Deep learning image reconstruction algorithms in low-dose radiation abdominal computed tomography: assessment of image quality and lesion diagnostic confidence
Introduction
Computed tomography (CT) is an extremely common imaging modality for displaying anatomical structures and lesions, maintaining the advantages of high resolution and fast imaging speed. For a large of number patients who undergo CT examinations, there are numerous issues to consider. For example, radiation exposure is a requisite concern for patient safety, especially for pediatric patients or those who undergo multiple CT scans (1,2). Therefore, the application of low-dose radiation has attracted much attention in a bid to reduce its harmful effects. Many techniques have been applied to reduce the radiation dose, such as patient-customized tube parameter selection, beam-shaping filter, and automatic tube current modulation (ATCM) (3). The abdomen contains many parenchyma organs with small density differences. The detection and screening of low-contrast liver lesions are more difficult under a low radiation dose, and thus, various image reconstruction algorithms have been developed to maintain the balance of good image quality and the reduction of radiation dose.
Filtered back projection (FBP) is the original standard reconstruction technology. However, prominent image noise and artifacts appear when images are reconstructed under low-dose radiation (4). With the development of computational power, iterative reconstruction (IR) techniques have been applied to clinical practice, which, under a lower dose of radiation, can reduce image noise and maintain or improve image quality compared with FBP. IR includes hybrid- and model-based techniques (5). Among the hybrid-IR techniques is adaptive statistical iterative reconstruction (ASiR), which was developed by GE Healthcare (6). It can suppress image noise and improve image quality under reduced radiation dose conditions (7). The noise index (NI) is key to controlling the output of tube current, and the radiation dose can be reduced by adapting the NI. Model-based iterative reconstruction (MBIR) can dramatically reduce noise but with a significantly longer reconstruction time. In addition, its associated altered image texture is a major concern (8-10). Adaptive statistical iterative reconstruction-Veo (ASiR-V), a more advanced hybrid reconstruction algorithm, can function better under a lower radiation dose than can ASiR (11,12). Nevertheless, multiple studies have reported that with the increase of IR strength, “blotchy”, “plastic-looking”, or “unnatural” noise texture can appear in images (13-18).
To overcome the shortcomings of IR, deep leaning (DL)–based algorithms, in pace with the advancement of artificial intelligence (AI), have been developed and applied in clinic. Some DL image reconstruction algorithms include (DLIR, TrueFidelity, GE Healthcare) and Advanced intelligent Clear-IQ Engine (AiCE, Canon Medical Systems). The DLIR uses deep neural networks (DNNs) to simulate high-quality FBP images while ensuring low image noise, suppression of streak artifacts, and high resolution (19-22). DLIR has been shown to significantly reduce image noise and enhance spatial resolution and detectability in the lungs, abdomen, coronary artery, and the head (21,23-26). Several studies have examined abdominal CT with DLIR or with AiCE using phantom or patients (20,27,28). However, this DLIR research has focused mainly on comparisons with FBP or single- or double-strength ASiR. Additionally, the influence of DLIR on image quality and diagnostic confidence in low-dose radiation abdominal CT remains unclear.
In this study, we used phantom data to evaluate the DLIR algorithm’s ability to improve image quality without changing image texture and used patient data to determine the effect of the DLIR algorithm on the diagnostic confidence of low-dose radiation abdominal CT. We also examined the influence of DLIR and IR of different strengths on image quality and diagnostic confidence by comparing DLIR at low, medium, and high strengths with ASiR-V and FBP reconstructions of various strengths.
Methods
Phantom and CT technique
The Catphan 500 phantom (The Phantom Laboratory, Greenwich, NY, USA; Figure 1A) was scanned on a 256 slice CT scanner (Revolution CT, GE Healthcare, Chicago, IL, USA) with different tube currents, including 50, 100, 150, and 200 mA. The following imaging parameters were applied for all groups: 120 kVp tube voltage and 0.5 s rotation speed. Eight sets of CT images were reconstructed with a 0.625-mm slice thickness using FBP; ASiR-V at 30% (AV-30), 50% (AV-50), 80% (AV-80), and 100% (AV-100); and deep learning image reconstruction (DLIR) at low (DL-L), medium (DL-M), and high (DL-H) levels. We recorded the noise power spectrum (NPS) and the in-plane task-based transfer function (TTF) under various levels of ASiR-V and DLIR strengths. NPS was measured from uniform images of the water-only volume (Figure 1A), and the calculation of TTF was performed on the sensitometry module (Figure 1B) containing varying contrast targets.
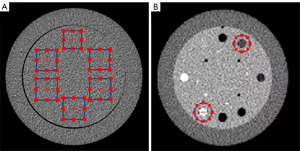
Patients
This study was conducted in accordance with the Declaration of Helsinki (as revised in 2013). The prospective study from August to December 2020 was approved by the local institutional review board of the First Affiliated Hospital of Shandong First Medical University, and all participants signed written informed consent. A total of 38 consecutive patients with suspected liver lesions underwent low-dose radiation contrast-enhanced abdominal CT. Figure 2 summarizes the inclusion and exclusion criteria. The following exclusion criteria were applied: anaphylactic reaction to iodinated contrast medium, renal dysfunction, and undergoing dual-energy CT acquisition. Finally, 30 patients (19 men and 11 women; age range 28–84 years; mean age 60.23±12.60 years), who had a mean body weight of 67.03±10.11 kg and a mean body mass index (BMI) of 23.36±2.14 kg/m2, were enrolled in the study (Table 1).
