Artificial intelligence with a deep learning network for the quantification and distinction of functional adrenal tumors based on contrast-enhanced CT images
Introduction
The incidence of adrenal incidentaloma has increased due to the advancement of imaging techniques and the popularization of chest computed tomography (CT) scans for physical examination (1,2). Although up to 80% of these lesions are benign and inert, there are still many functional tumors that cause hormonal overproduction of cortisol, aldosterone, catecholamine, or metanephrine. Histologically, adrenal adenoma is the most common type of adrenal incidentaloma (accounting for 41–52% of cases), followed by metastatic tumor (19%), myelolipoma (9%), and pheochromocytoma (PC; 8%) (1,3). Although their incidence rates are higher than that of PC, metastatic tumors and myelolipoma are rarely characterized by the overproduction of hormones, so consider from the functionality of adrenal tumor the Hyperaldosteronism (HA), Cushing’s syndrome (CS), and PC are the most common types among adrenal mass (4). Excessive hormone production results in various clinical symptoms, such as hypertension, electrolyte disorder, obesity, and harm to the human body. In some cases, excessive hormones may even result in mortality. Therefore, for these functional adrenal tumors (FATs), timely intervention is necessary. However, limited medical resources and a lack of clinical experience can lead to misdiagnosis of FATs. Furthermore, inappropriate preoperative evaluation may cause serious intraoperative and postoperative complications, such as adrenergic storm, cortisol deficiency, and related cardiovascular complications.
To diagnose FATs, image analysis and a detailed endocrine hormone workup combined with the assessment of clinical symptoms are required in every case (4). However, the assessment of hormones, such as adrenocorticotropic hormone, aldosterone, renin, the aldosterone/renin ratio, normetanephrine, and metanephrine, is expensive and time-consuming. Moreover, it needs to be performed by a professional doctor, requires special preparation and equipment, and can be easily affected by other factors, such as some foods and medicines (3,5). However, to our knowledge, different types of adrenal tumors have specific imaging characteristics that can be used to assist in the preliminary diagnosis and treatment. A method that can determine the functionality of adrenal lesions by CT texture analysis would assist physicians in the preliminary diagnosis and timely and accurate treatment of FATs.
Researchers have evaluated the reliability of radiological parameters of CT images in predicting the functionality of hormonally active tumors. Previous studies have assessed the function of these tumors through different parameters, such as the native attenuation value in Hounsfield units (HU), maximum diameter, absolute percentage washout (which refers to the characteristics of contrast media enhancement and de-enhancement), and heterogeneity (2,6,7). Recent developments in artificial intelligence (AI), especially deep learning, have been promoted extensively for application in a various quantification and distinction tasks of lesions in contrast CT imaging. Great progress has been achieved in applying deep convolutional neural networks (DCNNs) in the field of medicine (8-11), especially in CT imaging. These networks are widely used in the diagnosis of pulmonary diseases. Various diagnostic models have been developed that use CT in combination with deep learning networks, including for the diagnosis of COVID-19 (12,13). Xie et al. (14) proposed a multiview knowledge-based collaborative deep model to separate malignant from benign nodules using limited chest CT data.
In this study, we developed an automated framework for image-level FATs distinction and pixel-level tumor lesion quantification based on enhanced CT images. The purpose of the model is to assist physicians in the primary diagnosis of FATs and in selecting further tests for the final diagnosis. The proposed AI-based model with a deep learning network was trained on a dataset consisting of HA, CS, and PC cases. We validated its diagnostic performance with that of clinical diagnosis by physicians in extensive experiments. We present the following article in accordance with the TRIPOD reporting checklist (available at https://qims.amegroups.com/article/view/10.21037/qims-22-539/rc).
Methods
The study was conducted in accordance with the Declaration of Helsinki (as revised in 2013). The study was approved by the institutional ethics board of Ruijin Hospital, Shanghai Jiao Tong University, School of Medicine, Shanghai, China, and informed consent was obtained from all the patients.
