Deep learning-assisted knee osteoarthritis automatic grading on plain radiographs: the value of multiview X-ray images and prior knowledge
Introduction
Knee osteoarthritis (OA) is one of the most common types of degenerative OA in the world, with a significant incidence in middle-aged and older adult individuals (1). Knee OA is characterized by articular cartilage degeneration, joint inflammation, and secondary bone hyperplasia (2). Knee OA has a range of clinical manifestations, including knee joint discomfort, tenderness, stiffness with a functional disorder, and even disability (3), which have a considerably negative impact on individuals, families, and society. In China, the overall prevalence of knee OA is about 13.8%, and the incidence is significantly higher in people over 40 years of age (4). The intensification of global aging requires that greater attention be paid to the occurrence and development of knee OA (5). Currently, the diagnosis of this disease is mainly based on the judgment of clinicians and radiologists, which is highly subjective and cannot be quantified. Subtle changes are challenging to observe in plain radiographs of the knee, resulting in the loss of crucial information which might indicate the earliest knee OA progression.
X-ray imaging is a safe, cost-effective, and widely available examination method, in which the gold standard for evaluating the degree of knee OA degeneration—the Kellgren-Lawrence (K-L) grading system—is made (6). Despite these advantages, ordinary radiography is notoriously insensitive when trying to detect early changes in OA. Making an early diagnosis of knee OA using an X-ray is difficult for a number of reasons. First, the best site for viewing early signs of OA and its progression is articular cartilage tissue that cannot be directly seen on plain radiographs. Second, the imaging modality uses only 2-dimensional (2D) projections, and much critical information is obscured. Finally, an experienced practitioner is needed to interpret the final image (7).
The development of deep learning (DL) has made it possible to establish accurate and rapid diagnostic methods (8). DL can allow the learning of features directly from the data, and it has recently revolutionized the field of medical image analysis by surpassing the conventional computer vision techniques that require the manual engineering of data representation methods (9). DL can undertake tasks such as image alignment, image recognition, image detection, image segmentation, and image classification and prediction, among others (10-16). These DL models include the well-known U-Net network and Residual Net (ResNet) network (9,17). In terms of medical image processing, the DL model can automatically extract the features of X-ray images through the learning of neural networks and classify the images to assist clinicians in diagnosis and treatment. Recently, DL has been extensively used in the analysis of imaging data from a variety of diseases (18), such as pulmonary nodules, degenerative OA, tumors, and nervous system diseases (19-25). In the field of OA research, several studies have shown that the use of X-ray images and magnetic resonance imaging (MRI) data can evaluate the progression of knee OA well by establishing DL models which can not only classify and grade knee OA but predict its future development to assist clinical diagnosis and treatment (10,26-28). These studies have improved the diagnostic accuracy of knee OA using medical images, but there is still room for improvement in the DL methods for knee X-rays. The information of the relevant anatomy and typical pathologies typically visualized on the lateral radiograph of the knee can also provide better information concerning the disease process as compared to the use of anteroposterior images (29). Unfortunately, there are few studies on lateral knee radiographs. Minciullo et al. (30) presented a fully automated method based on a random forest regression voting constrained local model (RFCLM) to interpret knee OA radiographs, which showed that automated analysis of the lateral view achieves classification performance comparable if not better than that of similar techniques applied to the frontal view. Therefore, the information contained in lateral knee radiographs should not be discarded. Furthermore, the use of prior knowledge has been shown to be useful in improving DL model performance in knee segmentation (31). However, the application of prior knowledge on X-ray images is relatively uncommon. It is necessary to study the effect of prior zonal knowledge on the performance of DL models in grading knee OA.
In this study, 4 groups of DL models were established according to whether they adopted multiview images and automatic zonal segmentation as the DL prior knowledge. We assessed and compared the performance of 4 DL models of knee OA grading. The effects of multiview images and zonal segmentation as prior knowledge in the diagnostic capabilities of DL models were explored. We present this article in accordance with the STARD reporting checklist (available at https://qims.amegroups.com/article/view/10.21037/qims-22-1250/rc).
Methods
Image data set
The study was conducted in accordance with the Declaration of Helsinki (as revised in 2013). The study was approved by the institutional review board of the Fifth Affiliated Hospital of Sun Yat-sen University (No. K163-1), and individual consent for this retrospective analysis was waived. The data set of this research was retrospectively collected in the Fifth Affiliated Hospital of Sun Yat-sen University (Zhuhai, China) and included patients who underwent knee radiography with both anteroposterior and lateral images between July 2017 and July 2020. The inclusion criteria were as follows: (I) adults over 18 years old with closed epiphysis, and (II) those with both anteroposterior and lateral images available for each examination. The exclusion criteria were as follows: (I) tumors that destroyed the bone in the knee; (II) other inflammatory arthritis; (III) fractures occurring in the knee area, including the lower femur, upper tibia, patella, and fibular head; (IV) congenital deformity of knee joint development and other bone and joint diseases; and (V) insufficient quality of the X-ray images. A total of 1,846 patients were included. For these patients, we used a single knee joint as a sample because the severity of the left and right knees may be inconsistent in the same patient. Furthermore, some patients had more than one X-ray scan, and the knee OA grade may not be the same in 2 or more scans. Data were randomly grouped at a ratio of 8:2 for training and testing. The intensity of images was normalized before the analysis. The size of the Digital Imaging and Communications in Medicine (DICOM) images was not fixed and ranged from approximately 1,726×2,158 to 1,942×2,431 pixels.
