Comparative study of pathological response evaluation systems after neoadjuvant chemotherapy for breast cancer: developing predictive models of multimodal ultrasound features including shear wave elastography combined with puncture pathology
Introduction
Neoadjuvant chemotherapy (NAC) permits less extensive breast and axillary surgery by downstaging the tumor and allows monitoring of the treatment response, has been the standard therapeutic option for patients with locally advanced breast cancer over the past 10 years (1,2). The advantages of NAC are a reduction in tumor burden, increased possibility of breast and axillary conservation surgery, and improved survival of patients as indicated by pathologic complete response (pCR) of the breast or axilla (3). Increasingly, the presence or absence of residual disease is being used to guide decisions in adjuvant therapy following NAC. However, some patients with breast cancer harbor residual tumor after NAC. Currently, there are various pathologic classification methods to evaluate therapy efficacy and residual breast cancer. The Miller-Payne grading (MPG) system is widely accepted, frequently used, and is an independent predictor of disease-free survival (DFS) or overall survival (OS). The MPG assesses the relative treatment response by comparing the cellularity of tumors before and after NAC (4). In contrast to the MPG, which does not take into account tumor size and nodal status, the residual tumor can be quantified using the residual cancer burden (RCB) calculation. The RCB was first described in 2007 and provides a set of standard techniques to assess and quantify the degree of residual cancer in the breast and axillary lymph nodes after NAC (5).
Shear wave elastography (SWE) is an imaging technique for visualizing and quantifying tissue stiffness (6). In addition to conventional ultrasound features, SWE provides an easily repeatable, real-time, in vivo assessment of tissue elasticity (7). Studies exploiting the association between tumor stiffness, carcinogenesis, and disease progression have reported high mean stiffness values in breast cancer to be significantly associated with lymph node involvement, large invasive size, aggressive subtypes, and high histologic grade (8-10). In addition, it has been found that increasing extracellular matrix stiffness activates and/or inactivates specific transcription factors in cancer and stromal cells to regulate cancer progression and chemotherapeutic resistance (11,12).
Several studies have related SWE characteristics to the MPG system (13) or RCB (14,15), but fewer studies have compared SWE characteristics with various pathological response assessment systems. Moreover, no studies have evaluated the additional diagnostic utility of SWE in conjunction with conventional ultrasound. Therefore, the aims of this study were to compare SWE features before NAC with the MPG system and RCB, to analyze the relationships between these features and pathological response assessment systems, and to assess the predictive performance of multimodal models for different pathological response assessment systems after NAC in combination with conventional ultrasound and puncture pathology results. We present the following article in accordance with the TRIPOD reporting checklist (available at https://qims.amegroups.com/article/view/10.21037/qims-22-910/rc).
Methods
Study design and patient cohort
The study was conducted in accordance with the Declaration of Helsinki (as revised in 2013) and was approved by the institutional review board of Gansu Cancer Hospital. Due retrospective nature of the study, the written informed consent was waived.
Eligible patients (n=161), namely those treated with NAC and subsequent surgical resection of primary invasive breast cancer at Gansu Cancer Hospital between January 2021 and June 2022 were identified from clinical records. The specific inclusion criteria were as follows: (I) breast cancer diagnosed with histopathology of core needle biopsy (CNB) before chemotherapy with routine ultrasound and SWE examinations of the lesion; (II) clinical stage IIa–IIIc; (III) normal results on routine examinations (routine blood, liver, kidney, heart, and lung function) before chemotherapy, excluding distant metastases; and (IV) no history of local radiotherapy or systemic chemotherapy. Patients with breast lesions >5 cm in diameter or who were unable to undergo SWE were excluded from the study. Of the 161 patients initially identified from the clinical records, 16 were excluded (14 with masses >5 cm in diameter and 2 who were unable to undergo ultrasound examination due to skin breakdown). Thus, 145 patients (mean age 49.6±9.0 years; age range 26–74 years) were included in the study. The pretreatment tumor size ranged from 13 to 50 mm (mean size 27.08±9.6 mm). All patients underwent conventional ultrasound and SWE before NAC, and the pathological response was assessed with postoperative results after NAC (Figure 1).
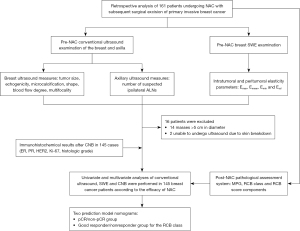
Conventional ultrasound and SWE
Conventional ultrasound pictures of the breast and axilla were obtained using the Resona 7 Premium Ultrasound System (Mindray, Shenzhen, China). The ultrasound examinations were performed by 2 radiologists (X. M. Song, W. L. Wang), with a combined experience of >5 years in breast examination and at least 1 year of specialized training in breast radiology. Conventional ultrasound images were acquired by thoroughly scanning the bilateral breast and axillary both transversely and longitudinally. Based on the fifth edition of the American College of Radiology (ACR) Breast Imaging-Reporting and Data System (BI-RADS) classification (16), the size (the largest of 3 diameters), echogenicity, microcalcification, shape, and blood flow of the primary breast tumor were recorded. In addition, the number of suspected ipsilateral axillary lymph nodes was recorded.
We have provided a detailed description of the method of SWE examination of breast lesions elsewhere (17). Using grayscale velocity dual mode, the outer edge of the lesion was tracked on grayscale images, and internal elasticity parameters were automatically calculated, including maximum (Emax), mean (Emean), minimum (Emin), and standard deviation (Esd) elasticity. Then, Emax, Emean, Emin, and Esd values at shells of 2 mm from the edge of the lesion were measured (18,19). In the case of multiple ipsilateral breast tumors, only the largest lesion was evaluated.
Treatment
Indications for NAC were based on the opinion of breast surgeons, and NAC was performed according to national guidelines (20). All patients received 6–8 cycles of anthracycline- and/or taxane-based chemotherapy. Patients with human epidermal growth factor receptor 2 (HER2)–positive disease also received trastuzumab + pertuzumab. All patients underwent breast-conserving therapy or mastectomy after NAC.
