Predicting coronary atherosclerosis heart disease with pericoronary adipose tissue attenuation parameters based on dual-layer spectral detector computed tomography: a preliminary exploration
Introduction
Coronary atherosclerosis heart disease (CAD) is a chronic vascular inflammatory condition. Vascular inflammation is strongly associated with the development of atherosclerosis. Inflammation plays a crucial role in the various stages of atherosclerosis, from the formation of lipid stripes to the rupture of vulnerable plaques (1,2). Atherosclerotic plaques form in the walls of coronary arteries and cause coronary stenosis, which is the leading cause of myocardial ischemia. Pericoronary adipose tissue (PCAT) is defined as adipose tissue located within a distance from the outer vessel wall that is equal to the diameter of the coronary vessel. It is part of the epicardial adipose tissue (EAT) (3). PCAT is an active metabolic fat pool that is directly affected by coronary inflammation. Recently, evidence has emerged of a complex bidirectional paracrine pathway between the coronary artery wall and PCAT. Dysfunctional PCAT secretes inflammatory adipokines, causing vascular inflammation and leading to coronary atherosclerotic plaque formation. Vascular inflammation prevents lipid accumulation in PCAT by inhibiting preadipocyte differentiation. The change of adipocyte is reflected as the change in adipose tissue attenuation on computed tomography (CT) images (4-7).
Coronary CT angiography (CCTA) is a principal technique used to screen for CAD. CT imaging can reveal atherosclerotic plaques and PCAT. Coronary artery inflammation can be indirectly detected by evaluating the attenuation of PCAT on CCTA. Research by Dang et al., showed that PCAT attenuation increased with the degree of CAD (8). Higher PCAT attenuation has been associated with an increased risk of acute coronary syndrome (9). Recently, a new noninvasive biomarker of coronary artery inflammation was established: the fat attenuation index (FAI). The FAI is defined as the standardized mean attenuation of PCAT (4,10), and a high FAI is an indicator of increased cardiac mortality. Therefore, quantitative measurement of PCAT attenuation can improve the ability of CCTA to predict and stratify cardiac risk (10).
Most previous studies about PCAT attenuation were based on polyenergetic conventional images. However, virtual monoenergetic images (VMIs) at low energy levels based on dual-energy CT can increase the contrast of soft tissue, and can better display the difference in fat attenuation than conventional images (11). By using dual-layer spectral detector CT (SDCT), a VMI can be reconstructed from a simple conventional scan without additional special scans; therefore, VMIs are convenient to use for clinical applications. Some studies based on dual-layer SDCT have reported that FAI40 keV had higher sensitivity and specificity for detecting differences in PCAT attenuation than did FAI120 kVp (8,12). In the present study, we used SDCT to analyze PCAT attenuation parameters on VMIs (obtained at 40, 50, 60, 70, 80, 90, and 100 keV) and explored whether this method could improve the identification of patients with CAD. In addition, we investigated the relationship between PCAT attenuation parameters and the formation of coronary atherosclerotic plaques. The following article is presented in accordance with the STROBE reporting checklist (available at https://qims.amegroups.com/article/view/10.21037/qims-22-1019/rc).
Methods
Study population
This study was conducted in accordance with the Declaration of Helsinki (as revised in 2013). The study was approved by the Ethics Committee of the First Affiliated Hospital of Harbin Medical University (No. 202214), and informed consent was obtained from all individual participants.
A total of 235 patients who underwent CCTA examination by SDCT for suspected CAD at the First Affiliated Hospital of Harbin Medical University between April 2021 and September 2021 were enrolled in this retrospective cross-sectional study. Patients with symptoms of myocardial ischemia (e.g., chest pain, dyspnea after activity) or electrocardiogram changes were considered suspicious for CAD. According to the following exclusion criteria, 60 patients were excluded: the patient had non-atherosclerotic coronary heart disease; the patient had a history of percutaneous coronary intervention or coronary artery bypass graft; the patient had a malignant tumor or severe inflammatory disease; the CCTA image quality was poor; the patient had incomplete clinical data; or atherosclerotic plaques were present only in the left main coronary artery or in small branches of three main coronary arteries.
The patients were divided into the CAD group and the non-CAD group. Patients were defined as non-CAD if no atherosclerotic plaque was found in major coronary arteries (left anterior descending, LAD; left circumflex, LCX; right coronary artery, RCA) or their branches. Patients were defined as CAD if the atherosclerotic plaque was found in at least one major coronary artery (LAD, LCX, or RCA). Propensity score matching was used to match patients with and without CAD according to their clinical characteristics [age, body mass index (BMI), diabetes, and hyperlipidemia]. The study flowchart is shown in Figure 1.
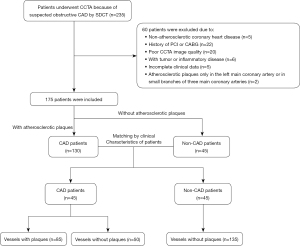
All CCTA images were independently evaluated by two radiologists, both with more than 10 years of experience in cardiac imaging diagnosis. In cases where the two radiologists had different opinions, a consensus was reached through discussion with each other. The following clinical data of patients were collected: BMI; statins use status; history of smoking and drinking; hypertension status; diabetes status; hyperlipidemia status; and the quantitative value of total cholesterol, triglyceride, high-density lipoprotein, and low-density lipoprotein.