Dataset and annotations
In this study, we screened the clinical and imaging data of patients who underwent adrenalectomy in our clinical center within the year from March 2015 to June 2020. The CT imaging data of 375 useful HA, CS, and PC cases were selected and randomly divided into three data sets: the training set (270 cases), the testing set (60 cases), and the retrospective trial set (45 cases). The specific information of the cases and the three data sets is listed in Table 1 and Table 2, respectively. Patients who did not undergo enhanced CT perioperatively or whose tumors were not diagnosed as HA, CS, or PC by endocrine tests and postoperative pathology were excluded. In this study, the adrenal lesions in the CT scans of the patients were observed, and all of them showed excessive hormone secretion. For the diagnosis of HA, patients were tested for aldosterone, renin, angiotensin, and blood potassium, and screened by the aldosterone–renin ratio (ARR). If the ARR was greater than 30, the patient underwent a further confirmatory test, such as a saline infusion test, and then adrenal venous sampling to identify the dominant side. Patients with CS showed high cortisol, low adreno-cortico-tropic-hormone (ACTH) levels, and a disappeared circadian rhythm of cortisol. The final diagnosis was made with the help of a dexamethasone suppression test combined with CT image and clinical symptoms assessments. For the diagnosis of PC, patients’ levels of normetanephrine and metanephrine were tested. All selected cases were confirmed by postoperative biology.
Table 1
HA | PC | CS | |
---|---|---|---|
Median age (years) (IQR) | 43 [36–51] | 49 [40–57] | 47 [37–57] |
Male, n (%) | 65 (59.6) | 68 (47.9) | 43 (34.7) |
Female, n (%) | 44 (40.3) | 74 (52.1) | 81 (65.3) |
No. of patients (%) | 109 (29.1) | 142 (37.9) | 124 (33.0) |
Median largest diameter of tumor (cm) (IQR) | 1.8 (1.4–2.3) | 4.1 (3.2–4.7) | 2.8 (2.1–3.2) |
IQR, interquartile range; BMI, body mass index; HA, hyperaldosteronism; CS, Cushing’s syndrome; PC, pheochromocytoma.
Table 2
HA | PC | CS | |
---|---|---|---|
All | 109 (29.1%) | 142 (37.9%) | 124 (33.0%) |
Training | 75 | 108 | 87 |
Testing | 19 | 18 | 23 |
Retrospective trial | 15 | 16 | 14 |
HA, hyperaldosteronism; CS, Cushing’s syndrome; PC, pheochromocytoma.
Each CT scan consisted of three phases: the arterial phase, venous phase, and unenhanced phase. For the unenhanced and contrast-enhanced CT examinations, a slice thickness of 2.5–5 mm was used, iodine contrast material was injected intravenously using a power injector for 40 s at a rate of 3.0 mL/s, and arterial and venous phase scans were performed 25–30 and 60–70 s respectively after the injection of the contrast material. All adrenal tumors in the training data set were labeled on the CT images manually. The model recognized and learned the CT image texture characteristics of adrenal tumors for quantification and distinction through the deep learning network.
Annotations for model training were first acquired by a junior physician who labeled along the outer contours of the tumor and the adrenal regions in all slices of the CT images. The coordinates (x, y) of the outer contours were saved as EXtensible Markup Language (XML) format files with an ordered list. These annotations were then confirmed and modified by a senior physician.
Algorithm development and implementation
The input images had to be preprocessed before being fed into the network. To ensure the same shape and voxel spacing of each phase, the arterial phase and nonenhanced phase were registered to the venous phase. Moreover, voxel values were normalized (z score normalization) to the mean and standard deviation of all voxels.
The workflow of our algorithm is presented in Figure 1 and contains three stages: kidney segmentation, adrenal and tumor segmentation, and refined segmentation and classification.
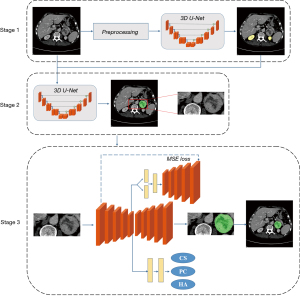
In stage one, a three-dimensional (3D) U-Net, consisting of an encoder and a decoder, is employed to obtain the location of the kidneys and is further used as guidance to locate the adrenals and tumors. The encoder contains four down-sampling operations and constructs four feature maps, which are later used in the decoder part via skip connections. The decoder up-samples from the latent variables (the deepest feature maps in the network) and concatenates them with previous encoder feature maps. In stage two, the concatenation of the segmented kidney masks and original CT series are fed into another 3D U-Net for rough segmentation of the adrenals and tumor. The loss function of the models in the first two stages is as follows:
where Lce is the cross entropy loss function and Ldc is the dice loss function of the multiclass (15); x and y represent the one-hot encoding segmentation map of model’s output and ground truth, respectively; I represents the pixel set of the segmentation map; and C is the class set.