Reference test: pathology
Pathological examinations and immunohistochemistry (IHC) of the CNB specimen before and the surgical specimen after NAC were performed by dedicated breast pathologists. The MPG system, the RCB scores, and RCB score components, which was evaluated from the resection specimens by a specialized breast pathologist (Y. Q. Kang) were compared with the elasticity values from SWE of breast lesions obtained prior to NAC. The pathologist was blinded to the SWE findings. In this study, pCR was defined as the absence of residual invasive tumor cells (ductal carcinoma in situ may have been present) in the breast. The measurement of residual tumor size included both invasive and noninvasive components. By comparing cancer cellularity in the pretreatment CNB with that in the posttreatment resected tumor, the MPG system was used to evaluate tumor response based on the reduction in tumor cellularity, with results graded from 1 (no reduction in overall cellularity) to 5 (no invasive malignant cells identifiable in sections from the site of the tumor) (4). To calculate the RCB, the percentage of invasive cancer cellularity, residual tumor size, the size of the largest axillary metastasis, and the number of positive lymph nodes were evaluated. On a continuous scale, RCB was quantified and further divided into categories 0 (=pCR), I (minimal residual disease), II (moderate residual disease), and III (extensive residual disease).
All masses underwent IHC, which included detection of estrogen (ER) and progesterone (PR) receptors, HER2 overexpression and/or amplification, and the Ki-67 labeling index, allowing for the classification of the tumor subtypes. For HER2-positive masses, HER2 gene amplification was detected with strong staining (3+) of an intact membrane and/or fluorescence in situ hybridization (FISH) in more than 10% of cells detected with IHC. The intrinsic molecular subtypes of breast cancer were classified as luminal A, luminal B (HER2−), HER2 positive (HR+), HER2 positive (HR−), and TNBC (21). The ER- and/or PR-positive, HER2-negative, and low–Ki-67 (<20%) masses were categorized as luminal A. ER- and/or PR-positive, HER2-negative, and high (≥20%) Ki-67 masses were categorized as luminal B (HER2−). ER- and/or PR-positive, HER2-overexpressing/amplified, and any Ki-67 were categorized as HR+. The HER2-overexpressing/amplified and ER- and PR-negative masses were categorized as HR−. ER-, PR-, and HER2-negative masses were categorized as TNBC.
Statistical analysis
The MPG, age, postoperative pathological characteristics, and RCB scores (and components thereof) were correlated with pretreatment stiffness readings using Pearson’s correlation coefficient (r). All elasticity results were used for the statistical analysis. Prior to analysis, tests to determine the normality of distribution of the outcome variable were conducted.
In the analysis of the reactions to NAC, pathologic responses were categorized as pCR or non-pCR, MPG good responders or nonresponders, and RCB good responders or nonresponders. MPG good responders were defined as patients with grades 3, 4, and 5, whereas nonresponders were defined as those with grades 1 and 2. RCB nonresponders were defined as classes II and III, whereas RCB good responders were defined as classes 0 and I. These 3 dichotomizations and the chi-squared test, Mann-Whitney test, and Fisher exact test were used to compare baseline patient, pathological, and ultrasound characteristics of the study population. Variables that showed a univariate significance with a pCR or good response were entered into a binary logistic regression analysis using a forward stepwise method to ascertain independent risk factors and establish prediction models. Receiver operating characteristic (ROC) curves obtained with MedCalc (MedCalc Software Ltd., Ostend, Belgium) were used to evaluate the models’ performance. The corresponding area under the curve (AUC), sensitivity, and specificity were calculated at the optimal cutoff values.
All statistical analyses were performed using SPSS version 25.0 (IBM Corporation, Armonk, NY, USA). P<0.05 two-tailed was considered significant.
Results
SWE parameters and pathological response assessment system
We recruited 145 patients with breast cancer treated with NAC to the study: 128 with invasive ductal carcinoma, 5 with invasive lobular carcinoma, 6 with papillary carcinoma, 3 with squamous cell carcinoma, 2 with medullary carcinoma, and 1 with metaplastic carcinoma. Table 1 summarizes the distribution of RCB scores and MPG for breast cancer subgroups and histological types. There was marked variability in RCB scores for patients with breast cancer of different molecular subtypes: the HR− group had a lower RCB score, whereas the luminal A group had a higher RCB score.
Table 1
RCB score (mean) | No. tumors | Miller-Payne grade | |||||
---|---|---|---|---|---|---|---|
1 | 2 | 3 | 4 | 5 | |||
Molecular subtype | |||||||
HER2+ (HR−) | 0.358 | 20 | 0 | 0 | 2 | 1 | 17 |
HER2+ (HR+) | 1.050 | 27 | 0 | 0 | 7 | 8 | 12 |
TNBC | 1.391 | 23 | 0 | 2 | 8 | 5 | 8 |
Luminal A | 2.254 | 34 | 0 | 7 | 19 | 6 | 2 |
Luminal B (HER2−) | 2.206 | 41 | 0 | 6 | 22 | 5 | 8 |
P value | <0.001 | ||||||
Histological type | |||||||
Ductal | 1.517 | 128 | 0 | 10 | 48 | 24 | 46 |
Lobular | 2.433 | 5 | 0 | 2 | 2 | 1 | 0 |
Squamous cell | 1.725 | 3 | 0 | 0 | 2 | 0 | 1 |
Papillary | 2.852 | 6 | 0 | 2 | 4 | 0 | 0 |
Other | 1.987 | 3 | 0 | 1 | 2 | 0 | 0 |
P value | 0.484 |
RCB, residual cancer burden; HER2, human epidermal growth factor receptor 2; HR, hormone receptor; TNBC, triple-negative breast cancer.
Correlations of intratumor Emax, Emean, Emin, and Esd with age, MPG, RCB score, and components of the RCB score are presented in Table 2. In the correlation analysis, only the RCB score was significantly associated with Emax (r=−0.176; 95% CI: −0.346 to 0.002; P=0.034). Statistically significant associations were observed between Emean and both MPG and RCB score components. The correlation between Emean and RCB score was stronger (r=−0.277; P=0.001; 95% CI: −0.429 to −0.114) than that between Emean and MPG (r=0.129; 95% CI: −0.002 to 0.260; P=0.042), RCB class (r=−0.184; 95% CI: −0.318 to −0.047; P=0.004), the size of the largest axillary metastasis (r=−0.246; 95% CI: −0.398 to −0.080; P=0.003), the number of positive nodes (r=−0.231; 95% CI: −0.379 to −0.065; P=0.005), and invasive size (bidimensional diameters of the primary tumor bed in the resection specimen) (r=−0.170; 95% CI: −0.313 to −0.031; P=0.041). There were no significant correlations analysis between any of these indicators and either Emin or Esd.