Table 1
Variable | Result |
---|---|
Participant demographics | |
Age (year) | 60.23±12.60 |
Gender | |
Male | 19 (63.3) |
Female | 11 (36.7) |
Body weight (kg) | 67.03±10.11 |
Body mass index (kg/m2) | 23.36±2.14 |
Liver lesion | |
Simple cyst | 141 (64.7) |
Liver metastasis | 25 (11.5) |
Hepatic hamartoma | 21 (9.6) |
Bile duct hamartoma in liver | 20 (9.2) |
Hepatic hemangioma | 5 (2.3) |
Hepatocellular carcinoma | 4 (1.8) |
Intrahepatic cholangiocarcinoma | 1 (0.5) |
Mesenchymal hamartoma of the liver | 1 (0.5) |
Data are presented as the mean ± standard deviation or as n (%).
Imaging technique and reconstruction
All participants consecutively underwent low-dose radiation abdominal CT in the portal venous phase under the following parameters: rotation time, 0.5 s; pitch, 0.992:1; scan slice thickness, 5 mm; reconstructed thickness 0.625 mm; tube voltage, 120 kV; automatic tube current modulation range, 100–600 mA; and NI, 15 (defined at 5-mm slice thickness). Images on the portal venous phase were reconstructed with FBP, AV-30, AV-50, AV-80, AV-100, DL-L, DL-M, and DL-H using the standard kernel.
The intravenous injection (IV) contrast agent iohexol (Omnipaque 300, Yangtze River Pharmaceutical Group, Taizhou, China) was used. A weight-based IV contrast volume determination of 1.2 mL/kg was applied with an injection speed of 3.0 mL/s. The bolus tracking technique was adopted to monitor the area of interest, and the trigger threshold was set at 150 Hounsfield units (HU) on the abdominal aorta of the second hepatic portal level. The arterial phase scan started with a delay of 5.6 seconds after the trigger threshold in the monitoring region of interest (ROI), which reached the triggering threshold. The portal venous phase scan started with a delay of 25 seconds after the arterial phase scan.
Quantitative image assessment
One radiologist (with 5 years of experience in abdominal radiology) who was blinded to all reconstructed images placed the ROIs in the hepatic parenchyma and the right side of the paraspinal muscle on an Advantage Workstation 4.6 (GE Healthcare). Eight reconstructed images were linked to delineate identical ROIs in the same anatomic structure for different reconstructed images. The CT values (HU) and standard deviation (SD) were calculated by measuring the ROIs. ROIs of approximately 100 mm2 in area were drawn on the hepatic parenchyma of the right hepatic lobe on the 3 adjacent slices at the porta hepatis. ROIs 100–150 mm2 in area were placed in the right side of paraspinal muscle on same 3 adjacent slices at the previous hepatic parenchyma. Visible hepatic vessels, bile ducts, focal lesions, calcification, edges, and artifacts were carefully avoided. Each value of the different structures was calculated by averaging 3 measurements. The SD of the paraspinal muscle was regarded as an objective measurement of image noise. Signal-to-noise ratio (SNR) and contrast-to-noise ratio (CNR) of the liver were calculated as follows:
For the phantom study, NPS was calculated to evaluate the noise texture and magnitude (29,30). NPS analysis was performed using the radial frequency method based on a 2-dimensional Fourier transform (31). Noise spectrum peaks denoted the maximum noise at 1 spatial frequency, and the average spatial frequency indicated the frequency at which the maximum NPS was reached. The NPS peak and average spatial frequency for the 8 sets of reconstructed images were evaluated and compared.
TTF was performed to assess spatial resolution (13). Two circular ROIs were placed around two inserts, and a circular-edge technique was used to measure the edge spread function (ESF). The line spread function (LSF) was then achieved via derivation of the ESF. TTF was calculated from the LSF-normalized Fourier transformation (32). Similarly, the TTF values at 50% (TTF50%, mm−1) for the 8 sets of reconstructed images were evaluated and compared to quantify the changes of spatial resolution.
Qualitative image assessment
Eight sets of reconstructed images were randomly reviewed via standard clinical protocol with high-resolution monitors, and the reconstruction information was eliminated.
Two abdominal radiologists (with 9 and 11 years of experience) independently completed the subjective assessment. The initial window width and level of all images were 400 and 40 HU, respectively. Readers could adjust the window width and level while analyzing lesions. A 5-point Likert scale (33) was used to rank subject image noise, image texture and sharpness, small-vessel visibility, and diagnostic confidence (Table 2). The mean value of the 2 radiologists was considered the final score.
Table 2
Score | Subject image noise | Image texture and sharpness | Small-vessel visibility | Diagnostic confidence |
---|---|---|---|---|
1 | Unacceptable | Serious blurred delineation | Unacceptable | Unacceptable |
2 | Above average | Suboptimal blurred delineation | Suboptimal | Suboptimal |
3 | Average | Moderate blurred delineation | Acceptable | Acceptable |
4 | Less than average | Minimal blurred delineation | Good | Good |
5 | Minimal | Hardly any blurring and well-displayed delineation | Excellent | Excellent |
Radiation dose evaluation
The CT dose index (CTDIvol) in milligray (mGy) and dose length product (DLP) in milligray-centimeter (mGy·cm) were recorded from the dose report. The effective radiation (ED) in millisievert (mSv) was calculated to represent radiation exposure using the DLP in mGy·cm multiplied by an abdomen conversion coefficient of 0.015 mSv/mGy·cm.
