Deep learning for predicting the human epidermal growth factor receptor 2 status of breast cancer liver metastases based on contrast-enhanced computed tomography: a development and validation study
Introduction
Breast cancer has the highest incidence rate of all malignant tumors in women, surpassing both lung and colon cancer, and is the fifth highest cause of cancer mortality overall (1). The leading cause of death in patients with breast cancer is distant metastases (2). Breast cancer is one of the most common primary cancers to metastasize to the liver (3). Due to the heterogeneity of breast cancer, there are differences in the expression of hormone receptors (HRs) and human epidermal growth factor receptor 2 (HER2) between primary and metastatic lesions in patients. These differences directly affect the treatment strategies and prognoses of patients, and are the main cause of treatment failure in patients with advanced breast cancer (4). At present, clinical precision treatment of advanced breast cancer is still guided by invasive procedures.
Deep learning (DL) algorithms can automatically learn from a large amount of data to obtain effective features, thus improving the performance of various machine learning tasks, and this technology has been widely used in the medical field (5,6). In recent years, DL methods have been applied to breast cancer lesion segmentation, imaging diagnosis, and molecular prediction; however, few studies have been conducted on metastatic lesions in patients with this malignancy (7-10). Currently, neural networks are used to process medical images to help with medical treatment. For example, Razmjooy et al. proposed a method to detect melanoma through image detection that eliminates additional information through morphological manipulation and is used to focus on areas where there may be melanoma boundaries (11). Xu et al. proposed an automatic computer-aided method for the early diagnosis of skin cancer that used the optimization features of the Satin Bowerbird optimization algorithm to trim excessive information (12). Cai et al. used image processing techniques and duct structures to analyze mammogram images to diagnose cancerous masses, thereby improving the quality of mammogram images and the contrast of abnormal areas in the images (13). However, these methods are traditional machine learning methods that require manual marking of some features and, as a result, some higher-order features may be missed.
With the rise of DL, its powerful feature extraction and differentiation capabilities have seen its application in the classification of breast cancer. For example, the Faster R-CNN (convolutional neural network) has been used to detect and classify tumors in single images from dynamic contrast-enhanced magnetic resonance imaging (MRI) (14), and the You Only Look Once (YOLO) network based on Darknet has been used to detect benign and malignant tumors on 600 mammograms (15). However, such technologies have rarely been used in the case of metastatic tumors. Therefore, in the present study, we wanted to use a deep neural network to determine the HER2 category of liver metastases from breast cancer so as to provide a reasonable basis for the selection of specific treatments for patients. Our proposed method makes use of the strong learning ability of deep neural networks. Unlike machine learning, our method does not require manually marking of some features, and it simplifies the heavy work process. In this study, we compared results using our method with those of some mainstream networks using the same batch of data.
In the past, breast cancer metastases could only be identified by invasive puncture biopsy, but prediction models represent a non-invasive technique. This study specifically investigated whether a DL model could be used to predict the HER2 expression status in breast cancer liver metastases on contrast-enhanced computed tomography (CT)-enhanced images to non-invasively guide clinical precision treatment and improve the curative effect. The new predictive model was established and validated internally to confirm its reliability. We present the following article in accordance with the TRIPOD reporting checklist (available at https://qims.amegroups.com/article/view/10.21037/qims-22-967/rc).
Methods
Participants
Patients with breast cancer liver metastases who, between January 2017 and March 2022, underwent contrast-enhanced CT imaging and for whom clinical and pathological data were available were enrolled in the present study. All patients were recruited from Affiliated Hospital of Hebei University.
This study was conducted with approval from the Ethics Committee of the Affiliated Hospital of Hebei University (No. HDFY-LL-2022-203) and was carried out in accordance with the Declaration of Helsinki (as revised in 2013). Written informed consent was obtained from all participants.