Table 2
Emax | Emean | Emin | Esd | ||||||||
---|---|---|---|---|---|---|---|---|---|---|---|
r | P value | r | P value | r | P value | r | P value | ||||
Age | −0.101 | 0.227 | −0.148 | 0.075 | −0.086 | 0.306 | 0.103 | 0.217 | |||
MPG | 0.088 | 0.164 | 0.129 | 0.042 | 0.012 | 0.855 | −0.052 | 0.411 | |||
RCB score | −0.176 | 0.034 | −0.277 | 0.001 | −0.126 | 0.131 | 0.075 | 0.371 | |||
RCB class | −0.103 | 0.104 | −0.184 | 0.004 | −0.082 | 0.197 | 0.039 | 0.542 | |||
Size of the largest axillary metastasis | −0.157 | 0.059 | −0.246 | 0.003 | −0.156 | 0.060 | 0.060 | 0.477 | |||
No. positive nodes | −0.134 | 0.108 | −0.231 | 0.005 | −0.159 | 0.056 | 0.041 | 0.628 | |||
Invasive size | −0.121 | 0.146 | −0.170 | 0.041 | −0.049 | 0.559 | 0.104 | 0.215 |
MPG, Miller-Payne grade; RCB, residual cancer burden; Emax, maximum elasticity; Emean, mean elasticity; Emin, minimum elasticity; Esd, standard deviation elasticity.
Correlations of peritumor Emax, Emean, Emin, and Esd with age, MPG, RCB score, and RCB score components are presented in Table 3. Only the RCB score was significantly correlated with Emax (r=−0.189; 95% CI: −0.353 to −0.029; P=0.023). Statistically significant correlations were observed between Esd and age, MPG, and RCB score components. Statistically significant correlations were also observed between Emean and RCB score components (all P values <0.05).
Table 3
Emax | Emean | Emin | Esd | ||||||||
---|---|---|---|---|---|---|---|---|---|---|---|
r | P value | r | P value | r | P value | r | P value | ||||
Age | −0.135 | 0.106 | −0.146 | 0.080 | 0.046 | 0.581 | −0.176 | 0.035 | |||
MPG | 0.096 | 0.132 | 0.123 | 0.051 | −0.062 | 0.328 | 0.126 | 0.047 | |||
RCB score | −0.189 | 0.023 | −0.284 | 0.001 | −0.048 | 0.564 | −0.235 | 0.005 | |||
RCB class | −0.116 | 0.067 | −0.163 | 0.010 | −0.007 | 0.908 | −0.139 | 0.029 | |||
Size of largest axillary metastasis | −0.126 | 0.130 | −0.232 | 0.005 | −0.121 | 0.148 | −0.178 | 0.033 | |||
Number of positive nodes | −0.139 | 0.096 | −0.257 | 0.002 | −0.136 | 0.103 | −0.187 | 0.024 | |||
Invasive size | −0.156 | 0.060 | −0.199 | 0.016 | 0.074 | 0.374 | −0.233 | 0.005 |
MPG, Miller-Payne grade; RCB, residual cancer burden; Emax, maximum elasticity; Emean, mean elasticity; Emin, minimum elasticity; Esd, standard deviation elasticity.
Pathologic response assessment system evaluations of NAC
Of the 145 patients, 47 (32.4%) obtained a pCR. According to the MPG system, 0 and 15 (10.3%) reached grades 1 and 2, respectively, and these patients were categorized as nonresponders. In the MPG system, 58 (40.0%), 25 (17.2%), and 47 (32.4%) patients, were categorized as grade 3, 4, and 5, respectively, and these patients were considered good responders. Of the 145 patients, 48 (33.1%), 14 (9.7%), 59 (40.7%), and 24 (16.6%) were classified as RCB class 0, I, II, and III, respectively; those in class II and III were considered nonresponders, and those in class 0 and I were considered good responders. These response categories were used to compare the pathological and ultrasound characteristics (Table 4). Tumor diameter, ER, PR, HER2, Ki-67 levels, histologic grade, number of suspected lymph nodes, intratumor Emean, and peritumoral Esd differed significantly between the pCR and non-pCR groups (P<0.05). In addition, all these characteristics, except peritumoral Esd, differed significantly between RCB good responders and nonresponders (P<0.05). However, intratumor Emean and peritumoral Esd did not differ significantly between MPG good responders and nonresponders.
Table 4
Pathologic response | Miller-Payne grade | RCB class | |||||||||
---|---|---|---|---|---|---|---|---|---|---|---|
pCR (n=47) | Non-pCR (n=98) | P value | Good responder (n=130) | Nonresponder (n=15) | P value | Good responder (n=62) | Nonresponder (n=83) | P value | |||
Age (years) | 49.4±10.0 | 49.7±8.5 | 0.825 | 49.4±9.0 | 49.7±8.1 | 0.287 | 48.4±10.0 | 50.5±8.0 | 0.207 | ||
Tumor size (mm) | 23.9±8.7 | 28.8±9.9 | 0.013 | 26.1±9.7 | 31.4±8.2 | 0.042 | 24.1±8.0 | 27.9±10.3 | 0.010 | ||
Echogenicity | 0.577 | 0.513 | 0.705 | ||||||||
Nonhypoechoic | 10 (28.6) | 25 (71.4) | 32 (94.1) | 2 (5.9) | 14 (40.0) | 21 (60.0) | |||||
Hypoechoic | 37 (33.6) | 73 (66.4) | 98 (88.3) | 13 (11.7) | 48 (43.6) | 62 (56.4) | |||||
Tumor shape | 0.405 | 0.140 | 0.508 | ||||||||
Regular | 4 (23.5) | 13 (76.5) | 13 (76.5) | 4 (23.5) | 6 (35.3) | 11 (64.7) | |||||
Irregular | 43 (33.6) | 85 (66.4) | 117 (91.4) | 11 (8.6) | 56 (43.8) | 72 (56.2) | |||||
Microcalcification | 0.716 | 0.571 | 0.337 | ||||||||
No | 13 (30.2) | 30 (69.8) | 40 (93.0) | 3 (7.0) | 21 (48.8) | 22 (51.2) | |||||
Yes | 34 (33.3) | 68 (66.7) | 90 (88.2) | 12 (11.8) | 41 (40.2) | 61 (59.8) | |||||
Blood flow | 0.398 | 0.390 | 0.607 | ||||||||
0–I | 35 (30.7) | 79 (69.3) | 104 (91.2) | 10 (8.8) | 50 (43.9) | 64 (56.1) | |||||