The input of the third stage is a bounding box, consisting of the adrenals and tumors cropped from each whole CT scan. We selected the same encoder architecture as that used in the first two stages for feature extraction. The decoder part consists of a classification branch and a segmentation branch to identify the tumor types and refine tumor segmentation. A variational autoencoder (VAE) branch was adopted to improve the segmentation accuracy. A VAE can perform efficient approximate inferences and can learn with directed probabilistic models whose continuous latent variables have intractable posterior distributions (16). In the VAE branch, the latent variables are assumed to follow a probability distribution. Through a fully connected layer, the latent variables construct a mean vector (1024-d) and a standard deviation vector (1024-d). These two vectors are compared to the mean and the standard deviation of the normal distribution. Kullback-Leibler (KL) divergence is used in this comparison. In stage three, the loss function is divided into three parts:
where Lclsrepresents the cross-entropy loss function for the classification branch, and Lvae represents the loss function for the VAE branch. LKL is the KL divergence, which is defined as the difference between two probability distributions. Since the probability distribution of latent variables in the VAE is assumed to be a Gaussian distribution, μI and σI are the parameters of the Gaussian distribution. LMSE represents the mean-squared loss between the input image and the output of the VAE branch.
In our research, all CT images were acquired in the arterial phase, venous phase, and nonenhanced phase. The data distribution of the training set, which was used to optimize network parameters, is shown in Table 2. We used the Adam optimizer with a learning rate of 0.001 to update the parameters of the network. The deep learning model was trained on an NVIDIA Tesla V100 GPU. To prevent network overfitting, early stopping was performed, and we chose the model in which loss did not decrease over 50 iterations as the final model.
Model performance assessment
For image-level FAT distinction, a testing set of 60 CT images from 60 patients was used to compare our the performance of our model with the diagnostic results of a detailed endocrine hormone assessment. Then, to assess the robustness of the proposed model, a further 45 independently collected cases were tested in a retrospective dataset. The dataset consisted of 14 CS cases (31.1%), 15 HA cases (33.3%), and 16 PC cases (35.6%). The evaluation indices were receiver operating characteristic (ROC) curves and a confusion matrix.
For pixel-level tumor lesion quantification, we considered the results of adrenal tumor segmentation as quantitative representation and evaluated the segmentation performance in terms of the Dice score. The venous phase was regarded as the template during registration, so the annotated masks were obtained from venous-phase images.
Finally, we selected 40 CT scans from 60 patients in the testing group to assess how the AI model improved the diagnostic accuracy of physicians. The assistance of AI was provided with image-level functional FAT distinction and pixel-level tumor lesion segmentation. Four physicians (with 10, 4, 2, and 1 years of experience, respectively) were involved in the study. Forty CT scans were randomly divided into two groups of 20 scans: group A and group B. To remove observer bias, group A used AI model assistance, while group B was without assistance in the first round of reviews. In the second round of reviews, the AI assistance was switched between group A and group B. There was an interval of 4 weeks between the two rounds of reviewing to eliminate the interference of the first review. We get two groups of data, one is the result of diagnostic accuracy that the physician quantifies the tumor without AI assistance, the other group is the diagnostic accuracy with AI assistance. We called this experiment an observer study.
Results
Assessment of the model distinction performance
The ROC curves and confusion matrices for image-level FAT distinction in the testing set are illustrated in Figure 2A. The area under the ROC curve (AUC) is one of the most common evaluation indicators of model performance. The proposed deep learning model achieved an average AUC of 0.915, and all the AUCs for the three types of FAT were greater than 0.882. As presented in the confusion matrix, almost all cases of CS were identified, and the results for PC and HA were also satisfactory. The AUC of the model tested on the retrospective dataset reached above 0.849 (Figure 2B). More specifically, the distinction performance for HA and PC was similar to that of the testing data, and the distinction performance for CS was significantly reduced (Figure 2C).