Definition
During model training, the original DICOM format X-ray images needed to be processed and converted into a common JPG format for subsequent processing. Due to the differences in patient volume and radiographic machines, the size of the images was inconsistent, so we adjusted the size of the images and resized them into a uniform size of 512×512 pixels. The knee joint regions were labeled (Figure 1) with rectangular boxes by 2 radiologists (JF and JL), who had 3 and 6 years of diagnostic experience, respectively, using an image labeling tool developed by Radcloud software (v.1.2, Huiying Medical Technology Co., Ltd.), which provided segmentation labels for the subsequent segmentation model. In addition, all the plain radiographs were assessed by 2 musculoskeletal radiologists (WL and YF) with 4 and 8 years of diagnostic experience, respectively, and were divided into 5 levels according to the K-L grading scheme (6). Each grade was labeled as class 0, class 1, class 2, class 3, or class 4, according to the increasing severity of OA, with class 0 signifying no presence of OA and class 4 signifying severe OA. These labels were used as the classification labels for the subsequent classification model. Due to the class imbalance caused by the limitation of the data, we performed data augmentation on minority images, such as random rotation, to increase the data in the training cohort. Images were rotated 45°, 90°, 135°, and 180° so that the number of images in each category did not differ significantly.
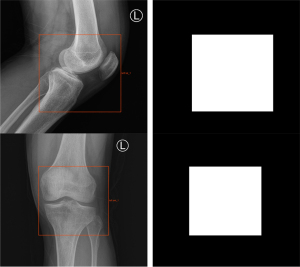
DL framework
The proposed DL framework is illustrated in Figure 2. In this paper, we did not cut out the knee joint region or send it into the network separately for classification. Instead, we fused the prior knowledge of the location of the knee region with the original image and sent this information into the classification network. We developed a 2-stage cascaded framework to determine the severity of knee arthritis: (I) U-Net architecture (32) focused on extracting the knee joint region from the original X-ray images and provided zonal prior knowledge; (II) zonal prior knowledge was then incorporated into the ResNet-50 (33) for the classification of severity in knee OA. Since the X-ray images came from different devices, they needed to be preprocessed before being sent to the network. Preprocessing included the normalization of the original image using the max-min method and contrast enhancement to adjust the Hounsfield unit of the X-ray images to highlight the target area. The preprocessed images were then fed into the U-Net network for extraction of the knee joint region.
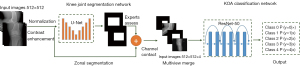
U-Net was used as the first biomedical image segmentation network. The network structure is shown in Figure 3A. The network structure of the U-Net model consisted of an encoder, decoder, and skip connection. The encoder performed convolution, batch normalization (BN), activation, and maximum pooling operations in sequence to implement feature extraction. Symmetrically, the decoding path used 4 upsampling operations to restore the feature maps to the original input size and interpreted the extracted features from the encoding path to restore the segmentation results. The U-Net used skip connections to strengthen the learning of the features because the encoder feature information was transferred to the decoder, which showed comparable performance in medical image segmentation. The training data process had 3 steps. First, multiview images were fed into U-Net, and the knee joint regions labeled with rectangular boxes provided segmentation labels for the segmentation model. Second, a U-Net segmentation network was used to perform image segmentation training on the labeled knee joint regions in the training data, and the trained U-Net for automatic identification of knee joint regions was obtained. Third, to verify the performance of the U-Net segmentation model, the testing data were used for segmentation evaluation. In addition, 2 radiologists (JF and JL) with 3 and 6 years of diagnostic experience, respectively, were invited to conduct a double-blind review of the segmentation results and to modify the initial findings to obtain the final segmentation results.
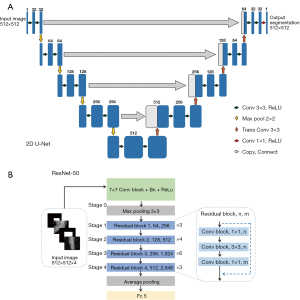
In the second step, we used zonal segmentation as extra channels to incorporate zonal prior knowledge into the classification model. We combined X-ray images of different views and zonal prior knowledge with early fusion. Specifically, we used X-ray images from 2 angles of view to generate 2D images of 2 channels, and zonal segmentation provided the other 2D images of 2 channels. Finally, they ended up with an input region of interest (ROI) size of 512×512×4 and were fed into the ResNet-50 network. ResNet-50 networks (named for their 50 network layers with learnable parameters) are widely used in the field of target classification, often as part of the classical neural network of the computer vision task backbone. ResNet-50 used the pretrained parameters on the ImageNet data set. Then, for weight transfer, the pretrained convolutional neural network (CNN) structure was further fine-tuned with our own data set, which guided the network to learn the general shallow information quickly. In this work, ResNet-50 was used for the classification of the severity of arthritis in the knee. The network structure is shown in Figure 3B. A residual network was designed to directly introduce the data output of one layer of the earlier layer to the input part of the later data layer, implying that the content of the later feature layer was partially and linearly contributed to by the previous layer. This residual learning strategy achieved state-of-the-art performances on established benchmark data sets for medical image classification tasks. Next, several convolutional layers followed by BN and rectified linear unit (ReLU) as the activation functions were used. Multiple convolutions and pooling operations were performed. The final feature vector was input to a fully connected layer of 5 nodes and a SoftMax function, yielding the probability of prediction. For selecting the output node, the node with the maximum probability could be selected as the prediction target.
Experiments
To verify the effectiveness of the proposed method, we conducted comparative experiments to assess the effect of multiview images and zonal prior knowledge on the classification of knee OA. For the model without prior knowledge, we used ResNet-50 to classify X-ray images directly. Model 1 used ResNet-50 to directly classify the K-L grading of anteroposterior knee joint images, with 512×512 as the input size. Model 2 used ResNet-50 to directly classify the K-L grading of multiview X-ray images (including anteroposterior and lateral knee joint images), with 512×512×2 as the input size. Models 1 and 2 belonged to the category of weak classification, in which the sample category is known but the lesion area is not. To demonstrate the inference process of the DL model in a visual manner, we used the gradient-weighted class activation map (Grad-CAM) technique (33) to extract features from the final convolutional layer of ResNet-50, which generated a weighted activation map for each image for displaying the areas of focus of the model.