II–III | 12 (38.7) | 19 (61.3) | 26 (83.9) | 5 (16.1) | 12 (38.7) | 19 (61.3) | |||||
Multifocality | 0.450 | 0.790 | 0.449 | ||||||||
No | 39 (33.9) | 76 (66.1) | 104 (90.4) | 11 (9.6) | 51 (44.3) | 64 (55.7) | |||||
Yes | 8 (26.7) | 22 (73.3) | 26 (86.7) | 4 (13.3) | 11 (36.7) | 19 (63.3) | |||||
ER | <0.001 | 0.245 | <0.001 | ||||||||
Negative | 25 (58.1) | 18 (41.9) | 41 (95.3) | 2 (4.7) | 29 (67.4) | 14 (32.6) | |||||
Positive | 22 (21.6) | 80 (78.4) | 89 (87.3) | 13 (12.7) | 33 (32.4) | 69 (67.6) | |||||
PR | <0.001 | 0.309 | 0.004 | ||||||||
Negative | 36 (47.4) | 40 (52.6) | 70 (92.1) | 6 (7.9) | 41 (53.9) | 35 (46.1) | |||||
Positive | 11 (15.9) | 58 (84.1) | 60 (87.0) | 9 (13.0) | 21 (30.4) | 48 (69.6) | |||||
HER2 | <0.001 | 0.022 | <0.001 | ||||||||
Negative | 18 (18.6) | 79 (81.4) | 83 (85.6) | 14 (14.4) | 29 (29.9) | 68 (70.1) | |||||
Positive | 29 (60.4) | 19 (39.6) | 47 (97.9) | 1 (2.1) | 33 (68.7) | 15 (31.3) | |||||
Ki-67 (%) | 41.9±19.1 | 31.3±19.3 | 0.001 | 35.7±19.7 | 24.1±19.6 | 0.037 | 41.9±21.3 | 31.3±16.8 | <0.001 | ||
Histologic grade | 0.016 | 0.679 | 0.001 | ||||||||
II | 24 (25.5) | 70 (74.5) | 85 (90.4) | 9 (9.6) | 31 (33.0) | 63 (67.0) | |||||
III | 23 (45.1) | 28 (54.9) | 45 (88.2) | 6 (11.8) | 31 (60.8) | 20 (39.2) | |||||
No. suspicious lymph nodes | 0.046 | 0.001 | 0.032 | ||||||||
0 | 8 (22.9) | 27 (77.1) | 27 (77.1) | 8 (22.9) | 14 (40.0) | 21 (60.0) | |||||
1–2 | 28 (43.1) | 37 (56.9) | 64 (98.5) | 1 (1.5) | 35 (53.8) | 30 (46.2) | |||||
≥3 | 11 (24.4) | 34 (75.6) | 39 (86.7) | 6 (13.3) | 13 (28.9) | 32 (71.1) | |||||
Intratumor Emean | 85.19±12.58 | 67.15±10.58 | 0.015 | 45.16±10.71 | 47.25±14.79 | 0.603 | 46.50±12.06 | 43.43±8.94 | 0.042 | ||
Peritumoral Esd | 31.92±16.43 | 24.87±13.03 | 0.008 | 11.16±10.36 | 27.91±17.13 | 0.907 | 29.27±15.17 | 25.70±14.07 | 0.098 |
Unless indicated otherwise, data are expressed as the mean ± SD or n (%). RCB, residual cancer burden; pCR, pathologic complete response; ER, estrogen receptor; PR, progesterone receptor; HER2, human epidermal growth factor receptor 2; Emean, mean elasticity; Esd, standard deviation elasticity.
Multivariate analysis combining pretreatment conventional ultrasound, SWE, and CNB results for assessment of pathological response in breast cancer
We developed 2 prediction models: one for the pCR/non-pCR groups and another for the RCB good responders/nonresponders. The MPG response groups were excluded because there were no significant differences in stiffness parameters between them. For all statistically significant variables in conventional ultrasound, SWE, and relevant histopathological variables, binary logistic regression was used.
According to the findings of binary logistic regression analysis (Table 5), the independent predictors of pCR/non-pCR were HER2 (95% CI: 0.058–0.358; P<0.001), PR (95% CI: 1.971–13.578; P=0.001), histologic grade (95% CI: 0.162–0.989; P=0.047), Ki-67 (95% CI: 3.245–281.011; P=0.003), and intratumor Emean (95% CI: 1.019–1.112; P=0.005). The regression equation established was follows:
Table 5
Characteristic | B | SE | Wald | P value | OR (95% CI) |
---|---|---|---|---|---|
HER2 | −1.934 | 0.463 | 17.452 | <0.001 | 0.145 (0.058−0.358) |
PR | 1.644 | 0.492 | 11.148 | 0.001 | 5.174 (1.971–13.578) |
Histologic grade | −0.916 | 0.461 | 3.938 | 0.047 | 0.400 (0.162−0.989) |
Ki-67 | 3.408 | 1.138 | 8.965 | 0.003 | 30.196 (3.245−281.011) |
Intratumor Emean | 0.063 | 0.022 | 7.745 | 0.005 | 1.065 (1.019−1.112) |
Constant | −4.113 | 1.327 | 9.599 | 0.002 |
OR, odds ratio; HER2, human epidermal growth factor receptor 2; PR, progesterone receptor; Emean, mean elasticity.
The categorical independent risk predictor variables were assigned a value of “1” if present and “0” if absent.
According to the findings of binary logistic regression analysis (Table 6), the independent predictors of RCB good responders/nonresponders were HER2 (95% CI: 0.087–0.517; P=0.001), ER (95% CI: 1.597–11.436; P=0.004), histological grade (95% CI: 0.129–0.778; P=0.012), tumor diameter (95% CI: 0.870–0.980; P=0.008), Ki-67 (95% CI: 3.388–335.772; P=0.003), and intratumoral Emean (95% CI: 0.999–1.089; P=0.055). The regression equation established was as follows:
Table 6
Characteristic | B | SE | Wald | P value | OR (95% CI) |
---|---|---|---|---|---|
HER2 | −1.551 | 0.455 | 11.610 | 0.001 | 0.212 (0.087−0.517) |
ER | 1.453 | 0.502 | 8.368 | 0.004 | 4.274 (1.597−11.436) |
Histologic grade | −1.148 | 0.457 | 6.298 | 0.012 | 0.317 (0.129−0.778) |
Tumor diameter | −0.080 | 0.030 | 7.003 | 0.008 | 0.923 (0.870−0.980) |
Ki-67 | 3.518 | 1.173 | 9.004 | 0.003 | 33.728 (3.388−335.772) |
Intratumor Emean | 0.042 | 0.022 | 3.692 | 0.055 | 1.043 (0.999−1.089) |
Constant | −0.025 | 1.319 | 0.000 | 0.985 |
OR, odds ratio; HER2, human epidermal growth factor receptor 2; ER, estrogen receptor; Emean, mean elasticity.