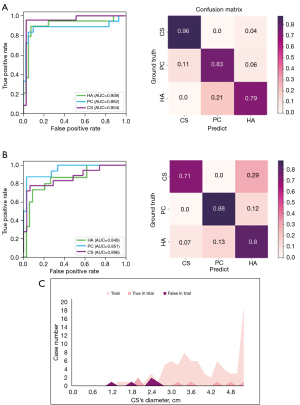
To visually explain the relationship between the proposed model and the distinction results, heatmaps were used to show which part of the input images had played a role in the final distinction outputs. These heatmaps are presented in Figure 3. The region of interest highlighted in a heatmap was the region predicted by the model to be a potential tumor lesion. The regions of interest were mainly concentrated in the tumor lesions, which indicated that the proposed model could distinguish the type of FAT in an explainable way and facilitate tumor segmentation.
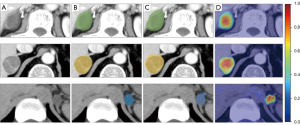
Assessment of model quantification performance
The model’s quantification performance was assessed in the testing dataset, and it achieved a mean Dice coefficient of 0.69. Although this result indicated that there was room to improve the segmentation accuracy, Figure 4, which presents the detailed segmentation results for the three types of FAT, shows that the tumor lesion regions segmented by the proposed model were generally accurate.
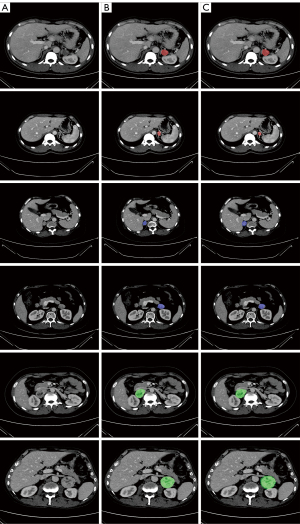
Observer study
To evaluate whether our proposed model could assist in the diagnosis of FATs by physicians, four physicians with different levels of experience and 40 CT scans with three phases from the test dataset were involved in the next part of the study. The results of the physicians’ diagnoses with and without AI assistance are depicted in Figure 5. The diagnostic accuracies for all four physicians were higher with AI assistance than without. The average number of cases correctly diagnosed without AI was 32.3 (average accuracy rate: 80.6%), while the average number of cases diagnosed with the assistance of AI was 37.0 (average accuracy rate: 92.5%). The difference was statistically significant (P<0.05). The confusion matrices in Figure 2 are presented to demonstrate the diagnostic performance for the three types of FAT in detail. Figure 5B shows the relationship between diagnostic accuracy and the diameter and average CT value of the tumor.
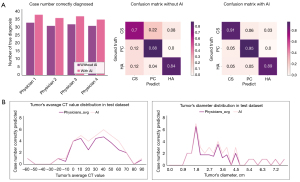
Discussion
The quantification and distinction of adrenal tumors are key to their treatment, especially for FATs, which can cause overproduction of various endocrine hormones. Failure to differentiate FATs may cause various crises. For example, misdiagnosis of PC during preoperative examination can lead to unexpected blood pressure fluctuations, which can cause serious cardiovascular complications during surgery. In some cases, the surgical intervention may stimulate the tumor, resulting in excessive secretion of catecholamine, which poses a threat to the patient’s life. For HA and CS, timely monitoring of cortical hormones and electrolytes may be delayed, leading to corticosteroid deficiency or other serious complications. In some cases, patients with nonfunctional adrenal lesions can be wrongly diagnosed and exposed to unnecessary surgery (17). Therefore, accurate diagnosis and timely intervention are vital. In this study, we established a novel diagnostic model with a deep learning network to quantify and distinguish common FATs based on enhanced CT.
Computed tomography is one of the most common tools for diagnosing adrenal tumors with different radiological features (18). In the field of adrenal tumors, several methods have been proposed to assess the benignity and functionality of adrenal lesions using different CT parameters, such as the tumor maximum diameter, attenuation value in HU, regular margins, and absolute percentage washout (3,17,19,20). Most adrenal adenomas are rich in lipids, which results in them having low attenuation values on plain CT images; a threshold attenuation value lower than 10 HU has been shown to distinguish adrenal adenomas with high accuracy (17). Siegal et al. reported that most (79%) cortisol-producing adrenal incidentalomas had an HU value of greater than 10 (21). Another study showed that the patients with CS had an HU value of greater than 18.5. The authors explained that the reason for this high HU value was reduced levels of intracytoplasmic fat, which caused a change in the structure of cortisol-producing cells from lipid-rich cells to lipid-poor cells (6). Korobkin et al. (22) reported that using a threshold value of 18 HU correctly diagnosed all nonadenomas, including PCs, in 124 patients who underwent contrast-enhanced CT. Computed tomography has high sensitivity for adrenal adenomas of less than 3 cm in size; however, its sensitivity is poor for large adenomas due to the broad overlap of the tumor size, cystic or necrotic change, and tumor margin (20,23,24).