To investigate the effect of knee zonal information as prior knowledge, we used the U-Net network to obtain zonal segmentation as extra channels for the input of the ResNet-50 model to incorporate zonal prior knowledge into the model. Model 3 provided additional zonal prior knowledge for the anteroposterior knee partition based on model 1, which combined the original images with zonal segmentation to produce a 2-channel 2D image with an input size of 512×512×2. Model 4 was built on models 2 and 3 and incorporated both anteroposterior knee images and a priori partitioning knowledge into the analysis, producing a 4-channel 2D image with an input size of 512×512×4. Models 3 and 4 used prior knowledge and focused on the knee joint region, so we did not use the Grad-CAM technique. Furthermore, to validate the effectiveness of models 3 and 4, we implemented the t-distributed stochastic neighbor embedding (t-SNE) visualization (34) of the model on all data. T-SNE is a dimensionality reduction technique that maps high-dimensional data to 2 or more dimensions suitable for human observation.
Finally, we compared the radiologist-DL diagnosis capability based on the testing cohort by evaluating the accuracy between the experienced radiologist and the best-performing DL model. We enlisted 1 radiologist (WY) with 4 years of musculoskeletal diagnostic experience who was not involved in annotating the training cohort.
Training process optimization
There were 2 steps included in the entire process: forward computation and backward propagation. In the training stage, anteroposterior and lateral knee joint images and zonal segmentation were fed into the network to update model parameters by backward propagation. The outputs of the network were used as the classification results, and the cross-entropy of the outputs and the labels were calculated as the loss function. We used a fixed learning rate of 0.0001 and applied the Adam optimizer to update the model parameters with a batch size of 64. All data were run in Python version 3.6.12. To reduce the likelihood of overfitting, strategies included L2 regularization (with a weight decay of 0.0005) and early stopping. The PyTorch framework was used to train and test the model on 2 NVIDIA RTX 1080 Ti graphics processing units for up to 1,500 epochs, and the model with the lowest validation loss was selected.
Statistical analysis
All statistical analyses were performed using SPSS 25.0 (IBM Corp.) and R software version 4.1.2 (The R Foundation of Statistical Computing). A P value <0.05 was considered statistically significant. The performance of the 4 DL models was evaluated by precision, recall, F1 score, and accuracy. Receiver operating characteristic (ROC) analysis was used to further evaluate the diagnostic performance of the knee OA lesion detection system. For the ROC analysis, the area under the ROC curve (AUC) was calculated for the discrimination performance of established models. The AUC obtained was compared between models using the DeLong nonparametric approach. The McNemar test was used to compare the accuracy of the DL model with that of experienced radiologists.
Results
Characteristics of images
A total of 4,200 knee joint images (including 4,200 anteroposterior images and 4,200 lateral images paired) from 1,846 patients (864 males and 982 females) were available for analysis. Each sample contained paired anteroposterior and lateral images. The ages of patients ranged from 18 to 92 years (mean 51.13±15.11 years). K-L grades of all data were as follows: grade 0, 1994 anteroposterior and lateral images; grade 1, 1063 images; grade 2, 630 images; grade 3, 360 images; and grade 4, 153 images. We randomly selected 80% of each grade to form the training cohort (n=3,359), and the remaining data were used for the testing cohort (n=841). The distribution of the knee X-ray images conditioned on the K-L grading scheme is shown in Table 1.
Table 1
Cohort | K-L 0 | K-L 1 | K-L 2 | K-L 3 | K-L 4 |
---|---|---|---|---|---|
All data (n=4,200) | 1,994 | 1,063 | 630 | 360 | 153 |
Training cohort (n=3,359) | 1,595 | 850 | 504 | 288 | 122 |
Testing cohort (n=841) | 399 | 213 | 126 | 72 | 31 |
Percentage (%) | 47 | 25 | 15 | 9 | 4 |
The data are the number of knees used in each cohort. The ratio between the training and testing cohort is 8 to 2. K-L, Kellgren-Lawrence.
Multiview images-based results
The overall performance of the different models based on the training cohort is summarized in Table 2. Compared to models 1 and 3, models 2 and 4 with lateral images combined achieved an overall accuracy of 0.92 and 0.96, respectively (Table 3). As shown in Table 4 and Figure 4, models 2 and 4 with lateral images combined achieved a higher microaverage AUC and macroaverage AUC of 0.92/0.91 and 0.96/0.95, respectively, compared to models 1 and 3 without lateral images combined in the testing cohort (the microaverage is concerned with the study of individual classes and the macroaverage is concerned with aggregations or totals). With or without the addition of prior knowledge, models 2 and 4 trained with lateral images combined performed better than did the models trained using only anteroposterior images. By comparing the AUC at each grade for the 4 models with and without lateral images, we observed an increased performance of the lateral images with AUCs of 0.94, 0.91, 0.89, 0.90, and 0.92 for model 2 and 0.99, 0.96, 0.92, 0.92 and 0.99 for model 4 in the testing cohort from knee OA class 0 to class 4, respectively. This finding suggests that the DL models based on anteroposterior and lateral knee joint images had a better diagnostic performance.