The inclusion criteria were as follows: (I) female patient with breast cancer who had liver metastases, (II) primary tumor and liver metastases had been pathologically confirmed via surgery or puncture, (III) the HER2 status had been confirmed via immunohistochemistry or fluorescence in situ hybridization (FISH), and (IV) patient had undergone contrast-enhanced CT examination prior to treatment of their liver metastases. Patients with a history of malignancies other than breast cancer, those who had undergone contrast-enhanced CT examination after treatment, and those for whom only poor-quality CT images were available were excluded from the study.
Based on the guidelines for HER2 testing in patients with breast cancer (16), the HER2 results from immunohistochemical tests were divided into four categories: 0, +, ++, and +++. If the HER2 result was negative, it was recorded as 0 or +. If the HER2 result was positive, it was recorded as +++. If the HER2 result was ++ and amplification was detected by FISH, it was deemed to be HER2 positive; in the absence of amplification, it was deemed to be HER2 negative.
CT examination
Contrast-enhanced CT imaging was performed on all patients using CT scanners (GE Discovery HD750 64 and Optima CT680). All patients had fasted for 6–8 h prior to the examination. For CT imaging, the contrast agent iohexol (0.5 mL/kg) was injected through the cubital vein at a flow rate of 3.0–3.5 mL/s. The scanning parameters were as follows: thickness, 5 mm; pitch, 0.992; field of view, 350 mm × 350 mm; matrix, 512×512; tube voltage, 100–120 kV; and tube current, 160–300 mA. Images of the liver in the arterial, portal venous, and delayed phases were obtained at 30–35, 50–60, and 180 s, respectively, after injection of the contrast agent. Contrast-enhanced CT images from the portal venous phase were selected to establish the model given that the liver metastases were best displayed in this phase. The procedure used for data processing and constructing the DL model is shown in Figure 1.
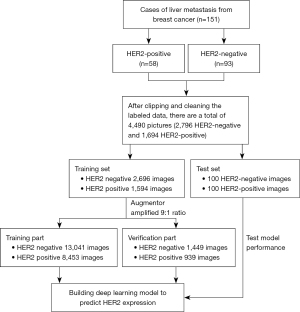
Data preprocessing
Digital Imaging and Communications in Medicine (DICOM) images were converted into a bitmap image file. The image is converted from DICOM format image to bmp format using RadiAnt DICOM Viewer software. Then, the region of interest (ROI) in the tumor was manually contoured by two radiologists (one with 8 years’ experience and the other with 13 years’ experience). Anaconda software for Windows (www.anaconda.com/products/individual) was used to analyze the tumors on the contrast-enhanced CT images in the portal venous phase. As shown in Figure 2, the regions were marked with rectangular boxes, layer by layer. Finally, a rectangular box was drawn outward by 0.5–1.0 cm, and JavaScript Object Notation files were saved based on the tumor’s maximum transverse and longitudinal diameters.
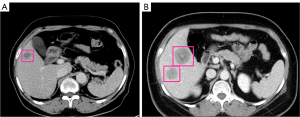
First, the annotated data were tailored to obtain small image blocks containing only tumors. At the same time, the data were cleaned to delete non-standard images. The 4,490 images that were obtained were divided into a training set (2,696 HER2 negative, 1,594 HER2 positive) and test set (100 HER2 negative, 100 HER2 positive). Data enhancement using Augmentor included seven image amplification methods: image counterclockwise random rotation of 90°, image clockwise random rotation of 90°, unfixed Angle microrotation, perspective deformation/vertical deformation, perspective deformation/oblique quadrangle deformation, cross-cut transformation, and random region erasure (17). These methods were used to increase the number of HER2-negative samples in the training set to 14,490 pieces and the number of HER2-positive samples to 9,392. The samples were then randomly divided at a 9:1 ratio into a training set (13,041 HER2-negative samples, 8,453 HER2-positive samples) and a validation set (1,449 HER2-negative samples, 939 HER2-positive samples).