In the observer study, results showed that our proposed model improved the physicians’ diagnoses by an average of 11.9%. The two groups of data showed statistical differences, which demonstrates the effectiveness of the model in assisting physicians. Physicians mainly diagnose the type of adrenal tumor based on the attenuation value and diameter of the tumor area. To find the internal relationship between images and adrenal tumor classifications, the AI-based system proposed in this paper uses multiple levels of features extracted from the deep learning model architecture. These features include apparent features, such as textures, and many uninterpretable potential features, which will help physicians to make an accurate diagnosis.
Some previous studies have attempted to correlate the radiological features and functionality of adrenal incidentalomas. Yi et al. (3) reported that machine learning-based unenhanced CT texture analysis could effectively differentiate subclinical PCs from lipid-poor adrenal adenomas. They analyzed unenhanced CT images of 80 lipid-poor adrenal adenomas and classified subclinical PCs with an accuracy of 80%. In recent years, many studies in the field of CT have achieved promising results based on deep learning networks (25). For example, Wang et al. (26) proposed a data-driven model, the central focused convolutional neural network, to segment lung nodules from heterogeneous CT images. Another effective model-based approach was used for computer-aided kidney segmentation of abdominal CT images (27). In addition, Chilamkurthy et al. (28) developed a set of deep learning algorithms for the automated detection of key lesions in CT scans, and Man et al. (29) introduced a deep Q network-driven approach with a deformable U-Net to accurately segment the pancreas. Nevertheless, studies on the use of deep learning networks for CT-based FAT quantification are rare. The main approach for FAT distinction mentioned in previous studies is machine learning-based radiomics analysis, which requires human participation in feature selection.
The algorithm described in this study uses nnU-Net as a backbone and combines a VAE branch to extract more useful information to improve segmentation and classification results. Compared to prior art network configurations, nnU-Net encodes precise spatial information into high-resolution low-level features and builds hierarchical object concepts at multiple scales. Furthermore, the VAE branch ensures that our proposed model is very efficient, by creating information-rich latent variables and improving generalization. These operations provide efficient guidance for classification and segmentation. Currently, our model can analyze different image characteristics through the deep learning network. The model segments the CT images of adrenal tumors and extracts the image characteristics of each part in each phase. Unlike those in previous studies, our model extracts some image features that cannot be directly recognized by radiologists and surgeons. As shown in Figure 4B, the accuracy of the model is significantly higher than distinction by physicians alone for FATs with a diameter of 1.8–4.6 cm and an average CT value of 0–70 HU. We have attributed this finding to the fact that the model can extract a greater abundance of features as well as the average CT value and diameter.
In the retrospective dataset, the distinction performance of the model for CS was relatively lower than that in the testing group. Figure 2C demonstrates the difference in the CS tumor diameter distribution between the training dataset and the retrospective trial dataset. The CS tumor diameter in the trainset was 2.1–5.1 cm, while that in the retrospective trial dataset was 1.0–5.1 cm. Previous study has shown that the mean size of most adrenal adenomas is 2.1–2.4 cm, with only a small number of adenomas having a diameter of 4.1–6.0 cm (20). In this study, the median tumor diameter was 1.8 [interquartile range (IQR), 1.4–2.3 cm] and 2.8 (IQR, 2.1–3.2 cm) for HA and CS, respectively. Previous studies and our experience show that when in the diameter range of 1–3 cm, HA and CS have more overlapping imaging features than other diameter sections of these two types of tumors (18,20,24). In addition, as shown in Figure 2C, tumors with sizes ranging from 1.0 to 2.4 cm hardly appeared in the training set, which resulted in a poor performance of the model in this group.