Scan protocol and image reconstruction for CCTA
All scans were performed on a 64-slice SDCT scanner (IQon Spectral CT, Philips Healthcare, Best, The Netherlands). Iodine contrast agent was injected (350 mg/mL, 0.8 mL/kg; flow rate 4–5 mL/s, varying according to the vascular status of the patient). A total of 40 mL saline was used before and after the injection of the iodine contrast agent. A retrospective electrocardiogram-gated exam was adopted. The CCTA scan was triggered automatically when the descending aorta reached the 110 HU threshold. All scans were performed according to the following protocol: tube voltage 120 kVp, automatic tube current modulation, slice thickness 0.9 mm, slice interval 0.9 mm, detector collimation 64×0.625 mm, tube rotation time 270 ms, and matrix 512×512. The original data were reconstructed as follows: conventional image, and VMI from 40 to 100 keV with a 10 keV interval. All data were transferred to the post-processing workstation (IntelliSpace Portal vision 10, Philips Healthcare).
Measurement and analysis of PCAT attenuation parameters
The FAI was measured at the proximal RCA, LAD, and LCX by semiautomatic software (ShuKun Technology, Beijing, China). The semiautomatic software automatically segmented coronary arteries and identified PCAT. The measurement starting point and measurement length and width of the PCAT were set manually. The starting point of the RCA measurement was 10 mm from the ostium. The LAD and LCX were measured from the bifurcation of the left main coronary artery. The measurement length was proximal 40 mm of coronary artery, and the measurement width was the mean diameter of the proximal coronary artery. Based on previous studies (11,12), adipose tissue voxels were identified with different thresholds on different images , which were conventional images (−190 to −30 HU), 40 keV (−280 to −40 HU), 50 keV (−260 to −40 HU), 60 keV (−240 to −40 HU), 70 keV (−220 to −30 HU), 80 keV (−210 to −30 HU), 90 keV (−200 to −30 HU), and 100 keV (−190 to −30 HU) . An example of the FAI measurement method is shown in Figure 2. The slope of the spectral attenuation curve (λ) was computed with the following equation: λ40–70 keV = (CT40 keV − CT70 keV)/30, λ40–100 keV = (CT40 keV − CT100 keV)/60, λ70–100 keV = (CT70 keV − CT100 keV)/30. The mean FAI and λ of the LAD, LCX, and RCA were calculated to represent the overall level of the patient. Ultimately, PCAT attenuation parameters (FAI120 kVp, FAIVMI, and λ) were evaluated at the patient and vessel levels.
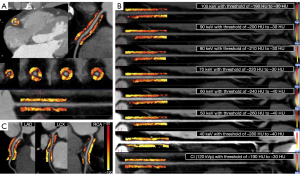
Statistical analysis
PASS 11 software (PASS, Kaysville, UT, USA) was used to calculate the sample size. We hypothesized that PCAT attenuation could effectively distinguish between patients with and without CAD [area under curve (AUC) >0.5]. Pre-experiments showed that the AUC of PCAT attenuation for predicting CAD was 0.7. We determined that α =0.05 and β=0.10, and the sample ratio of the CAD group and the non-CAD group was 1:1. The estimation result showed that each group would require no fewer than 41 patients. To compensate for possible dropouts, 45 patients without CAD and 45 patients with CAD were included in this study. To reduce bias due to confounding variables and to balance the distribution of baseline characteristics, we used propensity score matching, which was performed via logistic regression analysis and included age, BMI, diabetes, and hyperlipidemia. According to the distribution, continuous variables were expressed as the average ± standard deviation (SD), and categorical variables were recorded as numbers (n) and percentages (%). An independent samples t-test was used for comparison at the patient level, while analysis of variance was used for comparison at the vessel level. To evaluate the relationship between PCAT attenuation parameters and CAD, binary logistic regression was implemented. A logical regression model was used to evaluate the ability of PCAT attenuation parameters to predict CAD. Based on logistic regression analysis, the receiver operating characteristic curve was plotted. A P value <0.05 was considered to be statistically significant. SPSS 26.0 (IBM Corp., Armonk, NY, USA) was used for all statistical analysis.
Results
Patients
A total of 90 patients were enrolled in this study, including 45 patients with CAD (85 vessels with plaques and 50 vessels without plaques) and 45 patients without CAD (135 vessels without plaques). The clinical characteristics of the included patients are shown in Table 1. None of the clinical characteristics showed a statistically significant difference between patients with and without CAD (all P values >0.05).
Table 1
Clinical characteristics | Patients with CAD (n=45) | Patients without CAD (n=45) | P value |
---|---|---|---|
Age (years) | 61.18±8.17 | 58.04±9.24 | 0.092 |
Male | 21 [47] | 14 [31] | 0.130 |
BMI (kg/m²) | 25.22±5.56 | 24.72±3.73 | 0.615 |
Statins use | 25 [56] | 17 [38] | 0.091 |
Smoking | 14 [31] | 10 [22] | 0.340 |
Drinking | 8 [18] | 5 [11] | 0.368 |
Hypertension | 24 [53] | 18 [40] | 0.205 |
Diabetes | 11 [24] | 5 [11] | 0.098 |
Hyperlipidemia | 8 [18] | 10 [22] | 0.598 |
TC (mmol/L) | 4.63±1.06 | 4.58±1.29 | 0.812 |
TG (mmol/L) | 1.75±1.18 | 1.59±0.91 | 0.484 |
HDL (mmol/L) | 1.15±0.23 | 1.18±0.27 | 0.505 |
LDL (mmol/L) | 2.83±0.82 | 2.86±1.08 | 0.883 |
Continuous variables are described as the average ± SD; categorical variables are described as numbers (n) and percentages [%]. CAD, coronary atherosclerotic heart disease; BMI, body mass index; TC, total cholesterol; TG, triglyceride; HDL, high-density lipoprotein; LDL, low-density lipoprotein; SD, standard deviation.