Table 2
Model | Multiview | Prior knowledge | Precision (m/M) | Recall (m/M) | F1 score (m/M) | AUC (95% CI) (m/M) | Accuracy |
---|---|---|---|---|---|---|---|
Model 1 | 0.88/0.84 | 0.88/0.85 | 0.88/0.84 | 0.89 (0.86–0.92)/0.88 (0.85–0.91) | 0.88 | ||
Model 2 | √ | 0.93/0.92 | 0.93/0.92 | 0.93/0.92 | 0.93 (0.90–0.96)/0.92 (0.89–0.95) | 0.93 | |
Model 3 | √ | 0.96/0.95 | 0.96/0.95 | 0.96/0.95 | 0.96 (0.93–0.99)/0.95 (0.92–0.98) | 0.96 | |
Model 4 | √ | √ | 0.97/0.96 | 0.97/0.97 | 0.97/0.96 | 0.97 (0.96–0.98)/0.96 (0.95–0.97) | 0.97 |
m, microaverage; M, macroaverage; AUC, area under the curve; CI, confidence interval.
Table 3
Class | Model 1 | Model 2 | Model 3 | Model 4 | |||||||||||||||
---|---|---|---|---|---|---|---|---|---|---|---|---|---|---|---|---|---|---|---|
Precision | Recall | F1 score | Accuracy | Precision | Recall | F1 score | Accuracy | Precision | Recall | F1 score | Accuracy | Precision | Recall | F1 score | Accuracy | ||||
Class 0 | 0.94 | 0.95 | 0.95 | 0.95 | 0.95 | 0.97 | 0.96 | 0.97 | 0.97 | 0.98 | 0.98 | 0.98 | 0.98 | 0.99 | 0.99 | 0.99 | |||
Class 1 | 0.87 | 0.75 | 0.81 | 0.75 | 0.93 | 0.83 | 0.88 | 0.83 | 0.95 | 0.88 | 0.91 | 0.88 | 0.97 | 0.91 | 0.94 | 0.91 | |||
Class 2 | 0.72 | 0.85 | 0.78 | 0.85 | 0.81 | 0.91 | 0.86 | 0.91 | 0.86 | 0.94 | 0.89 | 0.94 | 0.89 | 0.94 | 0.92 | 0.94 | |||
Class 3 | 0.73 | 0.76 | 0.75 | 0.76 | 0.86 | 0.86 | 0.86 | 0.86 | 0.89 | 0.90 | 0.90 | 0.90 | 0.91 | 0.93 | 0.92 | 0.93 | |||
Class 4 | 0.87 | 0.84 | 0.85 | 0.84 | 0.93 | 0.90 | 0.92 | 0.90 | 0.97 | 0.90 | 0.93 | 0.90 | 0.97 | 0.94 | 0.95 | 0.94 | |||
Macro-avg | 0.83 | 0.83 | 0.83 | 0.90 | 0.90 | 0.90 | 0.93 | 0.92 | 0.92 | 0.94 | 0.94 | 0.94 | |||||||
Weighted-avg | 0.87 | 0.87 | 0.87 | 0.92 | 0.94 | 0.92 | 0.94 | 0.94 | 0.94 | 0.96 | 0.96 | 0.96 | |||||||
Overall accuracy | 0.87 | 0.92 | 0.94 | 0.96 |
Macro-avg, macroaverage; Weighted-avg, weighted average.
Table 4
Class | Model 1 | Model 2 | Model 3 | Model 4 |
---|---|---|---|---|
Class 0 (AUC) (95% CI) | 0.91 (0.88–0.94) | 0.94 (0.92–0.96) | 0.98 (0.97–0.99) | 0.99 (0.99–1.00) |
Class 1 (AUC) (95% CI) | 0.89 (0.86–0.92) | 0.91 (0.88–0.94) | 0.92 (0.88–0.96) | 0.96 (0.93–0.99) |
Class 2 (AUC) (95% CI) | 0.82 (0.79–0.85) | 0.89 (0.84–0.94) | 0.89 (0.85–0.93) | 0.92 (0.88–0.96) |
Class 3 (AUC) (95% CI) | 0.85 (0.81–0.89) | 0.90 (0.87–0.93) | 0.92 (0.87–0.97) | 0.92 (0.87–0.97) |
Class 4 (AUC) (95% CI) | 0.89 (0.83–0.95) | 0.92 (0.88–0.96) | 0.92 (0.88–0.96) | 0.99 (0.99–1.00) |
mAUC (95% CI) | 0.88 (0.84–0.92) | 0.92 (0.88–0.96) | 0.94 (0.92–0.96) | 0.96 (0.93–0.99) |
MAUC (95% CI) | 0.87 (0.83–0.91) | 0.91 (0.88–0.94) | 0.93 (0.90–0.96) | 0.95 (0.92–0.98) |
AUC, area under the curve; CI, confidence interval; m, microaverage; M, macroaverage.
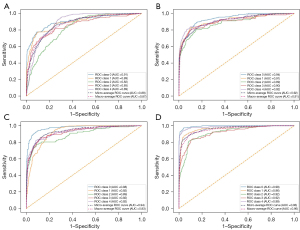
Prior knowledge-based results
The U-Net used an end-to-end prediction procedure. The input was the X-ray image, and the output was the segmentation mask. The Dice similarity coefficient (Dice) was selected as a segmentation evaluation metric. Then, we used the U-Net to test all samples. The Dice was above 0.98, and all results were reviewed by experienced radiologists. The results of knee region segmentation were good, so experts made no modifications. By comparing the overall accuracy for both experiments with and without zonal segmentation as prior knowledge, we observed an increased performance using prior zonal segmentation in the testing cohort (Table 3). In the same position level, the overall accuracy was significantly higher after adding the zonal segmentation as prior knowledge for model 3 (overall accuracy 0.94) and model 4 (overall accuracy 0.96). On the anteroposterior view, addition of a prior segmentation could improve the microaverage AUC and macroaverage AUC to 0.94 and 0.93, respectively, and when the lateral images were added, the microaverage and macroaverage AUCs were as high as 0.96 and 0.95, respectively (Figure 4C,4D, respectively). DL models trained using prior zonal of interest performed better than those without this addition.