Compared with adrenal adenoma, PC is a hypervascular tumor that results in an increased attenuation value on unenhanced CT. If an adrenal lesion has an attenuation value of less than 10 HU and rapid washout of more than 50%, the tumor is likely to be a benign adenoma. In contrast, a lesion with an HU value of more than 20 and with rapid washout of less than 50% is considered to be PC, adrenal carcinoma, or metastasis (1,30). Studies have reported that 87% to 100% of patients with PC have a CT value of more than 10 HU and an absolute percentage washout of less than 60% (1,6,31). Al-Waeli et al. (6) analyzed the CT characteristics of 38 patients with adrenal incidentaloma. They mentioned that native HU was the most significant radiological parameter for predicting the functionality of CS and PC, and that the maximum diameter was significant for the differentiation of PC and HA.
In this study, the accuracy of the model for CS in the testing data set was 83.3%. Two cases of PC were misdiagnosed as CS, and one was misdiagnosed as HA. The diameter of these tumors was less than 3.0 cm, and the average CT value in the plain scan was less than 20 HU. After enhancement, tumors showed mild uniform enhancement. These characteristics are similar to those of adrenal adenoma. Currently, the model is in the preliminary stage of learning the image characteristics of tumors. Some tumors have CT characteristics that overlap with those of other types of tumors and can easily be misdiagnosed. However, patients with CS have specific clinical signs and symptoms, such as moon face, buffalo hump, and hypertension; patients with HA may have hypokalemia and hypertension; and patients with PC may experience palpitations, sweating, and hypertension. In the follow-up study, we will include these parameters to provide more assistance and improve the diagnostic accuracy of our model.
In terms of its practical value, the diagnostic model with a deep learning network cannot replace endocrine tests and experiments, which are essential for a definitive diagnosis of the functionality of adrenal tumors. We aimed to establish a complete model for the distinction and quantification of adrenal tumors that could assist physicians in making a primary diagnosis of the functionality of these lesions and improve the value of adrenal CT imaging. However, there are still some limitations to our study. First, the amount of data used to train the model was limited, and there were many features of adrenal tumors that were not learned. More abundant data, such as clinical symptoms, are still needed to improve the diagnostic accuracy and robustness of the model. Second, our system can only analyze and differentiate the three common types of FATs. However, other types of adrenal tumors have high incidence rates, such as nonfunctional adrenal adenoma. In the follow-up research, we will increase the numbers of cases of nonfunctional tumors and adrenal cortical carcinoma to make the model more complete.
Conclusions
In this study, we established an AI-based model for differentiating the three most common types of FAT by analyzing contrast CT images. Our model demonstrated a satisfactory performance in quantifying and distinguishing the three common types of FAT. It could automatically locate the tumor and analyze the tumor functionality. The model assisted the diagnosis of surgeons by providing AI analysis. This is the initial stage of our work; in the future, we will be working to develop a more complete AI-based model that can diagnose more types of adrenal tumor without endocrine tests. In the meantime, the model we have developed can be used to quantify and distinguish adrenal tumors under the conditions of limited clinical resources and experience, and can assist physicians in the diagnostic process.
Acknowledgments
We express our sincere gratitude to the patients and their family members for their participation in this study.
Funding: This research was funded by the National Natural Science Foundation of China (Grant No. 81972494) and the Scientific Research Project of Shanghai Health Committee (Grant No. 202040018).
Footnote
Reporting Checklist: The authors have completed the TRIPOD reporting checklist. Available at https://qims.amegroups.com/article/view/10.21037/qims-22-539/rc
Conflicts of Interest: All authors have completed the ICMJE uniform disclosure form (available at https://qims.amegroups.com/article/view/10.21037/qims-22-539/coif). YH, CX, JW, YC, LY, CL, GX are the employees of “Ping An Healthcare Technology”. The other authors have no conflicts of interest to declare.
Ethical Statement: The authors are accountable for all aspects of the work in ensuring that questions related to the accuracy or integrity of any part of the work are appropriately investigated and resolved. The study was conducted in accordance with the Declaration of Helsinki (as revised in 2013). The study was approved by institutional ethics board of Ruijin Hospital, Shanghai Jiao Tong University, School of Medicine, Shanghai, China, and informed consent was obtained from all the patients.
Open Access Statement: This is an Open Access article distributed in accordance with the Creative Commons Attribution-NonCommercial-NoDerivs 4.0 International License (CC BY-NC-ND 4.0), which permits the non-commercial replication and distribution of the article with the strict proviso that no changes or edits are made and the original work is properly cited (including links to both the formal publication through the relevant DOI and the license). See: https://creativecommons.org/licenses/by-nc-nd/4.0/.