Differences in PCAT attenuation parameters between patients with and without CAD
FAI120 kVp was significantly higher in patients with CAD than in patients without CAD (−72.64±5.94 vs. −77.19±3.68 HU; P<0.001). FAIVMI differed significantly between patients with and without CAD at each monoenergetic level (all P values <0.05), with the difference being more obvious on low-energy VMIs, especially at 40 keV (−128.93±13.03 vs. −138.47±8.29 HU; P<0.001; Figure 3A; Table 2). The shape of the PCAT spectral attenuation curve was similar for the two groups of patients, but the curve location in the CAD group was significantly higher than that in the non-CAD group. The λ in the CAD group was also higher than that in the non-CAD group (all P values <0.01; Figure 3B,3C; Table 2).
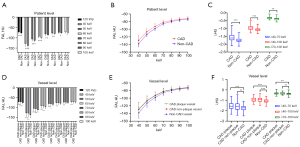
Table 2
PCAT attenuation parameters | Patient level | Vessel level | ||||||||||
---|---|---|---|---|---|---|---|---|---|---|---|---|
CAD group (n=45) | Non-CAD group (n=45) | P value | CAD plaque vessels (n=85) | CAD non-plaque vessels (n=50) | P value† | Non-CAD vessels (n=135) | P value‡ | P value§ | F | P value¶ | ||
Conventional image | ||||||||||||
FAI120 kVp (HU) | −72.64±5.94 | −77.19±3.68 | <0.001 | −72.60±7.56 | −72.72±7.57 | 0.929 | −77.19±5.81 | <0.001 | <0.001 | 15.34 | <0.001 | |
VMI | ||||||||||||
FAI40 keV (HU) | −128.93±13.03 | −138.47±8.29 | <0.001 | −128.59±16.67 | −129.52±19.03 | 0.768 | −138.47±14.21 | <0.001 | 0.001 | 12.08 | <0.001 | |
FAI50 keV (HU) | −106.67±8.85 | −113.13±6.89 | <0.001 | −106.25±12.13 | −107.38±12.71 | 0.610 | −113.13±10.83 | <0.001 | 0.003 | 10.59 | <0.001 | |
FAI60 keV (HU) | −92.99±6.99 | −98.42±5.76 | <0.001 | −92.73±9.36 | −93.44±9.93 | 0.678 | −98.43±8.51 | <0.001 | 0.001 | 12.26 | <0.001 | |
FAI70 keV (HU) | −82.15±5.98 | −85.68±4.50 | 0.002 | −81.89±8.11 | −82.60±8.27 | 0.626 | −85.69±6.86 | <0.001 | 0.011 | 7.56 | 0.001 | |
FAI80 keV (HU) | −77.39±5.18 | −79.99±3.84 | 0.008 | −77.27±6.85 | −77.60±7.10 | 0.791 | −79.99±5.76 | 0.002 | 0.020 | 5.64 | 0.004 | |
FAI90 keV (HU) | −74.33±4.74 | −76.25±3.38 | 0.029 | −74.08±5.99 | −74.74±6.51 | 0.552 | −76.25±5.08 | 0.004 | 0.099 | 4.12 | 0.017 | |
FAI100 keV (HU) | −72.16±4.36 | −73.87±3.14 | 0.036 | −71.92±5.51 | −72.56±5.74 | 0.521 | −73.86±4.61 | 0.005 | 0.112 | 4.01 | 0.019 | |
λ | ||||||||||||
40–70 keV | −1.56±0.28 | −1.76±0.17 | <0.001 | −1.56±0.35 | −1.57±0.42 | 0.916 | −1.76±0.30 | <0.001 | 0.004 | 11.42 | <0.001 | |
40–100 keV | −0.94±0.17 | −1.08±0.12 | <0.001 | −0.94±0.22 | −0.95±0.25 | 0.910 | −1.07±0.20 | <0.001 | <0.001 | 12.10 | <0.001 | |
70–100 keV | −0.33±0.07 | −0.39±0.09 | 0.001 | −0.33±0.12 | −0.34±0.11 | 0.913 | −0.39±0.13 | 0.001 | 0.005 | 8.03 | <0.001 |
Continuous variables are described as average ± SD. P value†, the difference between “CAD plaque vessels” and “CAD non-plaque vessels”; P value‡, the difference between “CAD plaque vessels” and “non-CAD vessels”; P value§, the difference between “CAD non-plaque vessels” and “non-CAD vessels”; P value¶, analysis of variance. PCAT, pericoronary adipose tissue; CAD, coronary atherosclerotic heart disease; FAI, fat attenuation index; VMI, virtual monoenergetic image; λ, slope of spectral attenuation curve; SD, standard deviation.
Differences in PCAT attenuation parameters between vessels
At the vessel level, all coronary arteries were divided into three groups according to the existence of coronary atherosclerotic plaques: CAD vessels without plaques, CAD vessels with plaques, and non-CAD vessels. There were obvious differences in the FAI and λ between the groups (all P values <0.01). Compared with non-CAD vessels, CAD vessels (with or without plaques) had a significantly higher FAI120 kVp, FAIVMI (except for FAI90 keV and FAI100 keV), and λ (all P values <0.05; Table 2). The PCAT attenuation of CAD plaque vessels was increased compared to that of CAD non-plaque vessels, but the differences were not statistically significant (all P values >0.05; Figure 3D; Table 2). As shown in Figure 3E, the spectral attenuation curves representing CAD vessels with and without plaques (green curve and red curve, respectively) almost overlap, and their locations are higher than that of the curve representing non-CAD vessels (blue curve). The λ of CAD vessels with and without plaques was also higher than that of non-CAD vessels (Figure 3F).