The multiview matrices of the 4 models were plotted to determine whether the devised modules could intuitively improve the classification (Figure 5). Model 4 achieved the best diagnostic performance, with accuracy values of 0.99, 0.91, 0.94, 0.93, and 0.94 for knee OA grades 0 to 4, respectively. The probability that the model predicted that the sample was the real label was statistically summarized, and then a pairwise comparison between the models was performed. The final DeLong test results are shown in Table 5.
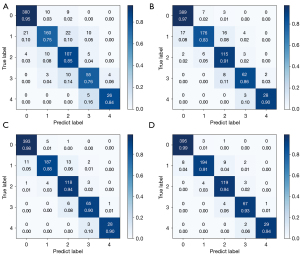
Table 5
Model | P value |
---|---|
Model 1 vs. model 2 | 0.242 |
Model 3 vs. model 4 | 0.436 |
Model 1 vs. model 3 | 0.143 |
Model 2 vs. model 4 | 0.211 |
The multicategory DeLong test was used to compare the microaverage AUC and macroaverage AUC of the different models; additionally, statistical analysis was used to summarize the probability that the model could predict that the sample was the real label, after which a pairwise comparison of the 4 models was performed. AUC, area under the curve.
Visual analysis of model performance
The Grad-CAM tool can identify the regions that the network considers important. Figure 6 illustrates an example of the different treatments of the knee X-ray images for the 5 K-L grades in models 1 and 2. These class activation maps were used to describe each model’s receptive field in the case of given classes, with the results being shown Figure 6, where the blue represents low attention and red represents high attention. The CAM activation region was more obvious in the knee area, which was reasonable. However, for some images, the study found that the CAM activation region also appeared in other tissues outside the knee joint with models 1 and 2, which may indicate that some extra-articular tissues were also important for diagnosing knee OA.
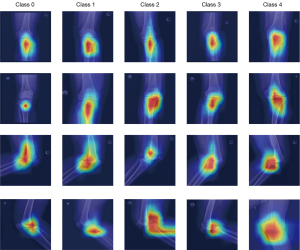
We visualized models 3 and 4 according to the features learned from the last feature extraction layer of the network and performed t-SNE diagrams for all images from classes 0 to 4 based on our model, as displayed in Figure 7. The relatively clear boundaries in Figure 7 indicate that images belonging to the same grade were clustered together, and the different grades of knee OA were well separated.
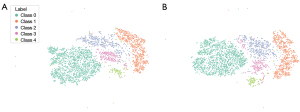
Comparison of radiologist and DL diagnosis capability
In the testing cohort, the performance of model 4 was better than that of an experienced radiologist, with accuracies of 0.96 and 0.86 (McNemar test, P<0.05), respectively. The final knee OA diagnostic DL model established in this study performed better than did the experienced radiologists.
Discussion
This work is the first attempt to assess the effect of multiview images and prior knowledge on the diagnostic performance of knee OA classification. The DL model with multiview images and prior knowledge obtained the best classification performance among the 4 DL models in the testing cohort, with a microaverage AUC and macroaverage AUC of 0.96 and 0.95, respectively. An accurate reading of knee X-ray images is crucial but strongly depends on expertise, which is closely related to the experience level of the radiologist. Many studies have demonstrated that DL models have better diagnostic precision than do radiologists (35). This finding is consistent with the results of our study, which found that the overall accuracy of the DL model with multiview images and prior knowledge was better compared to that of an experienced radiologist.
In this study, we developed a DL-based method to perform automatic K-L grading from knee radiographs. In early studies, such as those by Oka et al. (36,37), knee osteoarthritis computer-aided diagnosis (KOACAD) was conducted to measure joint space narrowing at medial and lateral sides, osteophyte formation, and joint angulation. Although KOACAD was shown to be useful for objective, accurate, and easy evaluation of the radiographic knee OA severity compared with these conventional categorical grading systems, these studies had limitations of few quantitative indicators and relatively small test set sizes. In a later study, Hirvasniemi et al. (38) used advanced image analysis methods to quantify differences in bone texture between participants with different stages of knee OA, but their sample size was only 203 knee joints.
In recent years, there has been an increasing amount of big data analysis based on public data sets, such as those performed by the Osteoarthritis Initiative (OAI) and Multicenter Osteoarthritis Study (MOST), and relatively good results have been achieved. Suresha et al. (39) collected 7,549 knee radiographs and used a DL model to automatically grade the severity of knee OA. The object-classification network predicted K-L scores of 4 with 87% accuracy, but the accuracy of the K-L scores of 1 was only 23%. Although our study did not have as much data, the results showed that the 4 models we established also had an accuracy of 0.87 to 0.96. These results may be related to our accurate annotation and use of segmented images as prior knowledge. Swiecicki et al. (40) used a data set of 9,739 exams from 2,802 patients from MOST and obtained a multiclass accuracy of 71.90% on the entire test set using a multi-input CNN architecture. The lowest accuracy in our study was 0.87, but this only occurred when anteroposterior images were used. In addition, many researchers found using the ResNet network framework for grading knee OA to be highly effective, with the overall AUC value reaching more than 90% and the highest accuracy rate reaching 98.90% (41-43). These results are in good agreement with our experimental results, as we obtained an overall accuracy of 0.94 using the ResNet-50 network on the anteroposterior images. Posteroanterior images in knee OA studies are recommended by OAI and MOST, but this does not mean lateral images are useless. Lateral radiographs were included in our study because the DL model was believed to reveal some features that we did not observe, and these features may be helpful for the judgment of knee OA. Our final results also confirmed that the addition of lateral images improves the diagnostic performance of the DL model.