Building the DL model
The training set data were verified using five basic networks: ResNet34, ResNet50, ResNet101, ResNeXt50, and Swim Transformer. The parameters were optimized on the better model by comparing the training loss value (Train Loss), validation accuracy, and test accuracy of the different basic networks (PseudoCode in the Appendix 1). The model’s performance was improved using the method of weight decay (wd) to solve the problem of overfitting, and the model’s performance was finally determined using the test set.
The gradient-weighted class activation mapping (Grad-CAM) visualization method was used to generate a heat map, visualize the ROI, reasonably explain the decision logic of the DL network, and intuitively view the ROI area that the model pays attention to (18).
Statistical analysis
Statistical analyses were performed using SPSS 25.0, with P<0.05 considered to be statistically significant. Measurement data are presented as the mean ± standard deviation (SD), and were compared using independent samples t-tests. Categorical data are presented as numbers and percentages (%), and were compared using the Chi-squared test. Receiver operating characteristic (ROC) curves were used to calculate the area under the curve (AUC), accuracy, sensitivity, specificity, and other parameters to comprehensively evaluate the performance of the model in predicting HER2 expression in patients with breast cancer liver metastasis.
Results
Patient characteristics
Samples from 151 female patients with breast cancer liver metastasis were collected in this study. Of the 151 patients, 58 had HER2-positive liver metastases (age range, 29–75 years; mean 54.97±9.96 years) and 93 were HER2 negative (age range, 32–78 years; mean 52.97±11.54 years). There was no significant difference in age or the number of liver metastases between the two groups (P>0.05; Table 1).
Table 1
Characteristic | HER2-negative group (n=93) | HER2-positive group (n=58) | t/χ2 | P value |
---|---|---|---|---|
Age (years) | 52.97±11.54 | 54.97±9.96 | 1.089 | 0.278 |
No. of liver metastases | 0.001 | 0.969 | ||
Single lesion | 19 (20.4) | 12 (20.7) | ||
Multiple lesions | 74 (79.6) | 46 (79.3) |
Unless indicated otherwise, data are given as the mean ± SD or n (%). HER2, human epidermal growth factor receptor 2; SD, standard deviation.
Classification performance of the DL model
Comparison of the five basic network models showed that the ResNet34 network performed best. On this basis, the learning rate (lr) and wd were adjusted. The ROC curve indicated that the performance of the model based on the ResNet34 network was the best when the parameters were lr =1e-4, wd =1e-3, and epoch (e) =350. The model’s accuracy in the validation and test sets was 87.4% and 80.5%, respectively. The prediction results of each model indicated that the AUC of the best model for predicting the expression of HER2 in breast cancer liver metastasis in the test set was 0.778, with a sensitivity of 77.0% and a specificity of 84.0% (Tables 2,3; Figure 3).
Table 2
Network | Train loss | Validation accuracy | Test accuracy |
---|---|---|---|
ResNet34 | 0.237 | 0.934 | 0.765 |
ResNet50 | 0.252 | 0.927 | 0.775 |
ResNet101 | 0.246 | 0.923 | 0.750 |
ResNeXt50 | 0.377 | 0.850 | 0.690 |
Swim Transformer | 0.592 | 0.684 | 0.670 |
The table shows the classification performance of the different base models, which provides a basis for the subsequent selection of base models.
Table 3
Model | Sensitivity | Specificity | AUC |
---|---|---|---|
ResNet34 | 0.69 | 0.84 | 0.767 |
ResNet50 | 0.76 | 0.79 | 0.774 |
ResNet101 | 0.71 | 0.79 | 0.778 |
ResNeXt50 | 0.60 | 0.78 | 0.800 |
Swim-Transformer | 0.54 | 0.80 | 0.745 |
ResNet34 (lr =1e-4) | 0.69 | 0.84 | 0.767 |
ResNet34 (lr =3e-4) | 0.67 | 0.79 | 0.793 |
ResNet34 (lr =7e-4) | 0.64 | 0.79 | 0.768 |
ResNet34 (lr =1e-4, wd =1e-3) | 0.74 | 0.73 | 0.793 |
ResNet34 (lr =3e-4, wd =1e-3) | 0.73 | 0.71 | 0.756 |
ResNet34 (lr =7e-4, wd =1e-3) | 0.66 | 0.73 | 0.737 |
ResNet34 (lr =1e-4, wd =1e-3, e=350) | 0.77 | 0.84 | 0.778 |
The table shows the sensitivity, specificity, and AUC of the different models, which were used to evaluate the performance of the models. AUC, area under the curve; lr, learning rate; wd, weight decay; e, epoch.