Receiver operating characteristic curve analysis for the prediction of CAD
Binary logistic regression analysis showed that FAIVMI on each monoenergetic level, the λ of each energetic interval, and FAI120 kVp were all independent risk factors for CAD [all P values <0.05 and odds ratio (OR) >1; Table 3]. Receiver operating characteristic curve analysis was used to evaluate the established regression model of FAIVMI, FAI120 kVp, λ, and a combination of FAIVMI, FAI120 kVp, and λ; the AUCs were 0.8123, 0.7444, 0.7230, and 0.8296, respectively. The FAIVMI model contained FAI40 keV, FAI50 keV, FAI60 keV, FAI70 keV, and FAI80 keV. The λ model contained λ40–70 keV, λ40–100 keV, and λ70–100 keV. The combination model obtained the highest AUC, with a sensitivity of 0.6444 and a specificity of 0.9111 (Figure 4).
Table 3
PCAT attenuation parameters | B | SE | P value | OR (95% CI) |
---|---|---|---|---|
Conventional image | ||||
FAI120 keV (HU) | 0.194 | 0.053 | <0.001 | 1.214 (1.094–1.348) |
VMI | ||||
FAI40 keV (HU) | 0.087 | 0.025 | 0.001 | 1.091 (1.038–1.145) |
FAI50 keV (HU) | 0.105 | 0.031 | 0.001 | 1.111 (1.045–1.182) |
FAI60 keV (HU) | 0.134 | 0.039 | 0.001 | 1.144 (1.060–1.234) |
FAI70 keV (HU) | 0.128 | 0.044 | 0.004 | 1.137 (1.042–1.241) |
FAI80 keV (HU) | 0.128 | 0.051 | 0.011 | 1.136 (1.029–1.255) |
FAI90 keV (HU) | 0.116 | 0.054 | 0.033 | 1.123 (1.009–1.249) |
FAI100 keV (HU) | 0.121 | 0.059 | 0.040 | 1.128 (1.006–1.266) |
λ | ||||
40–70 keV | 0.039 | 0.012 | 0.001 | 1.040 (1.017–1.064) |
40–100 keV | 0.064 | 0.018 | <0.001 | 1.066 (1.029–1.106) |
70–100 keV | 0.089 | 0.030 | 0.003 | 1.093 (1.031–1.158) |
PCAT, pericoronary adipose tissue; CAD, coronary atherosclerotic heart disease; SE, standard error; OR, odds ratio; CI, confidence interval; FAI, fat attenuation index; VMI, virtual monoenergetic image; λ, slope of spectral attenuation curve.
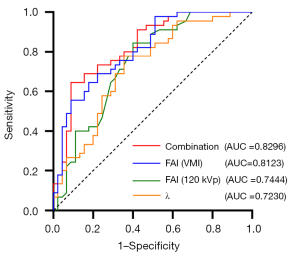
Discussion
In this retrospective cross-sectional study, we found that PCAT attenuation parameters in patients with CAD were higher than those in patients without CAD. The performance in predicting CAD based on PCAT attenuation was better when VMIs were used than when conventional images were used (AUC =0.8123 vs. 0.7444). However, the highest AUC (0.8296) was achieved when PCAT attenuation parameters obtained from both VMIs and conventional images were combined with λ. Simultaneously, our study showed that PCAT attenuation parameters of coronary arteries without atherosclerotic plaques in patients with CAD were higher than those of vessels in patients in the non-CAD group. Compared with conventional imaging, SDCT could provide more information for the prediction of atherosclerotic plaque formation based on PCAT attenuation.
Inflammation is key to the formation of atherosclerosis plaques (13). The deposition and accumulation of low-density lipoprotein at the inner wall of the artery gives rise to inflammatory reactions. Aggregations of monocytes and lymphocytes and the release of proinflammatory cytokines (such as interleukin-6, interleukin-8, and tumor necrosis factor-alpha) lead to atherosclerosis (13-15). The traditional “inside-out” theory regards atherosclerosis as a result of vascular inflammation caused by intimal injury (13,16). However, recent studies have proposed the “outside-in” theory, according to which inflammation begins in PCAT and spreads to blood vessels (17-19). Due to the complex bidirectional influence of PCAT and the coronary artery wall (4), it is critical that noninvasive imaging methods are used to detect coronary inflammation. Some studies have demonstrated that PCAT inflammation can be noninvasively evaluated by positron emission tomography/CT (20-22). The results showed that 18F-fluorodeoxyglucose uptake of PCAT in patients with CAD was higher than that in patients without CAD (20). PCAT around high-risk plaques increased density was associated with focal 18F-sodium fluoride uptake, it had more 18F-sodium fluoride uptake than PCAT around non-high-risk plaques (21,22). However, the method is limited by its poor spatial resolution, conspicuous background noise, and exorbitant price; consequently, the acceptability of its clinical application is low. In a landmark study, Antonopoulos et al. (4) obtained adipose tissue from around the RCA in patients who underwent coronary artery bypass graft surgery and confirmed that adipose tissue CT attenuation was negatively related to fatty acid binding protein-4 (FABP4) gene expression and the average adipocyte size. Both FABP4 expression and adipocyte size are affected by vascular inflammation: the more severe the vascular inflammation, the lower expression of FABP4 and the lower size of adipocytes in perivascular adipose tissue. Therefore, to reflect the size and lipid content of local adipocytes, researchers developed the CT FAI. By measuring the FAI, the inflammation level of the corresponding vascular segments can be inferred. In another recent study about coronary inflammation, PCAT attenuation of the RCA in patients with diabetes was significantly higher than that in non-diabetic patients (−83.60±9.51 vs. −88.58±9.37 HU; P<0.001) (23). Diabetes may induce PCAT dysfunction, which promotes the secretion of inflammatory adipokines and cytokines to the vascular wall and causes specific focal vascular wall inflammation (3,24). In the present study, to eliminate the influence of potential clinical confounding factors, such as diabetes, we used propensity score matching. Ultimately, logistic regression analysis showed a significant correlation between PCAT attenuation parameters and CAD in our study.