DL models require the collection of a sufficient amount of data, but the effect of different body positions of plain radiographs should be taken into account (18,29). Lateral radiographs have been proven to be useful for the detection of knee OA (30). To compare the effect of different body positions on the study results, our study included a large number of samples, and each image included anteroposterior and lateral radiographs of the knee joint. In the first part of the study, our results showed a significant effect on the performance when the lateral images were added to the training cohort (micro-average AUCs: 0.88 of model 1 to 0.92 of model 2 and 0.94 of model 3 to 0.96 of model 4; macro-average AUCs: 0.87 of model 1 to 0.91 of model 2 and 0.93 of model 3 to 0.95 of model 4). The overall accuracy increased from 0.87 of model 1 to 0.92 of model 2 and 0.94 of model 3 to 0.96 model 4, respectively, when lateral images were added. The results showed that the addition of lateral images could improve the performance of the model. To the best of our knowledge, this may be the first study to report a DL study on knee OA using a combination of anteroposterior and lateral radiographs. The performance of this model cannot be directly compared with previous models, as other studies almost always used anteroposterior images.
To prove that narrowing the detection range of the model also improved the detection efficacy of the DL model, we designed the following experimental steps to examine 2 crucial aspects: (I) we used ResNet 50 to directly identify and classify the K-L grading of knee joint images, and (II) we used a rectangular zonal segmentation as prior knowledge and then U-Net for detection and classification. This part of the study showed that adding zonal segmentation as prior knowledge improved the performance of DL models significantly. The AUC value increased from 0.87 to 0.94 and 0.92 to 0.96 for detection and classification, respectively, suggesting that prenarrowing the detection range of lesions can significantly improve the performance of the DL model in these areas. Manual segmentation of all images is generally used in radiomics studies, especially in MRI scans (44). The segmentation of prior knowledge regions we applied to knee OA DL models was influenced by the work of Hosseinzadeh et al. and Xie et al. (19,45), who concluded that prior zonal knowledge significantly affected the performance of DL models. Finally, we proved that the DL model established in this study performed better than did experienced radiologists, which indicates that the model could be a tool to help clinicians make accurate diagnoses and treatment decisions.
In summary, our research has several advantages. First, we used both anteroposterior and lateral radiographs and conducted a comparative study, providing more information and details of knee OA. We also narrowed the range of ROI by prior segmentation and improved the detection efficacy.
Our study had some limitations that should be considered. First, this was a retrospective study, and all data came from a single hospital. Although we used normalization methods to compensate for scanner and scanner setting variations, we cannot generalize our conclusions to all X-ray images from other centers. Extending the training to include more data from other providers is necessary to make generalizations. To create a more comprehensive DL model in the future, we intend to increase our data set by gathering multicenter data. Second, there was a difference in the number of images collected in each K-L grading. Since this difference in the number of grades was determined by the incidence of the disease itself, this difference was difficult to resolve. Third, in this study, some patients had more than 1 knee included, so part of the correlated (not independent) data were used in the DL algorithm analyses, which might have resulted in skewed results in the statistical analyses. Finally, our study only used X-ray data, and we did not have access to clinical data, laboratory test information, or follow-up data. The use of more information will lead to additional improvements to our model, such as a greater predictive ability (46).
To conclude, this study evaluated 4 DL models which could detect and classify knee OA on plain radiographs of the knee. Our study demonstrated that the performance of the DL model for the detection and classification of knee OA can be improved by using prior segmentation on plain radiographs and additional body positions including lateral knee images. These results can support further research to improve the use of DL algorithms as a noninvasive predictive method in the diagnosis and classification of knee OA. The DL grading model can help clinicians make a preliminary diagnosis and, to a certain extent, assist them in making treatment decisions.
Acknowledgments
Funding: This study received financial support from the National Natural Science Foundation of China (grant No. 82172053).
Footnote
Reporting Checklist: The authors have completed the STARD reporting checklist. Available at https://qims.amegroups.com/article/view/10.21037/qims-22-1250/rc
Conflicts of Interest: All authors have completed the ICMJE uniform disclosure form (available at https://qims.amegroups.com/article/view/10.21037/qims-22-1250/coif). BQ is an employee of Huiying Medical Technology Co. The other authors have no conflicts of interest to declare.
Ethical Statement: The authors are accountable for all aspects of the work in ensuring that questions related to the accuracy or integrity of any part of the work are appropriately investigated and resolved. The study was conducted in accordance with the Declaration of Helsinki (as revised in 2013). The study was approved by the institutional review board of the Fifth Affiliated Hospital of Sun Yat-sen University (No. K163-1), and individual consent for this retrospective analysis was waived.
Open Access Statement: This is an Open Access article distributed in accordance with the Creative Commons Attribution-NonCommercial-NoDerivs 4.0 International License (CC BY-NC-ND 4.0), which permits the non-commercial replication and distribution of the article with the strict proviso that no changes or edits are made and the original work is properly cited (including links to both the formal publication through the relevant DOI and the license). See: https://creativecommons.org/licenses/by-nc-nd/4.0/.