References
- Jason DS, Oltmann SC. Evaluation of an Adrenal Incidentaloma. Surg Clin North Am 2019;99:721-9. [Crossref] [PubMed]
- Zeiger MA, Siegelman SS, Hamrahian AH. Medical and surgical evaluation and treatment of adrenal incidentalomas. J Clin Endocrinol Metab 2011;96:2004-15. [Crossref] [PubMed]
- Yi X, Guan X, Chen C, Zhang Y, Zhang Z, Li M, Liu P, Yu A, Long X, Liu L, Chen BT, Zee C. Adrenal incidentaloma: machine learning-based quantitative texture analysis of unenhanced CT can effectively differentiate sPHEO from lipid-poor adrenal adenoma. J Cancer 2018;9:3577-82. [Crossref] [PubMed]
- Sherlock M, Scarsbrook A, Abbas A, Fraser S, Limumpornpetch P, Dineen R, Stewart PM. Adrenal Incidentaloma. Endocr Rev 2020;41:775-820. [Crossref] [PubMed]
- Umanodan T, Fukukura Y, Kumagae Y, Shindo T, Nakajo M, Takumi K, Nakajo M, Hakamada H, Umanodan A, Yoshiura T. ADC histogram analysis for adrenal tumor histogram analysis of apparent diffusion coefficient in differentiating adrenal adenoma from pheochromocytoma. J Magn Reson Imaging 2017;45:1195-203. [Crossref] [PubMed]
- Al-Waeli DK, Mansour AA, Haddad NS. Reliability of adrenal computed tomography in predicting the functionality of adrenal incidentaloma. Niger Postgrad Med J 2020;27:101-7. [Crossref] [PubMed]
- Fassnacht M, Arlt W, Bancos I, Dralle H, Newell-Price J, Sahdev A, Tabarin A, Terzolo M, Tsagarakis S, Dekkers OM. Management of adrenal incidentalomas: European Society of Endocrinology Clinical Practice Guideline in collaboration with the European Network for the Study of Adrenal Tumors. Eur J Endocrinol 2016;175:G1-G34. [Crossref] [PubMed]
- Litjens G, Kooi T, Bejnordi BE, Setio AAA, Ciompi F, Ghafoorian M, van der Laak JAWM, van Ginneken B, Sánchez CI. A survey on deep learning in medical image analysis. Med Image Anal 2017;42:60-88. [Crossref] [PubMed]
- Milletari F, Navab N, Ahmadi SA. V-Net: Fully Convolutional Neural Networks for Volumetric Medical Image Segmentation. 2016 Fourth International Conference on 3D Vision (3DV), Stanford, CA, USA, 2016:565-71. Available online: https://ieeexplore.ieee.org/document/7785132/authors#authors
- Ronneberger O, Fischer P, Brox T. U-Net: Convolutional Networks for Biomedical Image Segmentation. Available online: https://link.springer.com/chapter/10.1007/978-3-319-24574-4_28
- Schwyzer M, Messerli M, Eberhard M, Skawran S, Martini K, Frauenfelder T. Impact of dose reduction and iterative reconstruction algorithm on the detectability of pulmonary nodules by artificial intelligence. Diagn Interv Imaging 2022;103:273-80. [Crossref] [PubMed]
- Fu BJ, Lv ZM, Lv FJ, Li WJ, Lin RY, Chu ZG. Sensitivity and specificity of computed tomography hypodense sign when differentiating pulmonary inflammatory and malignant mass-like lesions. Quant Imaging Med Surg 2022;12:4435-47. [Crossref] [PubMed]
- Fervers P, Fervers F, Jaiswal A, Rinneburger M, Weisthoff M, Pollmann-Schweckhorst P, Kottlors J, Carolus H, Lennartz S, Maintz D, Shahzad R, Persigehl T. Assessment of COVID-19 lung involvement on computed tomography by deep-learning-, threshold-, and human reader-based approaches-an international, multi-center comparative study. Quant Imaging Med Surg 2022;12:5156-70. [Crossref] [PubMed]
- Xie Y, Xia Y, Zhang J, Song Y, Feng D, Fulham M, Cai W. Knowledge-based Collaborative Deep Learning for Benign-Malignant Lung Nodule Classification on Chest CT. IEEE Trans Med Imaging 2019;38:991-1004. [Crossref] [PubMed]
- Wang S, Zhu Y, Yu L, Chen H, Lin H, Wan X, Fan X, Heng PA. RMDL: Recalibrated multi-instance deep learning for whole slide gastric image classification. Med Image Anal 2019;58:101549. [Crossref] [PubMed]
- Kingma DP, and Welling M. Auto-encoding variational bayes. arXiv.org 2014.
