
- Zhang F, Qiao Y, Zhang H. Value of CT Features in the Diagnosis of Papillary Thyroid Tumors in Incidental Thyroid Nodules. Int J Endocrinol 2020;2020:9342317. [Crossref] [PubMed]
- Han ZJ, Shu YY, Lai XF, Chen WH. Value of computed tomography in determining the nature of papillary thyroid microcarcinomas: evaluation of the computed tomographic characteristics. Clin Imaging 2013;37:664-8. [Crossref] [PubMed]
- Wu Q, Wang Y, Li Y, Hu B, He ZY. Diagnostic value of contrast-enhanced ultrasound in solid thyroid nodules with and without enhancement. Endocrine 2016;53:480-8. [Crossref] [PubMed]
- Li X, Gao F, Li F, Han XX, Shao SH, Yao MH, Li CX, Zheng J, Wu R, Du LF. Qualitative analysis of contrast-enhanced ultrasound in the diagnosis of small, TR3-5 benign and malignant thyroid nodules measuring ≤1 cm. Br J Radiol 2020;93:20190923. [Crossref] [PubMed]
- Li B, Zhang Y, Yin P, Zhou J, Jiang T. Ultrasonic features of papillary thyroid microcarcinoma coexisting with a thyroid abnormality. Oncol Lett 2016;12:2451-6. [Crossref] [PubMed]
- Galrão AL, Camargo RY, Friguglietti CU, Moraes L, Cerutti JM, Serrano-Nascimento C, Suzuki MF, Medeiros-Neto G, Rubio IG. Hypermethylation of a New Distal Sodium/Iodide Symporter (NIS) enhancer (NDE) is associated with reduced NIS expression in thyroid tumors. J Clin Endocrinol Metab 2014;99:E944-52. [Crossref] [PubMed]
- Radzina M, Ratniece M, Putrins DS, Saule L, Cantisani V. Performance of Contrast-Enhanced Ultrasound in Thyroid Nodules: Review of Current State and Future Perspectives. Cancers (Basel) 2021.
- Chen HY, Liu WY, Zhu H, Jiang DW, Wang DH, Chen Y, Li W, Pan G. Diagnostic value of contrast-enhanced ultrasound in papillary thyroid microcarcinoma. Exp Ther Med 2016;11:1555-62. [Crossref] [PubMed]
- Gao L, Xi X, Jiang Y, Yang X, Wang Y, Zhu S, Lai X, Zhang X, Zhao R, Zhang B. Comparison among TIRADS (ACR TI-RADS and KWAK- TI-RADS) and 2015 ATA Guidelines in the diagnostic efficiency of thyroid nodules. Endocrine 2019;64:90-6. [Crossref] [PubMed]