Statistical analysis
Statistical analyses were performed with SPSS statistical software (version 26 for Windows; IBM Corp. Armonk, NY, USA). Continuous variables are expressed as mean ± SD and were tested for normality using the Shapiro-Wilk test. Categorical variables are expressed as numbers. Quantitative data with normal distributions were compared using analysis of variance (ANOVA), and quantitative data without normal distributions were compared using the Kruskal-Wallis test. Bonferroni correction was used to adjust for pairwise comparisons if there were statistically significant differences. The kappa test was used to assess agreement between 2 radiologists. A kappa value in the range of 0.00–0.20 was slight, 0.21–0.40 was fair, 0.41–0.60 was moderate, 0.61–0.80 was substantial, and 0.81–1.00 was almost perfect.
Results
Quantitative assessment of the phantom
The noise, NPS peaks, and average spatial frequencies for the phantom are detailed in Table 3. The noise level is indicated in SD (HU). A higher strength of DLIR and ASiR-V led to a lower level of noise, and a higher dose resulted in a lower level of noise. At each dose level, noise levels of DL-L were comparable to those of AV-50. Moreover, with the increment of reconstructed strength, the noise decreased and images became smoother for both ASiR-V and DLIR. Compared with those of ASiR-V, DLIR reconstructions better maintained the noise texture of FBP reconstructions, with the images being less blurred.
Table 3
50 mA | 100 mA | 150 mA | 200 mA | |
---|---|---|---|---|
Noise (HU) | ||||
FBP | 41.3043 | 30.5444 | 25.0197 | 21.4577 |
AV-30 | 32.5606 | 24.0994 | 19.7209 | 16.8955 |
AV-50 | 26.7706 | 19.8267 | 16.2084 | 13.8587 |
AV-80 | 18.4297 | 13.6588 | 11.1071 | 9.4756 |
AV-100 | 13.1694 | 10.0578 | 8.1843 | 6.9447 |
DL-L | 27.3244 | 19.4344 | 16.0818 | 13.9488 |
DL-M | 21.9028 | 15.8037 | 13.1265 | 11.4632 |
DL-H | 16.0927 | 11.8503 | 9.9109 | 8.7437 |
fpeak (mm–1) | ||||
FBP | 0.2362 | 0.2677 | 0.2992 | 0.2835 |
AV-30 | 0.2047 | 0.2577 | 0.2362 | 0.2677 |
AV-50 | 0.2047 | 0.2205 | 0.2047 | 0.2520 |
AV-80 | 0.1890 | 0.1732 | 0.1260 | 0.1417 |
AV-100 | 0.1575 | 0.1102 | 0.1260 | 0.1417 |
DL-L | 0.2047 | 0.2677 | 0.2992 | 0.2835 |
DL-M | 0.2047 | 0.2677 | 0.2677 | 0.2835 |
DL-H | 0.2047 | 0.2205 | 0.2677 | 0.2835 |
favg (mm–1) | ||||
FBP | 0.3160 | 0.3188 | 0.3229 | 0.3213 |
AV-30 | 0.2959 | 0.2989 | 0.3032 | 0.3017 |
AV-50 | 0.2759 | 0.2788 | 0.2831 | 0.2825 |
AV-80 | 0.2293 | 0.2314 | 0.2358 | 0.2355 |
AV-100 | 0.1849 | 0.1895 | 0.1924 | 0.1934 |
DL-L | 0.2980 | 0.3019 | 0.3080 | 0.3066 |
DL-M | 0.2858 | 0.2926 | 0.2992 | 0.2987 |
DL-H | 0.2638 | 0.2764 | 0.2848 | 0.2845 |
NPS, noise power spectrum; FBP, filtered back projection; AV, adaptive statistical iterative reconstruction-Veo; DL-L, deep learning image reconstruction-low; DL-M, deep learning image reconstruction-medium; DL-H, deep learning image reconstruction-high.
As tube current increased, the peak/average frequency values of the DLIR algorithms were closer to those of FBP. Therefore, a better similarity was found between the DLIR and FBP. Meanwhile, average spatial frequency did not change significantly. AV-80 and AV-100 resulted in a lower peak/average frequency compared to DL-M and DL-H. Moreover, with the strengthening of levels, these 2 parameters also declined for both ASiR-V and DLIR.
The TTF50% values of all reconstruction algorithms are summarized in Table 4. For the polystyrene insert, the TTF50% values tended to increase with the enhancement of ASiR-V strength, especially at lower dose levels. Moreover, the TTF50% values of DLIR were higher than those of FBP at 150 mA. For the Teflon insert, the TTF50% values tended to decrease with the enhancement of ASiR-V and DLIR strength, except at 200 mA. The task-based transfer functions of FBP and ASiR-V reconstructions were comparable at 100 and 150 mA. The TTF50% values for DLIR were higher than those for FBP, except at 50 mA.
Table 4
Reconstruction algorithm | TTF50% polystyrene (mm−1) | TTF50% Teflon (mm−1) | |||||||
---|---|---|---|---|---|---|---|---|---|
50 mA | 100 mA | 150 mA | 200 mA | 50 mA | 100 mA | 150 mA | 200 mA | ||
FBP | 0.4341 | 0.4209 | 0.4076 | 0.4402 | 0.6105 | 0.3946 | 0.4164 | 0.4177 | |
AV-30 | 0.4328 | 0.4187 | 0.4178 | 0.4421 | 0.5431 | 0.3965 | 0.4221 | 0.4293 | |
AV-50 | 0.4385 | 0.4262 | 0.4206 | 0.4436 | 0.4910 | 0.3968 | 0.4214 | 0.4386 | |
AV-80 | 0.4460 | 0.4327 | 0.4254 | 0.4460 | 0.4180 | 0.3988 | 0.4214 | 0.4561 | |
AV-100 | 0.4294 | 0.4350 | 0.4264 | 0.4455 | 0.3657 | 0.3980 | 0.4148 | 0.4443 | |
DL-L | 0.4182 | 0.4252 | 0.4180 | 0.4403 | 0.4505 | 0.4286 | 0.4456 | 0.4450 | |
DL-M | 0.4177 | 0.4178 | 0.4166 | 0.4375 | 0.4253 | 0.4180 | 0.4410 | 0.4520 | |
DL-H | 0.4175 | 0.4188 | 0.4146 | 0.4378 | 0.4041 | 0.4152 | 0.4238 | 0.4472 |
TTF50%, task-based transfer function values at 50%; FBP, filtered back projection; AV, adaptive statistical iterative reconstruction-Veo; DL-L, deep learning image reconstruction-low; DL-M, deep learning image reconstruction-medium; DL-H, deep learning image reconstruction-high.