The categorical independent risk predictor variables were assigned a value of “1” if present and “0” if absent.
Nomogram establishment and evaluation
Based on the findings of the regression study, a nomogram was created to forecast the pathogenic response (Figures 2,3). The model had an AUC of 0.855 (95% CI: 0.787–0.922) in discriminating between the pCR and non-pCR groups. The AUC for discriminating between the RCB good responders/nonresponders was 0.845 (95% CI: 0.780–0.910). A concordance between the projected value and the actual value was evident in the calibration curve (Figures 4,5). We used the nomogram in 2 patients to demonstrate its use (Figures 6,7).
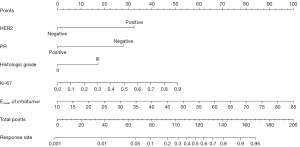
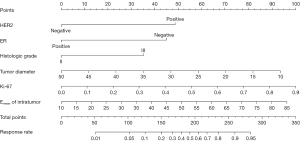
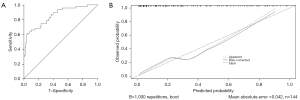
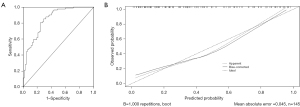
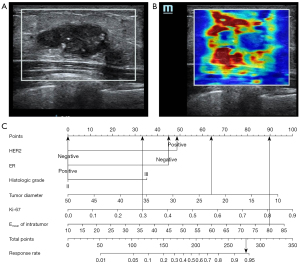
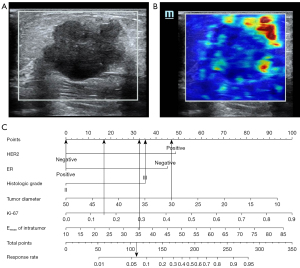
Discussion
We observed a statistically significant correlation between pretreatment breast cancer tissue elasticity measured with SWE and the pathologic response evaluation systems after NAC in daily clinical practice. This study compared both the MPG and RCB systems with the SWE intratumor and peritumor features; our results showed that intratumor Emean and peritumoral Esd differed significantly according to the grade in the MPG system, class in the RCB system, and different components of the RCB score. Furthermore, based on the efficacy evaluation of different pathological response assessment systems, this study compared the pathological findings of CNB and ultrasound features before NAC to screen for statistically significant parameters. Ultimately, 2 prediction models were developed by performing binary logistic regression analysis for all significant variables: one for the pCR/non-pCR group and the other for the RCB good responders/nonresponders. The AUC for the pCR/non-pCR group was 0.855 (sensitivity 63.8%, specificity 93.7%) and that for the RCB good responders/nonresponders was 0.845 (sensitivity 85.2%, specificity 71.0%). The MPG system was excluded because no significant differences were found in stiffness parameters across grades.
The MPG system includes changes in cellularity between the biopsy and resected specimen but excludes lymph node responses. In contrast, ypTNM stage, residual breast and lymph node lesions, RCB, Sataloff stage, and Chevallier classification have been used to assess the features of residual cancer in breast parenchymal and lymph nodes (22,23). The RCB system was developed in 2007. Symmans et al. (5) showed that RCB is a prognostic marker independent of the main chemotherapy type, with significant differences between RCB categories in hormone receptor-positive (ER+ and/or PR+, HER2−) breast cancer, TNBC (ER−, PR−, HER2−) and HER2-positive (hormone receptor-positive or -negative) breast cancer. The present study also found there to be significant differences in the distribution of RCB scores for breast cancer subgroups. According to Choi et al. (24), only RCB had prognostic value for all 4 immunohistochemical subtypes in terms of OS and DFS. This suggests that a pathologic classification system that assesses residual tumors in the breast and lymph nodes after NAC is better correlated with clinical outcome.
Ultrasound has multiple advantages over magnetic resonance imaging (MRI) and positron emission tomography/computed tomography in terms of clinical reproducibility. Grayscale ultrasound is generally used to measure changes in tumor size after NAC. However, most researchers have shown that ultrasound underestimates the size of breast tumors. The reduced prediction efficacy is likely due to the limited capacity of ultrasound to discriminate viable tumor tissue from fibrotic scar tissue. Previous studies (25-28) have found tumor stiffness assessed with SWE to be associated with the proportion of patients receiving NAC who experienced a pCR; their results further showed that elastography had a substantially higher ability to predict the effectiveness of NAC than did grayscale ultrasound and was approximately on par with contrast-enhanced ultrasound and MRI in this regard. Other studies have reported that elastography could predict NAC efficacy with a sensitivity of 59–100%, specificity of 63–100%, accuracy of 74–83%, positive predictive value (PPV) of 71–79%, negative predictive value (NPV) of 66–86%, and AUC of 0.75–0.88 (2,3). It may be a helpful tool for assessing lesions because NAC alters the stiffness of breast cancers by reducing cancer cellularity and vascularity in addition to causing fibrotic changes in the tumor stroma (7). The MPG system and RCB scores, which were used in the present study to evaluate chemotherapy response, have been found to produce richer predictive information when subjected to multivariate analysis. We analyzed the correlation between SWE parameters and MPG, RCB class, and RCB score components and showed that intratumor Emean and peritumoral Esd were significantly correlated with the above assessment of NAC indicators. In contrast with MPG, the RCB score offers a continuous variable of therapy response. Interestingly, both the RCB class and MPG suggest that a good response to chemotherapy is associated with an initial “greater stiffness” of breast cancer. Recent research reported that the maximum area of stiffness in malignant tumors was consistently found in the peritumoral stroma rather than inside the tumor (29,30). Potential causes of the “stiff rim” sign have been suggested to be a desmoplastic response and infiltration of tumor cells into the peritumoral stroma (2,31). As a consequence, the tissues surrounding the tumor’s edge or periphery are often stiffer (referred to as the “stiff rim”) (32), increasing their stiffness by a factor of 2–10 or more (33). Both intratumoral and peritumoral SWE parameters were included in the present study, and statistical analysis showed that patients with breast cancer with a higher stiffness are more likely to achieve pCR after NAC, which may be related to the fact that TNBC and HER2-positive tumors exhibit more stiffness than do ER-positive tumors (34). Ultimately, intratumor Emean was retained in the prediction models because of its good discriminatory ability. In this retrospective study, while collecting SWE parameters from patients, we found that some patients did not undergo SWE after NAC. Therefore, only pretreatment data were used in this study (see Limitations, below).