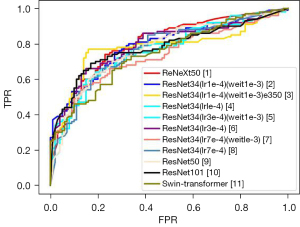
Results of ROI visualization
Figure 4 shows the ROI visualization results. It was clear that most of the edges of the liver metastases in the contrast-enhanced CT images in the portal venous phase images were enhanced and that the corresponding ROIs were mainly concentrated on the edge of the liver metastases. The model could accurately focus on the tumor location. A heat map was generated using the Grad-CAM visualization method and comprised mainly three colors, blue, yellow, and red. Blue indicated that the network model paid less attention to this area during the classification task; yellow indicated that the network model paid more attention to this area during the classification task; red indicated that the network model paid the greatest amount of attention to this area during the classification task.
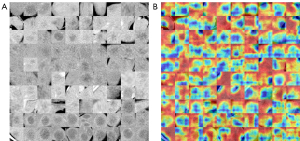
Discussion
Expression of HER2 is one of the most important molecular targets in breast cancer and is key to determining the molecular type. The characteristics of HER2-positive breast cancer include its strong invasiveness, high mortality, and insensitivity to traditional chemotherapy (19,20). Multiple studies (21,22) have demonstrated differences in HER2 expression between primary breast cancers and metastatic lesions, with changes in HER2 expression prompting changes in treatment strategies (23). Experts suggest that patients with recurrent and metastatic breast cancer are retested for HER2 expression to help clarify the HER2 status of any recurrent and metastatic lesions (24,25). Currently, only invasive immunohistochemistry or FISH testing can be used to determine the HER2 status of primary breast cancers and metastatic lesions. However, these methods cannot be used in some patients because of their physical condition does not tolerate invasive puncture examination or because the location of the lesion makes it unsuitable for biopsy. Therefore, developing a non-invasive and accurate method for assessing the HER2 status of metastases in patients with breast cancer is of critical importance.
In recent years, many studies have investigated the use of traditional machine learning and DL methods based on images for the prediction of HER2 expression in primary breast cancers. Zhang et al. (26) divided patients with breast cancer into three subtypes according to HR and HER2 expression (i.e., HR+/HER2−, HER2+, and triple negative), and devised a model to predict classification. Based on DCE sequence images, the traditional convolutional neural network and convolutional long and short time memory were used to establish the prediction models, and the accuracy of the two models in the verification set reached 0.91 and 0.83, respectively in the validation set. In another study, Xue et al. (27) constructed models based on MRI radiomic features and achieved a strong diagnostic performance. Zhou et al. (28) used multiparametric MRI radiomic labels to predict HER2 expression in breast cancer to distinguish HER2-positive from HER2-negative breast cancers, with an AUC of 0.70 in the validation set. However, few studies have focused on HER2 expression in breast cancer metastases based on imaging.
In the present study, a model to predict HER2 expression in breast cancer liver metastases was constructed based on a contrast-enhanced CT DL method. The results indicated that the best model predicted HER2 expression with an accuracy of 80.5%, an AUC of 0.778, a sensitivity of 77.0%, and a specificity of 84.0% in the test set. The model demonstrated good stability and diagnostic performance. For most of the models, the accuracy was generally higher for the negative samples than it was for the positive samples. We speculate that this difference in accuracy was due to the data imbalance caused by the 5:3 ratio of negative to positive training samples in the training set.