Lesion characteristics and radiation dose of patients
Patient demographic and pathologic information are detailed in Table 1. A total of 218 liver lesions were detected in 30 patients, including 141 simple cysts, 25 liver metastases, 21 hepatic hamartomas, 20 bile duct hamartomas in the liver (LBDH), 5 hepatic hemangiomas, 4 hepatocellular carcinomas (HCCs), 1 intrahepatic cholangiocarcinoma (ICCA) and 1 mesenchymal hamartoma of the liver (MHL).
The mean CTDIvol, DLP, and ED of low-dose radiation CT in the portal venous phase was 7.45±1.88 mGy (range, 3.66–11.55 mGy), 292.82±115.45 mGy·cm (range, 123.18–538.17 mGy·cm), and 4.39±1.73 mSv (range, 2.54–5.47 mSv), respectively. The mean CTDIvol in the portal venous phase was about 45.5% of that in the arterial phase which was 16.37±1.25 mGy (10.76–17mGy), representing a 55.5% dose reduction.
Quantitative image assessment
The objective image quality parameters are summarized in Table 5. According to the measured SD and calculated SNR, FBP generated the highest noise and the lowest SNR, and there was statistical differences when compared with the different strengths of ASiR-V and DLIR (P<0.001). The SD values of AV-80 were lower than those of DL-L and DL-M (P<0.01), and the SNR of AV-80 was higher than that of DL-L (P<0.001). However, the SNR of AV-80 was not significantly different to that of DL-M (P>0.05). AV-50 produced higher SD values and a lower SNR than did DL-M and DL-H (P<0.001), but there was no statistical difference with DL-L (P>0.05). The CNR of DL-H and was higher than that of AV-50 (P<0.05), but AV-50 was not statistically different from DL-L (P>0.05). There was no statistical difference between DL-M and AV-80 or between DL-H and AV-80 (P>0.05). Among the DLIR algorithms, there were significant differences in SD, SNR, and CNR between D-L and DL-H (P<0.01); however, CNR showed no significant difference between DL-M and DL-H (P>0.05).
Table 5
FBP | AV-30 | AV-50 | AV-80 | AV-100 | DL-L | DL-M | DL-H | P value | |
---|---|---|---|---|---|---|---|---|---|
SD | 36.56±2.92c,d,e,f,g,h | 29.17±2.38d,e,g,h | 24.21±2.10d,e,h | 17.09±1.89e,f | 12.51±1.87f, g | 23.50±1.91h | 18.61±1.78h | 13.51±1.59 | ≤0.001 |
SNR | 2.87±0.48c,d,e,f,g,h | 3.63±0.60d,e,g,h | 4.38±0.73d,e,h | 6.24±0.95f | 8.77±1.41f, g | 4.51±0.78h | 5.67±0.98h | 7.92±1.37 | ≤0.001 |
CNR | 1.17±0.37d,e,f,g,h | 1.47±0.46d,e,g,h | 1.77±0.56d,e,h | 2.52±0.80 | 3.48±1.18f | 1.82±0.55h | 2.31±0.70 | 3.18±0.97 | ≤0.001 |
Data are presented as the mean ± standard deviation. c, Statistical significance with AV-50, P≤0.001. d, Statistical significance with AV-80, P<0.05. e, Statistical significance with AV-100, P<0.05. f, Statistical significance with DL-L, P<0.05. g, Statistical significance with DL-M, P≤0.001. h, Statistical significance with DL-H, P<0.05. SD, standard deviation for paraspinal muscle; SNR, signal-to-noise ratio of liver; CNR, contrast-to-noise ratio of liver.
Qualitative image assessment
All metrics are summarized in Table 6 and Figure 3. According to the kappa test, the consistency between the 2 readers was more than 0.70. For subject image noise, with the improvement of reconstruction strength, the noise gradually decreased and the Likert score gradually increased. There were no statistical differences between AV-50 and DL-L or between AV-80 and DL-M (P>0.05). The image noise score from DL-M was higher than that from AV-30, AV-50, and DL-L (P<0.01). For image texture and sharpness, small-vessel visibility, and lesion diagnostic confidence, DL-M acquired significantly higher scores compared with the other algorithm strengths (P<0.05). Among the ASiR-V algorithms, AV-50 best balanced image noise, image texture, and spatial resolution when reconstructing patient images. DL-H generated significantly lower image noise, both subjectively and objectively compared to AV-50 and DL-L. However, there were no statistically significant differences among these modalities in terms of image texture and sharpness, small-vessel visibility, or lesion diagnostic confidence. Furthermore, these scores were significantly higher than those of FBP, AV-30, AV-80, and AV-100 (P<0.05); however, for small-vessel visibility, there was no significant difference between AV-80 and DL-H.