At the patient level, our study revealed that the FAI of patients with CAD was significantly higher than that of patients in the non-CAD group. Another recent study also showed a difference in the FAI of patients with and without CAD (P=0.001) (4). In that study, patients with CAD were defined as having obstructive coronary artery disease (>50% stenosis) in the RCA. However, we defined patients with CAD as those who had atherosclerotic plaques in three main coronary arteries regardless of the degree of coronary artery stenosis. Compared with previous study mentioned, the purpose of adopting different criteria for CAD in this study was to compare of the FAI between patients with and without coronary atherosclerosis, and to explore the relationship between FAI and formation of coronary atherosclerotic plaque.
Vascular inflammation plays a pivotal role in coronary atherosclerotic plaque rupture and acute coronary syndrome occurrence (13,25), and the detection of coronary inflammation has important implications for cardiovascular risk stratification. A CRIP-CT study showed that an increased FAI of the proximal RCA has a high predictive value for cardiac mortality (10). Patients with acute coronary syndrome and stable coronary artery disease were compared in a single-center retrospective study (9), which demonstrated that PCAT attenuation around culprit lesions was significantly higher than that around non-culprit lesions (−69.1 vs. −74.8 HU; P=0.01). Another study that showed PCAT attenuation in stable patients with CAD was significantly higher than that in the non-CAD control group (−90.6±5.7 vs. −95.8±6.2 HU; P=0.01) (26), which was similar to the findings in our study. It is worth noting that the EAT attenuation of patients with CAD is not always higher than that of patients without CAD. In a recent study, Ma et al. (27) found there was no conspicuous difference in EAT attenuation between patients with and without CAD (−95.6±7.8 vs. −96.2±7.1 HU; P=0.644). This result may have occurred because the study only detected adipose tissue within 1 mm of the outer wall of coronary vessels with a width of 1 mm. A deficiency of adipose tissue does not accurately reflect the holistic situation of PCAT. Ma et al. (27) also found that PCAT attenuation around plaques was observably higher than proximal PCAT attenuation of non-plaque vessels. This finding suggests that lesion-specific PCAT attenuation may have a stronger correlation with the presence of atherosclerotic plaques than does proximal PCAT attenuation. However, another study found that overall EAT attenuation in patients with obstructive CAD was significantly lower than that in patients without obstructive CAD {−86 HU [interquartile range (IQR): −88 to −82 HU] vs. −84 HU (IQR: −87 to −82 HU); P=0.0486} (28). Decreased adipose tissue attenuation has been proven to be caused by adipocyte hypertrophy and lipid accumulation. Antonopoulos et al. (4) found that the EAT attenuation value decreased gradually from 1 mm away to 20 mm away from the vascular wall. This finding means that the non-PCAT part of EAT may have a lower CT attenuation value than the PCAT part. This gradient change in EAT attenuation means that the overall EAT attenuation is influenced by the overall EAT volume. Furthermore, another study demonstrated that interstitial fibrosis in adipose tissue led to increased EAT attenuation (29). In patients with a lower EAT volume, the effect of fibrosis on EAT attenuation exceeded that of adipocyte hypertrophy. Conversely, in patients with a higher EAT volume, the effect of adipocyte hypertrophy on EAT attenuation exceeded that of fibrosis, resulting in lower EAT attenuation. Liu et al. (30) further demonstrated that EAT attenuation was negatively correlated with EAT volume. Based on the above studies, we speculated that patients with CAD with more EAT were likely to have lower EAT attenuation than patients without CAD. However, this hypothesis requires further observation in a future study.
Few studies have explored the difference in PCAT attenuation in patients with coronary diseases before and after coronary atherosclerotic plaque formation (27,31). At the vessel level, our study found that the FAI of CAD vessels with or without plaques was significantly higher than that of non-CAD vessels. This finding was consistent with that of a previous study (27). The PCAT attenuation of CAD vessels with atherosclerotic plaque was higher than that of non-CAD vessels because of coronary inflammation, and the PCAT attenuation of CAD vessels without atherosclerotic plaque was also increased. The mechanism in patients with CAD may be that inflammation of plaque-free vessels comes from adjacent vessels with plaque or is caused by adverse vascular factors, such as vascular damage. Coronary artery inflammation generally arises in the early stages of coronary endothelial dysfunction. Vascular inflammation formation precedes atherosclerotic plaques (15,32). Thus, an increase in PCAT attenuation may precede the formation of atherosclerotic plaque. In a follow-up study, patients underwent sequential CCTA (scan interval 3.4±1.6 years) (7), and increased PCAT attenuation in the proximal RCA was associated with the progression of uncalcified plaques (NCP; r=0.55; P<0.001). The attenuation of PCAT varies with the development of coronary heart disease. Based on the above results and discussion, we believe that the formation and progression of atherosclerotic plaques are closely related to increased PCAT attenuation. An increased FAI in vessels without atherosclerotic plaques might predict atherosclerotic plaque formation. This finding will benefit early personalized intervention for individuals with an increased cardiovascular risk. A previous study confirmed that higher PCAT attenuation was related to the characteristics of high-risk plaques and was also related to 18F-sodium fluoride uptake in positron emission tomography (22). These findings further demonstrate the reliability of PCAT attenuation as an indicator of coronary artery inflammation. Compared with the proximal LAD and LCX, the proximal RCA has fewer branches and more PCAT. Most previous studies have regarded PCAT attenuation of the proximal RCA as a marker of overall coronary artery inflammation (7,10); however, there are frequent differences in atherosclerotic plaque distribution between different vascular branches. In our study, three main coronary arteries were included simultaneously to more accurately reflect the relationship between atherosclerotic plaque formation and PCAT attenuation.