A previous study (29) found that the central area of liver metastases primarily consists of necrotic tissue and a small number of tumor cells, with mainly tumor cells and new capillaries at the periphery; as a result, most liver metastases show ring enhancement on contrast-enhanced CT images. ROI visualization results showed that the model’s decision basis for predicting HER2 status was mostly located at the tumor margin, which is consistent with previous reports that metastatic tumor cells are primarily located at the edge of the lesion. However, there were a few cases in which the model could not correctly focus on the tumor location, which affected the accuracy of the model’s judgment. Conducting further in-depth research and improving the model’s recognition of the tumor area could improve the accuracy of the model.
The present study has a number of limitations. First, it was a single-center, small-sample, retrospective study involving a single data source and similar scanning parameters. As a result, the generalization of the model constructed in this study needs to be further improved and its general application in clinical practice is limited. In future research, a multicenter, large-sample, prospective study is required to establish a more objective and accurate prediction model. Furthermore, the data for the two sample types were unbalanced, which may have contributed to differences in the accuracy rate. In addition, the model requires human-labeled ROIs as inputs, making it expensive and introducing potential for error; therefore, a model that works with the entire CT image rather than requiring radiologists to crop out cancerous regions needs to be created.
In conclusion, the DL model established in this study can be used as a non-invasive tool for predicting the expression of HER2 in breast cancer liver metastases.
Acknowledgments
Funding: This study was supported by Tianjin Key Medical Discipline (Specialty) Construction Project (No. TJYXZDXK-041A), the Natural Science Foundation of Tianjin, China (No. 21JCYBJC01050), and the CT Radiomics Study on the Correlation between Colorectal Liver Metastasis and Microsatellite Instability, and The Financial Department of Hebei Province, Health Commission of Hebei Province (No. 361007).
Footnote
Reporting Checklist: The authors have completed the TRIPOD reporting checklist. Available at https://qims.amegroups.com/article/view/10.21037/qims-22-967/rc
Conflicts of Interest: All authors have completed the ICMJE uniform disclosure form (available at https://qims.amegroups.com/article/view/10.21037/qims-22-967/coif). The authors have no conflicts of interest to declare.
Ethical Statement: The authors are accountable for all aspects of the work in ensuring that questions related to the accuracy or integrity of any part of the work are appropriately investigated and resolved. This study was conducted with approval from the Ethics Committee of the Affiliated Hospital of Hebei University (No. HDFY-LL-2022-203). This study was conducted in accordance with the Declaration of Helsinki (as revised in 2013). Written informed consent was obtained from all participants.
Open Access Statement: This is an Open Access article distributed in accordance with the Creative Commons Attribution-NonCommercial-NoDerivs 4.0 International License (CC BY-NC-ND 4.0), which permits the non-commercial replication and distribution of the article with the strict proviso that no changes or edits are made and the original work is properly cited (including links to both the formal publication through the relevant DOI and the license). See: https://creativecommons.org/licenses/by-nc-nd/4.0/.