Thus far, dynamic contrast-enhanced MRI has been demonstrated to provide the most accurate evaluation of tumor response following NAC (35-37). Some reports have focused on the diagnostic performance of MRI and ultrasound images after NAC in predicting the response to NAC in patients with breast cancer (13,29). However, MRI has its limitations because it cannot be used in all patients because of the expense, the need for intravenous contrast injection, and other contraindications, such as renal insufficiency, claustrophobia, and the presence of ferromagnetic metal next to a vital structure. In the present study, we explored parameters with good pathological responsiveness to NAC by characterizing grayscale ultrasound, SWE, and puncture pathology findings of breast masses before treatment in order to create a simple and convenient efficacy prediction model before NAC. The findings of binary logistic regression analysis revealed that the independent predictive factors of pCR/non-pCR were HER2, PR, histologic grade, Ki-67, and intratumor Emean; independent predictive factors of RCB class good responders/nonresponders were HER2, ER, histological grade, size, Ki-67, and intratumoral Emean. Grayscale ultrasound features, including echogenicity, mass shape, microcalcifications, blood flow, and multifocality, were not included in either prediction model. The findings indicated that the initial conventional ultrasound features of breast cancer may not be useful as predictors to evaluate the efficacy of NAC. However, further analysis is needed to explore the dynamic changes in these features. The number of suspicious axillary lymph nodes (0, 1–2, ≥3) was an independent risk factor in univariate analysis but was excluded from the regression equation, possibly due to the stronger correlation between NAC and pathological findings. The same was true for “size” in the pCR/non-pCR groups. It has been reported that the presence of microcalcifications on imaging is associated with residual disease after NAC in TNBC (38). In the present study, the correlation between the presence of microcalcifications on grayscale ultrasound images and pathological residuals after NAC was evaluated in all patients with molecular subtypes (and not only TNBC), with no significant differences being found. Some promising results were obtained when we analyzed the primary lesion for puncture pathology. In both prediction models developed in this study, HER-2, PR, ER, Ki-67, and histologic grade were statistically significant in univariate analysis. In contrast, PR− showed good predictive performance for pCR/non-pCR in the multivariate analysis. Boland et al. (39) reported that in the ER+ and HER2− subgroups, PR negativity increased the chance of pCR in the breast 4-fold compared with the PR+ subgroup. Although the specific subtype of breast cancer in this study was not qualified, it is easy to see that HER2+ and PR− are positively correlated with breast pCR. When the RCB class was used to assess the pathological response of breast cancer, HER2+ and ER− were associated with better chemotherapy responses. In addition, we noted that the 95% CI of Ki-67 was too wide in the binary logistic regression analysis. We then reviewed the literature on the predictive value of Ki-67 before NAC in breast cancer. In a meta-analysis (40) that included 53 studies (10,848 patients), high Ki-67 before NAC was found to be related to a greater number of pCR events, regardless of subtype, Ki-67 cutoffs, and the definition of pCR. Furthermore, a nomogram including the Ki-67 index had good discriminatory ability in predicting the pCR of breast cancer on MRI (41). We speculated that there may be stronger predictors in the binary logistic regression analysis, which would result in a wider 95% CI for Ki-67. Therefore, we decided to keep Ki-67 in the prediction model after careful consideration of the reports in the literature.
Limitations
This study has some limitations. First, there is inevitable bias due to the limited sample size and single-center observation study design. The accuracy of our forecasting model requires validation in a multicenter prospective trial with a larger sample size. The addition of clinicopathological factors, such as tumor subtypes, to the response to NAC may improve model performance. However, no subgroup analysis of specific molecular types of breast cancer was performed in our prediction model. Second, only the initial SWE parameters of pretreatment breast cancer were considered in this study, and dynamic changes in grayscale ultrasound and SWE during NAC were not included. Third, previous studies have demonstrated the predictive value of axillary lymph node status for NAC (42-44). The present study investigated the number of ultrasound-suspected axillary lymph nodes based on RCB score components. Other indices, such as lymph node shape, fatty hilum, and cortical thickness, were not included, and their potential usefulness for assessing efficacy after NAC is unclear.
Conclusions
The preoperative nomogram based on multimodal ultrasound features and primary lesion biopsy results can effectively guide clinicians in predicting pathologic response after NAC for breast cancer and has potential implications for individualized treatment.
Acknowledgments
Funding: This study was supported by Lanzhou Science and Technology Plan Project (No. 2021-1-90).
Footnote
Reporting Checklist: The authors have completed the TRIPOD reporting checklist. Available at https://qims.amegroups.com/article/view/10.21037/qims-22-910/rc
Conflicts of Interest: All authors have completed the ICMJE uniform disclosure form (available at https://qims.amegroups.com/article/view/10.21037/qims-22-910/coif). The authors have no conflicts of interest to declare.
Ethical Statement: The authors are accountable for all aspects of the work in ensuring that questions related to the accuracy or integrity of any part of the work are appropriately investigated and resolved. The study was conducted in accordance with the Declaration of Helsinki (as revised in 2013) and approved by the institutional review board of Gansu Cancer Hospital. The need for written informed consent was waived due to the retrospective nature of the study.
Open Access Statement: This is an Open Access article distributed in accordance with the Creative Commons Attribution-NonCommercial-NoDerivs 4.0 International License (CC BY-NC-ND 4.0), which permits the non-commercial replication and distribution of the article with the strict proviso that no changes or edits are made and the original work is properly cited (including links to both the formal publication through the relevant DOI and the license). See: https://creativecommons.org/licenses/by-nc-nd/4.0/.