References
- Lee LS, Chan PK, Fung WC, Chan VWK, Yan CH, Chiu KY. Imaging of knee osteoarthritis: A review of current evidence and clinical guidelines. Musculoskeletal Care 2021;19:363-74. [Crossref] [PubMed]
- Kraus VB, Karsdal MA. Osteoarthritis: Current Molecular Biomarkers and the Way Forward. Calcif Tissue Int 2021;109:329-38. [Crossref] [PubMed]
- Felson DT. Clinical practice. Osteoarthritis of the knee. N Engl J Med 2006;354:841-8. [Crossref] [PubMed]
- Zhang Z, Huang C, Jiang Q, Zheng Y, Liu Y, Liu S, et al. Guidelines for the diagnosis and treatment of osteoarthritis in China (2019 edition). Ann Transl Med 2020;8:1213. [Crossref] [PubMed]
- Jang S, Lee K, Ju JH. Recent Updates of Diagnosis, Pathophysiology, and Treatment on Osteoarthritis of the Knee. Int J Mol Sci 2021;22:2619. [Crossref] [PubMed]
- Kellgren JH, Lawrence JS. Radiological assessment of osteo-arthrosis. Ann Rheum Dis 1957;16:494-502. [Crossref] [PubMed]
- Altman R, Asch E, Bloch D, Bole G, Borenstein D, Brandt K, Christy W, Cooke TD, Greenwald R, Hochberg M. Development of criteria for the classification and reporting of osteoarthritis. Classification of osteoarthritis of the knee. Diagnostic and Therapeutic Criteria Committee of the American Rheumatism Association. Arthritis Rheum 1986;29:1039-49. [Crossref] [PubMed]
- Rueckert D, Glocker B, Kainz B. Learning clinically useful information from images: Past, present and future. Med Image Anal 2016;33:13-8. [Crossref] [PubMed]
- Karim MdR, Jiao J, Döhmen T, Cochez M, Beyan O, Rebholz-Schuhmann D, Decker S. DeepKneeExplainer: Explainable Knee Osteoarthritis Diagnosis From Radiographs and Magnetic Resonance Imaging. IEEE Access 2021;9:39757-80.
- Norman B, Pedoia V, Noworolski A, Link TM, Majumdar S. Applying Densely Connected Convolutional Neural Networks for Staging Osteoarthritis Severity from Plain Radiographs. J Digit Imaging 2019;32:471-7. [Crossref] [PubMed]
- Shelhamer E, Long J, Darrell T. Fully Convolutional Networks for Semantic Segmentation. IEEE Trans Pattern Anal Mach Intell 2017;39:640-51. [Crossref] [PubMed]
- Gupta D, Kim M, Vineberg KA, Balter JM. Generation of Synthetic CT Images From MRI for Treatment Planning and Patient Positioning Using a 3-Channel U-Net Trained on Sagittal Images. Front Oncol 2019;9:964. [Crossref] [PubMed]
- Liu Y, Zhang X, Cai G, Chen Y, Yun Z, Feng Q, Yang W. Automatic delineation of ribs and clavicles in chest radiographs using fully convolutional DenseNets. Comput Methods Programs Biomed 2019;180:105014. [Crossref] [PubMed]
- Guan B, Liu F, Haj-Mirzaian A, Demehri S, Samsonov A, Neogi T, Guermazi A, Kijowski R. Deep learning risk assessment models for predicting progression of radiographic medial joint space loss over a 48-MONTH follow-up period. Osteoarthritis Cartilage 2020;28:428-37. [Crossref] [PubMed]
- Schiratti JB, Dubois R, Herent P, Cahané D, Dachary J, Clozel T, Wainrib G, Keime-Guibert F, Lalande A, Pueyo M, Guillier R, Gabarroca C, Moingeon P. A deep learning method for predicting knee osteoarthritis radiographic progression from MRI. Arthritis Res Ther 2021;23:262. [Crossref] [PubMed]
- Zhao Y, Zhao T, Chen S, Zhang X, Serrano Sosa M, Liu J, Mo X, Chen X, Huang M, Li S, Zhang X, Huang C. Fully automated radiomic screening pipeline for osteoporosis and abnormal bone density with a deep learning-based segmentation using a short lumbar mDixon sequence. Quant Imaging Med Surg 2022;12:1198-213. [Crossref] [PubMed]
- Wu Z, Shen C, van den Hengel A. Wider or Deeper: Revisiting the ResNet Model for Visual Recognition. Pattern Recognition 2019;90:119-33. [Crossref]
- van Ooijen PMA, Nagaraj Y, Olthof A. Medical imaging informatics, more than 'just' deep learning. Eur Radiol 2020;30:5507-9. [Crossref] [PubMed]
- Hosseinzadeh M, Saha A, Brand P, Slootweg I, de Rooij M, Huisman H. Deep learning-assisted prostate cancer detection on bi-parametric MRI: minimum training data size requirements and effect of prior knowledge. Eur Radiol 2022;32:2224-34. [Crossref] [PubMed]
- Zhu Y, Ma J, Yuan C, Zhu X. Interpretable learning based Dynamic Graph Convolutional Networks for Alzheimer’s Disease analysis. Information Fusion 2022;77:53-61. [Crossref]
- Gu Y, Chi J, Liu J, Yang L, Zhang B, Yu D, Zhao Y, Lu X. A survey of computer-aided diagnosis of lung nodules from CT scans using deep learning. Comput Biol Med 2021;137:104806. [Crossref] [PubMed]
- Tiulpin A, Thevenot J, Rahtu E, Lehenkari P, Saarakkala S. Automatic Knee Osteoarthritis Diagnosis from Plain Radiographs: A Deep Learning-Based Approach. Sci Rep 2018;8:1727. [Crossref] [PubMed]
- Zhao X, Wu Y, Song G, Li Z, Zhang Y, Fan Y. A deep learning model integrating FCNNs and CRFs for brain tumor segmentation. Med Image Anal 2018;43:98-111. [Crossref] [PubMed]
- Lyu B, Haque A. Deep Learning Based Tumor Type Classification Using Gene Expression Data. In: Proceedings of the 2018 ACM International Conference on Bioinformatics, Computational Biology, and Health Informatics. New York, NY, USA: Association for Computing Machinery, 2018:89-96.