Table 6
Radiologists | FBP | AV-30 | AV-50 | AV-80 | AV-100 | DL-L | DL-M | DL-H | |
---|---|---|---|---|---|---|---|---|---|
Image noise κ value =0.796 | 1 | 1.00±0.00 | 2.93±0.37 | 3.03±0.61 | 3.97±0.41 | 4.80±0.41 | 2.97±0.49 | 4.00±0.45 | 4.87±0.35 |
2 | 1.00±0.00 | 2.77±3.10 | 3.07±0.52 | 4.00±0.45 | 4.83±0.46 | 3.13±0.57 | 4.07±0.45 | 4.90±0.31 | |
Average* | 1.00±0.00 | 2.85±0.44 | 3.03±0.55 | 3.98±0.43 | 4.82±0.43 | 3.05±0.53 | 4.03±0.45 | 4.88±0.32 | |
Image texture and sharpness κ value =0.802 | 1 | 1.07±0.25 | 1.83±0.53 | 3.10±0.48 | 1.03±0.18 | 1.00±0.00 | 3.13±0.57 | 4.60±0.50 | 2.90±0.61 |
2 | 1.07±0.25 | 1.80±0.48 | 3.03±0.49 | 1.10±0.31 | 1.03±0.18 | 3.10±0.55 | 4.70±0.47 | 2.83±0.59 | |
Average* | 1.07±0.25 | 1.82±0.50 | 3.07±0.48 | 1.07±0.25 | 1.02±0.13 | 3.12±0.56 | 4.65±0.48 | 2.87±0.60 | |
Small vessels visibility κ value =0.809 | 1 | 1.10±0.31 | 1.40±0.50 | 3.10±0.48 | 1.97±0.49 | 1.07±0.25 | 3.17±0.59 | 4.63±0.49 | 2.83±0.38 |
2 | 1.07±0.25 | 1.43±0.51 | 3.03±0.41 | 1.90±0.48 | 1.07±0.25 | 3.13±0.57 | 4.70±0.47 | 2.80±0.41 | |
Average* | 1.08±0.28 | 1.42±0.50 | 3.07±0.46 | 1.93±0.48 | 1.07±0.25 | 3.15±0.58 | 4.67±0.48 | 2.82±0.39 | |
Diagnostic confidence κ value =0.888 | 1 | 1.03±0.18 | 1.87±0.35 | 2.93±0.25 | 1.87±0.35 | 1.07±0.25 | 3.07±0.52 | 4.40±0.50 | 2.77±0.43 |
2 | 1.03±0.18 | 1.90±0.31 | 2.93±0.37 | 1.83±0.38 | 1.07±0.25 | 3.00±0.53 | 4.43±0.51 | 2.80±0.41 | |
Average* | 1.03±0.18 | 1.88±0.32 | 2.93±0.31 | 1.85±0.36 | 1.07±0.25 | 3.03±0.52 | 4.42±0.50 | 2.78±0.42 |
Data are represented as the mean ± standard deviation. *, P≤0.001. FBP, filtered back projection; AV, adaptive statistical iterative reconstruction-Veo; DL-L, deep learning image reconstruction-low; DL-M, deep learning image reconstruction-medium; DL-H, deep learning image reconstruction-high.
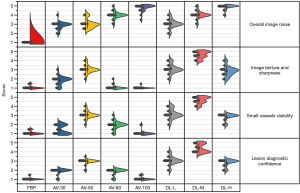
Discussion
DLIR provided better image quality and noise texture compared with FBP and ASiR-V, both in the phantom study and the clinical study. Meanwhile DL-M maintained the best image quality and lesion diagnostic confidence using low-dose radiation CT.
In the phantom study, the NPS average spatial frequency of DL-L was superior to that of ASiR-V and closest to that of FBP when compared with DL-M and DL-H. DL-M and DL-H were superior to AV-80 and AV-100 in average spatial frequency. Although DL-M and DL-H had slightly higher SD values and lower CNR compared to AV-80 and AV-100, respectively, images reconstructed with AV-80 and AV-100 appeared excessively smooth and waxy. “Overly smooth” images can make low-contrast detection tasks challenging. Therefore, subjectively, AV-80 and AV-100 had lower scores compared to DL-M and DL-H. The texture of DLIR was more similar to that of FBP, and DLIR was better able to reduce noise amplitude and maintain texture compared to ASiR-V. The TTF50% values of DLIR were higher than those of FBP, except at 50 mA using the Teflon insert. Compared with FBP, the spatial resolution of low- and high-contrast objects was improved using DLIR. In contrast to the results reported by Franck et al. (34), our findings for TTF50% values of DLIR were slightly higher than those of FBP for both the polystyrene insert and the Teflon insert. The reason for this might be that the placements and selected slices of the ROI were different between our study and that of Franck et al. In general, in contrast to the linear processing methods of FBP, IR adopts nonlinear methods to reconstruct images, which could change the noise texture and reduce spatial resolution to some extent. Moreover, spatial resolution depends on the comparison of surrounding structures and noise levels for both low-contrast lesion diagnosis and low-dose radiation scans. It is difficult for IR to distinguish the real boundary and image noise, and it is unable to maintain a satisfactory spatial resolution (15). Compared with IR, DLIR is also a nonlinear reconstruction algorithm, but it is trained by high-quality FBP data. Therefore, compared to FBP, DLIR produces similar image texture and spatial resolution.