References
- Yau C, Osdoit M, van der Noordaa M, Shad S, Wei J, de Croze D, et al. Residual cancer burden after neoadjuvant chemotherapy and long-term survival outcomes in breast cancer: a multicentre pooled analysis of 5161 patients. Lancet Oncol 2022;23:149-60. [Crossref] [PubMed]
- Lee SH, Chang JM, Han W, Moon HG, Koo HR, Gweon HM, Kim WH, Noh DY, Moon WK. Shear-Wave Elastography for the Detection of Residual Breast Cancer After Neoadjuvant Chemotherapy. Ann Surg Oncol 2015;22:S376-84. [Crossref] [PubMed]
- Wang J, Chu Y, Wang B, Jiang T. A Narrative Review of Ultrasound Technologies for the Prediction of Neoadjuvant Chemotherapy Response in Breast Cancer. Cancer Manag Res 2021;13:7885-95. [Crossref] [PubMed]
- Ogston KN, Miller ID, Payne S, Hutcheon AW, Sarkar TK, Smith I, Schofield A, Heys SD. A new histological grading system to assess response of breast cancers to primary chemotherapy: prognostic significance and survival. Breast 2003;12:320-7. [Crossref] [PubMed]
- Symmans WF, Peintinger F, Hatzis C, Rajan R, Kuerer H, Valero V, Assad L, Poniecka A, Hennessy B, Green M, Buzdar AU, Singletary SE, Hortobagyi GN, Pusztai L. Measurement of residual breast cancer burden to predict survival after neoadjuvant chemotherapy. J Clin Oncol 2007;25:4414-22. [Crossref] [PubMed]
- Săftoiu A, Gilja OH, Sidhu PS, Dietrich CF, Cantisani V, Amy D, et al. The EFSUMB Guidelines and Recommendations for the Clinical Practice of Elastography in Non-Hepatic Applications: Update 2018. Ultraschall Med 2019;40:425-53. [Crossref] [PubMed]
- Maier AM, Heil J, Harcos A, Sinn HP, Rauch G, Uhlmann L, Gomez C, Stieber A, Funk A, Barr RG, Hennigs A, Riedel F, Schäfgen B, Hug S, Marmé F, Sohn C, Golatta M. Prediction of pathological complete response in breast cancer patients during neoadjuvant chemotherapy: Is shear wave elastography a useful tool in clinical routine? Eur J Radiol 2020;128:109025. [Crossref] [PubMed]
- Bae JS, Chang JM, Lee SH, Shin SU, Moon WK. Prediction of invasive breast cancer using shear-wave elastography in patients with biopsy-confirmed ductal carcinoma in situ. Eur Radiol 2017;27:7-15. [Crossref] [PubMed]
- Evans A, Whelehan P, Thomson K, McLean D, Brauer K, Purdie C, Baker L, Jordan L, Rauchhaus P, Thompson A. Invasive breast cancer: relationship between shear-wave elastographic findings and histologic prognostic factors. Radiology 2012;263:673-7. [Crossref] [PubMed]
- Zhu Y, Jia Y, Pang W, Duan Y, Chen K, Nie F. Ultrasound contrast-enhanced patterns of sentinel lymph nodes: predictive value for nodal status and metastatic burden in early breast cancer. Quant Imaging Med Surg 2023;13:160-70. [Crossref] [PubMed]
- Ishihara S, Haga H. Matrix Stiffness Contributes to Cancer Progression by Regulating Transcription Factors. Cancers (Basel) 2022.
- Ondeck MG, Kumar A, Placone JK, Plunkett CM, Matte BF, Wong KC, Fattet L, Yang J, Engler AJ. Dynamically stiffened matrix promotes malignant transformation of mammary epithelial cells via collective mechanical signaling. Proc Natl Acad Sci U S A 2019;116:3502-7. [Crossref] [PubMed]
- Kim Y, Kim SH, Song BJ, Kang BJ, Yim KI, Lee A, Nam Y. Early Prediction of Response to Neoadjuvant Chemotherapy Using Dynamic Contrast-Enhanced MRI and Ultrasound in Breast Cancer. Korean J Radiol 2018;19:682-91. [Crossref] [PubMed]
- Candelaria RP, Bassett RL, Symmans WF, Ramineni M, Moulder SL, Kuerer HM, Thompson AM, Yang WT. Performance of Mid-Treatment Breast Ultrasound and Axillary Ultrasound in Predicting Response to Neoadjuvant Chemotherapy by Breast Cancer Subtype. Oncologist 2017;22:394-401. [Crossref] [PubMed]
- Evans A, Armstrong S, Whelehan P, Thomson K, Rauchhaus P, Purdie C, Jordan L, Jones L, Thompson A, Vinnicombe S. Can shear-wave elastography predict response to neoadjuvant chemotherapy in women with invasive breast cancer? Br J Cancer 2013;109:2798-802. [Crossref] [PubMed]
- Comstock CE, Mercado CL. Radiologic Clinics of North America. Breast imaging. Preface. Radiol Clin North Am 2014;52:xi. [Crossref] [PubMed]
- Duan Y, Zhu Y, Nie F, Guan L, Jia Y, Chen K, Wang W. Predictive value of combining clinicopathological, multimodal ultrasonic characteristics in axillary lymph nodal metastasis burden of patients with cT1-2N0 breast cancer. Clin Hemorheol Microcirc 2022;81:255-69. [Crossref] [PubMed]
- Xu P, Wu M, Yang M, Xiao J, Ruan ZM, Wu LY. Evaluation of internal and shell stiffness in the differential diagnosis of breast non-mass lesions by shear wave elastography. World J Clin Cases 2020;8:2510-9. [Crossref] [PubMed]
- Zhou J, Zhan W, Chang C, Zhang X, Jia Y, Dong Y, Zhou C, Sun J, Grant EG. Breast lesions: evaluation with shear wave elastography, with special emphasis on the "stiff rim" sign. Radiology 2014;272:63-72. [Crossref] [PubMed]
- Gradishar WJ, Anderson BO, Balassanian R, Blair SL, Burstein HJ, Cyr A, et al. NCCN Guidelines Insights: Breast Cancer, Version 1.2017. J Natl Compr Canc Netw 2017;15:433-51. [Crossref] [PubMed]
- Li JB, Jiang ZF. Zhonghua yi xue za zhi 2021;101:1835-8. [Chinese Society of Clinical Oncology Breast Cancer Guideline version 2021: updates and interpretations]. [PubMed]
- Sejben A, Kószó R, Kahán Z, Cserni G, Zombori T. Examination of Tumor Regression Grading Systems in Breast Cancer Patients Who Received Neoadjuvant Therapy. Pathol Oncol Res 2020;26:2747-54. [Crossref] [PubMed]
- Chollet P, Abrial C, Durando X, Thivat E, Tacca O, Mouret-Reynier MA, Leheurteur M, Kwiatkowski F, Dauplat J, Penault-Llorca F. A new prognostic classification after primary chemotherapy for breast cancer: residual disease in breast and nodes (RDBN). Cancer J 2008;14:128-32. [Crossref] [PubMed]