References
- Sung H, Ferlay J, Siegel RL, Laversanne M, Soerjomataram I, Jemal A, Bray F. Global Cancer Statistics 2020: GLOBOCAN Estimates of Incidence and Mortality Worldwide for 36 Cancers in 185 Countries. CA Cancer J Clin 2021;71:209-49. [Crossref] [PubMed]
- Hu JY, Yi W, Wei X, Zhang MY, Xu R, Zeng LS, Huang ZJ, Chen JS. miR-601 is a prognostic marker and suppresses cell growth and invasion by targeting PTP4A1 in breast cancer. Biomed Pharmacother 2016;79:247-53. [Crossref] [PubMed]
- Savci-Heijink CD, Halfwerk H, Hooijer GK, Horlings HM, Wesseling J, van de Vijver MJ. Retrospective analysis of metastatic behaviour of breast cancer subtypes. Breast Cancer Res Treat 2015;150:547-57. [Crossref] [PubMed]
- McAnena PF, McGuire A, Ramli A, Curran C, Malone C, McLaughlin R, Barry K, Brown JAL, Kerin MJ. Breast cancer subtype discordance: impact on post-recurrence survival and potential treatment options. BMC Cancer 2018;18:203. [Crossref] [PubMed]
- Park HJ, Park B, Lee SS. Radiomics and Deep Learning: Hepatic Applications. Korean J Radiol 2020;21:387-401. [Crossref] [PubMed]
- Chan HP, Samala RK, Hadjiiski LM, Zhou C. Deep Learning in Medical Image Analysis. Adv Exp Med Biol 2020;1213:3-21. [Crossref] [PubMed]
- Hirsch L, Huang Y, Luo S, Rossi Saccarelli C, Lo Gullo R, Daimiel Naranjo I, et al. Radiologist-Level Performance by Using Deep Learning for Segmentation of Breast Cancers on MRI Scans. Radiol Artif Intell 2021;4:e200231. [Crossref] [PubMed]
- Truhn D, Schrading S, Haarburger C, Schneider H, Merhof D, Kuhl C. Radiomic versus Convolutional Neural Networks Analysis for Classification of Contrast-enhancing Lesions at Multiparametric Breast MRI. Radiology 2019;290:290-7. [Crossref] [PubMed]
- Ha R, Mutasa S, Karcich J, Gupta N, Pascual Van Sant E, Nemer J, Sun M, Chang P, Liu MZ, Jambawalikar S. Predicting Breast Cancer Molecular Subtype with MRI Dataset Utilizing Convolutional Neural Network Algorithm. J Digit Imaging 2019;32:276-82. [Crossref] [PubMed]
- Ye G, He S, Pan R, Zhu L, Zhou D, Lu R. Research on DCE-MRI Images Based on Deep Transfer Learning in Breast Cancer Adjuvant Curative Effect Prediction. J Healthc Eng 2022;2022:4477099. [Crossref] [PubMed]
- Razmjooy N, Sheykhahmad FR, Ghadimi N. A Hybrid Neural Network – World Cup Optimization Algorithm for Melanoma Detection. Open Med (Wars) 2018;13:9-16. [Crossref] [PubMed]
- Xu Z, Sheykhahmad FR, Ghadimi N, Razmjooy N. Computer-aided diagnosis of skin cancer based on soft computing techniques. Open Med (Wars) 2020;15:860-71. [Crossref] [PubMed]
- Cai X, Li X, Razmjooy N, Ghadimi N. Breast Cancer Diagnosis by Convolutional Neural Network and Advanced Thermal Exchange Optimization Algorithm. Comput Math Methods Med 2021;2021:5595180. [Crossref] [PubMed]
- Jiao H, Jiang X, Pang Z, Lin X, Huang Y, Li L. Deep Convolutional Neural Networks-Based Automatic Breast Segmentation and Mass Detection in DCE-MRI. Comput Math Methods Med 2020;2020:2413706. [Crossref] [PubMed]
- Al-Masni MA, Al-Antari MA, Park JM, Gi G, Kim TY, Rivera P, Valarezo E, Han SM, Kim TS. Detection and classification of the breast abnormalities in digital mammograms via regional Convolutional Neural Network. In: 2017 39th Annual International Conference of the IEEE Engineering in Medicine and Biology Society (EMBC). Jeju: IEEE, 2017:1230-3.