As a mainstream technique, ASiR-V supports low dose scanning because of the reduced noise and improved image quality (35). Our study examined the lowest to the highest blending factors, including AV-0 (FBP), AV-30, AV-50, AV-80, and AV-100, to compare the overall ability of denoising and image-quality between the ASiR-V and DLIR algorithms; meanwhile, the radiation dose was decreased about 55.5% on the portal venous comparing with arterial phase. In their study, Nam et al. reported that DLIR chest CT scans showed similar image quality in the upper abdomen to that of dedicated ASiR abdominal CT with a 50% reduction of the radiation dose (36). Tamura et al. noted that AiCE could reduce the overall radiation dose by more than 40% without affecting image quality compared to routine-dose abdominal CT with adaptive iterative dose reduction 3D, while generating better diagnostic acceptability (27). These results suggest that DLIR can better ensure image quality even under a low dose of radiation.
In general, lower-noise images are desirable, but excessive reconstruction strength may produce undesirable loss of image details. As shown in Figure 4, small vessels become thinner as the DLIR strength increases. In our study, DL-M was better than all other strength reconstructions, except in the areas of overall image noise in the qualitative assessment (P<0.05). Progressively higher strengths of DLIR resulted in minor blurring of small vessels. Figure 5 shows a 69-year-old male with bile duct hamartomas in the liver. Lesions could be detected on every reconstruction strength, but the lesion boundary appeared blurred on the images of DL-H, AV-80, and AV-100. DL-M showed the best lesion morphology and contrast between the lesion and the surrounding tissue. This conclusion is also consistent with the report of Kaga et al. (37). However, in contrast to Kaga et al. (NI =7; reconstruction thickness, 5 mm), we used low dose scanning (NI =15) and 0.625-mm thickness for our reconstructions. The NI settings for a routine abdomen CT in clinical practice are generally 7 to 10 (37). Since the abdomen is mostly a solid organ, a certain dose level is required for the imaging of small lesions. Recent studies have reported that DL-M maintained observer lesion detection for lesions at a low-dose (>0.5 mm) relative to standard-dose FBP (25). Jensen et al. compared DL-M with FBP and AV-60 (20) and found DL-M to be better suited to abdominal imaging since tiny liver lesions and vessels become blurred on images with excessive strength DLIR and omissions may arise. These findings are consistent with those of the low-dose abdominal CT scans in our study. This is in contrast to the result of Cao et al., who compared DL-H with ASiR-V 50% at a 1.25-mm reconstruction thickness using extremely low-dose radiation (NI =24). They found that DLIR-H could facilitate a 76% reduction of radiation dose, provide clinically acceptable quality and diagnostic confidence, and maintain image texture (38). As a new reconstruction method, clinical research of DLIR warrants further investigation in the future.
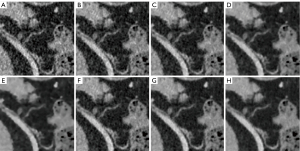
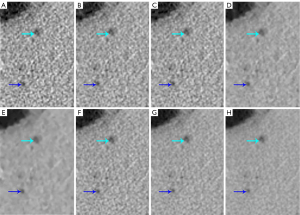
This study had several limitations. First, the study population was relatively small, and only abdominal data were reconstructed via a single manufacturer. The feasibility of DL-based algorithms with other manufacturers and for other organs needs further verification. Second, only a few types of lesions were examined, and most were cysts, which are clear compared with normal tissues. Moreover, we only qualitatively evaluated the lesion diagnostic confidence but did not quantitatively assess lesion detectability, and pathological confirmation was lacking. Future studies should examine the detection ability of low-contrast lesions. Third, we only selected the portal venous phase for image quality comparison. Subsequent research should compare the arterial phase with the portal venous phase quantitatively and qualitatively using the ASiR-V and DLIR algorithms.
Conclusions
DLIR provided better image quality and noise texture compared to ASiR-V both in the phantom and clinical study. The images of DLI-M represented the best balance between image quality and lesion diagnostic confidence in low-dose radiation CT scanning of the abdomen.
Acknowledgments
Funding: This work was supported by the Technology Development Plan of Shandong Province (grant No. 2014GSF118091) and the Shandong Medical and Health Science and Technology Development Plan (grant No. 2017WS715).
Footnote
Conflicts of Interest: All authors have completed the ICMJE uniform disclosure form (available at https://qims.amegroups.com/article/view/10.21037/qims-22-1227/coif). LL is an employee of GE Healthcare, the manufacturer of the CT system used in this study. The other authors have no conflicts of interest to declare.
Ethical Statement: The authors are accountable for all aspects of the work in ensuring that questions related to the accuracy or integrity of any part of the work are appropriately investigated and resolved. This study was conducted in accordance with the Declaration of Helsinki (as revised in 2013). The prospective study from August to December 2020 was approved by the local institutional review board of the First Affiliated Hospital of Shandong First Medical University, and all participants signed written informed consent.
Open Access Statement: This is an Open Access article distributed in accordance with the Creative Commons Attribution-NonCommercial-NoDerivs 4.0 International License (CC BY-NC-ND 4.0), which permits the non-commercial replication and distribution of the article with the strict proviso that no changes or edits are made and the original work is properly cited (including links to both the formal publication through the relevant DOI and the license). See: https://creativecommons.org/licenses/by-nc-nd/4.0/.