- Wu QY, Liu SL, Sun P, Li Y, Liu GW, Liu SS, Hu JL, Niu TY, Lu Y. Establishment and clinical application value of an automatic diagnosis platform for rectal cancer T-staging based on a deep neural network. Chin Med J (Engl) 2021;134:821-8. [Crossref] [PubMed]
- Nguyen HH, Saarakkala S, Blaschko MB, Tiulpin A. Semixup: In- and Out-of-Manifold Regularization for Deep Semi-Supervised Knee Osteoarthritis Severity Grading From Plain Radiographs. IEEE Trans Med Imaging 2020;39:4346-56. [Crossref] [PubMed]
- Liu B, Luo J, Huang H. Toward automatic quantification of knee osteoarthritis severity using improved Faster R-CNN. Int J Comput Assist Radiol Surg 2020;15:457-66. [Crossref] [PubMed]
- Chaudhari AS, Stevens KJ, Wood JP, Chakraborty AK, Gibbons EK, Fang Z, Desai AD, Lee JH, Gold GE, Hargreaves BA. Utility of deep learning super-resolution in the context of osteoarthritis MRI biomarkers. J Magn Reson Imaging 2020;51:768-79. [Crossref] [PubMed]
- Kong AP, Robbins RM, Stensby JD, Wissman RD. The Lateral Knee Radiograph: A Detailed Review. J Knee Surg 2022;35:482-90. [Crossref] [PubMed]
- Minciullo L, Cootes T. Fully automated shape analysis for detection of Osteoarthritis from lateral knee radiographs. In: 2016 23rd International Conference on Pattern Recognition (ICPR). 2016:3787-91.
- Fripp J, Crozier S, Warfield SK, Ourselin S. Automatic segmentation of the bone and extraction of the bone-cartilage interface from magnetic resonance images of the knee. Phys Med Biol 2007;52:1617-31. [Crossref] [PubMed]
- Ronneberger O, Fischer P, Brox T. U-net: Convolutional networks for biomedical image segmentation. In: Navab N, Hornegger J, Wells W, Frangi A. editors. International Conference on Medical image computing and computer-assisted intervention. Cham: Springer, 2015:234-41.
- Selvaraju RR, Cogswell M, Das A, Vedantam R, Parikh D, Batra D. Grad-cam: Visual explanations from deep networks via gradient-based localization. In: Proceedings of the IEEE international conference on computer vision. 2017:618-26.
- van der Maaten L, Hinton G. Visualizing data using t-SNE. J Mach Learn Res 2008;9:2579-605.
- Teoh YX, Lai KW, Usman J, Goh SL, Mohafez H, Hasikin K, Qian P, Jiang Y, Zhang Y, Dhanalakshmi S. Discovering Knee Osteoarthritis Imaging Features for Diagnosis and Prognosis: Review of Manual Imaging Grading and Machine Learning Approaches. J Healthc Eng 2022;2022:4138666. [Crossref] [PubMed]
- Oka H, Muraki S, Akune T, Mabuchi A, Suzuki T, Yoshida H, Yamamoto S, Nakamura K, Yoshimura N, Kawaguchi H. Fully automatic quantification of knee osteoarthritis severity on plain radiographs. Osteoarthritis Cartilage 2008;16:1300-6. [Crossref] [PubMed]
- Oka H, Muraki S, Akune T, Nakamura K, Kawaguchi H, Yoshimura N. Normal and threshold values of radiographic parameters for knee osteoarthritis using a computer-assisted measuring system (KOACAD): the ROAD study. J Orthop Sci 2010;15:781-9. [Crossref] [PubMed]
- Hirvasniemi J, Thevenot J, Immonen V, Liikavainio T, Pulkkinen P, Jämsä T, Arokoski J, Saarakkala S. Quantification of differences in bone texture from plain radiographs in knees with and without osteoarthritis. Osteoarthritis Cartilage 2014;22:1724-31. [Crossref] [PubMed]
- Suresha S, Kidziński L, Halilaj E, Gold GE, Delp SL. Automated staging of knee osteoarthritis severity using deep neural networks. Osteoarthritis Cartilage 2018;26:S441. [Crossref]
- Swiecicki A, Li N, O'Donnell J, Said N, Yang J, Mather RC, Jiranek WA, Mazurowski MA. Deep learning-based algorithm for assessment of knee osteoarthritis severity in radiographs matches performance of radiologists. Comput Biol Med 2021;133:104334. [Crossref] [PubMed]
- Abdullah SS, Rajasekaran MP. Automatic detection and classification of knee osteoarthritis using deep learning approach. Radiol Med 2022;127:398-406. [Crossref] [PubMed]
- Tiulpin A, Saarakkala S. Automatic Grading of Individual Knee Osteoarthritis Features in Plain Radiographs Using Deep Convolutional Neural Networks. Diagnostics (Basel) 2020;10:932. [Crossref] [PubMed]
- Olsson S, Akbarian E, Lind A, Razavian AS, Gordon M. Automating classification of osteoarthritis according to Kellgren-Lawrence in the knee using deep learning in an unfiltered adult population. BMC Musculoskelet Disord 2021;22:844. [Crossref] [PubMed]
- Hirvasniemi J, Klein S, Bierma-Zeinstra S, Vernooij MW, Schiphof D, Oei EHG. A machine learning approach to distinguish between knees without and with osteoarthritis using MRI-based radiomic features from tibial bone. Eur Radiol 2021;31:8513-21. [Crossref] [PubMed]
- Xie X, Song Y, Ye F, Yan H, Wang S, Zhao X, Dai J. Prior information guided auto-contouring of breast gland for deformable image registration in postoperative breast cancer radiotherapy. Quant Imaging Med Surg 2021;11:4721-30. [Crossref] [PubMed]
- Leung K, Zhang B, Tan J, Shen Y, Geras KJ, Babb JS, Cho K, Chang G, Deniz CM. Prediction of Total Knee Replacement and Diagnosis of Osteoarthritis by Using Deep Learning on Knee Radiographs: Data from the Osteoarthritis Initiative. Radiology 2020;296:584-93. [Crossref] [PubMed]