To the best of our knowledge, this is the first study to explore the differences in PCAT attenuation parameters between coronary arteries with and without atherosclerotic plaques using SDCT. This is also the first study to systematically compare PCAT attenuation on conventional images and different spectral monoenergetic images (40, 50, 60, 70, 80, 90, and 100 keV) between patients with and without CAD. In addition, we built CAD prediction models based on conventional images and VMIs, and found that a combined model improved the performance of CAD prediction. Spectral detector CT is the newest generation dual-energy CT that uses a dual-layer detector to simultaneously collect low- and high-energy data. It achieves accurate “homologous, simultaneous, codirectional, and synchronous” energy spectrum scanning. By using a conventional scan on SDCT, we can retrospectively obtain any VMI from 40 to 200 keV. The low-energy VMI can improve image quality and effectively reduce the radiation dose and the amount of contrast agents (33,34). It can also reflect the differences in X-ray absorption by different tissue components and reveal differences in fat attenuation more effectively (11,35). Previous studies have reported that VMIs at 40 keV showed higher discriminatory power for detecting changes in PCAT attenuation than did conventional images (8,12), which was further confirmed in our study. The CT attenuation of adipose tissue increases obviously from 40 to 100 keV. When the energy exceeds 100 keV, the spectral attenuation curve tends to smooth. Therefore, we selected VMIs obtained at seven energy levels (from 40 to 100 keV) to analyze PCAT attenuation and plotted spectral curves. The shape of the spectral attenuation curve varies with the tissue attenuation characteristics (36); thus, the spectral curve and the slope of the curve could offer qualitative and quantitative analyses. The results showed that the spectral curve and λ were significantly different at both the patient level and the vessel level. In other words, the spectral curve and λ could provide important information regarding the presence of atherosclerotic plaques, and they may be predictive risk factors for CAD. However, our study results also showed that the FAI90 keV and FAI100 keV of vessels without plaques in patients with CAD were not significantly higher than those of vessels without plaques in patients without CAD (all P values >0.05). The human body has different absorption rates for different keV X-rays. Low keV X-rays can be absorbed by tissues more easily and show higher CT attenuation values, while most high keV X-rays can penetrate the human body and thus show lower CT attenuation values. High keV images can suppress beam hardening artifacts from high-density components, but a decrease in the CT attenuation values will cause the difference between adipose tissue to become smaller. Compared to reducing image artifacts, keeping the density resolution of adipose tissues is more meaningful for studying the correlation between the FAI and CAD. Therefore, based on the results of this study, we suggest that VMIs above 90 keV are not suitable for FAI analysis. By establishing the receiver operating characteristic curve, we found that the combination model and FAIVMI achieved higher AUCs for detecting CAD than did FAI120 kVp. These findings show that using SDCT to detect PCAT attenuation parameters can better distinguish patients with CAD from patients without CAD.
There are some limitations to this study. First, this was a small-sample, single-center, retrospective study. As a consequence, the causal relationship between the formation of atherosclerotic plaque and PCAT attenuation parameters could not be directly explained by our current work. This causal relationship needs to be verified by further larger sample sizes and multiple centers studies. Second, our study did not discuss the relationship between PCAT attenuation parameters and cardiac events, plaque characteristics (including the amount of plaque, coronary artery calcification score, plaque type, and plaque burden). Furthermore, the current study did not have available follow-up data. Therefore, a follow-up study is needed in order to further assess the prognostic potential of the PCAT attenuation parameters. Third, relevant inflammatory factors (such as C-reactive protein, interleukin-6, interleukin-8, and tumor necrosis factor-alpha) were not collected from the patients in this study. Therefore, the level of coronary inflammation could not be judged comprehensively. Although PCAT attenuation parameters are expected to become a new biomarker for evaluating the change in patients after anti-inflammatory treatment, further randomized controlled trials are required to verify this hypothesis.
Conclusions
PCAT attenuation parameters based on dual-layer SDCT can better distinguish patients with CAD from patients without CAD. These attenuation parameters may become valuable predictors for the formation of atherosclerotic plaques, which could make early detection of subclinical CAD and the identification of high-risk patients without coronary atherosclerosis heart disease possible.
Acknowledgments
Funding: None.
Footnote
Reporting Checklist: The authors have completed the STROBE reporting checklist. Available at https://qims.amegroups.com/article/view/10.21037/qims-22-1019/rc
Conflicts of Interest: All authors have completed the ICMJE uniform disclosure form (available at https://qims.amegroups.com/article/view/10.21037/qims-22-1019/coif). SD, an employee of Philips Healthcare, contributed to experimental design and the description of technical principles without impacting research results. The other authors have no conflicts of interest to declare.
Ethical Statement: The authors are accountable for all aspects of the work in ensuring that questions related to the accuracy or integrity of any part of the work are appropriately investigated and resolved. This study was conducted in accordance with the Declaration of Helsinki (as revised in 2013). The study was approved by the ethics committee of the First Affiliated Hospital of Harbin Medical University (No. 202214), and informed consent was obtained from all individual participants.