References
- Hong JY, Han K, Jung JH, Kim JS. Association of Exposure to Diagnostic Low-Dose Ionizing Radiation With Risk of Cancer Among Youths in South Korea. JAMA Netw Open 2019;2:e1910584. [Crossref] [PubMed]
- Lurz M, Lell MM, Wuest W, Eller A, Scharf M, Uder M, May MS. Automated tube voltage selection in thoracoabdominal computed tomography at high pitch using a third-generation dual-source scanner: image quality and radiation dose performance. Invest Radiol 2015;50:352-60. [Crossref] [PubMed]
- Greffier J, Pereira F, Macri F, Beregi JP, Larbi A. CT dose reduction using Automatic Exposure Control and iterative reconstruction: A chest paediatric phantoms study. Phys Med 2016;32:582-9. [Crossref] [PubMed]
- Fält T, Söderberg M, Hörberg L, Christoffersen C, Lång K, Abul-Kasim K, Leander P. Simulated Dose Reduction for Abdominal CT With Filtered Back Projection Technique: Effect on Liver Lesion Detection and Characterization. AJR Am J Roentgenol 2019;212:84-93. [Crossref] [PubMed]
- Willemink MJ, Noël PB. The evolution of image reconstruction for CT-from filtered back projection to artificial intelligence. Eur Radiol 2019;29:2185-95. [Crossref] [PubMed]
- Thibault JB, Sauer KD, Bouman CA, Hsieh J. A three-dimensional statistical approach to improved image quality for multislice helical CT. Med Phys 2007;34:4526-44. [Crossref] [PubMed]
- Li W, Zhang CQ, Li AY, Deng K, Shi H. Preliminary study of dose reduction and image quality of adult pelvic low-dose CT scan with adaptive statistical iterative reconstruction. Acta Radiol 2015;56:1222-9. [Crossref] [PubMed]
- Goodenberger MH, Wagner-Bartak NA, Gupta S, Liu X, Yap RQ, Sun J, Tamm EP, Jensen CT. Computed Tomography Image Quality Evaluation of a New Iterative Reconstruction Algorithm in the Abdomen (Adaptive Statistical Iterative Reconstruction-V) a Comparison With Model-Based Iterative Reconstruction, Adaptive Statistical Iterative Reconstruction, and Filtered Back Projection Reconstructions. J Comput Assist Tomogr 2018;42:184-90. [Crossref] [PubMed]
- Jensen CT, Telesmanich ME, Wagner-Bartak NA, Liu X, Rong J, Szklaruk J, Qayyum A, Wei W, Chandler AG, Tamm EP. Evaluation of Abdominal Computed Tomography Image Quality Using a New Version of Vendor-Specific Model-Based Iterative Reconstruction. J Comput Assist Tomogr 2017;41:67-74. [Crossref] [PubMed]
- Telesmanich ME, Jensen CT, Enriquez JL, Wagner-Bartak NA, Liu X, Le O, Wei W, Chandler AG, Tamm EP. Third version of vendor-specific model-based iterativereconstruction (Veo 3.0): evaluation of CT image quality in the abdomen using new noise reduction presets and varied slice optimization. Br J Radiol 2017;90:20170188. [Crossref] [PubMed]
- Lim K, Kwon H, Cho J, Oh J, Yoon S, Kang M, Ha D, Lee J, Kang E. Initial phantom study comparing image quality in computed tomography using adaptive statistical iterative reconstruction and new adaptive statistical iterative reconstruction v. J Comput Assist Tomogr 2015;39:443-8. [Crossref] [PubMed]
- Chen LH, Jin C, Li JY, Wang GL, Jia YJ, Duan HF, Pan N, Guo J. Image quality comparison of two adaptive statistical iterative reconstruction (ASiR, ASiR-V) algorithms and filtered back projection in routine liver CT. Br J Radiol 2018;91:20170655. [Crossref] [PubMed]
- Samei E, Richard S. Assessment of the dose reduction potential of a model-based iterative reconstruction algorithm using a task-based performance metrology. Med Phys 2015;42:314-23. [Crossref] [PubMed]
- Geyer LL, Schoepf UJ, Meinel FG, Nance JW Jr, Bastarrika G, Leipsic JA, Paul NS, Rengo M, Laghi A, De Cecco CN. State of the Art: Iterative CT Reconstruction Techniques. Radiology 2015;276:339-57. [Crossref] [PubMed]
- Mileto A, Guimaraes LS, McCollough CH, Fletcher JG, Yu L. State of the Art in Abdominal CT: The Limits of Iterative Reconstruction Algorithms. Radiology 2019;293:491-503. [Crossref] [PubMed]
- Mileto A, Zamora DA, Alessio AM, Pereira C, Liu J, Bhargava P, et al. CT Detectability of Small Low-Contrast Hypoattenuating Focal Lesions: Iterative Reconstructions versus Filtered Back Projection. Radiology 2018;289:443-54. [Crossref] [PubMed]
- Greffier J, Frandon J, Pereira F, Hamard A, Beregi JP, Larbi A, Omoumi P. Optimization of radiation dose for CT detection of lytic and sclerotic bone lesions: a phantom study. Eur Radiol 2020;30:1075-8. [Crossref] [PubMed]
- Greffier J, Frandon J, Larbi A, Beregi JP, Pereira F. CT iterative reconstruction algorithms: a task-based image quality assessment. Eur Radiol 2020;30:487-500. [Crossref] [PubMed]
- Benz DC, Benetos G, Rampidis G, von Felten E, Bakula A, Sustar A, Kudura K, Messerli M, Fuchs TA, Gebhard C, Pazhenkottil AP, Kaufmann PA, Buechel RR. Validation of deep-learning image reconstruction for coronary computed tomography angiography: Impact on noise, image quality and diagnostic accuracy. J Cardiovasc Comput Tomogr 2020;14:444-51. [Crossref] [PubMed]