- Zhang H, Moisini I, Ajabnoor RM, Turner BM, Hicks DG. Applying the New Guidelines of HER2 Testing in Breast Cancer. Curr Oncol Rep 2020;22:51. [Crossref] [PubMed]
- Bloice MD, Roth PM, Holzinger A. Biomedical image augmentation using Augmentor. Bioinformatics 2019;35:4522-4. [Crossref] [PubMed]
- Selvaraju RR, Cogswell M, Das A, Vedantam R, Parikh D, Batra D. Grad-CAM: Visual Explanations from Deep Networks via Gradient-Based Localization. Int J Comput Vis 2020;128:336-59. [Crossref]
- Kreutzfeldt J, Rozeboom B, Dey N, De P. The trastuzumab era: current and upcoming targeted HER2+ breast cancer therapies. Am J Cancer Res 2020;10:1045-67. [PubMed]
- Rojas K, Stuckey A. Breast Cancer Epidemiology and Risk Factors. Clin Obstet Gynecol 2016;59:651-72. [Crossref] [PubMed]
- Cejalvo JM, Martínez de Dueñas E, Galván P, García-Recio S, Burgués Gasión O, Paré L, et al. Intrinsic Subtypes and Gene Expression Profiles in Primary and Metastatic Breast Cancer. Cancer Res 2017;77:2213-21. [Crossref] [PubMed]
- Idirisinghe PK, Thike AA, Cheok PY, Tse GM, Lui PC, Fook-Chong S, Wong NS, Tan PH. Hormone receptor and c-ERBB2 status in distant metastatic and locally recurrent breast cancer. Pathologic correlations and clinical significance. Am J Clin Pathol 2010;133:416-29. [Crossref] [PubMed]
- El Nemr Esmail RS, El Farouk Abdel-Salam LO, Abd El Ellah MM. Could the Breast Prognostic Biomarker Status Change During Disease Progression? An Immunohistochemical Comparison between Primary Tumors and Synchronous Nodal Metastasis. Asian Pac J Cancer Prev 2015;16:4317-21. [Crossref] [PubMed]
- Gianni L, Pienkowski T. Im YH, Tseng LM, Liu MC, Lluch A, Starosławska E, de la Haba-Rodriguez J, Im SA, Pedrini JL, Poirier B, Morandi P, Semiglazov V, Srimuninnimit V, Bianchi GV, Magazzù D, McNally V, Douthwaite H, Ross G, Valagussa P. 5-year analysis of neoadjuvant pertuzumab and trastuzumab in patients with locally advanced, inflammatory, or early-stage HER2-positive breast cancer (NeoSphere): a multicentre, open-label, phase 2 randomised trial. Lancet Oncol 2016;17:791-800. [Crossref] [PubMed]
- Martínez-Sáez O, Prat A. Current and Future Management of HER2-Positive Metastatic Breast Cancer. JCO Oncol Pract 2021;17:594-604. [Crossref] [PubMed]
- Zhang Y, Chen JH, Lin Y, Chan S, Zhou J, Chow D, Chang P, Kwong T, Yeh DC, Wang X, Parajuli R, Mehta RS, Wang M, Su MY. Prediction of breast cancer molecular subtypes on DCE-MRI using convolutional neural network with transfer learning between two centers. Eur Radiol 2021;31:2559-67. [Crossref] [PubMed]
- Xue K, Li ZL, Li ZH, Zhao ML, Sun SY, Ding YY. Identify HER-2 over expression breast on radiomics of multi-oarametric MRI. Radiol Pract 2020;35:186-9.
- Zhou J, Tan H, Li W, Liu Z, Wu Y, Bai Y, Fu F, Jia X, Feng A, Liu H, Wang M. Radiomics Signatures Based on Multiparametric MRI for the Preoperative Prediction of the HER2 Status of Patients with Breast Cancer. Acad Radiol 2021;28:1352-60. [Crossref] [PubMed]
- van Amerongen MJ, Vos AM, van der Woude W, Nagtegaal ID, de Wilt JHW, Fütterer JJ, Hermans JJ. Does perfusion computed tomography correlate to pathology in colorectal liver metastases? PLoS One 2021;16:e0245764. [Crossref] [PubMed]