- Choi M, Park YH, Ahn JS, Im YH, Nam SJ, Cho SY, Cho EY. Assessment of pathologic response and long-term outcome in locally advanced breast cancers after neoadjuvant chemotherapy: comparison of pathologic classification systems. Breast Cancer Res Treat 2016;160:475-89. [Crossref] [PubMed]
- Hayashi M, Yamamoto Y, Ibusuki M, Fujiwara S, Yamamoto S, Tomita S, Nakano M, Murakami K, Iyama K, Iwase H. Evaluation of tumor stiffness by elastography is predictive for pathologic complete response to neoadjuvant chemotherapy in patients with breast cancer. Ann Surg Oncol 2012;19:3042-9. [Crossref] [PubMed]
- Huang Y, Le J, Miao A, Zhi W, Wang F, Chen Y, Zhou S, Chang C. Prediction of treatment responses to neoadjuvant chemotherapy in breast cancer using contrast-enhanced ultrasound. Gland Surg 2021;10:1280-90. [Crossref] [PubMed]
- Wang B, Jiang T, Huang M, Wang J, Chu Y, Zhong L, Zheng S. Evaluation of the response of breast cancer patients to neoadjuvant chemotherapy by combined contrast-enhanced ultrasonography and ultrasound elastography. Exp Ther Med 2019;17:3655-63. [Crossref] [PubMed]
- Evans A, Whelehan P, Thompson A, Purdie C, Jordan L, Macaskill J, Henderson S, Vinnicombe S. Identification of pathological complete response after neoadjuvant chemotherapy for breast cancer: comparison of greyscale ultrasound, shear wave elastography, and MRI. Clin Radiol 2018;73:910.e1-6. [Crossref] [PubMed]
- Choi WJ, Kim HH, Cha JH, Shin HJ, Chae EY. Comparison of Pathologic Response Evaluation Systems After Neoadjuvant Chemotherapy in Breast Cancers: Correlation With Computer-Aided Diagnosis of MRI Features. AJR Am J Roentgenol 2019;213:944-52. [Crossref] [PubMed]
- Xu YJ, Gong HL, Hu B, Hu B. Role of "Stiff Rim" sign obtained by shear wave elastography in diagnosis and guiding therapy of breast cancer. Int J Med Sci 2021;18:3615-23. [Crossref] [PubMed]
- Tang Y, Liang M, Tao L, Deng M, Li T. Machine learning-based diagnostic evaluation of shear-wave elastography in BI-RADS category 4 breast cancer screening: a multicenter, retrospective study. Quant Imaging Med Surg 2022;12:1223-34. [Crossref] [PubMed]
- Pratt SJP, Lee RM, Martin SS. The Mechanical Microenvironment in Breast Cancer. Cancers (Basel) 2020.
- Insana MF, Pellot-Barakat C, Sridhar M, Lindfors KK. Viscoelastic imaging of breast tumor microenvironment with ultrasound. J Mammary Gland Biol Neoplasia 2004;9:393-404. [Crossref] [PubMed]
- Chang JM, Park IA, Lee SH, Kim WH, Bae MS, Koo HR, Yi A, Kim SJ, Cho N, Moon WK. Stiffness of tumours measured by shear-wave elastography correlated with subtypes of breast cancer. Eur Radiol 2013;23:2450-8. [Crossref] [PubMed]
- Partridge SC, Zhang Z, Newitt DC, Gibbs JE, Chenevert TL, Rosen MA, Bolan PJ, Marques HS, Romanoff J, Cimino L, Joe BN, Umphrey HR, Ojeda-Fournier H, Dogan B, Oh K, Abe H, Drukteinis JS, Esserman LJ, Hylton NM. ACRIN 6698 Trial Team and I-SPY 2 Trial Investigators. Diffusion-weighted MRI Findings Predict Pathologic Response in Neoadjuvant Treatment of Breast Cancer: The ACRIN 6698 Multicenter Trial. Radiology 2018;289:618-27. [Crossref] [PubMed]
- Kim WH, Kim HJ, Park CS, Lee J, Park HY, Jung JH, Kim WW, Chae YS, Lee SJ, Kim SH. Axillary Nodal Burden Assessed with Pretreatment Breast MRI Is Associated with Failed Sentinel Lymph Node Identification after Neoadjuvant Chemotherapy for Breast Cancer. Radiology 2020;295:275-82. [Crossref] [PubMed]
- Bian T, Wu Z, Lin Q, Wang H, Ge Y, Duan S, Fu G, Cui C, Su X. Radiomic signatures derived from multiparametric MRI for the pretreatment prediction of response to neoadjuvant chemotherapy in breast cancer. Br J Radiol 2020;93:20200287. [Crossref] [PubMed]
- van la Parra RFD, Tadros AB, Checka CM, Rauch GM, Lucci A Jr, Smith BD, Krishnamurthy S, Valero V, Yang WT, Kuerer HM. Baseline factors predicting a response to neoadjuvant chemotherapy with implications for non-surgical management of triple-negative breast cancer. Br J Surg 2018;105:535-43. [Crossref] [PubMed]
- Boland MR, Ryan ÉJ, Nugent T, Gilroy D, Kelly ME, Kennedy J, Maguire A, Alazawi D, Boyle TJ, Connolly EM. Impact of progesterone receptor status on response to neoadjuvant chemotherapy in estrogen receptor-positive breast cancer patients. J Surg Oncol 2020;122:861-8. [Crossref] [PubMed]
- Chen X, He C, Han D, Zhou M, Wang Q, Tian J, Li L, Xu F, Zhou E, Yang K. The predictive value of Ki-67 before neoadjuvant chemotherapy for breast cancer: a systematic review and meta-analysis. Future Oncol 2017;13:843-57. [Crossref] [PubMed]
- Kim SY, Cho N, Choi Y, Lee SH, Ha SM, Kim ES, Chang JM, Moon WK. Factors Affecting Pathologic Complete Response Following Neoadjuvant Chemotherapy in Breast Cancer: Development and Validation of a Predictive Nomogram. Radiology 2021;299:290-300. [Crossref] [PubMed]
- Huang JX, Lin SY, Ou Y, Shi CG, Zhong Y, Wei MJ, Pei XQ. Combining conventional ultrasound and sonoelastography to predict axillary status after neoadjuvant chemotherapy for breast cancer. Eur Radiol 2022;32:5986-96. [Crossref] [PubMed]
- Kim R, Chang JM, Lee HB, Lee SH, Kim SY, Kim ES, Cho N, Moon WK. Predicting Axillary Response to Neoadjuvant Chemotherapy: Breast MRI and US in Patients with Node-Positive Breast Cancer. Radiology 2019;293:49-57. [Crossref] [PubMed]
- Tadros AB, Yang WT, Krishnamurthy S, Rauch GM, Smith BD, Valero V, Black DM, Lucci A Jr, Caudle AS, DeSnyder SM, Teshome M, Barcenas CH, Miggins M, Adrada BE, Moseley T, Hwang RF, Hunt KK, Kuerer HM. Identification of Patients With Documented Pathologic Complete Response in the Breast After Neoadjuvant Chemotherapy for Omission of Axillary Surgery. JAMA Surg 2017;152:665-70. [Crossref] [PubMed]