- Jensen CT, Liu X, Tamm EP, Chandler AG, Sun J, Morani AC, Javadi S, Wagner-Bartak NA. Image Quality Assessment of Abdominal CT by Use of New Deep Learning Image Reconstruction: Initial Experience. AJR Am J Roentgenol 2020;215:50-7. [Crossref] [PubMed]
- Park C, Choo KS, Jung Y, Jeong HS, Hwang JY, Yun MS. CT iterative vs deep learning reconstruction: comparison of noise and sharpness. Eur Radiol 2021;31:3156-64. [Crossref] [PubMed]
- Zeng L, Xu X, Zeng W, Peng W, Zhang J, Sixian H, Liu K, Xia C, Li Z. Deep learning trained algorithm maintains the quality of half-dose contrast-enhanced liver computed tomography images: Comparison with hybrid iterative reconstruction: Study for the application of deep learning noise reduction technology in low dose. Eur J Radiol 2021;135:109487. [Crossref] [PubMed]
- Jiang B, Li N, Shi X, Zhang S, Li J, de Bock GH, Vliegenthart R, Xie X. Deep Learning Reconstruction Shows Better Lung Nodule Detection for Ultra-Low-Dose Chest CT. Radiology 2022;303:202-12. [Crossref] [PubMed]
- Li W, Diao K, Wen Y, Shuai T, You Y, Zhao J, Liao K, Lu C, Yu J, He Y, Li Z. High-strength deep learning image reconstruction in coronary CT angiography at 70-kVp tube voltage significantly improves image quality and reduces both radiation and contrast doses. Eur Radiol 2022;32:2912-20. [Crossref] [PubMed]
- Jensen CT, Gupta S, Saleh MM, Liu X, Wong VK, Salem U, Qiao W, Samei E, Wagner-Bartak NA. Reduced-Dose Deep Learning Reconstruction for Abdominal CT of Liver Metastases. Radiology 2022;303:90-8. [Crossref] [PubMed]
- Tatsugami F, Higaki T, Nakamura Y, Yu Z, Zhou J, Lu Y, Fujioka C, Kitagawa T, Kihara Y, Iida M, Awai K. Deep learning-based image restoration algorithm for coronary CT angiography. Eur Radiol 2019;29:5322-9. [Crossref] [PubMed]
- Tamura A, Mukaida E, Ota Y, Nakamura I, Arakita K, Yoshioka K. Deep learning reconstruction allows low-dose imaging while maintaining image quality: comparison of deep learning reconstruction and hybrid iterative reconstruction in contrast-enhanced abdominal CT. Quant Imaging Med Surg 2022;12:2977-84. [Crossref] [PubMed]
- Tamura A, Mukaida E, Ota Y, Kamata M, Abe S, Yoshioka K. Superior objective and subjective image quality of deep learning reconstruction for low-dose abdominal CT imaging in comparison with model-based iterative reconstruction and filtered back projection. Br J Radiol 2021;94:20201357. [Crossref] [PubMed]
- Kijewski MF, Judy PF. The noise power spectrum of CT images. Phys Med Biol 1987;32:565-75. [Crossref] [PubMed]
- Samei E, Bakalyar D, Boedeker KL, Brady S, Fan J, Leng S, Myers KJ, Popescu LM, Ramirez Giraldo JC, Ranallo F, Solomon J, Vaishnav J, Wang J. Performance evaluation of computed tomography systems: Summary of AAPM Task Group 233. Med Phys 2019;46:e735-56. [Crossref] [PubMed]
- Boedeker KL, Cooper VN, McNitt-Gray MF. Application of the noise power spectrum in modern diagnostic MDCT: part I. Measurement of noise power spectra and noise equivalent quanta. Phys Med Biol 2007;52:4027-46. [Crossref] [PubMed]
- Richard S, Husarik DB, Yadava G, Murphy SN, Samei E. Towards task-based assessment of CT performance: system and object MTF across different reconstruction algorithms. Med Phys 2012;39:4115-22. [Crossref] [PubMed]
- Lee S, Kwon H, Cho J. The Detection of Focal Liver Lesions Using Abdominal CT: A Comparison of Image Quality Between Adaptive Statistical Iterative Reconstruction V and Adaptive Statistical Iterative Reconstruction. Acad Radiol 2016;23:1532-8. [Crossref] [PubMed]
- Franck C, Zhang G, Deak P, Zanca F. Preserving image texture while reducing radiation dose with a deep learning image reconstruction algorithm in chest CT: A phantom study. Phys Med 2021;81:86-93. [Crossref] [PubMed]
- Ye K, Chen M, Zhu Q, Lu Y, Yuan H. Effect of adaptive statistical iterative reconstruction-V (ASiR-V) levels on ultra-low-dose CT radiomics quantification in pulmonary nodules. Quant Imaging Med Surg 2021;11:2344-53. [Crossref] [PubMed]
- Nam JG, Hong JH, Kim DS, Oh J, Goo JM. Deep learning reconstruction for contrast-enhanced CT of the upper abdomen: similar image quality with lower radiation dose in direct comparison with iterative reconstruction. Eur Radiol 2021;31:5533-43. [Crossref] [PubMed]
- Kaga T, Noda Y, Fujimoto K, Suto T, Kawai N, Miyoshi T, Hyodo F, Matsuo M. Deep-learning-based image reconstruction in dynamic contrast-enhanced abdominal CT: image quality and lesion detection among reconstruction strength levels. Clin Radiol 2021;76:710.e15-24. [Crossref] [PubMed]
- Cao L, Liu X, Li J, Qu T, Chen L, Cheng Y, Hu J, Sun J, Guo J. A study of using a deep learning image reconstruction to improve the image quality of extremely low-dose contrast-enhanced abdominal CT for patients with hepatic lesions. Br J Radiol 2021;94:20201086. [Crossref] [PubMed]