- Nwariaku FE, Champine J, Kim LT, Burkey S, O'keefe G, Snyder WH 3rd. Radiologic characterization of adrenal masses: the role of computed tomography--derived attenuation values. Surgery 2001;130:1068-71. [Crossref] [PubMed]
- Ozturk E, Onur Sildiroglu H, Kantarci M, Doganay S, Güven F, Bozkurt M, Sonmez G, Cinar Basekim C. Computed tomography findings in diseases of the adrenal gland. Wien Klin Wochenschr 2009;121:372-81. [Crossref] [PubMed]
- Lin TY, Goyal P, Girshick R, He K, Dollar P. Focal Loss for Dense Object Detection. IEEE Trans Pattern Anal Mach Intell 2020;42:318-27. [Crossref] [PubMed]
- Park SY, Park BK, Park JJ, Kim CK. CT sensitivities for large (≥3 cm) adrenal adenoma and cortical carcinoma. Abdom Imaging 2015;40:310-7. [Crossref] [PubMed]
- Siegel RL, Miller KD, Jemal A. Cancer statistics, 2015. CA Cancer J Clin 2015;65:5-29. [Crossref] [PubMed]
- Korobkin M, Brodeur FJ, Yutzy GG, Francis IR, Quint LE, Dunnick NR, Kazerooni EA. Differentiation of adrenal adenomas from nonadenomas using CT attenuation values. AJR Am J Roentgenol 1996;166:531-6. [Crossref] [PubMed]
- Caoili EM, Korobkin M, Francis IR, Cohan RH, Dunnick NR. Delayed enhanced CT of lipid-poor adrenal adenomas. AJR Am J Roentgenol 2000;175:1411-5. [Crossref] [PubMed]
- Caoili EM, Korobkin M, Francis IR, Cohan RH, Platt JF, Dunnick NR, Raghupathi KI. Adrenal masses: characterization with combined unenhanced and delayed enhanced CT. Radiology 2002;222:629-33. [Crossref] [PubMed]
- Monshi MMA, Poon J, Chung V. Deep learning in generating radiology reports: A survey. Artif Intell Med 2020;106:101878. [Crossref] [PubMed]
- Wang S, Zhou M, Liu Z, Liu Z, Gu D, Zang Y, Dong D, Gevaert O, Tian J. Central focused convolutional neural networks: Developing a data-driven model for lung nodule segmentation. Med Image Anal 2017;40:172-83. [Crossref] [PubMed]
- Lin DT, Lei CC, Hung SW. Computer-aided kidney segmentation on abdominal CT images. IEEE Trans Inf Technol Biomed 2006;10:59-65. [Crossref] [PubMed]
- Chilamkurthy S, Ghosh R, Tanamala S, Biviji M, Campeau NG, Venugopal VK, Mahajan V, Rao P, Warier P. Deep learning algorithms for detection of critical findings in head CT scans: a retrospective study. Lancet 2018;392:2388-96. [Crossref] [PubMed]
- Man Y, Huang Y, Feng J, Li X, Wu F, Deep Q. Learning Driven CT Pancreas Segmentation With Geometry-Aware U-Net. IEEE Trans Med Imaging 2019;38:1971-80. [Crossref] [PubMed]
- Szolar DH, Korobkin M, Reittner P, Berghold A, Bauernhofer T, Trummer H, Schoellnast H, Preidler KW, Samonigg H. Adrenocortical carcinomas and adrenal pheochromocytomas: mass and enhancement loss evaluation at delayed contrast-enhanced CT. Radiology 2005;234:479-85. [Crossref] [PubMed]
- Lenders JW, Duh QY, Eisenhofer G, Gimenez-Roqueplo AP, Grebe SK, Murad MH, Naruse M, Pacak K, Young WF Jr. Endocrine Society. Pheochromocytoma and paraganglioma: an endocrine society clinical practice guideline. J Clin Endocrinol Metab 2014;99:1915-42. [Crossref] [PubMed]