Open Access Statement: This is an Open Access article distributed in accordance with the Creative Commons Attribution-NonCommercial-NoDerivs 4.0 International License (CC BY-NC-ND 4.0), which permits the non-commercial replication and distribution of the article with the strict proviso that no changes or edits are made and the original work is properly cited (including links to both the formal publication through the relevant DOI and the license). See: https://creativecommons.org/licenses/by-nc-nd/4.0/.
References
- Galkina E, Ley K. Immune and inflammatory mechanisms of atherosclerosis Annu Rev Immunol 2009;27:165-97. [Crossref] [PubMed]
- Hansson GK. Inflammation, atherosclerosis, and coronary artery disease. N Engl J Med 2005;352:1685-95. [Crossref] [PubMed]
- Guglielmo M, Lin A, Dey D, Baggiano A, Fusini L, Muscogiuri G, Pontone G. Epicardial fat and coronary artery disease: Role of cardiac imaging. Atherosclerosis 2021;321:30-8. [Crossref] [PubMed]
- Antonopoulos AS, Sanna F, Sabharwal N, Thomas S, Oikonomou EK, Herdman L, et al. Detecting human coronary inflammation by imaging perivascular fat. Sci Transl Med 2017;9:eaal2658. [Crossref] [PubMed]
- Mancio J, Oikonomou EK, Antoniades C. Perivascular adipose tissue and coronary atherosclerosis. Heart 2018;104:1654-62. [Crossref] [PubMed]
- Goeller M, Achenbach S, Duncker H, Dey D, Marwan M. Imaging of the Pericoronary Adipose Tissue (PCAT) Using Cardiac Computed Tomography: Modern Clinical Implications. J Thorac Imaging 2021;36:149-61. [Crossref] [PubMed]
- Goeller M, Tamarappoo BK, Kwan AC, Cadet S, Commandeur F, Razipour A, Slomka PJ, Gransar H, Chen X, Otaki Y, Friedman JD, Cao JJ, Albrecht MH, Bittner DO, Marwan M, Achenbach S, Berman DS, Dey D. Relationship between changes in pericoronary adipose tissue attenuation and coronary plaque burden quantified from coronary computed tomography angiography. Eur Heart J Cardiovasc Imaging 2019;20:636-43. [Crossref] [PubMed]
- Dang Y, Chen X, Ma S, Ma Y, Ma Q, Zhou K, Liu T, Wang K, Hou Y. Association of Pericoronary Adipose Tissue Quality Determined by Dual-Layer Spectral Detector CT With Severity of Coronary Artery Disease: A Preliminary Study. Front Cardiovasc Med 2021;8:720127. [Crossref] [PubMed]
- Goeller M, Achenbach S, Cadet S, Kwan AC, Commandeur F, Slomka PJ, Gransar H, Albrecht MH, Tamarappoo BK, Berman DS, Marwan M, Dey D. Pericoronary Adipose Tissue Computed Tomography Attenuation and High-Risk Plaque Characteristics in Acute Coronary Syndrome Compared With Stable Coronary Artery Disease. JAMA Cardiol 2018;3:858-63. [Crossref] [PubMed]
- Oikonomou EK, Marwan M, Desai MY, Mancio J, Alashi A, Hutt Centeno E, et al. Non-invasive detection of coronary inflammation using computed tomography and prediction of residual cardiovascular risk (the CRISP CT study): a post-hoc analysis of prospective outcome data. Lancet 2018;392:929-39. [Crossref] [PubMed]
- Rodriguez-Granillo GA, Capunay C, Deviggiano A, De Zan M, Carrascosa P. Regional differences of fat depot attenuation using non-contrast, contrast-enhanced, and delayed-enhanced cardiac CT. Acta Radiol 2019;60:459-67. [Crossref] [PubMed]
- Chen X, Dang Y, Hu H, Ma S, Ma Y, Wang K, Liu T, Lu X, Hou Y. Pericoronary adipose tissue attenuation assessed by dual-layer spectral detector computed tomography is a sensitive imaging marker of high-risk plaques. Quant Imaging Med Surg 2021;11:2093-103. [Crossref] [PubMed]
- Libby P, Ridker PM, Maseri A. Inflammation and atherosclerosis. Circulation 2002;105:1135-43. [Crossref] [PubMed]
- Libby P. Inflammation in atherosclerosis. Arterioscler Thromb Vasc Biol 2012;32:2045-51. [Crossref] [PubMed]
- Ross R. Atherosclerosis—an inflammatory disease. N Engl J Med 1999;340:115-26. [Crossref] [PubMed]
- Kawabe J, Hasebe N. Role of the vasa vasorum and vascular resident stem cells in atherosclerosis. Biomed Res Int 2014;2014:701571. [Crossref] [PubMed]
- Britton KA, Fox CS. Perivascular adipose tissue and vascular disease. Clin Lipidol 2011;6:79-91. [Crossref] [PubMed]
- Ansaldo AM, Montecucco F, Sahebkar A, Dallegri F, Carbone F. Epicardial adipose tissue and cardiovascular diseases. Int J Cardiol 2019;278:254-60. [Crossref] [PubMed]
- Knudson JD, Dick GM, Tune JD. Adipokines and coronary vasomotor dysfunction. Exp Biol Med (Maywood) 2007;232:727-36. [PubMed]
- Mazurek T, Kobylecka M, Zielenkiewicz M, Kurek A, Kochman J, Filipiak KJ, Mazurek K, Huczek Z, Królicki L, Opolski G. PET/CT evaluation of (18)F-FDG uptake in pericoronary adipose tissue in patients with stable coronary artery disease: Independent predictor of atherosclerotic lesions’ formation? J Nucl Cardiol 2017;24:1075-84. [Crossref] [PubMed]
- Joshi NV, Vesey AT, Williams MC, Shah AS, Calvert PA, Craighead FH, Yeoh SE, Wallace W, Salter D, Fletcher AM, van Beek EJ, Flapan AD, Uren NG, Behan MW, Cruden NL, Mills NL, Fox KA, Rudd JH, Dweck MR, Newby DE. 18F-fluoride positron emission tomography for identification of ruptured and high-risk coronary atherosclerotic plaques: a prospective clinical trial. Lancet 2014;383:705-13. [Crossref] [PubMed]
- Kwiecinski J, Dey D, Cadet S, Lee SE, Otaki Y, Huynh PT, Doris MK, Eisenberg E, Yun M, Jansen MA, Williams MC, Tamarappoo BK, Friedman JD, Dweck MR, Newby DE, Chang HJ, Slomka PJ, Berman DS. Peri-Coronary Adipose Tissue Density Is Associated With (18)F-Sodium Fluoride Coronary Uptake in Stable Patients With High-Risk Plaques. JACC Cardiovasc Imaging 2019;12:2000-10. [Crossref] [PubMed]
- Yu Y, Ding X, Yu L, Dai X, Wang Y, Zhang J. Increased coronary pericoronary adipose tissue attenuation in diabetic patients compared to non-diabetic controls: A propensity score matching analysis. J Cardiovasc Comput Tomogr 2022;16:327-35. [Crossref] [PubMed]
- Lin A, Dey D, Wong DTL, Nerlekar N. Perivascular Adipose Tissue and Coronary Atherosclerosis: from Biology to Imaging Phenotyping. Curr Atheroscler Rep 2019;21:47. [Crossref] [PubMed]
- Libby P, Theroux P. Pathophysiology of coronary artery disease. Circulation 2005;111:3481-8. [Crossref] [PubMed]
- Lin A, Nerlekar N, Yuvaraj J, Fernandes K, Jiang C, Nicholls SJ, Dey D, Wong DTL. Pericoronary adipose tissue computed tomography attenuation distinguishes different stages of coronary artery disease: a cross-sectional study. Eur Heart J Cardiovasc Imaging 2021;22:298-306. [Crossref] [PubMed]
- Ma R, van Assen M, Ties D, Pelgrim GJ, van Dijk R, Sidorenkov G, van Ooijen PMA, van der Harst P, Vliegenthart R. Focal pericoronary adipose tissue attenuation is related to plaque presence, plaque type, and stenosis severity in coronary CTA. Eur Radiol 2021;31:7251-61. [Crossref] [PubMed]
- Pandey NN, Sharma S, Jagia P, Kumar S. Epicardial fat attenuation, not volume, predicts obstructive coronary artery disease and high risk plaque features in patients with atypical chest pain. Br J Radiol 2020;93:20200540. [Crossref] [PubMed]
- Muir LA, Neeley CK, Meyer KA, Baker NA, Brosius AM, Washabaugh AR, Varban OA, Finks JF, Zamarron BF, Flesher CG, Chang JS, DelProposto JB, Geletka L, Martinez-Santibanez G, Kaciroti N, Lumeng CN, O’Rourke RW. Adipose tissue fibrosis, hypertrophy, and hyperplasia: Correlations with diabetes in human obesity. Obesity (Silver Spring) 2016;24:597-605. [Crossref] [PubMed]
- Liu Z, Wang S, Wang Y, Zhou N, Shu J, Stamm C, Jiang M, Luo F. Association of epicardial adipose tissue attenuation with coronary atherosclerosis in patients with a high risk of coronary artery disease. Atherosclerosis 2019;284:230-6. [Crossref] [PubMed]
- Marwan M, Hell M, Schuhbäck A, Gauss S, Bittner D, Pflederer T, Achenbach S. CT Attenuation of Pericoronary Adipose Tissue in Normal Versus Atherosclerotic Coronary Segments as Defined by Intravascular Ultrasound. J Comput Assist Tomogr 2017;41:762-7. [Crossref] [PubMed]
- Choi BJ, Matsuo Y, Aoki T, Kwon TG, Prasad A, Gulati R, Lennon RJ, Lerman LO, Lerman A. Coronary endothelial dysfunction is associated with inflammation and vasa vasorum proliferation in patients with early atherosclerosis. Arterioscler Thromb Vasc Biol 2014;34:2473-7. [Crossref] [PubMed]
- Yi Y, Zhao XM, Wu RZ, Wang Y, Vembar M, Jin ZY, Wang YN. Low Dose and Low Contrast Medium Coronary CT Angiography Using Dual-Layer Spectral Detector CT. Int Heart J 2019;60:608-17. [Crossref] [PubMed]
- Huang X, Gao S, Ma Y, Lu X, Jia Z, Hou Y. The optimal monoenergetic spectral image level of coronary computed tomography (CT) angiography on a dual-layer spectral detector CT with half-dose contrast media. Quant Imaging Med Surg 2020;10:592-603. [Crossref] [PubMed]
- Ehn S, Sellerer T, Muenzel D, Fingerle AA, Kopp F, Duda M, Mei K, Renger B, Herzen J, Dangelmaier J, Schwaiger BJ, Sauter A, Riederer I, Renz M, Braren R, Rummeny EJ, Pfeiffer F, Noël PB. Assessment of quantification accuracy and image quality of a full-body dual-layer spectral CT system. J Appl Clin Med Phys 2018;19:204-17. [Crossref] [PubMed]
- Tatsugami F, Higaki T, Nakamura Y, Honda Y, Awai K. Dual-energy CT: minimal essentials for radiologists. Jpn J Radiol 2022;40:547-59. [Crossref] [PubMed]